Genetic relationships among yearling fertility, body composition and weight traits in tropically adapted composite cattle
M. L. Facy



A Davies Livestock Research Centre, School of Animal and Veterinary Sciences, University of Adelaide, Roseworthy, SA 5371, Australia.
B Robinson Research Institute, Adelaide Medical School, University of Adelaide, Adelaide, SA 5006, Australia.
Animal Production Science 63(11) 983-995 https://doi.org/10.1071/AN22453
Submitted: 13 December 2022 Accepted: 9 March 2023 Published: 6 April 2023
© 2023 The Author(s) (or their employer(s)). Published by CSIRO Publishing. This is an open access article distributed under the Creative Commons Attribution-NonCommercial-NoDerivatives 4.0 International License (CC BY-NC-ND)
Abstract
Context: Reproduction is an economically important trait in both males and females; however, the relationships between fertility and body composition traits are little researched, but essential to breeding programs, as they will help inform selection decisions and allow the greatest opportunity for genetic gain.
Aims: Estimate genetic and phenotypic correlations between male and female yearling fertility traits and investigate their relationship with yearling body composition traits, which have an effect on the attainment of puberty.
Methods: Genotype and phenotype data were obtained from a tropical composite commercial cattle population and imputed to 27 638 single nucleotide polymorphisms. A series of univariate and bivariate linear mixed models using a genomic relationship matrix were run to estimate genetic parameters, genetic and phenotypic correlations for a series of male and female fertility and body composition traits. These parameters were then compared to help understand the genetic relationships.
Key results: Scrotal circumference was favourably genetically correlated with weight (0.34), fat traits (0.06–0.24), muscle (0.24) and heifer days to calving (−0.32). Heifer days to calving was favourably correlated with muscle (−0.18) but not fat traits (0.11 to 0.21). The genetic correlations between heifer days to calving and sperm morphology traits were generally unfavourable (−0.32 to 0.25). Sperm morphology traits were favourably genetically correlated with fat traits (−0.84 to 0.31) and muscle (−0.61 to 0.31) but not weight (−0.15 to 0.09).
Conclusions and implications: Yearling sperm morphology traits were unfavourably correlated with heifer days to calving, indicating that they are not good candidates for indirect selection on improving female fertility in the herd. A different trend was found for yearling scrotal circumference and heifer days to calving, identifying it as a good candidate for indirect selection of heifer fertility as it is easy to measure and heritable. The genetic correlations estimated between composition traits with male and female fertility traits allow breeding programs to make an informed selection decision to optimise genetic gain across all traits.
Keywords: cattle, fertility, genetic correlation, genetic evaluation, heritability, sperm morphology, ultrasound, variance components.
Introduction
Reproduction in the northern Australian cattle industry is a key profit and sustainability driver (Johnston et al. 2014a). Nevertheless, traditionally, fertility traits are ignored in breeding programs due to their low heritability and complexity to measure. Despite this, fertility traits, particularly those of yearlings, have the potential for great genetic progress and improved productivity (Johnston et al. 2014a; Chilcott et al. 2020). Business-as-usual approaches will not help improve productivity; new and innovative approaches need to be identified (Chilcott et al. 2020). One of these approaches is using female and male early-in-life fertility traits to improve the lifetime weaning rate.
A major sustainability issue in northern Australia is the late attainment of puberty in heifers, which is intensified by the harsh climate (Johnston et al. 2009; Schatz 2011). Reducing the age at which puberty is attained will allow reproductive life to begin earlier, reducing the time that heifers are non-productive. Typically age of puberty is recorded through ovarian scans that need to be conducted regularly leading into joining, which is expensive and labour-intensive. However, there are indicative fertility traits that can be easily measured in females and males at yearling age. The fact that these yearling traits are measured early in life allow genetic gain to be maximised through reduced generation intervals (Boichard et al. 2012). Improving yearling fertility traits in both sexes will allow for increases in production efficiency (weaning rates) without an increase in the herd size.
Improving reproduction in both sexes also creates numerous benefits across the supply chain and will improve the beef industry’s productivity. Further, there is potential to genetically select for increased fertility in females through correlated traits expressed in males, Land (1973) first proposed the interplay between male and female fertility in mammals; however, since then, several studies in beef cattle have shown favourable genetic correlations between bull and cow fertility (Brinks et al. 1978; Smith et al. 1989; Johnston et al. 2014b; Raidan et al. 2019). The key finding is a significant correlation between bull scrotal circumference and age of puberty, leading to the adoption of scrotal circumference to improve heifer fertility (Brinks et al. 1978; Morris et al. 2000); however, other male fertility traits have not been extensively researched, with specific sperm abnormalities not being investigated.
Investigating the correlations between yearling male and female fertility traits with body composition and weight traits is important. Growth and body composition traits have a positive association with the age of puberty and, potentially, with yearling fertility traits (Johnston et al. 2014b). There have been very few studies looking into the associations between yearling fertility and yearling composition traits, with none using yearling joining in tropically adapted cattle (Davis 1993; Mercadante et al. 2003; Forni and Albuquerque 2005). Despite this, Wolcott et al. (2014a) reported genetic correlations between days to calving at first (at 24–28 months) and second (36–40 months) joining, with heifer (18 months) growth and body composition traits in tropical composites being low to moderately correlated.
A better understanding of the genetic relationships between yearling male and female fertility traits is needed, as it will help improve herd reproduction ability, improving productivity and sustainability. Further, the genetic relationship between fertility, growth and composition traits in tropically adapted cattle will help inform cattle breeders’ selection decisions to reduce the age of puberty and potentially improve fertility. The study herein presents the results of the genetic and phenotypic relationships between male and female yearling fertility traits, with the objective of identifying male traits that are associated with attainment of puberty, and so can add to indirect selection criteria for female reproduction. The study also examines the genetic and phenotypic correlations between fertility traits and growth and composition traits.
Materials and methods
Dataset
Popplewell Composites, a breeding company focused on increased fertility, reduced age of puberty and maintaining adaptability to tropical environments provided phenotypic and genotypic data. All cattle in the Popplewell Composites breeding herd are mated as yearlings (12–15 months), providing yearling phenotypes as outlined below on males and females. The bull breeding program is situated in south-eastern Queensland, with all traits being measured there, and bulls being sold across northern Australia.
Phenotype data included days to calving (yearling joining), 400-day weight, 400-day ultrasound carcass traits (P8, rib fat (Rib), eye-muscle area (EMA) and intra-muscular fat (IMF)) and yearling bull breeding soundness evaluation (BBSE) traits. A detailed description of traits is included (Table 1) as well as a summary (Table 2). Days to calving was used as the main female fertility measurement as it is a composite trait that encompasses age of puberty, conception rate, gestation length and how early they conceive (Mucari et al. 2007; Cammack et al. 2009).
![]() |
![]() |
Days to calving is the time from the day the bull goes in with the heifer to the day the heifer gives birth (Meyer et al. 1990). For yearling days to calving (DC1), there was a total of 649 records or a total of 649 heifers joined, with 435 pregnant heifers and 214 empty heifers as described in Facy et al. (2023). A penalty of +32 days (1½ oestrus cycles) above the maximum value within each join group was given to heifers that did not conceive, thus assuming they would conceive in the next cycle and a half, given the opportunity. The common penalty value used is 21 days (one oestrus cycle; Graser et al. 2005), but a more extended period was used herein to allow for low-quality subtropical pastures, although this has little impact on the breeding values (Facy et al. 2023).
The male traits used were scrotal circumference (SC), percentage normal sperm (PNS), proximal droplets (PD), distal mid-piece reflex (DMR), knobbed acrosomes (KA), swollen acrosomes (SA), vacuoles and teratoids (VT) and sheath score (Sheath). These measurements were attained from BBSE and detailed morphology spermiogram conducted in a laboratory on yearling bulls. PD, DMR, KA, SA and VT were all transformed using the natural log, as they were all extremely skewed towards zero. Scrotal circumference and sheath score were recorded on one date each year about 12-months of age, sperm morphology traits (PNS, PD, DMR, KA, SA and VT) were recorded over multiple dates to allow for recording age to be as close to 12 months of age as possible.
Yearling weight (YWT) followed the common definition of 400-day weight, being between the ages of 301 and 500 days recorded on both heifers (1005 records) and bulls (1140 records). Ultrasound measurement of carcass traits was conducted on live animals aged 12 months (yearlings), generally on the same day as YWT on both males and females; there were 979 heifer records and 1069 bull records (Table 2).
Genotyping was conducted over many years, so multiple SNP chips were used. The chips included Illumina 777k, Illumina GGPLD V3 30K, Illumina GGPLD V4 30K, Illumina ICB 50K and Weatherby’s Scientific Versa50K, with a total of 2613 animals genotyped. There were 10 830 SNPs common across all chips. The common SNPs were used to impute each chip up to GGPLD30K (27 755 SNPs) on all genotyped animals using FImpute 2.1 (Sargolzaei et al. 2011). After imputation, genotype data were filtered down to 2609 animals and 27 638 single-nucleotide polymorphisms (SNPs); SNPs with <1% minor allele frequency and duplicate animals were removed. Further, from the genotype data, the heterozygosity fraction (Het) was calculated as the proportion of imputed heterozygote SNPs for each individual animal (Pitchford et al. 2022).
Genomic model
Univariate models were run for all traits to obtain variance components and heritability estimates. A linear mixed model used to estimate breeding values for multiple traits can be written as

where y is the vector of phenotypic values for days to calving; b represents fixed effects with design matrix X; the fixed effects included in all models are listed in Table 3; Z is the design matrix for hybrid additive effects u with ; and e is the residual effects.
![]() |
The genomic relationship matrix is calculated as follows using VanRaden’s first method (VanRaden 2008):
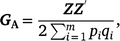
where the matrix Z has the dimensions of n × m, n is the number of individuals, and m is the number of markers. Matrix Z has the elements zij for the ith marker at the jth individual, as follows:
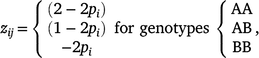
where pi is the allele frequency of the most frequent (major) allele at marker i, and qi = 1 − pi.
Bivariate models were run among all traits to obtain genetic and phenotypic correlations. The mixed model for the bivariate is now written as

where , the combined vector of data between two traits;
is the vector of fixed effects (Table 3), with
the associated design matrix;
is the sector of additive random effects, with
the associated design matrix and
the vector of residual variance.
Birth group, dam age, joining management group, sex, contemporary group and heterozygosity fraction were included as fixed effects in the model, where appropriate, for the trait under analysis (Table 3). Weight was not fitted as a covariate for the ultrasound traits (EMA, P8, Rib and IMF). Birth group was defined within the year of birth as early (1 June−4 September), mid (5 September−30 November) or late (1 December to the end of calving). Dam age was defined as heifer for first calving or mature for any other calving. Contemporary group definitions and number of groups are listed in Table 3. Scrotal circumference and sheath were recorded at one time point during the annual cycle but all sperm morphology traits (PNS, PD, DMR, KA, SA and VT) were recorded at multiple dates in the annual cycle to ensure the bulls were as close to 12 months as possible.
All univariate and bivariate models were fitted for all traits utilising a genomic relationship matrix for additive effects, and heterozygosity fraction (%) as a linear covariate (Pitchford et al. 2022). All univariate and bivariate models were run using R (R Core Team 2021) within RStudio (RStudio 2022), by using the package ASREML-R (Butler et al. 2017).
Results
Univariate models
Estimates of variance components, heritabilities and heterozygosity coefficient were evaluated for all traits (Table 4). Heifer days to calving, DC1, had a heritability of 0.20 and a heterozygosity coefficient of −1.90 days/%, indicating that more heterozygous animals conceived earlier in the joining period. SC, Sheath and two sperm traits, PNS and PD were all highly heritable. PNS and Sheath had the highest heritability of 0.49, next was SC at 0.45 and PD had a heritability of 0.39. DMR, SA and VT had lower heritability estimates of 0.18, 0.12 and 0.16 respectively (Table 4). The heterozygosity coefficient for male traits was only significant for SC and PD, with coefficients indicating earlier age at puberty (+0.30%/cm and −0.11ln(%%) respectively, Table 4).
The yearling composition traits were all moderately to highly heritable, ranging from 0.21 to 0.40 (Table 4). YWT had the greatest heritability (0.40) and a heterozygosity coefficient of 4.23 kg/%, the largest for all traits herein. The yearling ultrasound traits all had similar heritabilities, ranging from 0.21 to 0.33, with significant heterozygosity coefficients (Table 4).
Genetic and phenotypic correlations between male and female traits compared with yearling composition traits
Genetic and phenotypic correlations were estimated between the fertility and composition traits (Table 5). The genetic correlations between DC1 and composition traits were generally low and favourable. EMA and IMF were most strongly correlated with DC1 of −0.18 and −0.30 respectively. In contrast, P8 and Rib had low unfavourable correlations with DC1 of 0.11 and 0.21 respectively. The genetic correlation between DC1 and YWT was also low (−0.09). The phenotypic correlations between DC1 and yearling composition traits were low to moderate and favourable (Table 5).
![]() |
The genetic correlation between SC, PNS, PD, SA, VT and sheath and yearling composition traits were generally favourable, particularly for EMA and IMF, with reported values ranging from low to high (−0.51–0.31, Table 5). Note that the favourable direction differs between SC/PNS and PD, SA, VT and Sheath. For DMR and KA, the genetic correlations with EMA and IMF were unfavourable to no correlation, ranging from low to moderate (−0.03–0.39, Table 5). YWT generally had low genetic correlations with male traits, except for SC (0.34). P8 and Rib generally had a favourable genetic correlation with male traits. The strongest genetic correlations were between VT and fat, being −0.77 and −0.84 for P8 and Rib respectively. Similar trends were seen for phenotypic correlations between yearling composition traits and male or female traits, with all the estimates either being favourable or uncorrelated.
Genetic correlations female and male fertility traits
Bivariate models were run between the yearling male traits and DC1, yet only genetic correlations could be estimated due to the sex-limited nature of the data. The genetic correlation between DC1 and SC was moderate and favourable (−0.32). Sheath score and DMR were also favourably correlated with DC1 (0.20 and 0.25 respectively). The other sperm morphology traits (PNS, PD, KA, SA and VT) were all low, but unfavourably correlated with DC1 (Table 5).
Discussion
Heritability of male, female and composition traits
The heritability estimate for DC1 was 0.20, which is similar to previous published estimates of 0.22 in Brahmans and 0.13 in Tropical Composites, despite these latter animals being first joined at 2 years old (Johnston et al. 2014a). One other study estimated the heritability of DC1 in Angus to be 0.10; however, the age range of joining was from 12 to 20 months, with an average age of 15 months (Johnston and Bunter 1996). Further, previous publications have found a genetic correlation between DC1 and age of puberty to be high in Brahmans (0.79), indicating that DC1 is a good indicator of age of puberty in heifers, although this correlation was much lower in Tropical Composites (0.10) (Johnston et al. 2014b). Days to calving is often improved when heifers have attained puberty prior to exposure to the bull; therefore, if all heifers are pubertal prior to joining, then there is likely to be little effect on DC1 and age of puberty. This could cause the difference between genetic correlations of Brahmans and Tropical Composites in Johnston et al. (2014b) for DC1 and age of puberty. At the point of joining, most Tropical Composite heifers have reached puberty; however, only half the Brahmans had (Johnston et al. 2014a). The current study heritability estimate of YWT was 0.40, which is comparable to previous published estimates for both Bos taurus indicus and Bos taurus taurus cattle, ranging from 0.28 to 0.53 (Meyer et al. 1991; Burrow 2001; Boligon and Albuquerque 2011; Weik et al. 2022). Similarly, the ultrasound traits (EMA, P8, Rib and IMF) had moderate heritability, ranging from 0.21 to 0.31, which is similar to previous published estimates (Reverter et al. 2000; Stelzleni et al. 2002; Caetano et al. 2013; Naserkheil et al. 2021; Weik et al. 2022).
The heritability of SC at 12 months was 0.45 (Table 4), in agreement with previous published estimates, ranging 0.40–0.57 (Burrow 2001; Eler et al. 2004; Kealey et al. 2006; Garmyn et al. 2011; Corbet et al. 2013; Jeyaruban and Johnston 2017). The heritability estimate of PNS at 12 months was 0.49. Published heritability estimates range greatly (Smith et al. 1989; Yilmaz et al. 2004; Kealey et al. 2006; Corbet et al. 2013); however, this is likely to be due to the difference in the age of measurement. The estimate herein was similar to those provided for cattle at 12 months in both temperate and tropically adapted herds, which ranged from 0.35 to 0.47 (Yilmaz et al. 2004; Kealey et al. 2006; Corbet et al. 2013).
Heritability estimates of sperm abnormalities have not been extensively researched, with only one previous publication estimating heritabilities for some defects. Herein, the heritability estimate was 0.39 for PD, which is similar to the heritability estimated by Roberts et al. (2010) of 0.37 in a taurine composite breed. Other heritabilities estimated by Roberts et al. (2010) were for KA and DMR, found to be lowly heritable (0.02 and 0.01 respectively). Herein, the heritabilities were much larger; 0.32 for KA and 0.18 for DMR. The differences between estimates could be due to the difference in populations/environments or different laboratories analysing the ejaculates. The study herein also estimated the heritability of SA and VT to be 0.12 and 0.16 respectively. Finally, sheath score was estimated to have a high heritability of 0.49, this is higher than previous estimates, which ranged from 0.18 to 0.38 (Bignardi et al. 2011; Gordo et al. 2012; Corbet et al. 2013). This higher heritability is likely to be due to difference in Sheath between parent breeds.
Covariance between yearling heifer days to calving and composition traits
Understanding how yearling composition traits affect fertility traits such as heifer days to calving is important for breeding programs. It allows breeders to ensure that heifers reach optimal body composition before joining. In this study, DC1 had a small favourable correlation with YWT (−0.09). Few published studies have treated heifer days to calving separate from overall days to calving, and even fewer have estimated the genetic correlation between DC1 and YWT. A previous study estimated the genetic correlation between DC1 and YWT in Angus to be 0.08 (Johnston and Bunter 1996). The genetic estimation is different from the current study herein; however, it is still low, indicating little association between weight and DC1. Wolcott et al. (2014b) estimated genetic correlations between DC1 and 18-month-old weights to be 0.06 in Tropical Composites, similar to correlations estimated herein for YWT. It should be noted that the study herein mated all DC1 animals as yearlings, but this is not true for Wolcott et al. (2014b), where animals were joined at 2-year olds. Further, using the same dataset, Johnston et al. (2014b) estimated DC1 and puberty weight of 0.12, slightly stronger than 18-month-old weights, although still low. These correlations indicated that, genetically, there is little association between DC1 and YWT. The phenotypic correlation between DC1 and YWT (−0.20, Table 5) was stronger than was the genetic correlation. Johnston and Bunter (1996) found a similar phenotypic correlation of −0.10. These phenotypic correlations suggest a stronger association between DC1 and YWT through environmental influences rather than genetic influences.
Yearling ultrasound EMA and IMF had a moderately favourable genetic correlation with DC1 (−0.18 and −0.30 respectively); this correlation indicated that a genetic improvement in EMA and IMF would result in an improvement in DC1. These favourable genetic correlations could be exploited in a breeding program by indirect selection on DC1 through EMA and IMF. Wolcott et al. (2014b) estimated genetic correlations between 18-month ultrasound EMA and DC1 in Tropical Composites, and Brahmans reporting correlations of 0.10 and −0.35 respectively. Wolcott et al. (2014b) did not adjust for weight, which is equivalent to the model herein. The Brahman result is more similar to that in the study herein, possibly due to the age of first joining and puberty. Heifers were first joined as yearlings in this study, but Wolcott et al. (2014a) first joined heifers as 2-year olds. However, there is a difference in the age of puberty, with Tropical Composites reaching puberty at a younger age than do Brahmans (Johnston et al. 2014b). Thus, for DC1 most animals for Tropical Composites at 2 years of age would have reached puberty prior to joining and the more appropriate comparison is between Brahmans first mated as 2-year old from Johnston et al. (2014b) and the Tropical Composites herein first mated at 12–15 months. It is likely that yearling DC1 in Tropical Composites is more similar to 2-year-old DC1 in Brahmans, a similar point in puberty. Wolcott et al. (2014b) also estimated the genetic correlation between the age of puberty and ultrasound EMA to be −0.36 in Brahmans and −0.33 in Tropical Composites. This indicates that body muscle is likely to be correlated with age at puberty, and since herein heifers are joined as yearlings, it is more important than when a maiden joining is at 2 years, as reported by Wolcott et al. (2014b). These genetic correlations indicate the importance of body muscle and fat on the attainment age of puberty and yearling breeding capability.
Despite the favourable genetic correlations estimated for DC1 with EMA and IMF, a different trend was found for P8 and rib fat depth. The genetic correlation was low and unfavourable (0.11 and 0.21 respectively). These genetic correlations would indicate that as P8 or rib fat is genetically increased, there would be an associated increase in DC1, leading to reduced reproductive efficiency and lowering lifetime weaning rates. Weik et al. (2022) found fat (ultrasound Rib and P8) to be uncorrelated with ultrasound EMA in Angus/Hereford cattle at 18 months; this may explain the difference in genetic correlations between fat and muscle with DC1. Wolcott et al. (2014b) also estimated the genetic correlation between DC1 and P8 of −0.18 in Brahmans and 0.26 in Tropical Composites. The genetic correlation for Tropical Composites is similar to the study herein; however, the Brahman genetic correlation is different; this could be due to the age differences, as the study herein records yearlings, whereas Wolcott et al. (2014a) recorded 18-month olds. Weik et al. (2022) estimated genetic correlations between days to conception in 2-year olds and ultrasound P8 and Rib at 18 months of age for Hereford/Angus to be −0.35 and −0.37 respectively. These genetic correlations are similar to the ones estimated by Wolcott et al. (2014b), although once again the latter were recorded at an older age than in the current study. The difference between the genetic correlations could be due to the amount of variation in fat; herein the amount of variation is lower in tropical cattle than that in Weik et al. (2022) in temperate cattle, hence differing relationships. Facy et al. (2023) reported that the genetic correlation between DC1 and DC2 was −0.06, with a higher genetic correlation in mature joinings of 0.66 between mature DC wet animals and DC1, indicating that DC1 is genetically different from older joinings. Thus, different genetic associations would be expected.
Bivariate models between yearling male traits and composition traits
Genetic improvement in male traits is regarded as both important directly for serving capacity and also indirectly as indicators of early puberty in females. Yearling male traits are important but often sperm morphology traits are not genetically evaluated in a breeding program. Despite this, infertility through males tends to have a big impact on the herd, with 20–40% of bulls having subfertility (Chenoweth 2002; Kastelic and Thundathil 2008); therefore, it is essential that the association between male traits and composition traits in understood. Very few studies have looked at the relationship between composition and male traits, with no there being published reports for correlations for sperm abnormalities. SC is a male trait that is commonly used in breeding programs. Herein the genetic correlation between YWT and SC was moderate and favourable (0.34), in consensus with other published correlations (Morris et al. 2000; Boligon et al. 2010). One study in Tropical Composites estimated the genetic correlation between SC at 12 months and weight at 15 months to be 0.57, being higher than estimates at yearling weight (Corbet et al. 2013). These correlations indicate that genetically selecting heavy animals as yearlings will result in genetic improvement in scrotal circumference at 12 months.
Similar favourable, moderate genetic correlations were found between SC and EMA (0.24, Table 5). This genetic correlation is similar to the 0.28 reported in Nellore and 0.42 in Angus (Yokoo et al. 2010; Vesela et al. 2022), which were both adjusted for age of scanning but not weight. Corbet et al. (2013) reported a lower genetic correlation in both Brahmans and Tropical Composites of 0.16 and 0.09 respectively. The lower genetic correlation could be due to the smaller variation in EMA and SC in the Corbet et al. (2013) study than herein. It also should be noted that Corbet et al. (2013) measured the ultrasound traits at 15 months, not as yearlings.
Rib fat was favourably correlated with SC (0.24), similar to the correlation reported by Vesela et al. (2022) of 0.20 in Angus and by Buzanskas et al. (2017) of 0.31 in Nellore. Not all breeding objectives will want greater fat, as excessive improvement leads to lower carcass meat yield. However, this correlation indicates that rib fat may be linked to attainment of puberty and increased fertility in harsh climates (such as tropical). These genetic correlations of EMA and rib fat with SC indicate that they both have a positive genetic influence over SC, indicating a potential for indirect selection on fertility through greater muscle and fat.
The genetic correlation between P8 fat and SC was very low (0.06). In Angus, the genetic correlation between these two traits was higher, at 0.19, than reported herein (Vesela et al. 2022). However, the opposite correlation was found in Brahmans and Tropical Composites at −0.13 and −0.21 respectively (Corbet et al. 2013). The difference in genetic correlations between the two breed types could be due to a genotype by environment interaction (G × E), as Brahmans and Angus are typically raised in different environments, this could lead to changes in gene expression. In Angus, P8 and SC share genes that influence each other, but this is not the same in Brahmans or Tropical Composites. A further explanation is the amount of variation in fat depth, with Angus in temperate environments typically having more variation than Tropical Composites, resulting in different genetic correlations between the breed types. A further study estimated the genetic correlation between P8 fat and SC to be 0.05 in Nellore (Buzanskas et al. 2017), being similar to the genetic correlation estimated herein. The wide range of genetic correlation estimates indicates a genotype difference between breeds. As far as the authors are aware, there has been no previous publication of genetic correlations between IMF and SC in tropical cattle. The genetic correlation between these traits in this Tropical Composite population was −0.14 (Table 5), indicating an antagonist relationship. In Angus, the genetic correlation was 0.60 (Vesela et al. 2022), which is stronger than the estimate for this population. Again, these differences between breeds could be due to a genotype difference or a G × E effect.
Sheath score is based on the angle of the prepuce, the vertical distance from the adnominal wall to the prepucial orifice and the size of the umbilical area. Sheath score is important, particularly in Bos indicus breed types, as an animal with larger sheath areas is more prone to injury, causing mating issues. The genetic relationship between sheath score and other traits is poorly understood, particularly with yearling composition traits. The genetic correlation between sheath and YWT was 0.05, demonstrating little association between the two traits; this is similar to the genetic correlation reported by Bignardi et al. (2011) in Nellore between navel score and YWT (0.06). The genetic correlation between Rib and Sheath estimated herein (−0.05; Table 5) is very similar to previous published genetic correlations of −0.06 (Gordo et al. 2012). The genetic correlations for P8 and EMA with sheath score (−0.20 and −0.34 respectively, Table 5) were stronger than the previously published estimates of 0.007 for P8 and −0.14 for EMA in Nellore cattle (Gordo et al. 2012). The genetic correlations between EMA and sheath score for Tropical Composites and Nellore cattle are similar, but P8 resulted in different correlations. Corbet et al. (2013) also estimated genetic correlations between sheath score at 18 months and P8 at 15 months and evaluated sheath score on a 1–9 scale, with nine being the tightest, so the genetic correlations have been flipped to match the results herein. Corbet et al. (2013) estimated a genetic correlation of −0.07 in Tropical Composites and −0.17 in Brahmans with P8 and sheath score, demonstrating that there is a wide range of genetic correlations across breeds. The genetic correlations estimated herein between yearling composition traits and sheath score are generally favourable, but low, allowing breeding programs to improve both traits with no detrimental effects on each other.
Percentage normal sperm (PNS) is considered the best practical measure of calf output in bulls and an important male fertility trait (Fitzpatrick et al. 2002). PNS has been reported to account for 35–57% of the variation in calf output among bulls (Holroyd et al. 2002). There has been little investigation into the relationship between PNS and yearling composition traits. The genetic correlation between PNS and YWT was −0.07, which is an unfavourable correlation, but very low. Smith et al. (1989) estimated a higher favourable genetic correlation between YWT and PNS to be 0.26 in Angus. Despite this favourable genetic correlation in Angus, a negative correlation was estimated in Tropical Composites of −0.21 between YWT and SC at 12 months (Corbet et al. 2009). This large range in genetic correlation could be due to genotype differences (indicine vs taurine), with the current study falling between the two genetic correlations. The genetic correlation estimated by Corbet et al. (2013) were −0.06 between SC at 12 months and weight at 15 months; despite being an older weight than the study herein, the genetic correlation was similar. The genetic correlation found herein indicates that PNS and YWT have little genetic influence, and genetic progress can be made in both without affecting each other.
Genetic correlations were also estimated between PNS at 12 months and yearling ultrasound composition traits. The highest genetic correlations were between EMA and P8, which both had a genetic correlation of 0.20 and 0.31 with PNS respectively (Table 5). Corbet et al. (2013) estimated genetic correlations between PNS at different ages and ultrasound composition traits at 15 months. For Tropical Composites, the genetic correlation was 0.08 with P8 and −0.07 with EMA for 12-month-old PNS. The genetic correlation reported by Corbet et al. (2013) for Tropical Composites was much lower than that in the study herein; this could be due to the age of ultrasound measurements or the small variation in the trait. At 18 months, the genetic correlations between PNS with EMA and P8 were 0.24 and 0.25 respectively, for Brahmans (Corbet et al. 2013). These genetic correlations were similar to those estimated herein, though the age of measurement is different. The similarity of the genetic correlations could be due to the age of puberty between the two breed types, with very few Brahmans at 12 months being able to produce an ejaculate compared with the Tropical Composites (Corbet et al. 2013). The genetic correlations between PNS and yearling composition traits were generally favourable, with low to moderate values indicating that, genetically, improving yearling composition traits will positively influence PNS in the breeding program.
Despite sperm abnormalities being important in the ability of the bull to reproduce, there has been little investigation into the genetic background behind these abnormalities and their relationship with yearling composition traits. The genetic correlation estimates between sperm morphology traits and YWT were generally low to not correlated, indicating that the two traits are genetically unrelated. Despite no previously published genetic correlation with specific sperm abnormalities and YWT, Smith et al. (1989) estimated the genetic correlation between primary and secondary abnormalities in yearling bulls to be 0.17 and −0.30 respectively, with YWT. Primary abnormalities are a defect of the head, and secondary abnormalities are slight defects of the tail. These genetic correlations are stronger than the study herein; however, they had large standard errors and a small sample size.
The ultrasound composition traits vary greatly within the genetic and phenotypic correlations with sperm morphology traits. In general, P8 and rib fat depth had a moderate–high favourable genetic correlation with semen morphology. The only exception is for KA, which had a genetic correlation of 0.07 with P8 and 0.06 with Rib (Table 5). The results indicated that fat at 12 months may be an indicator of puberty attainment. Herein, there was not an age of puberty measurement; however, it is assumed that it is linked to fewer sperm abnormalities at 12 months. These correlations were particularly strong for proximal droplet (PD) and vacuole/teratoid (VT) abnormalities. PD are usually observed in peri-pubertal bulls, with the incidence decreasing with age, and are considered a major cause of infertility in sperm, as PD is associated with early embryonic death (Barth and Oko 1989; Perry et al. 2002; Perry 2018). PD was highly heritable herein (0.39, Table 5) and by Roberts et al. (2010). These high heritabilities, genetic correlations and increased incidence at young ages would indicate that PD is a measure of the age of puberty in bulls, a possible indicator trait for the more difficult-to-measure ovarian scanning in heifers. While puberty in bulls is described at the time bulls provide an ejaculate with 50 × 106 sperm and 10% motility (Wolf et al. 1965), a single measurement of PD could be used as a proxy for bull puberty, meaning the bulls would need to be tested only once at 12 months. VT occur during spermiogenesis caused by extreme temperatures or stress; however, some bulls are more susceptible to this condition and, in some incidences, VT is thought to be heritable (Entwistle and Fordyce 2003), with the heritability of VT herein 0.16. The high genetic correlations with P8 and Rib (−0.77 and −0.84 respectively; Table 5) would indicate that animals with genetically more fat are genetically less predisposed to being stressed, reducing the incidence of this abnormality. Favourable moderate genetic correlations were also estimated between EMA and PD and VT, indicating that not only fat is correlated with PD and VT, but ultrasound muscle at 12 months is as well (−0.23 and −0.61 respectively). These genetic correlations would allow for selection on both fat and muscle at 12 months to genetically improve sperm morphology traits, particularly PD and VT, through correlated responses.
Despite the favourable genetic trend between fat (P8 and Rib) and muscle with sperm abnormalities, the trend was not as strong for IMF. IMF was favourably moderately genetically correlated with PD, SA and VT, indicating that selecting animals that genetically improve IMF will result in a reduction in those sperm abnormalities. However, the opposite was found between IMF and DMR, with a genetic correlation of 0.39. This unfavourable moderate genetic correlation would indicate that as IMF is genetically increased, it will engender a correlated response of more DMR. DMR is the most common defect seen in bulls and may arise due to cold shock or other environmental stresses, although it is thought to have a genetic disposition (McGowan 1995; Perry et al. 2002), the heritability herein was 0.18. Consideration must be made when selecting on IMF, as it has both favourable and unfavourable associations with sperm morphology traits.
Covariance between yearling heifer days to calving and male traits
The interplay between male and female fertility traits was proposed by Land (1973) in mammals, and several studies on beef have since investigated the genetic relationship and suggested that male traits could be a useful predictor of female fertility (Brinks et al. 1978; Smith et al. 1989; Johnston et al. 2014b). Scrotal circumference has previously been reported to be correlated with the age of puberty to varying extents (Brinks et al. 1978; Martin et al. 1992; Gargantini et al. 2005). These genetic correlations are important in a breeding program, as measuring SC is easy and inexpensive compared with age of puberty, allowing for indirect selection for the age of puberty in heifer offspring. Despite numerous studies highlighting the correlation between the age of puberty and SC, there have been few studies in heifer days to calving (DC1) and SC. The genetic correlation estimated herein was favourable and moderate (−0.32). These genetic correlations were similar to those in previously published studies. Johnston et al. (2014b) estimated the genetic correlation between DC1 and SC at 12 months to be −0.30 in Brahmans and −0.18 in Tropical Composites, indicating that selection for large SC would result in a genetically correlated response of reduced heifer days to calving through indirect selection. Johnston et al. (2014b) also estimated the genetic correlation between heifer pregnancy rate and SC to be 0.16 in Brahmans and 0.19 in Tropical Composites, with higher correlations with heifer weaning rate of 0.32 in Brahmans and 0.28 in Tropical Composites, further demonstrating the positive association between SC and heifer breeding success. Other studies have estimated genetic correlations between SC and an ‘overall days to calving’ treated as a repeatable trait with all joinings included, which ranged from −0.10 to −0.41 (Meyer et al. 1991; Forni and Albuquerque 2005), indicating that the relationship holds when mature joinings are considered.
Despite a favourable genetic correlation estimated between SC and DC1, a low unfavourable correlation was estimated between PNS and DC1 of 0.19. Johnston et al. (2014b) estimated a similar unfavourable genetic correlation in Tropical Composites between PNS at 12 months and DC1 of 0.10. However, in that study, PNS at 18 months had a favourable correlation of −0.50. These results indicated that PNS at 12 months is not a good selection criterion of DC1; however, there is a higher genetic association between DC1 and PNS at 18 months. It should be noted in Johnston et al. (2014b) heifers were not joined until 2-year olds; however, herein, they were joined at 12–15 months of age. Despite the age of first mating difference between the two studies, a similar genetic correlation was estimated, indicating that PNS has an unfavourable correlation with DC1 and cannot be used as indirect selection to improve heifer fertility. Johnston et al. (2014b) also estimated that genetic correlation between PNS and age of puberty, with similar results to DC1. Age of puberty and PNS at 12 months had a genetic correlation of −0.05, whereas at 18 months, they had a genetic correlation of −0.24 in Tropical Composites, further indicating that PNS at 12 months is not a good selection criterion for attainment of puberty. There was also an unfavourable genetic correlation estimated between sperm abnormalities and DC1. These unfavourable genetic correlations would indicate that sperm morphology at 12 months cannot be used as indirect selection for yearling heifer days to calving. Wolcott et al. (2018) estimated the genetic correlation between mid-piece defects, proximal droplets and abnormal heads and tails at 12 months and age of puberty to be −0.17, 0.13 and −0.05 respectively in a Tropical Composite population. This indicates that these male traits are not good indicators of female age of puberty. Therefore, in breeding programs it is important to record both yearling male and female fertility traits to ensure that improvement can be made in both.
Conclusions
Genetics plays an important role in fertility traits, particularly in those measured as yearlings and understanding the genetic and phenotypic relationship with composition traits is important for breeding programs. The genetic correlations estimated for yearling heifer days to calving suggest a favourable association with muscle, but little to no association with weight, and an unfavourable association with fat-related yearling ultrasound traits. Scrotal circumference had a moderately favourably genetic association with yearling weight, muscle and fat, all indicators of attainment of puberty. In general, sperm morphology traits were favourably genetically correlated with fat and muscle but not weight. The genetic correlations estimated between male and female traits indicated that scrotal circumference is useful as an indirect selection criterion for improving heifer days to calving; however, this is not true for the sperm morphology traits, which were low and unfavourable.
Data availability
Third-Party Data. Restrictions apply to the availability of these data. Data were obtained from Popplewell Composites Pty Ltd and are available with the permission of Greg Popplewell.
Conflicts of interest
The authors declare no conflicts of interest.
Declaration of funding
The primary author received scholarships from the University of Adelaide and Popplewell Composites.
Acknowledgements
The authors gratefully acknowledge the contributions of Greg Popplewell and all farm and technical staff involved in farm management practices and data collection.
References
Barth AD, Oko RJ (1989) ‘Abnormal morphology of bovine spermatozoa.’ (Iowa State University Press: Ames, IA, USA)Bignardi AB, Gordo DGM, Albuquerque LG, Sesana JC (2011) Genetics parameters for navel visual score in Nellore cattle. Arquivo Brasileiro de Medicina Veterinária e Zootecnia 63, 941–947.
| Genetics parameters for navel visual score in Nellore cattle.Crossref | GoogleScholarGoogle Scholar |
Boichard D, Chung H, Dassonneville R, David X, Eggen A, Fritz S, Gietzen KJ, Hayes BJ, Lawley CT, Sonstegard TS, Van Tassell CP, VanRaden PM, Viaud-Martinez KA, Wiggans GR, for the Bovine LD Consortium (2012) Design of a bovine low-density SNP array optimized for imputation. PLoS ONE 7, e34130
| Design of a bovine low-density SNP array optimized for imputation.Crossref | GoogleScholarGoogle Scholar |
Boligon AA, Albuquerque LG (2011) Genetic parameters and relationships of heifer pregnancy and age at first calving with weight gain, yearling and mature weight in Nelore cattle. Livestock Science 141, 12–16.
| Genetic parameters and relationships of heifer pregnancy and age at first calving with weight gain, yearling and mature weight in Nelore cattle.Crossref | GoogleScholarGoogle Scholar |
Boligon AA, Silva JAV, Sesana RC, Sesana JC, Junqueira JB, Albuquerque LG (2010) Estimation of genetic parameters for body weights, scrotal circumference, and testicular volume measured at different ages in Nellore cattle. Journal of Animal Science 88, 1215–1219.
| Estimation of genetic parameters for body weights, scrotal circumference, and testicular volume measured at different ages in Nellore cattle.Crossref | GoogleScholarGoogle Scholar |
Brinks JS, McInerney MJ, Chenoweth PJ (1978) Relationship of age at puberty in heifers to reproductive traits in young bulls. Proceedings of Western Section American Society of Animal Science 29, 28–30.
Burrow HM (2001) Variances and covariances between productive and adaptive traits and temperament in a composite breed of tropical beef cattle. Livestock Production Science 70, 213–233.
| Variances and covariances between productive and adaptive traits and temperament in a composite breed of tropical beef cattle.Crossref | GoogleScholarGoogle Scholar |
Butler DG, Cullis BR, Gilmour AR, Gogel BJ, Thompson R (2017) ‘ASReml-R reference manual version 4.’ (VSN International Ltd: Hemel Hempstead, UK)
Buzanskas ME, Pires PS, Chud TCS, Bernardes PA, Rola LD, Savegnago RP, Lôbo RB, Munari DP (2017) Parameter estimates for reproductive and carcass traits in Nelore beef cattle. Theriogenology 92, 204–209.
| Parameter estimates for reproductive and carcass traits in Nelore beef cattle.Crossref | GoogleScholarGoogle Scholar |
Caetano SL, Savegnago RP, Boligon AA, Ramos SB, Chud TCS, Lôbo RB, Munari DP (2013) Estimates of genetic parameters for carcass, growth and reproductive traits in Nellore cattle. Livestock Science 155, 1–7.
| Estimates of genetic parameters for carcass, growth and reproductive traits in Nellore cattle.Crossref | GoogleScholarGoogle Scholar |
Cammack KM, Thomas MG, Enns RM (2009) Reproductive traits and their heritabilities in beef cattle. The Professional Animal Scientist 25, 517–528.
| Reproductive traits and their heritabilities in beef cattle.Crossref | GoogleScholarGoogle Scholar |
Chenoweth PJ (2002) Objectives and outcomes from selection and management of fertile bulls. In ‘Bull fertility: selection and management in Australia’. (Ed. G Fordyce) (Australian Association of Cattle Veterinarians: Indooroopilly, Qld, Australia)
Chilcott C, Ash A, Lehnert S, Stokes C, Charmley E, Collins K, Pavey C, Macintosh A, Simpson A, Berglas R, White E, Amity M (2020) Northern Australia beef situation analysis: a report to the cooperative research centre for developing Northern Australia. CRC for Developing Northern Australia (CRCNA).
Corbet NJ, Burns BM, Corbet DH, Johnston DJ, Crisp JM, McGowan MR, Prayaga KC, Venus BK, Holroyd RG (2009) Genetic variation in growth, hormonal and seminal traits of young tropically adapted bulls. In ‘Proceedings of the association for the advancement of animal breeding and genetics, Vol. 18’, pp. 121–124. (Association for the Advancement of Animal Breeding and Genetics)
Corbet NJ, Burns BM, Johnston DJ, Wolcott ML, Corbet DH, Venus BK, Li Y, McGowan MR, Holroyd RG (2013) Male traits and herd reproductive capability in tropical beef cattle. 2. Genetic parameters of bull traits. Animal Production Science 53, 101–113.
| Male traits and herd reproductive capability in tropical beef cattle. 2. Genetic parameters of bull traits.Crossref | GoogleScholarGoogle Scholar |
Davis GP (1993) Genetic parameters for tropical beef cattle in northern Australia: a review. Australian Journal of Agricultural Research 44, 179–198.
| Genetic parameters for tropical beef cattle in northern Australia: a review.Crossref | GoogleScholarGoogle Scholar |
Eler JP, Silva JAIIV, Evans JL, Ferraz JBS, Dias F, Golden BL (2004) Additive genetic relationships between heifer pregnancy and scrotal circumference in Nellore cattle. Journal of Animal Science 82, 2519–2527.
| Additive genetic relationships between heifer pregnancy and scrotal circumference in Nellore cattle.Crossref | GoogleScholarGoogle Scholar |
Entwistle KW, Fordyce G (2003) ‘Evaluating & reporting bull fertility.’ (Australian Association of Cattle Veterinarians: Indooroopilly, Qld, Australia)
Facy ML, Hebart ML, Oakey H, McEwin RA, Pitchford WS (2023) Genetic correlations between days to calving across joinings and lactation status in a tropically adapted composite beef herd. Agriculture 13, 37
| Genetic correlations between days to calving across joinings and lactation status in a tropically adapted composite beef herd.Crossref | GoogleScholarGoogle Scholar |
Fitzpatrick LA, Fordyce G, McGowan MR, Bertram JD, Doogan VJ, De Faveri J, Miller RG, Holroyd RG (2002) Bull selection and use in northern Australia: part 2. Semen traits. Animal Reproduction Science 71, 39–49.
| Bull selection and use in northern Australia: part 2. Semen traits.Crossref | GoogleScholarGoogle Scholar |
Forni S, Albuquerque LG (2005) Estimates of genetic correlations between days to calving and reproductive and weight traits in Nelore cattle. Journal of Animal Science 83, 1511–1515.
| Estimates of genetic correlations between days to calving and reproductive and weight traits in Nelore cattle.Crossref | GoogleScholarGoogle Scholar |
Gargantini G, Cundiff LV, Lunstra DD, Van Vleck LD (2005) Genetic relationships between male and female reproductive traits in beef cattle. The Professional Animal Scientist 21, 195–199.
| Genetic relationships between male and female reproductive traits in beef cattle.Crossref | GoogleScholarGoogle Scholar |
Garmyn AJ, Moser DW, Christmas RA, Minick Bormann J (2011) Estimation of genetic parameters and effects of cytoplasmic line on scrotal circumference and semen quality traits in Angus bulls. Journal of Animal Science 89, 693–698.
| Estimation of genetic parameters and effects of cytoplasmic line on scrotal circumference and semen quality traits in Angus bulls.Crossref | GoogleScholarGoogle Scholar |
Gordo DGM, Baldi F, Lôbo RB, Filho WK, Sainz RD, Albuquerque LG (2012) Genetic association between body composition measured by ultrasound and visual scores in Brazilian Nelore cattle. Journal of Animal Science 90, 4223–4229.
| Genetic association between body composition measured by ultrasound and visual scores in Brazilian Nelore cattle.Crossref | GoogleScholarGoogle Scholar |
Graser H-U, Tier B, Johnston DJ, Barwick SA (2005) Genetic evaluation for the beef industry in Australia. Australian Journal of Experimental Agriculture 45, 913–921.
| Genetic evaluation for the beef industry in Australia.Crossref | GoogleScholarGoogle Scholar |
Holroyd RG, Doogan VJ, De Faveri J, Fordyce G, McGowan MR, Bertram JD, Vankan DM, Fitzpatrick LA, Jayawardhana GA, Miller RG (2002) Bull selection and use in northern Australia. 4. Calf output and predictors of fertility of bulls in multiple-sire herds. Animal Reproduction Science 71, 67–79.
| Bull selection and use in northern Australia. 4. Calf output and predictors of fertility of bulls in multiple-sire herds.Crossref | GoogleScholarGoogle Scholar |
Jeyaruban G, Johnston D (2017) Genetic association of young male traits with female reproductive performance in Brahman and Santa Gertrudis cattle. In ‘Proceedings of the association for the advancement of animal breeding and genetics, Vol. 22’, pp. 305–308. (Association for the Advancement of Animal Breeding and Genetics)
Johnston DJ, Bunter KL (1996) Days to calving in Angus cattle: genetic and environmental effects, and covariances with other traits. Livestock Production Science 45, 13–22.
| Days to calving in Angus cattle: genetic and environmental effects, and covariances with other traits.Crossref | GoogleScholarGoogle Scholar |
Johnston DJ, Barwick SA, Corbet NJ, Fordyce G, Holroyd RG, Williams PJ, Burrow HM (2009) Genetics of heifer puberty in two tropical beef genotypes in northern Australia and associations with heifer- and steer-production traits. Animal Production Science 49, 399
| Genetics of heifer puberty in two tropical beef genotypes in northern Australia and associations with heifer- and steer-production traits.Crossref | GoogleScholarGoogle Scholar |
Johnston DJ, Barwick SA, Fordyce G, Holroyd RG, Williams PJ, Corbet NJ, Grant T (2014a) Genetics of early and lifetime annual reproductive performance in cows of two tropical beef genotypes in northern Australia. Animal Production Science 54, 1–15.
| Genetics of early and lifetime annual reproductive performance in cows of two tropical beef genotypes in northern Australia.Crossref | GoogleScholarGoogle Scholar |
Johnston DJ, Corbet NJ, Barwick SA, Wolcott ML, Holroyd RG (2014b) Genetic correlations of young bull reproductive traits and heifer puberty traits with female reproductive performance in two tropical beef genotypes in northern Australia. Animal Production Science 54, 74–84.
| Genetic correlations of young bull reproductive traits and heifer puberty traits with female reproductive performance in two tropical beef genotypes in northern Australia.Crossref | GoogleScholarGoogle Scholar |
Kastelic JP, Thundathil JC (2008) Breeding soundness evaluation and semen analysis for predicting bull fertility. Reproduction in Domestic Animals 43, 368–373.
| Breeding soundness evaluation and semen analysis for predicting bull fertility.Crossref | GoogleScholarGoogle Scholar |
Kealey CG, MacNeil MD, Tess MW, Geary TW, Bellows RA (2006) Genetic parameter estimates for scrotal circumference and semen characteristics of Line 1 Hereford bulls. Journal of Animal Science 84, 283–290.
| Genetic parameter estimates for scrotal circumference and semen characteristics of Line 1 Hereford bulls.Crossref | GoogleScholarGoogle Scholar |
Land RB (1973) The expression of female sex-limited characters in the male. Nature 241, 208–209.
| The expression of female sex-limited characters in the male.Crossref | GoogleScholarGoogle Scholar |
Martin LC, Brinks JS, Bourdon RM, Cundiff LV (1992) Genetic effects on beef heifer puberty and subsequent reproduction. Journal of Animal Science 70, 4006–4017.
| Genetic effects on beef heifer puberty and subsequent reproduction.Crossref | GoogleScholarGoogle Scholar |
McGowan MR (1995) ‘The veterinary examination of bulls.’ (Australian Association of Cattle Veterinarians: Indooroopilly, Qld, Australia)
Mercadante MEZ, Packer IU, Razook AG, Cyrillo JNSG, Figueiredo LA (2003) Direct and correlated responses to selection for yearling weight onreproductive performance of Nelore cows. Journal of Animal Science 81, 376–384.
| Direct and correlated responses to selection for yearling weight onreproductive performance of Nelore cows.Crossref | GoogleScholarGoogle Scholar |
Meyer K, Hammond K, Parnell PF, Mackinnon MJ, Sivarajasingam S (1990) Estimates of heritability and repeatability for reproductive traits in Australian beef cattle. Livestock Production Science 25, 15–30.
| Estimates of heritability and repeatability for reproductive traits in Australian beef cattle.Crossref | GoogleScholarGoogle Scholar |
Meyer K, Hammond K, Mackinnon MJ, Parnell PF (1991) Estimates of covariances between reproduction and growth in Australian beef cattle. Journal of Animal Science 69, 3533–3543.
| Estimates of covariances between reproduction and growth in Australian beef cattle.Crossref | GoogleScholarGoogle Scholar |
Morris CA, Wilson JA, Bennett GL, Cullen NG, Hickey SM, Hunter JC (2000) Genetic parameters for growth, puberty, and beef cow reproductive traits in a puberty selection experiment. New Zealand Journal of Agricultural Research 43, 83–91.
| Genetic parameters for growth, puberty, and beef cow reproductive traits in a puberty selection experiment.Crossref | GoogleScholarGoogle Scholar |
Mucari TB, Alencar MMd, Barbosa PF, Barbosa RT (2007) Genetic analyses of days to calving and their relationships with other traits in a Canchim cattle herd. Genetics and Molecular Biology 30, 1070–1076.
| Genetic analyses of days to calving and their relationships with other traits in a Canchim cattle herd.Crossref | GoogleScholarGoogle Scholar |
Naserkheil M, Lee D-H, Kong H-S, Seong J, Mehrban H (2021) Estimation of genetic parameters and correlation between yearling ultrasound measurements and carcass traits in hanwoo cattle. Animals 11, 1425
| Estimation of genetic parameters and correlation between yearling ultrasound measurements and carcass traits in hanwoo cattle.Crossref | GoogleScholarGoogle Scholar |
Perry V (2018) A refresher on sperm morphology. In ‘Proceedings of AVA annual conference’. (Australian Veterinary Association: Brisbane, Qld, Australia)
Perry V, Phillips N, Fordyce G, Gardiner B, Entwistle K, Chenoweth P, Doogan VJ (2002) Semen collection and evaluation. In ‘Bull fertility: selection and management in Australia’. (Ed. G Fordyce) (Australian Association of Cattle Veterinarians: Indooroopilly, Qld, Australia)
Pitchford WS, Pitchford JM, Alexopoulos JG, Hebart ML (2022) Genomic analysis of purebred and crossbred angus cows quantifies heterozygosity, breed, and additive effects on components of reproduction. Animals 12, 61
| Genomic analysis of purebred and crossbred angus cows quantifies heterozygosity, breed, and additive effects on components of reproduction.Crossref | GoogleScholarGoogle Scholar |
Raidan FSS, Porto-Neto LR, Reverter A (2019) Across-sex genomic-assisted genetic correlations for sex-influenced traits in Brahman cattle. Genetics Selection Evolution 51, 41
| Across-sex genomic-assisted genetic correlations for sex-influenced traits in Brahman cattle.Crossref | GoogleScholarGoogle Scholar |
R Core Team (2021) ‘R: a language and environment for statistical computing.’ (R Foundation for Statistical Computing: Vienna, Austria)
Reverter A, Johnston DJ, Graser H-U, Wolcott ML, Upton WH (2000) Genetic analyses of live-animal ultrasound and abattoir carcass traits in Australian Angus and Hereford cattle. Journal of Animal Science 78, 1786–1795.
| Genetic analyses of live-animal ultrasound and abattoir carcass traits in Australian Angus and Hereford cattle.Crossref | GoogleScholarGoogle Scholar |
Roberts C, Geary T, MacNeil M, Waterman R, Roberts A, Alexander L (2010) Factors affecting spermatozoa morphology in beef bulls. In ‘Proceedings of western section American society of animal science’. (American Society of Animal Science)
RStudio (2022) ‘RStudio: intergrated development environment for R.’ (RStudio, PBC: Boston, MA, USA)
Sargolzaei M, Chesnais JP, Schenkel F (2011) FImpute – an efficient imputation algorithm for dairy cattle populations. Journal of Dairy Science 94, 421
Schatz TJ (2011) Understanding and improving heifer fertility in Northern Australia. Master’s Thesis, Charles Darwin University.
Smith BA, Brinks JS, Richardson GV (1989) Estimation of genetic parameters among breeding soundness examination components and growth traits in yearling bulls. Journal of Animal Science 67, 2892–2896.
| Estimation of genetic parameters among breeding soundness examination components and growth traits in yearling bulls.Crossref | GoogleScholarGoogle Scholar |
Stelzleni AM, Perkins TL, Brown AH, Pohlman FW, Johnson ZB, Sandelin BA (2002) Genetic parameter estimates of yearling live animal ultrasonic measurements in Brangus cattle. Journal of Animal Science 80, 3150–3153.
| Genetic parameter estimates of yearling live animal ultrasonic measurements in Brangus cattle.Crossref | GoogleScholarGoogle Scholar |
VanRaden PM (2008) Efficient methods to compute genomic predictions. Journal of Dairy Science 91, 4414–4423.
| Efficient methods to compute genomic predictions.Crossref | GoogleScholarGoogle Scholar |
Vesela Z, Birovas A, Brzakova M, Novotna A, Vostry L (2022) Genetic parameters for live animal ultrasound measures, scrotal circumference, carcass and growth traits in Aberdeen Angus. Interbull Bulletin 57, 58–62.
Weik F, Hickson RE, Morris ST, Garrick DJ, Archer JA (2022) genetic parameters for growth, ultrasound and carcass traits in New Zealand beef cattle and their correlations with maternal performance. Animals 12, 25
| genetic parameters for growth, ultrasound and carcass traits in New Zealand beef cattle and their correlations with maternal performance.Crossref | GoogleScholarGoogle Scholar |
Wolcott ML, Johnston DJ, Barwick SA, Corbet NJ, Williams PJ (2014a) The genetics of cow growth and body composition at first calving in two tropical beef genotypes. Animal Production Science 54, 37–49.
| The genetics of cow growth and body composition at first calving in two tropical beef genotypes.Crossref | GoogleScholarGoogle Scholar |
Wolcott ML, Johnston DJ, Barwick SA (2014b) Genetic relationships of female reproduction with growth, body composition, maternal weaning weight and tropical adaptation in two tropical beef genotypes. Animal Production Science 54, 60–73.
| Genetic relationships of female reproduction with growth, body composition, maternal weaning weight and tropical adaptation in two tropical beef genotypes.Crossref | GoogleScholarGoogle Scholar |
Wolcott ML, Johnston DJ, Corbet NJ, Venus BK (2018) Sperm abnormality traits can contribute to the genetic evaluation for male and female reproduction in tropical beef genotypes. In ‘Proceedings of the world congress on genetics applied to livestock production’. (Massey University: Palmerston North, New Zealand)
Wolf FR, Almquist JO, Hale EB (1965) Prepuberal Behavior and Puberal Characteristics of Beef Bulls on High Nutrient Allowance. Journal of Animal Science 24, 761–765.
| Prepuberal Behavior and Puberal Characteristics of Beef Bulls on High Nutrient Allowance.Crossref | GoogleScholarGoogle Scholar |
Yilmaz A, Davis ME, Simmen RCM (2004) Estimation of (co)variance components for reproductive traits in Angus beef cattle divergently selected for blood serum IGF-I concentration. Journal of Animal Science 82, 2285–2292.
| Estimation of (co)variance components for reproductive traits in Angus beef cattle divergently selected for blood serum IGF-I concentration.Crossref | GoogleScholarGoogle Scholar |
Yokoo MJ, Lobo RB, Araujo FRC, Bezerra LAF, Sainz RD, Albuquerque LG (2010) Genetic associations between carcass traits measured by real-time ultrasound and scrotal circumference and growth traits in Nelore cattle. Journal of Animal Science 88, 52–58.
| Genetic associations between carcass traits measured by real-time ultrasound and scrotal circumference and growth traits in Nelore cattle.Crossref | GoogleScholarGoogle Scholar |