A decision-support tool for investment analysis of automated oestrus detection technologies in a seasonal dairy production system
E. B. Thomas A B , K. A. Dolecheck

A Department of Animal and Food Sciences, University of Kentucky, Lexington, KY 40546, USA.
B Department of Agricultural Economics, University of Kentucky, Lexington, KY 40546, USA.
C DairyNZ, Private Bag 3221, Hamilton 3240, New Zealand.
D CowFocused Housing, 100 Killarney Drive, Bardstown, KY 40004, USA.
E Corresponding author. Email: karmella.dolecheck@uky.edu
Animal Production Science 59(12) 2280-2287 https://doi.org/10.1071/AN17730
Submitted: 24 October 2017 Accepted: 28 April 2019 Published: 13 September 2019
Journal Compilation © CSIRO 2019 Open Access CC BY-NC-ND
Abstract
Context: Advances in automated oestrus detection have made this an attractive technology to help reduce manual oestrus detection labour on dairy farms.
Aims: A decision-support tool was created to help farmers estimate the investment outcome of adopting automated oestrus detection technologies in a seasonal dairy production system.
Methods: A decision-support tool was created using Excel 2011 (Microsoft Inc., Redmond, WA, USA). The tool allows farmers to input both current herd reproductive management costs and performance and automated oestrus detection technology system costs and performance to receive herd-specific estimates of investment benefit. The investment analysis outputs include the net present value (NPV), internal rate of return (IRR), and payback period associated with automated oestrus detection adoption. Two different automated oestrus detection technologies were compared with visual oestrus detection aided by tail paint with a 72.0% oestrus detection rate (sensitivity) to demonstrate the value of the investment analysis tool. The alternative scenarios, technology one and technology two, were compared over an eight-year investment period.
Key results: Technology one, with a 62.4% oestrus detection rate, resulted in a negative NPV and IRR (–NZ$182 567 and –100% respectively), indicating a poor investment. Technology two, with an oestrus detection rate of 91.0%, provided a positive NPV and IRR (NZ$177 890 and 38.7% respectively), indicating a beneficial investment. The payback period for technology one was estimated as >10 years, whereas technology two’s payback period was <1 year.
Conclusions: The investment tool results are dependent on farm-specific and automated oestrus detection inputs.
Implications: Farmers can use farm-specific inputs in the tool to aid them when considering adoption of new automated oestrus detection technologies.
Additional keywords: precision dairy farming, precision dairy monitoring, seasonal dairying.
Introduction
Calving in seasonal, pasture-grazed, dairy farming acts to align herd feed demand with pasture availability, therefore conception date and calving date are key factors in optimising production. In seasonal calving dairy herds, when an individual cow calves relative to the herd’s planned mating commencement date is a major determinant of reproductive success (Alawneh et al. 2012). Farm personnel generally detect cows in oestrus by observing signs of oestrus-related behaviour; for example, willingness to stand while being mounted (Eradus et al. 1992; Kamphuis et al. 2012). Tail paint and mount detectors (e.g. scratch-off or pressure-activated devices placed on the tail head of the cow to detect mounts by other cows) are common aids for visual oestrus observation (Macmillan and Curnow 1977). These tools allow personnel to observe worn paint or activated mount detectors as a sign that the cow was in oestrus. Larger herd sizes, lack of labour, and untrained personnel can make visual observation challenging, even with aids (Holmann et al. 1987; Alawneh et al. 2006; Olynk and Wolf 2009). Additionally, oestrus is increasingly difficult to observe in the modern dairy cow with shorter and less intense oestrus periods (Reames et al. 2011; Homer et al. 2013).
Depending on variables such as herd size, technology cost, detection rates and labour rates, automated oestrus detection technologies can increase the success of oestrus detection and decrease labour costs (Olynk and Wolf 2009; Rutten et al. 2014; Dolecheck et al. 2016). Differing on-farm cost structures, reproductive performance levels, management ability, and other economic factors make different reproductive management programs attractive to farmers (Holmann et al. 1987; Olynk and Wolf 2008, 2009). In general, the decision of a farmer to invest depends on the expected profitability of the investment, the farm’s financial position, market prospects, farm size and the presence of a potential farm successor (Aramyan et al. 2007). Investments in precision technologies have shown to be impacted by uncertainty regarding technology performance, the presence of after-sales support, and the need for new skills among the farm team (Eastwood et al. 2016).
The objective of this study was to create a decision-support tool to estimate the investment outcome of adopting automated oestrus detection technologies in a seasonal dairy production system. The investment decision-support tool can be used to compare current reproductive performance to estimated performance after automated oestrus detection technology adoption. The Economics of Reproductive Performance Tool ver. NZ 2.0 (DairyNZ Ltd 2018) was used as a base to estimate the reproductive value of adopting an automated oestrus detection technology through changes in a herd’s 6-week in-calf rate and 12-week not-in-calf rate. Two example investment analyses were conducted considering a collar-mounted activity monitoring technology or an automated camera-based oestrus detection technology to demonstrate model functionality.
Materials and methods
The investment outcome of transitioning from visual oestrus detection based reproductive management to automated oestrus detection was examined via a decision-support tool using a partial budget analysis. The decision-support tool was created using Excel 2011 (Microsoft Inc., Redmond, WA, USA) and was separated into three specific sheets: adjustable user inputs, automated oestrus detection technology calculations and results. The input sheet was created for users to enter herd size, current reproductive performance information, labour time, and labour costs (NZ$ per hour). Additionally, information about potential automated oestrus detection technologies was entered into the input sheet. The input sheet captured details for current and proposed oestrus detection options including reproductive management costs, expected reproductive performance, assumptions for the gap calculations, and investment terms. Information from the inputs sheet was linked into the automated oestrus detection calculations sheet to calculate the reproductive management costs both before and after a proposed investment in automated oestrus detection.
Reproductive management costs
Reproductive management costs included the automated oestrus detection technology start-up cost, automated oestrus detection technology maintenance cost, oestrus detection aid cost and labour. The automated oestrus detection technology start-up costs included the hardware needed for the automated oestrus detection technology; no start-up cost was assumed for non-automated oestrus detection. The automated oestrus detection technology maintenance cost included a yearly fee charged by the technology manufacturer for upkeep; no maintenance cost was assumed for non-automated oestrus detection. The oestrus detection device cost for non-automated oestrus detection would be tail paint, mount detectors, or nothing in the case of visual observations alone. For consumables like tail paint and mount detectors, the inputs needed for the model included the mean number used per cow during a breeding season and the cost per item (i.e. total cost of consumables per year = herd size × number of visual detection devices used per cow per year × price per visual detection device). The oestrus detection device cost when using automated oestrus detection technologies would be the cost of the individual device. The investment tool used the farmer’s technology inputs to compute the total initial automated oestrus detection technology cost as:

where TIC is the total initial investment cost (NZ$), Start is the start-up cost of the automated oestrus detection technology (NZ$), DeviceCost is the oestrus detection device cost per unit (NZ$) and HS is herd size.
For automated oestrus detection devices placed on an individual animal, an additional cost was added to account for replacing non-reusable, faulty, damaged, or lost oestrus detection devices. The cost to replace oestrus detection devices each year was calculated as:

where ReplaceDevices is the cost to replace oestrus detection devices each year (NZ$) and ReplacePct is the percentage of oestrus detection devices to replace per year (%).
Because labour requirements vary during the breeding season, three different labour periods were defined: pre-mating, artificial breeding (AB) and bull mating. The pre-mating period was a period where observation and recording of cows in heat was conducted to confirm reproductive cyclicity. The AB period was a period of active breeding by artificial insemination. The bull mating period was a period of bull mating for cows not pregnant after the AB period. Within the model, users could define the length of each period and several labour hours per week for each of the three periods. Additionally, different labour rates could be defined for each labour period to account for the increased cost of more skilled staff during certain labour periods (e.g. during the AB period). The cost of labour for each labour period was calculated by multiplying the number of labour hours needed per week for that period (pre-mating, AB, or bull breeding), the labour rate, and the length of that period (pre-mating, AB, or bull breeding) in weeks. The costs of pre-mating labour, AB labour, and bull mating labour were summed to calculate total labour costs. The total labour costs before and after automated oestrus detection technology adoption were calculated using different inputs for labour required per period. The difference between the cost of labour for visual oestrus detection (pre-automated oestrus detection technology adoption) and the cost of labour required for the automated oestrus detection technology represented the amount a farmer could save in labour costs.
Reproductive performance calculations
The model calculated both the current reproductive performance and the reproductive performance if the farmer were to invest in an automated oestrus detection technology. The user is able to select either AB mating or bull mating for each week of breeding, up to 12 weeks. This was included to give the farmer an option to assess an alternative breeding program, such as an extended AB period using automated oestrus detection and eliminating the cost and potential health and safety risks associated with bulls. The pregnancy rate was calculated separately for AB and bull mating as the product of oestrus detection rate and conception rate during each period (as defined by the user). The percent of cows pregnant after three weeks, cycle one, was calculated as:
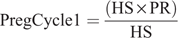
where PregCycle1 is the percentage of cows pregnant after first cycle (%) and PR is the pregnancy rate (%).
The percentage of cows that became pregnant after the first cycle was used to determine the number of cows eligible to be bred during the next week (i.e. nonpregnant cows), which in turn was used to calculate the percent of cows pregnant after each week:

where %Preg is the percentage of cows pregnant after week n (4 to 12) and PP is the percentage of cows pregnant at the end of the previous week (%).
The total percent of cows pregnant after week six and twelve for both the current oestrus detection methods and automated oestrus detection were used in the Economics of Reproductive Performance Tool ver. NZ 2.0 (DairyNZ Ltd 2018).
Economics of reproductive performance tool
The Economics of Reproductive Performance Tool ver. NZ 2.0 (DairyNZ Ltd 2018), also known as the gap calculator, was used to compare the herd’s level of overall reproductive performance to a target value and to estimate the opportunity for increasing operating profit through improved reproductive performance. The 6-week in-calf and 12-week not-in-calf gap values were calculated as:


where 6WeekGap is the 6-week in-calf rate gap value (NZ$), Desired6WeekRate is the desired 6-week in-calf rate (%), Actual6WeekRate is the actual 6-week in-calf rate (%), 6WeekPctValue is the value per percent change in 6-week in-calf rate (NZ$), 12WeekGap is the 12-week in-calf rate gap value (NZ$), Desired12WeekRate is the desired 12-week in-calf rate (%), Actual12WeekRate is the actual 12-week in-calf rate (%) and 12WeekPctValue is value per percent change in 12-week in-calf rate (NZ$).
The sum of the 6-week in-calf rate gap value and the 12-week not-in-calf gap value was the total reproductive gap value. The total reproductive gap value gives the farmer a dollar amount per year that the herd would be able to generate by obtaining the target proportion of cows in-calf within the defined mating period. The gap calculator was used to estimate the total reproductive gap value both before and after the adoption of an automated oestrus detection technology. The differences in the values before and after the adoption were compared to estimate the value of automated oestrus detection technology adoption.
Investment analysis
The investment analysis outputs from the model included net present value (NPV), internal rate of return (IRR), and payback period associated with automated oestrus detection technology adoption. For the investment analysis, a farmer would need to define the length of the investment term and the residual value. The investment term should be equal to the expected life of the technology (in years). The residual value reflects what the automated oestrus detection technology could be worth at the end of the investment term.
The NPV was used to compare investment in the automated oestrus detection technology to future cash flows. If the NPV was greater than zero, this indicated a good investment. The change in cash flow for each year consisted of the financial difference between the current oestrus detection method and automated oestrus detection, including costs associated with both reproductive management and overall reproductive performance:
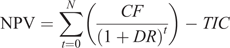
where NPV is the net present value of the automated oestrus detection technology over the investment period, n is the number of years in the investment period, t is the year of investment, CF is the cash flow at period t and DR is the discount rate.
The IRR can help a farmer understand the level of potential value associated with each investment option with comparable capital and risk. The IRR is calculated by setting the NPV equation equal to zero and solving for the discount rate. For the calculated IRR to be attractive for the farmer, the IRR should be greater than the discount rate considered. The IRR is useful for comparing multiple available automated oestrus detection technologies. Finally, the payback period, or number of years to break even, identified the point in time where the cost of the initial investment was returned. Each of these financial measures (NPV, IRR and payback period) needs to be evaluated across various scenarios when assessing the risk of a potential investment (Brigham 1985).
Investment analysis example
An investment analysis was conducted to demonstrate the functionality of the decision-support tool. Base herd input assumptions are shown in Table 1. Two different types of automated oestrus detection technologies were compared: technology one and technology two. Technology one was a collar-mounted sensor used to monitor a cow’s change in activity, which can be associated with oestrus (Dela Rue et al. 2014). Technology two used a camera and image analysis to determine when mount detection patches were activated as evidence that cows were in oestrus. The adjustable reproductive management strategy inputs were set to those in Table 2. Oestrus detection rates, representing sensitivity, were assumed to be 72.0%, 62.4%, and 90.5% for visual oestrus detection aided by tail paint, technology one, and technology two, respectively, based on estimates found in the literature (Jago et al. 2011; Kamphuis et al. 2012). Specificity was assumed identical across all scenarios. These performance levels were chosen to demonstrate how a range in performance affected the investment outcome and are not necessarily representative of current technology. The number of labour hours fluctuated depending on the premating, AB, or bull mating period and on the reproductive management strategy used (Table 3). Technology two required more hours of labour than technology one because the mount detectors are non-reusable and must be replaced after activation or when missing.
![]() |
![]() |
![]() |
The estimated price of each oestrus detection device per cow for visual oestrus detection, technology one, and technology two was NZ$2.00, NZ$160.00 and NZ$2.00 respectively. The price per oestrus detection device for the visual oestrus detection strategy covered the cost of tail paint use. Cows expected to come into oestrus were assumed to be marked with tail paint and visually observed. Technology one required a wearable unit for each cow, whereas technology two required only a mount detector per cow but was associated with a larger total initial investment cost than technology one (NZ$20 000 vs NZ$10 000) for the installation of a camera to scan and detect activated mount detection patches. Annual oestrus detection device replacement depended on the reproductive management strategy being used (Table 2).
Gap calculator
The price per percentage point of the 6-week in-calf rate gap was set to NZ$4.00 and the price per percentage point of the 12-week not-in-calf rate was set to NZ$10.00. These values assumed a price of NZ$5.50 per kg milk solids and a NZ$1000 value differential between a not-in-calf and in-calf cow (DairyNZ Ltd 2018). The NZ$4.00 and NZ$10.00 are subject to vary with changes in milk price or changes in the value of a not-in-calf cow. Decision-support tool users can adjust these values as needed.
Results and discussion
Reproductive performance
The reproductive performance results highlight the difference between visual oestrus detection aided by tail paint, technology one, and technology two in relation to in-calf and not-in-calf rates. Greater in-calf rates and lower not-in-calf rates represent improved reproductive performance. The conception rate was held constant for all reproductive management strategies. Therefore, the differences are the result of changes in oestrus detection rate alone, which can be defined and adjusted by the user. Using inputs from Tables 1 and 2, the 6-week in-calf rates were 58.2%, 52.2%, and 68.5% for visual oestrus detection aided by tail paint, technology one, and technology two respectively. The 12-week not-in-calf rates were 16.0%, 18.0%, and 11.7% for visual oestrus detection aided by tail paint, technology one, and technology two respectively. The lower oestrus detection rate associated with technology one (62.4%) resulted in inferior reproductive performance than either visual oestrus detection aided by tail paint (oestrus detection rate = 72.0%) or technology two (oestrus detection rate = 90.5%). The oestrus detection rate assumed for technology two (90.5%) was greater than assumptions used in other automated oestrus detection investment analyses which assumed oestrus detection rates of 80% or less (Rutten et al. 2014; Dolecheck et al. 2016). However, the 90.5% value was collected from a previously published, New Zealand based study (Jago et al. 2011), and may be plausible in a grazing-based, low production system compared with the higher-production systems assumed in previous work (Rutten et al. 2014; Dolecheck et al. 2016).
Failure to achieve efficient oestrus detection limits reproductive performance (Burke et al. 2012). With new technology available, there is potential for oestrus detection to be improved (Fricke et al. 2014). However, this depends on the relative success of oestrus detection by the new technology compared with the current reproductive management strategy (Rutten et al. 2014; Dolecheck et al. 2016). Only if the new automated oestrus detection technology improves the oestrus detection rate will reproductive performance improve. However, little or no improvements in reproductive performance using the technology may be acceptable to the farmer if other benefits are provided (i.e. less labour, alternative labour opportunities, improved quality of life) as discussed by Eastwood et al. (2016).
Labour
The total labour cost was NZ$3844, NZ$1060 and NZ$1700 per year for visual oestrus detection, technology one, and technology two respectively. Technology one was associated with the lowest labour costs because the collar-activity monitors tracked the cows’ activity to detect oestrus and did not need to be replaced as frequently as the mount detector needed for technology two. The labour cost associated with technology one may be more appealing to a farmer than technology two; however, other factors (i.e. oestrus detection rate, total initial investment cost and cash flow) need to be considered when evaluating an automated oestrus detection technology.
Both automated oestrus detection technologies decreased the number of labour hours needed compared with visual oestrus detection aided by tail paint. However, most farmers conduct visual oestrus detection while performing another task, such as milking or collecting cows (Rutten et al. 2014), making estimation of any labour savings associated with automated oestrus detection difficult. Labour costs need to decrease considerably to impact profitability of investing in automated oestrus detection technologies (Rutten et al. 2014). However, the use of automated detection may compensate for less well trained staff in farm teams (Edwards et al. 2015).
Investment analysis
The NPV, IRR and payback period were used to evaluate the investment potential of both automated oestrus detection technologies (Table 4). The negative NPV associated with investment in technology one (–NZ$18 2567) indicates that the farmer should not invest. The positive NPV associated with investment in technology two (NZ$17 7890) indicates the potential value added to the farm. Similarly, the positive IRR of technology two solidifies a much greater return on the investment than technology one (38.7% vs –100.0%). An investment in technology one was estimated to take longer than 10 whereas technology two would take <1 year (0.60 years) to break even. Previous investment analyses have assumed that the lifetime of an automated oestrus detection technology varies from 7 (Dolecheck et al. 2016) to 10 years (Rutten et al. 2014). Reaching the break-even point before the end of the technology’s usefulness is ideal. Technology one resulted in a decreased oestrus detection rate compared with technology two (62.0% vs 90.5%) and in a greater total initial investment cost (NZ$76 400 vs NZ$20 830) because of the oestrus detection device prices. These two factors contributed to its negative NPV, negative IRR, and longer time to break even.
![]() |
Dolecheck et al. (2016) similarly found that management and technology assumptions influence whether an investment in automated oestrus detection will be profitable or not. When considering 24 different investment scenarios, payback period ranged from 1.6 to >10.0 years. Rutten et al. (2014) found that the IRR associated with investing in automated oestrus detection ranged from –2.0% at the lowest oestrus detection rate tested (65.0%) to 13.0% at the highest oestrus detection rate tested (95.0%). However, investment in automated oestrus detection in a seasonal dairy system is expected to produce different results because of the differing breeding requirements.
Sensitivity analysis
Because automated oestrus detection rates are not guaranteed, sensitivity analyses were run to account for possible variations in oestrus detection rates for each technology. A range in oestrus detection rate from 10% less than to 10% more than the original inputs (Table 2) influenced both the NPV and payback period results. As the oestrus detection rate for each technology increased, NPV improved because the total reproductive gap value decreased (Fig. 1a). However, the NPV for technology one remained negative up to an oestrus detection rate of 72%. By using the ‘Goal Seek’ function of Excel, we identified that technology one oestrus detection rate would have to reach 79% for the NPV to reach zero.
![]() |
Regardless of the oestrus detection rate assumption used (–10% to +10% of the original input), the technology one payback period was longer than 10 years. The low labour cost of technology one was never able to offset the high total initial investment cost and low oestrus detection rate in these scenarios. Again, the ‘Goal Seek’ function of Excel was used to identify that technology one oestrus detection rate would have to reach 76.0% for the payback period to be equal to the assumed investment length (8.0 years). Therefore, a 76.0% oestrus detection rate could be considered the performance threshold required for these types of technologies to break even in a seasonal dairy system, if the lifetime of the technology is at least 8 years. Dela Rue et al. (2014) has shown that collar-based technologies can reach this 76.0% oestrus detection rate threshold, indicating that there is potential for these types of technologies to be profitable. When considering variation in the oestrus detection rate, payback period for technology two ranged from 0.4 to 1.1 years (Fig. 1b). The greater the oestrus detection rate, the less time needed for technology two to break even.
In addition to the automated oestrus detection technology oestrus detection rate, many other farm specific factors will influence the value of investment. To evaluate some of these factors, changes in herd size, visual oestrus detection rate, and length of the AB period were evaluated as outlined in Table 5. Increasing or decreasing the herd size had minimal overall effects on the value of both technologies because most technology related costs were calculated per cow. Conversely, changes in either the visual oestrous detection rate or the length of the AB period had much greater effects on the NPV per cow. As the visual oestrus detection rate decreased, the value of both technologies increased and as the visual oestrus detection rate increased, the value of both technologies decreased. This follows the assumption that a herd with better reproductive management before technology adoption would see less value from the investment. Finally, as the length of the AB breeding period increased from 4 weeks to 12 weeks the value of technology one decreased whereas the value of technology two increased. The difference in response for each technology resulted from the pregnancy rate (oestrus detection rate multiplied by the conception rate) for technology one being lesser than the bull mating pregnancy rate (32.6% vs. 45.6%) whereas the pregnancy rate for technology two was greater (47.2%). Therefore, using technology two for a longer period of time (i.e. increasing the AB breeding period) would be valuable, whereas using technology one for a longer period of time would not be.
The results of the sensitivity analyses emphasise that different herds will see more or less value from investing in automated oestrus detection technologies, depending on their current reproductive management situation and the expected technology performance. Similarly, both Rutten et al. (2014) and Dolecheck et al. (2016) concluded that herd-specific calculations are needed when evaluating the value of automated oestrus detection technologies. Using tools like the decision support tool developed in this study is valuable because they are able to be customised to individual herd scenarios.
Model limitations
This investment decision-support tool uses only a partial budget and individuals should consider its limitations before use. The model does not account for factors such as changes in the number of replacements produced that could add more value to the herd. The automated oestrus detection technology could also be beneficial in other management areas such as detecting disease and calving; these factors were not accounted for in our model. Additionally, the model needs accurate input information from both the user and technology companies to calculate accurate results. However, the exact values found by the model are less important than the overall ranking of different oestrus detection methods.
Conclusions
A decision-support tool was created to evaluate the economics of adopting automated oestrus detection technologies in a seasonal dairy production system. Farmers can use farm-specific inputs in the tool to aid them when considering adoption of new automated oestrus detection technologies. The main financial benefits associated with adopting automated oestrus detection technologies are the decrease in labour costs and improvements in 6-week in-calf rate and 12-week not in-calf rate. In an investment analysis example, the technology that improved oestrus detection rate above visual oestrus detection aided by tail paint improved reproductive performance and was a positive investment opportunity. Additionally, the use of either tested automated oestrus detection technology reduced the total cost of labour for a breeding season compared with visual oestrus detection aided by tail paint.
Conflicts of interest
The authors declare no conflicts of interest.
Acknowledgements
This study was supported by funds from New Zealand dairy farmers through DairyNZ Inc. in partnership with Ministry for Primary Industries Primary Growth Partnership funding.
References
Alawneh J, Williamson N, Bailey D (2006) Comparison of a camera-software system and typical farm management for detecting oestrus in dairy cattle at pasture. New Zealand Veterinary Journal 54, 73–77.| Comparison of a camera-software system and typical farm management for detecting oestrus in dairy cattle at pasture.Crossref | GoogleScholarGoogle Scholar | 16596158PubMed |
Alawneh J, Stevenson M, Williamson N, Lopez-Villalobos N, Otley T (2012) The effect of liveweight change on reproductive performance in a seasonally calving, pasture fed dairy herd. Livestock Science 145, 131–139.
| The effect of liveweight change on reproductive performance in a seasonally calving, pasture fed dairy herd.Crossref | GoogleScholarGoogle Scholar |
Aramyan LH, Lansink AGO, Verstegen JA (2007) Factors underlying the investment decision in energy-saving systems in Dutch horticulture. Agricultural Systems 94, 520–527.
| Factors underlying the investment decision in energy-saving systems in Dutch horticulture.Crossref | GoogleScholarGoogle Scholar |
Brigham EF (1985) ‘Financial management: theory and practice.’ (Atlantic Publishers: Ocala, FL)
Burke CR, Kamphuis C, Meier S, Pellow R (2012) An evaluation of a progesterone-based diagnostic as an aid to re-insemination decisions in a seasonal, pasture-grazed dairy cow herd. In ‘Proceedings of the New Zealand Society of Animal Production’. pp. 35–37. (New Zealand Society of Animal Production Inc.: Christchurch, NZ)
DairyNZ Ltd (2018) Economics of reproductive performance tool version NZ 2.0. Available at https://www.dairynz.co.nz/media/669759/economics_reproductive_v2.pdf [Verified 23 October 2018]
Dela Rue B, Kamphuis C, Burke C, Jago J (2014) Using activity-based monitoring systems to detect dairy cows in oestrus: a field evaluation. New Zealand Veterinary Journal 62, 57–62.
| Using activity-based monitoring systems to detect dairy cows in oestrus: a field evaluation.Crossref | GoogleScholarGoogle Scholar | 24156478PubMed |
Dolecheck KA, Heersche G, Bewley JM (2016) Retention payoff-based cost per day open regression equations: application in a user-friendly decision support tool for investment analysis of automated estrus detection technologies. Journal of Dairy Science 99, 10182–10193.
| Retention payoff-based cost per day open regression equations: application in a user-friendly decision support tool for investment analysis of automated estrus detection technologies.Crossref | GoogleScholarGoogle Scholar | 27665133PubMed |
Eastwood C, Jago J, Edwards J, Burke J (2016) Getting the most out of advanced farm management technologies: roles of technology suppliers and dairy industry organisations in supporting precision dairy farmers. Animal Production Science 56, 1752–1760.
| Getting the most out of advanced farm management technologies: roles of technology suppliers and dairy industry organisations in supporting precision dairy farmers.Crossref | GoogleScholarGoogle Scholar |
Edwards J, Rue BD, Jago J (2015) Evaluating rates of technology adoption and milking practices on New Zealand dairy farms. Animal Production Science 55, 702–709.
| Evaluating rates of technology adoption and milking practices on New Zealand dairy farms.Crossref | GoogleScholarGoogle Scholar |
Eradus W, Rossing W, Hogewerf P, Benders E (1992) Signal processing of activity data for oestrus detection in dairy cattle. EAAP Publication 65, 360–369.
Fricke PM, Carvalho PD, Giordano JO, Valenza A, Lopes G, Amundson MC (2014) Expression and detection of estrus in dairy cows: the role of new technologies. Animal 8, 134–143.
| Expression and detection of estrus in dairy cows: the role of new technologies.Crossref | GoogleScholarGoogle Scholar | 24680286PubMed |
Holmann FJ, Blake RW, Shumway CR (1987) Economic evaluation of fourteen methods of estrous detection. Journal of Dairy Science 70, 186–194.
| Economic evaluation of fourteen methods of estrous detection.Crossref | GoogleScholarGoogle Scholar | 3571621PubMed |
Homer E, Gao Y, Meng X, Dodson A, Webb R, Garnsworthy P (2013) Technical note: a novel approach to the detection of estrus in dairy cows using ultra-wideband technology. Journal of Dairy Science 96, 6529–6534.
| Technical note: a novel approach to the detection of estrus in dairy cows using ultra-wideband technology.Crossref | GoogleScholarGoogle Scholar | 23910546PubMed |
Jago J, Rue BD, Kamphuis C (2011) Automation of oestrus detection. DairyNZ Technical Series. Available at https://www.dairynz.co.nz/media/424967/technical_series_december_2011.pdf [Verified 16 October 2017]
Kamphuis C, DelaRue B, Burke C, Jago J (2012) Field evaluation of 2 collar-mounted activity meters for detecting cows in estrus on a large pasture-grazed dairy farm. Journal of Dairy Science 95, 3045–3056.
| Field evaluation of 2 collar-mounted activity meters for detecting cows in estrus on a large pasture-grazed dairy farm.Crossref | GoogleScholarGoogle Scholar | 22612940PubMed |
Macmillan K, Curnow R (1977) Tail painting – a simple form of oestrus detection in New Zealand dairy herds. New Zealand Journal of Experimental Agriculture 5, 357–361.
| Tail painting – a simple form of oestrus detection in New Zealand dairy herds.Crossref | GoogleScholarGoogle Scholar |
Olynk N, Wolf C (2008) Economic analysis of reproductive management strategies on US commercial dairy farms. Journal of Dairy Science 91, 4082–4091.
| Economic analysis of reproductive management strategies on US commercial dairy farms.Crossref | GoogleScholarGoogle Scholar | 18832236PubMed |
Olynk N, Wolf C (2009) Stochastic economic analysis of dairy cattle artificial insemination reproductive management programs. Journal of Dairy Science 92, 1290–1299.
| Stochastic economic analysis of dairy cattle artificial insemination reproductive management programs.Crossref | GoogleScholarGoogle Scholar | 19233822PubMed |
Reames PS, Hatler TB, Hayes SH, Ray DL, Silvia WJ (2011) Differential regulation of estrous behavior and luteinizing hormone secretion by estradiol-17β in ovariectomized dairy cows. Theriogenology 75, 233–240.
| Differential regulation of estrous behavior and luteinizing hormone secretion by estradiol-17β in ovariectomized dairy cows.Crossref | GoogleScholarGoogle Scholar | 20875680PubMed |
Rutten CJ, Steeneveld W, Inchaisri C, Hogeveen H (2014) An ex ante analysis on the use of activity meters for automated estrus detection: to invest or not to invest? Journal of Dairy Science 97, 6869–6887.
| An ex ante analysis on the use of activity meters for automated estrus detection: to invest or not to invest?Crossref | GoogleScholarGoogle Scholar | 25242421PubMed |