Is the laboratory report dead? AI and ChatGPT
Jack T. H. Wang A *A School of Chemistry and Molecular Biosciences, The University of Queensland, Brisbane, Qld 4072, Australia.
![]() Assoc. Prof. Jack Wang is a teaching-focused microbiologist at The University of Queensland. His work focuses on undergraduate research and technology-enabled assessment in science education. He was the recipient of the 2020 ASM David White Teaching Excellence award, and was named the 2020 Australian University Teacher of the year. |
Microbiology Australia 44(3) 144-148 https://doi.org/10.1071/MA23042
Submitted: 31 May 2023 Accepted: 20 June 2023 Published: 4 July 2023
© 2023 The Author(s) (or their employer(s)). Published by CSIRO Publishing on behalf of the ASM. This is an open access article distributed under the Creative Commons Attribution-NonCommercial-NoDerivatives 4.0 International License (CC BY-NC-ND)
Abstract
The launch of ChatGPT and artificial intelligence (AI) platforms capable of generating written responses to a vast range of text-based queries has transformed the conceptualisation of assessment in education. Apart from its potential for misuse in test and examinations, the laboratory report in Science Education may be vulnerable to AI-disruption. This article outlines five text-based prompts that educators can use to assess the quality of AI-generated output in scientific writing. When used to query the freely accessible version of ChatGPT (GPT-3.5) in June 2023, these prompts revealed its ability to produce written work that showcases high-level organisation of concepts relevant to a scientific topic. However, these AI-generated responses remain generalised, lacking specificity and without effective integration of peer-reviewed scientific literature. As these generative AI platforms continue to improve, educators can use this series of prompts to evaluate the quality of AI output and adapt the assessment criteria for this new era in scientific writing.
Keywords: artificial intelligence, assessment, Bloom’s taxonomy, ChatGPT, laboratory report.
Introduction
On 30 November 2022, Open AI launched ChatGPT (Generative Pre-trained Transformer), an artificial-intelligence- (AI) powered chat bot capable of generating human-like responses to text-based queries. This output is generated through statistical models built upon a vast corpus of online text. The language patterns embedded within the training data is what allows ChatGPT to use conversational language to respond to user queries across different genres of communication. ChatGPT generated responses to open-ended exam questions in physics that could not be distinguished from human submissions,1 and scored a passing grade with performance comparable to a third-year medical student in the standardised United States Medical Licensing Examination.2
Laboratory skills represent a significant component of the microbiology curriculum,3 and hands-on laboratory training cannot be completed by text-based AI chatbots. However, theoretical knowledge, problem-solving and communication skills underpin threshold learning outcomes for microbiology graduates,4 and these remain vulnerable to AI disruption. On-campus examinations that do not allow students access to an internet-connected device may resolve part of the problem, but invigilation is not readily scalable towards other forms of university assessment. Scientific reports that summarise, interpret and analyse experimental findings generated by students after a laboratory module represent the culmination of microbiology threshold learning outcomes. The viability of this assessment task in the face of ChatGPT will be the focus of this article.
ChatGPT and laboratory assessment
A hypothetical laboratory assignment on antibiotic resistance was designed to evaluate the extent to which ChatGPT can be used by students to complete laboratory reports on their behalf. The Antibiotic Resistance Report (ARR) covers an overarching concept within ASM’s introductory microbiology curriculum,3 and involves student completion of a laboratory module that tests the antibiotic sensitivity of specific strains of microbes against a range of antibiotics. The data collected from these experiments then form the basis of the ARR, where students need to interpret the sensitivity profiles and discuss the experimental findings compared to scientific literature. The report has five interconnected Learning Objectives (LOs) that span across different cognitive domains of learning complexity (Table 1), and when ordered from least to most complex using Bloom’s Taxonomy these are: Knowledge, Comprehension, Application, Analysis, Synthesis and Evaluation.5
Learning objective | Bloom’s taxonomy domain(s) |
---|---|
LO1. Explain the concept of antibiotic resistance and its significance in microbiology | Knowledge; comprehension |
LO2. Conduct disk diffusion assays that measure bacterial growth in response to a range of antibiotics | Application |
LO3. Develop a research question and hypothesis based on the experiments performed in LO2. | Analysis; synthesis |
LO4. Communicate antibiotic resistance data using in-text descriptions and graphical representations | Analysis; synthesis |
LO5. Compare and contrast results against scientific literature to identify significant trends | Analysis; synthesis; evaluation |
LO, learning objective.
Apart from LO2, which necessitates hands-on application of laboratory skills, the remainder of the assignment could theoretically be completed by generative AI. What teachers should interrogate more deeply are the series of prompts that students can pose to the most freely accessible version of AI at any point in time. To ask ChatGPT to complete the ARR on behalf of a student, five prompts were entered into the publicly accessible free version of ChatGPT (GPT-3.5) in June 2023. Individual instructors are encouraged to adapt these prompts towards assessment items in their own teaching contexts to observe how AI is improving over time.6
Prompt 1. Can you write a scientific report on testing antibiotic resistance across different bacteria?
This initial prompt is the ‘can you do my assignment for me’ umbrella query, which, given the lack of specificity, is unlikely to yield meaningful results. A snapshot of ChatGPT’s attempt at responding to this query is shown in Fig. 1. This response does not meet the passing threshold, but it does provide students with a sample report structure to build upon. The section that requires the least amount of human intervention appears to be the Materials and Methods section. Pending additional detail on bacterial strains, culture conditions and concentrations of antibiotics used, ChatGPT’s response can be readily edited to resemble a conventional methods section. The other sections require significant rewriting to align with scientific reporting standards.
Prompt 2. Can you develop a research question and hypothesis for a project on antibiotic resistance?
A sample ChatGPT response is below:
Research Question: What is the impact of prolonged antibiotic exposure on the development and prevalent of antibiotic resistance in a specific bacterial species?
Hypothesis: Prolonged exposure of a bacterial species to antibiotics will lead to an increased prevalence and level of antibiotic resistance due to the selective pressure exerted by the antibiotics.
Developing a research question and hypothesis requires more than knowledge and comprehension, but also necessitates higher order ‘Analysis’ and ‘Synthesis’ domains in Bloom’s taxonomy. ChatGPT’s research question and hypothesis is generalised and lacks specificity and will require further prompting by students to provide details on microbial strains and antibiotics being tested.
Prompt 3. Can you format these data into an appropriate type of graph?
In a normal learning sequence, students would generate their own experimental data for the ARR, but for this prompt a simulated zone of inhibition dataset was entered into ChatGPT (Table 2). ChatGPT misinterpreted the numerical values as percentage antibiotic resistance rates rather than zones of inhibition and suggests a clustered bar graph to visualise the data. It even attempted to draw this graph using dashes (Fig. 2). This misinterpretation can be corrected through additional prompts, but the text-based user interface is the main bottleneck that limits the use of AI to generate graphs and figures. This limitation may be short-lived, as Microsoft’s Copilot promises to integrate AI into the suite of Office 365 programs including Word, Powerpoint and Excel. The paid subscription version of ChatGPT Plus (GPT-4) also allows users to upload images as prompts; however, this functionality does not extend to any of the free AI chatbots (GPT-3.5, Bard, Bing) that all students have access to online.
Microbe | Replicate | Benzylpenicilin | Tetracycline | Erythromycin | Chloramphenicol |
---|---|---|---|---|---|
S. aureus | Replicate 1 | 38 | 34 | 34 | 32 |
Replicate 2 | 14 | 27 | 15 | 27 | |
Replicate 3 | 20 | 20 | 18 | 26 | |
E. coli | Replicate 1 | 8 | 30 | 19 | 32 |
Replicate 2 | 10 | 30 | 14 | 32 | |
Replicate 3 | 10 | 29 | 15 | 31 | |
K. pneumoniae | Replicate 1 | 0 | 32 | 13 | 31 |
Replicate 2 | 0 | 32 | 14 | 31 | |
Replicate 3 | 0 | 28 | 15 | 33 |
Three replicates were simulated for each experimental condition.
ChatGPT (GPT-3.5, accessed 1 June 2023) generated graph when prompted with simulated antibiotic resistance data from Table 2.
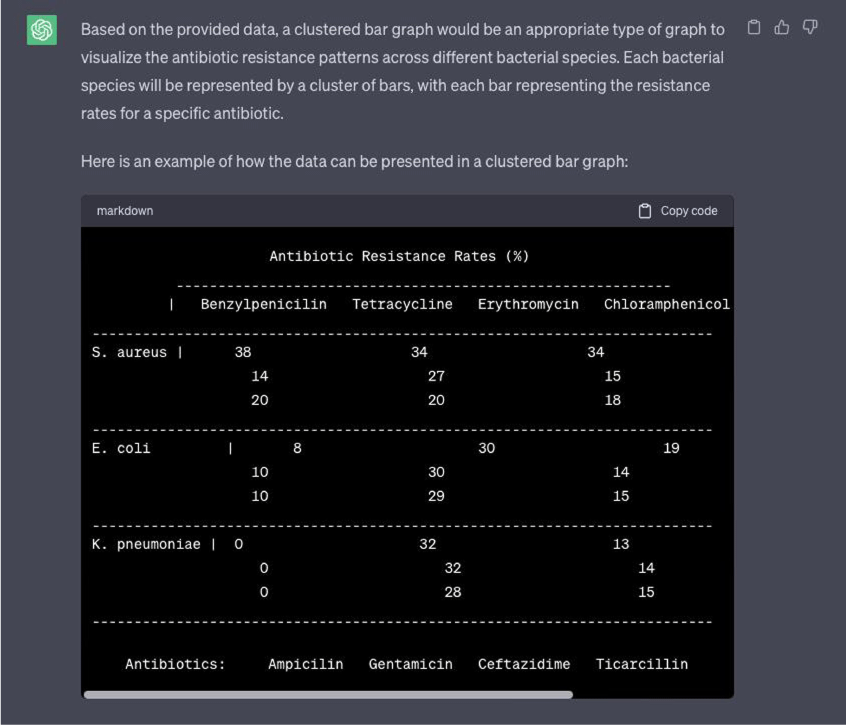
Prompt 4. Can you interpret this data and summarise the most significant findings?
ChatGPT’s interpretation of the data presented in Table 2 is as follows:
S. aureus: Benzylpenicillin, Tetracycline, Erythromycin and Chloramphenicol show relatively high resistance rates ranging from 32 to 38%.
E. coli: Benzylpenicillin, Erythromycin and Chloramphenicol demonstrate moderate resistance rates ranging from 19 to 32%.
K. pneumoniae: Tetracycline, Erythromycin and Chloramphenicol exhibit resistance rates ranging from 13 to 33%.
The findings highlight the antibiotic resistance data for each microbe, but do not make detailed comparisons between all three microbes.
Prompt 5. Can you find 10 peer-reviewed scientific studies published in the last 5 years that can explain the significance of antibiotic resistance?
This prompt was repeated three times, and ChatGPT (GPT-3.5) provided 30 studies in total. Twenty-one of these studies are fake and could not be found in Pubmed or Google Scholar databases; nine were real studies, albeit with erroneous journal names, incomplete author lists or incorrect year of publication. These results provide clear evidence of AI’s propensity for hallucinations – confident assertions fabricated by AI when presented with concepts outside of the scope of its training dataset.7
Conclusion
Scientific projects are open-ended and require significant human intervention to specify laboratory conditions and the relevant scientific context. Although the free and most accessible version of ChatGPT (GPT-3.5) can provide structure to a scientific report, formulating a hypothesis, visualising data and comparisons to peer-reviewed literature are all higher-order cognitive domains in Bloom’s taxonomy that are not readily approximated by AI. Students may be able to use ChatGPT to generate their first draft but will need a series of sophisticated and iterative prompts to refine the AI-generated text. Moreover, students should be strongly cautioned against entering primary research data into ChatGPT as this may transfer intellectual property to the AI platform. In research, journal editorial boards now need to contend with large volumes of AI-generated text.8 Although these AI-generated responses can be prone to hallucinations,7 their frequency has markedly decreased in Open AI’s next iteration, GPT-4.9
In addition to new software and AI-detection mechanisms,10 educators may also find value in pedagogical design. The five prompts outlined in this article can serve as a tool to establish the baseline AI response to a scientific report. Although students may utilise an unlimited number of prompts to refine the AI output, the weighting of assessment criterion should be considered relative to the quality of AI generated output to act as a deterrent for academic misconduct. For instance, ChatGPT’s attempts at the Introduction and Materials and Methods sections are closer to conventional report formatting than the Results and Discussion sections. Its attempts at data visualisation and identifying relevant scientific literature remain flawed for the time being, so assessment weighting across these sections should be adjusted accordingly. It is incumbent upon teachers to maintain currency with these technological innovations to ensure the ongoing evolution of science education.
References
1 Yeadon W et al. (2023) The death of the short-form physics essay in the coming AI revolution. Phys Educ 58, 035027.
| Crossref | Google Scholar |
2 Gilson A et al. (2023) How does ChatGPT perform on the United States Medical Licensing Examination? The implications of large language models for medical education and knowledge assessment. JMIR Med Educ 9, e45312.
| Crossref | Google Scholar |
3 Merkel S (2012) The development of curricular guidelines for introductory microbiology that focus on understanding. J Microbiol Biol Educ 13, 32-38.
| Crossref | Google Scholar |
4 Burke C et al. (2016) Threshold learning outcomes for a microbiology major. Microbiol Aust 37, 93-97.
| Crossref | Google Scholar |
5 Crowe A et al. (2008) Biology in bloom: implementing Bloom’s taxonomy to enhance student learning in biology. CBE Life Sci Educ 7, 368-381.
| Crossref | Google Scholar |
6 Sanderson K (2023) GPT-4 is here: what scientists think. Nature 615, 773.
| Crossref | Google Scholar |
7 Alkaissi H, McFarlane SI (2023) Artificial hallucinations in ChatGPT: implications in scientific writing. Cureus 15, e35179.
| Crossref | Google Scholar |
8 Thorp HH (2023) ChatGPT is fun, but not an author. Science 379, 313.
| Crossref | Google Scholar |
9 Lin JC et al. (2023) Comparison of GPT-3.5, GPT-4, and human user performance on a practice ophthalmology written examination. Eye 1-2.
| Crossref | Google Scholar |
10 Fergus S et al. (2023) Evaluating academic answers generated using chatgpt. J Chem Educ 100, 1672-1675.
| Crossref | Google Scholar |