Predicting unplanned readmission after myocardial infarction from routinely collected administrative hospital data
Santu Rana A , Truyen Tran A , Wei Luo A , Dinh Phung A , Richard L. Kennedy B and Svetha Venkatesh A CA Centre for Pattern Recognition and Data Analytics, Deakin University, Locked Bag 20000, Geelong, Vic. 3220, Australia. Email: santu.rana@deakin.edu.au; Truyen.tran@deakin.edu.au; wei.luo@deakin.edu.au; dinh.phung@deakin.edu.au
B School of Medicine, Deakin University, Locked Bag 20000, Geelong, Vic. 3220, Australia. Email: lee.kennedy@deakin.edu.au
C Corresponding author. Email: Svetha.venkatesh@deakin.edu.au
Australian Health Review 38(4) 377-382 https://doi.org/10.1071/AH14059
Submitted: 16 December 2013 Accepted: 18 April 2014 Published: 8 July 2014
Journal Compilation © AHHA 2014
Abstract
Objective Readmission rates are high following acute myocardial infarction (AMI), but risk stratification has proved difficult because known risk factors are only weakly predictive. In the present study, we applied hospital data to identify the risk of unplanned admission following AMI hospitalisations.
Methods The study included 1660 consecutive AMI admissions. Predictive models were derived from 1107 randomly selected records and tested on the remaining 553 records. The electronic medical record (EMR) model was compared with a seven-factor predictive score known as the HOSPITAL score and a model derived from Elixhauser comorbidities. All models were evaluated for the ability to identify patients at high risk of 30-day ischaemic heart disease readmission and those at risk of all-cause readmission within 12 months following the initial AMI hospitalisation.
Results The EMR model has higher discrimination than other models in predicting ischaemic heart disease readmissions (area under the curve (AUC) 0.78; 95% confidence interval (CI) 0.71–0.85 for 30-day readmission). The positive predictive value was significantly higher with the EMR model, which identifies cohorts that were up to threefold more likely to be readmitted. Factors associated with readmission included emergency department attendances, cardiac diagnoses and procedures, renal impairment and electrolyte disturbances. The EMR model also performed better than other models (AUC 0.72; 95% CI 0.66–0.78), and with greater positive predictive value, in identifying 12-month risk of all-cause readmission.
Conclusions Routine hospital data can help identify patients at high risk of readmission following AMI. This could lead to decreased readmission rates by identifying patients suitable for targeted clinical interventions.
What is known about the topic? Many clinical and demographic risk factors are known for hospital readmissions following acute myocardial infarction, including multivessel disease, high baseline heart rate, hypertension, diabetes, obesity, chronic obstructive pulmonary disease and psychiatric morbidity. However, combining these risk factors into indices for predicting readmission had limited success. A recent study reported a C-statistic of 0.73 for predicting 30-day readmissions. In a recent American study, a simple seven-factor score was shown to predict hospital readmissions among medical patients.
What does this paper add? This paper presents a way to predict readmissions following myocardial infarction using routinely collected administrative data. The model performed better than the recently described HOSPITAL score and a model derived from Elixhauser comorbidities. Moreover, the model uses only data generally available in most hospitals.
What are the implications for practitioners? Routine hospital data available at discharges can be used to tailor preventative care for AMI patients, to improve institutional performance and to decrease the cost burden associated with AMI.
Introduction
Nearly one patient in every five admitted to hospital with acute myocardial infarction (AMI) is readmitted within 30 days of discharge.1–3 Many of these readmissions are unplanned and avoidable. The high rate of unplanned readmissions detracts from the quality of patient care and institutional performance, and adds to the cost of managing AMI patients. Reducing unplanned readmissions requires identification of patients at high risk of readmission during their initial presentation, and tailored and targeted interventions on these patients.
To date, reliable risk stratification of AMI patients admitted has proved challenging despite several well-documented risk factors,4 including severe heart failure,4 multivessel disease,5 living alone,6 ethnic background,7 psychological comorbidities8,9 and socioeconomic factors.10 As with many clinical prediction problems, the known risk factors for readmission after AMI individually have only weak predictive ability.
Institutions that have adopted the electronic medical record (EMR) have access to a wealth of clinical and administrative data that, if used appropriately, could improve clinical care and institutional performance.11–13 In the present study we examined all the available administrative hospital factors associated with readmission in a cohort of patients admitted with AMI to a large regional hospital in Geelong (Vic., Australia). We used data from the EMR to derive and internally validate a model to predict unplanned ischaemic heart disease (IHD) readmissions over a 30-day period and all-cause readmission over 12 months after an admission with AMI. We defined an admission to be unplanned if the patient was admitted through the emergency department (ED). The EMR model was compared with the model derived from Elixhauser comorbidities and a recently described14 simple predictive scoring system (HOSPITAL) that was developed to predict unplanned admissions in patients admitted with acute medical conditions.
Methods
Datasets
The present study is a retrospective study using EMR of in-patient admissions and ED visits at Barwon Health, a regional health service in Australia. As the only public tertiary hospital in Greater Geelong, a catchment area with more than 350 000 residents, the hospital’s patient database provides a single point of access for information on hospitalisations, ED visits, medications and treatments. Detailed records of patient interactions with the hospital system are available through the EMR. This includes International Classification of Disease 10 (ICD-10),15 procedure and diagnosis-related Group (DRG)16 codes of each admission and ED visit; details of procedures; and departments involved in the patient’s care. Other information includes demographic data (age, gender and postcode) and details of access to primary care facilities. Ethics approval was obtained from the Hospital and Research Ethics Committee at Barwon Health (no. 12/83). Deakin University has reciprocal ethics authorisation with Barwon Health.
The patient cohort consisted of 1660 patients with a confirmed diagnosis of AMI admitted between January 2009 and December 2011. An AMI admission was defined by ICD-10 codes I21 (Acute myocardial infarction) or I22 (Subsequent myocardial infarction) in discharge diagnoses (either primary or secondary).17 In Australia, cardiac troponin (cTn)18 is the primary biomarker for AMI diagnosis.
For each patient, the index admission was defined to be the AMI admission of the patient starting from 1 January 2009. Readmission predictions were made (retrospectively) at the end of the index admission. Patient records before the index admission were used to construct independent variables. Two dependent variables were considered: (1) unplanned IHD readmissions in 30 days; and (2) unplanned all-cause admissions in 12 months following the index admission. An IHD readmission was defined as a readmission with a discharge diagnosis (either primary or secondary) within the ICD-10 code segment I20–I25 (as specified in the Australian Coding Standards19).
Comparators
A logistic regression model built upon Elixhauser comorbidities was chosen for comparison because the Elixhauser index has been shown to be more discriminatory than other comorbidity indices.20 The Elixhauser index consists of 31 comorbidities that can be mapped from ICD-10 codes.21 Diagnosis codes from the previous year were used to construct the comorbidities. The second baseline is the recently introduced predictive scoring system HOSPITAL,14 which has been shown to perform moderately well in predicting readmissions.
Derivation of the readmission prediction model
Through unrestricted randomisation, the cohort of 1660 patients was divided into a derivation set consisting of two-thirds, whereas the remaining one-third was used for validation. Figure 1 illustrates the process used to derive a prediction model. For each unit of observation, potential risk factors were extracted from the derivation cohort before the index admission. We considered all available administrative hospital data including static information (age, gender, occupation, insurance types) and time-stamped events associated with changes of postcode, emergency visits, hospitalisations, laboratory tests, length of stay, emergency attendance time, transfers after emergency, primary and secondary diagnoses, rare diagnoses, DRG codes, procedures, operations, theatre types and Elixhauser comorbidities. Age was coded as a binary variable in one of 10-year intervals. Occupation was a binary of value 1 if it was either pensioner, retired or home duties and 0 otherwise.
![]() |
Time-stamped events were aggregated over six periods of time before the index admission: 1, 3, 6, 12, 12–24 and 24–36 months. This procedure resulted in a list of 4471 independent variables with specific timing. These include 23 variables on demographics, 344 variables on laboratory test results, 36 variables on past hospitalisations and ED visits, 2460 variables on diagnoses, 582 variables on procedures, 792 variables on DRG codes and 48 variables on theatre use. The dependent variables were the presence or absence of readmissions in periods following the index admission.
The derivation cohort was used to build a logistic regression with lasso22 in the first step of variable screening. Bootstraps were used to estimate probability that a variable was stably selected against data variations.23 Variables with >70% chance of selection were then used to build a second logistic regression model. This model generated prediction of subsequent readmission in the validation cohort.
Let T be the length of prediction (e.g. 6 months). The model to predict the readmission probability in time T following an AMI hospitalisation takes the form below:
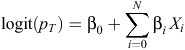
where Xi are N = 4471 independent variables and pT is the probability of readmission in time T following an AMI admission. To fit the model, lasso penalise below was used:

where c is a positive threshold selected through cross-validation.
Note that the logistic model was used to predict the readmission probability, not to estimate the effect size of independent variables. Therefore the size of each βi should not be interpreted as in regression with a relatively few number of independent variables.
Results
Patient characteristics
The study included 1660 patients admitted between January 2009 and December 2011. Patient readmissions during the 12 months following the index admission were measured. Only the first index admission during the study period was considered for analysis. Characteristics of the patients are given in Table 1. In-patient mortality was low in this cohort at 2.6%.
![]() |
Readmission rates are as follows: 105 patients (6.3%) had an unplanned IHD readmission within 30 days. This increased to 149 (9.0%), 191 (11.5%) and 245 (14.8%) at 3, 6 and 12 months respectively. The all-cause admission rates were higher: 212 (12.7%) at 30 days, 316 (19.0%) at 3 months, 419 (25.2%) at 6 months and 518 (31.2%) at 12 months. Although non-cardiac admissions were more common than cardiac admissions at all time points studied, the five most common single causes for readmission were still cardiac diagnoses (chest pain, angina, AMI, heart failure and atrial fibrillation) at 12 months. Other common causes for readmission were other cardiac conditions (rhythm disturbances and valvular disorders; 9.4% of all readmissions), as well as respiratory (9.4%), gastrointestinal (7.6%) and circulatory (arterial and venous, 4.3%) disorders.
Prediction of 30-day IHD readmission
Characteristics of the derivation and validation cohorts are given in Table 2. In all respects, the two cohorts had very similar characteristics. The derivation set of 1107 was used to generate a model to predict 30-day IHD readmissions. The performance of this EMR model was compared against the HOSPITAL score and with a model built on the Elixhauser comorbidities, using data from the testing group. The performance of the three models is given in Table 3. The EMR model performed better than the other models, with greater sensitivity and specificity. The area under the curve (AUC) was 0.78 (95% confidence interval (CI) 0.71–0.85). The major predictive factors (selected automatically) used by this model are listed in Table 4. Previous attendances with chest pain, diabetes, electrolyte abnormalities, use of preventative drugs and a history of cardiac investigations were all predictive of a subsequent IHD readmission.
![]() |
![]() |
![]() |
To illustrate what use of the EMR model may mean in practice, we examined the comparative positive predictive value (PPV) of the three predictive models. The PPV of the three models was examined for the 5%, 10% and 20% of patients identified as being at highest risk of readmission. In each instance, the PPV of the EMR model was higher than that of the other three models (e.g. for the patients estimated to be the highest 5% risk of readmission, the EMR model had a PPV of 0.21 (negative predictive value (NPV) = 0.836) compared with 0.13 for the HOSPITAL score (NPV = 0.789)). Thus, 21% of those judged to be at high-risk of admission were actually readmitted. The overall 30-day IHD readmission rate in the present study was approximately 6.3%. Thus, the EMR model could identify patients who were more than threefold more likely to require readmission than the cohort as a whole. Using the model at different thresholds (e.g. 10% or 20% highest risk of readmission) would lead to loss of PPV but would identify a larger number of patients at risk of readmission.
Prediction of 12-month all-cause readmission
The same derivation and validation procedures were performed for 12-month all-cause readmission. The AUCs for the three predictive methods (EMR, HOSPITAL and Elixhauser) on the testing group are given in Table 5. The EMR model performed better than the other two models, with an AUC of 0.72 (95% CI 0.66–0.78). Cardiac predictors predominate, even as predictors for non-cardiac admissions. Again, electrolyte disturbances and renal disease are major predictive factors. From the PPV of the three predictive models in identifying the 5%, 10% and 20% of patients estimated to be at highest risk of readmission, the EMR model again had greater PPV than the other three models. For example, the EMR model had a PPV of 0.42, meaning that 42% of patients placed in this risk category were readmitted within 1 year.
![]() |
Discussion
We have described a model for predicting readmission after AMI using administrative hospital data from EMRs. The model performed moderately well on the validation dataset and outperformed a model derived from Elixhauser comorbidities and the recently introduced HOSPITAL score.14 This approach has considerable potential to tailor preventative care for AMI patients, to improve institutional performance and to decrease the cost burden associated with AMI.
The considerable burden that unplanned readmissions after AMI places on the healthcare system1–3 could be decreased if we could identify patients at high risk of readmission and institute, or intensify, measures to prevent a further coronary event. Attempts to predict readmission after AMI have relied on biomarkers, the use of known clinical risk factors and comorbidity indices. Although several biomarkers24–28 are known to predict readmission after a coronary event, none is measured routinely in clinical practice at present and we do not know which biomarker or combination will yield the best prediction. There are several known clinical and demographic risk factors for readmission after AMI,5–8,29 but their individual predictive power is relatively low and models derived from such factors have performed poorly.4 Not surprisingly, the presence of comorbidities such as vascular disorders, hypertension, diabetes and other cardiorespiratory conditions increases the risk of readmission after AMI, and combining these into indices results in modest predictive power.30,31 We document here that Elixhauser comorbidities do have predictive power for readmission, but do not perform as well as the EMR model. The readmission rate after AMI in the present study was comparable to that in other recent studies.1,3,32
The use of data in the EMR to predict readmission is attractive for a variety of reasons. Data are routinely collected, forming a comprehensive overview of the patient’s health; data are updated continuously and the derived models can adapt in real time; and the EMR covers comorbidities and biomedical markers known to be predictive. The use of the EMR has considerable potential to improve institutional performance, including decreasing the readmission rate for prevalent conditions such as AMI.11–13 Because EMRs are widely adopted, predictive models that exploit these resources could be seamlessly integrated into clinical pathways, offering an inexpensive tool to assist clinicians in assessing risk. However, the dataset in a comprehensive EMR is complex and unless methods are developed to interpret and present data, the full benefit of the EMR may not be realised. Thus, having an EMR available in an institution does not automatically improve institutional performance.33,34
As demonstrated in the present study, modern statistical techniques such as lasso22 and stability selection23,35 are effective at screening a large number of potential risk factors in EMR, many of which could have been overlooked if a small number of hypotheses were tested.4 Such methods can be used as a fast screening tool to identify risk factors and subjects for further investigation.36
For ischaemic heart disease, algorithms have been developed for surveillance of acute events37,38 and drug safety surveillance39 using the EMR. To date, there has been no comprehensive study of predicting readmissions in AMI patients using EMR data. One recent study,40 using only 11 data items, showed that using routinely collected administrative data has considerable potential. Our EMR model performed better than the recently described HOSPITAL score.14 The latter has not been validated in individual medical conditions and may be of less use in AMI because two of the seven items used in HOSPITAL are invalid in this instance: only 1.6% of discharges were from an oncology service and all admissions were acute. The comprehensive EMR model also outperformed the model based solely on comorbidities. In fact, the top predictive variables also include prior emergency attendances or hospitalisations for both 30-day ischaemic heart disease readmission and 12-month all-cause readmission, and these agree with a recent finding.41
There are several limitations of the present study. First, the study was performed in a single centre and the EMR model has not been independently and externally validated. We did not track readmissions to other hospitals. However, we believe the effect was minimal because the institution under study is the only public hospital in the region (although the data may be biased due to patient migration to urban centres for better-quality care). The present study was based on administrative and clinical data routinely stored in hospital databases. Although such data have the advantage of being readily available, the do not cover the known risk factors for cardiac events, such as metabolic syndrome and waist circumference.
Competing interests
None declared.
References
[1] Dunlay SM, Weston SA, Killian JM, Bell MR, Jaffe AS, Roger VL. Thirty-day rehospitalizations after acute myocardial infarction: a cohort study. Ann Intern Med 2012; 157 11–18.| Thirty-day rehospitalizations after acute myocardial infarction: a cohort study.Crossref | GoogleScholarGoogle Scholar | 22751756PubMed |
[2] Dharmarajan K, Hsieh AF, Lin Z, Bueno H, Ross JS, Horwitz LI, Barreto-Filho JA, Kim N, Bernheim SM, Suter LG, Drye EE, Krumholz HM. Diagnoses and timing of 30-day readmissions after hospitalization for heart failure, acute myocardial infarction, or pneumonia. JAMA 2013; 309 355–63.
| Diagnoses and timing of 30-day readmissions after hospitalization for heart failure, acute myocardial infarction, or pneumonia.Crossref | GoogleScholarGoogle Scholar | 1:CAS:528:DC%2BC3sXhsFWrsrc%3D&md5=89b7f093e004ff2ba8f5d70f497273ceCAS | 23340637PubMed |
[3] Krumholz HM, Lin Z, Keenan PS, Chen J, Ross JS, Drye EE, Bernheim SM, Wang Y, Bradley EH, Han LF, Normand S-LT. Relationship between hospital readmission and mortality rates for patients hospitalized with acute myocardial infarction, heart failure, or pneumonia. JAMA 2013; 309 587–93.
| Relationship between hospital readmission and mortality rates for patients hospitalized with acute myocardial infarction, heart failure, or pneumonia.Crossref | GoogleScholarGoogle Scholar | 1:CAS:528:DC%2BC3sXivVSmsLY%3D&md5=fd691415cdc378cf93a61a47df01685cCAS | 23403683PubMed |
[4] Desai MM, Stauffer BD, Feringa HH, Schreiner GC. Statistical models and patient predictors of readmission for acute myocardial infarction: a systematic review. Circ Cardiovasc Qual Outcomes 2009; 2 500–7.
| Statistical models and patient predictors of readmission for acute myocardial infarction: a systematic review.Crossref | GoogleScholarGoogle Scholar | 20031883PubMed |
[5] Kociol RD, Lopes RD, Clare R, Thomas L, Mehta RH, Kaul P, Pieper KS, Hochman JS, Weaver WD, Armstrong PW, Granger CB, Patel MR. International variation in and factors associated with hospital readmission after myocardial infarction. JAMA 2012; 307 66–74.
| International variation in and factors associated with hospital readmission after myocardial infarction.Crossref | GoogleScholarGoogle Scholar | 1:CAS:528:DC%2BC38XpsVSmtA%3D%3D&md5=53160bd2f212863754a236558155b52dCAS | 22215167PubMed |
[6] Bucholz EM, Rathore SS, Gosch K, Schoenfeld A, Jones PG, Buchanan DM, Spertus JA, Krumholz HM. Effect of living alone on patient outcomes after hospitalization for acute myocardial infarction. Am J Cardiol 2011; 108 943–8.
| Effect of living alone on patient outcomes after hospitalization for acute myocardial infarction.Crossref | GoogleScholarGoogle Scholar | 21798499PubMed |
[7] Joynt KE, Orav EJ, Jha AK. Thirty-day readmission rates for Medicare beneficiaries by race and site of care. JAMA 2011; 305 675–81.
| Thirty-day readmission rates for Medicare beneficiaries by race and site of care.Crossref | GoogleScholarGoogle Scholar | 1:CAS:528:DC%2BC3MXit1amt7c%3D&md5=61d20c9b4d86a8ac01881bda4194f515CAS | 21325183PubMed |
[8] Andrés E, García-Campayo J, Magán P, Barredo E, Cordero A, León M, Botaya RM, García-Ortiz L, Gómez M, Alegría E, Casasnovas JA. Psychiatric morbidity as a risk factor for hospital readmission for acute myocardial infarction: an 8-year follow-up study in Spain. Int J Psychiatry Med 2012; 44 63–75.
| Psychiatric morbidity as a risk factor for hospital readmission for acute myocardial infarction: an 8-year follow-up study in Spain.Crossref | GoogleScholarGoogle Scholar | 23356094PubMed |
[9] Reese RL, Freedland KE, Steinmeyer BC, Rich MW, Rackley JW, Carney RM. Depression and rehospitalization following acute myocardial infarction. Circ Cardiovasc Qual Outcomes 2011; 4 626–33.
| Depression and rehospitalization following acute myocardial infarction.Crossref | GoogleScholarGoogle Scholar | 22010201PubMed |
[10] Lindenauer PK, Lagu T, Rothberg MB, Avrunin J, Pekow PS, Wang Y, Krumholz HM. Income inequality and 30 day outcomes after acute myocardial infarction, heart failure, and pneumonia: retrospective cohort study. BMJ 2013; 346 f521
| Income inequality and 30 day outcomes after acute myocardial infarction, heart failure, and pneumonia: retrospective cohort study.Crossref | GoogleScholarGoogle Scholar | 23412830PubMed |
[11] Murff HJ, FitzHenry F, Matheny ME, Gentry N, Kotter KL, Crimin K, Dittus RS, Rosen AK, Elkin PL, Brown SH, Speroff T. Automated identification of postoperative complications within an electronic medical record using natural language processing. JAMA 2011; 306 848–55.
| Automated identification of postoperative complications within an electronic medical record using natural language processing.Crossref | GoogleScholarGoogle Scholar | 1:CAS:528:DC%2BC3MXhtFSnu73M&md5=0f8e0cce67ef6669b917a20fcb043a08CAS | 21862746PubMed |
[12] Appari A, Eric Johnson M, Anthony DL. Meaningful use of electronic health record systems and process quality of care: evidence from a panel data analysis of U.S. acute-care hospitals. Health Serv Res 2013; 48 354–75.
| Meaningful use of electronic health record systems and process quality of care: evidence from a panel data analysis of U.S. acute-care hospitals.Crossref | GoogleScholarGoogle Scholar | 22816527PubMed |
[13] FitzHenry F, Murff HJ, Matheny ME, Gentry N, Fielstein EM, Brown SH, Reeves RM, Aronsky D, Elkin PL, Messina VP, Speroff T. Exploring the frontier of electronic health record surveillance: the case of postoperative complications. Med Care 2013; 51 509–16.
| Exploring the frontier of electronic health record surveillance: the case of postoperative complications.Crossref | GoogleScholarGoogle Scholar | 23673394PubMed |
[14] Donze J, Aujesky D, Williams D, Schnipper JL. Potentially avoidable 30-day hospital readmissions in medical patients: derivation and validation of a prediction model. JAMA Intern Med 2013; 173 632–8.
| 23529115PubMed |
[15] World Health Organization. International statistical classification of diseases and related health problems 10th revision (ICD-10). 2010. Available at http://apps.who.int/classifications/icd10/browse/2010/en [verified 19 May 2014].
[16] National Casemix and Classification Centre (NCCC). AR-DRG definitions manual V7.0. Wollongong: NCCC; 2013.
[17] Herrett E, Shah AD, Boggon R, Denaxas S, Smeeth L, van Staa T, Timmis A, Hemingway H. Completeness and diagnostic validity of recording acute myocardial infarction events in primary care, hospital care, disease registry, and national mortality records: cohort study. BMJ 2013; 346 f2350
| Completeness and diagnostic validity of recording acute myocardial infarction events in primary care, hospital care, disease registry, and national mortality records: cohort study.Crossref | GoogleScholarGoogle Scholar | 23692896PubMed |
[18] Thygesen K, Alpert JS, Jaffe AS, Simoons ML, Chaitman BR, White HD, the Writing Group on behalf of the Joint ESC/ACCF/AHA/WHF Task Force for the Universal Definition of Myocardial Infarction Third universal definition of myocardial infarction. Circulation 2012; 126 2020–35.
| Third universal definition of myocardial infarction.Crossref | GoogleScholarGoogle Scholar | 22923432PubMed |
[19] National Casemix and Classification Centre (NCCC). ACS 0940 ischaemic heart disease. In: Australian coding standards, 8th edn. Wollongong: NCCC; 2013. pp. 122–6.
[20] Elixhauser A, Steiner C, Harris DR, Coffey RM. Comorbidity measures for use with administrative data. Med Care 1998; 36 8–27.
| Comorbidity measures for use with administrative data.Crossref | GoogleScholarGoogle Scholar | 1:STN:280:DyaK1c%2FptlemtA%3D%3D&md5=86e99662caf3d2ee84f6b1ca2dfc4538CAS | 9431328PubMed |
[21] Quan H, Sundararajan V, Halfon P, Fong A, Burnand B, Luthi JC, Saunders LD, Beck CA, Feasby TE, Ghali WA. Coding algorithms for defining comorbidities in ICD-9-CM and ICD-10 administrative data. Med Care 2005; 43 1130–9.
| Coding algorithms for defining comorbidities in ICD-9-CM and ICD-10 administrative data.Crossref | GoogleScholarGoogle Scholar | 16224307PubMed |
[22] Tibshirani R. Regression shrinkage and selection via the Lasso. J R Stat Soc B Met 1996; 58 267–88.
[23] Meinshausen N, Buhlmann P. Stability selection. J R Stat Soc B 2010; 72 417–73.
| Stability selection.Crossref | GoogleScholarGoogle Scholar |
[24] Hartford M, Wiklund O, Mattsson Hultén L, Persson A, Karlsson T, Herlitz J, Caidahl K. C-Reactive protein, interleukin-6, secretory phospholipase A2 group IIA and intercellular adhesion molecule-1 in the prediction of late outcome events after acute coronary syndromes. J Intern Med 2007; 262 526–36.
| C-Reactive protein, interleukin-6, secretory phospholipase A2 group IIA and intercellular adhesion molecule-1 in the prediction of late outcome events after acute coronary syndromes.Crossref | GoogleScholarGoogle Scholar | 1:CAS:528:DC%2BD2sXhtlOhtLrJ&md5=bd030dbc4f16c84bf102c76422f9bfb2CAS | 17908161PubMed |
[25] Gao Y, Tong GX, Zhang XW, Leng JH, Jin JF, Wang NF, Yang JM. Interleukin-18 levels on admission are associated with mid-term adverse clinical events in patients with ST-segment elevation acute myocardial infarction undergoing percutaneous coronary intervention. Int Heart J 2010; 51 75–81.
| Interleukin-18 levels on admission are associated with mid-term adverse clinical events in patients with ST-segment elevation acute myocardial infarction undergoing percutaneous coronary intervention.Crossref | GoogleScholarGoogle Scholar | 1:CAS:528:DC%2BC3cXls1SktLo%3D&md5=f36089da447ac88181cb39e41dded368CAS | 20379038PubMed |
[26] Xin H, Chen ZY, Lv XB, Liu S, Lian ZX, Cai SL. Serum secretory phospholipase A2-IIa (sPLA2-IIA) levels in patients surviving acute myocardial infarction. Eur Rev Med Pharmacol Sci 2013; 17 999–1004.
| 1:STN:280:DC%2BC3snisFOltA%3D%3D&md5=0e19ba6df82f2b98eeaf21eec1e65252CAS | 23661511PubMed |
[27] Ephrem G. Red blood cell distribution width is a predictor of readmission in cardiac patients. Clin Cardiol 2013; 36 293–9.
| Red blood cell distribution width is a predictor of readmission in cardiac patients.Crossref | GoogleScholarGoogle Scholar | 23553899PubMed |
[28] Matsudaira K, Maeda K, Okumura N, Yoshikawa D, Morita Y, Mitsuhashi H, Ishii H, Kondo T, Murohara T, Nagoya Acute Myocardial Infarction Study (NAMIS) Group Impact of low levels of vascular endothelial growth factor after myocardial infarction on 6-month clinical outcome. Results from the Nagoya Acute Myocardial Infarction Study. Circ J 2012; 76 1509–16.
| Impact of low levels of vascular endothelial growth factor after myocardial infarction on 6-month clinical outcome. Results from the Nagoya Acute Myocardial Infarction Study.Crossref | GoogleScholarGoogle Scholar | 1:CAS:528:DC%2BC38Xpsl2ku7c%3D&md5=0a59a9c8aeb1470a40a9d292bcd70561CAS | 22452999PubMed |
[29] Rodriguez F, Joynt KE, Lopez L, Saldana F, Jha AK. Readmission rates for Hispanic Medicare beneficiaries with heart failure and acute myocardial infarction. Am Heart J 2011; 162 254–261.e253.
| Readmission rates for Hispanic Medicare beneficiaries with heart failure and acute myocardial infarction.Crossref | GoogleScholarGoogle Scholar | 21835285PubMed |
[30] Condon JR, You J, McDonnell J. Performance of comorbidity indices in measuring outcomes after acute myocardial infarction in Australian Indigenous and non-Indigenous patients. Intern Med J 2012; 42 e165–73.
| Performance of comorbidity indices in measuring outcomes after acute myocardial infarction in Australian Indigenous and non-Indigenous patients.Crossref | GoogleScholarGoogle Scholar | 1:STN:280:DC%2BC38zktlyrsw%3D%3D&md5=5eeaadaa047f519ea0e27b0ec785a79bCAS | 21627745PubMed |
[31] Gandjour A, Ku-Goto MH, Ho V. Comparing the validity of different measures of illness severity: a hospital-level analysis for acute myocardial infarction. Health Serv Manag Res 2012; 25 138–43.
| Comparing the validity of different measures of illness severity: a hospital-level analysis for acute myocardial infarction.Crossref | GoogleScholarGoogle Scholar |
[32] Kaboli PJ, Go JT, Hockenberry J, Glasgow JM, Johnson SR, Rosenthal GE, Jones MP, Vaughan-Sarrazin M. Associations between reduced hospital length of stay and 30-day readmission rate and mortality: 14-year experience in 129 Veterans Affairs hospitals. Ann Intern Med 2012; 157 837–45.
| Associations between reduced hospital length of stay and 30-day readmission rate and mortality: 14-year experience in 129 Veterans Affairs hospitals.Crossref | GoogleScholarGoogle Scholar | 23247937PubMed |
[33] Kazley AS, Ozcan YA. Do hospitals with electronic medical records (EMRs) provide higher quality care? An examination of three clinical conditions. Med Care Res Rev 2008; 65 496–513.
| Do hospitals with electronic medical records (EMRs) provide higher quality care? An examination of three clinical conditions.Crossref | GoogleScholarGoogle Scholar | 18276963PubMed |
[34] Jones SS, Adams JL, Schneider EC, Ringel JS, McGlynn EA. Electronic health record adoption and quality improvement in US hospitals. Am J Manag Care 2010; 16 SP64–71.
| 21314225PubMed |
[35] Austin PC, Tu JV. Bootstrap methods for developing predictive models. Am Stat 2004; 58 131–7.
| Bootstrap methods for developing predictive models.Crossref | GoogleScholarGoogle Scholar |
[36] He D, Mathews SC, Kalloo AN, Hutfless S. Mining high-dimensional administrative claims data to predict early hospital readmissions. JAMIA 2013; 21 272–9.
| 24076748PubMed |
[37] Krumholz HM, Lin Z, Drye EE, Desai MM, Han LF, Rapp MT, Mattera JA, Normand SL. An administrative claims measure suitable for profiling hospital performance based on 30-day all-cause readmission rates among patients with acute myocardial infarction. Circ Cardiovasc Qual Outcomes 2011; 4 243–52.
| An administrative claims measure suitable for profiling hospital performance based on 30-day all-cause readmission rates among patients with acute myocardial infarction.Crossref | GoogleScholarGoogle Scholar | 21406673PubMed |
[38] Kottke TE, Baechler CJ. An algorithm that identifies coronary and heart failure events in the electronic health record. Prev Chronic Dis 2013; 10 120 097
| An algorithm that identifies coronary and heart failure events in the electronic health record.Crossref | GoogleScholarGoogle Scholar |
[39] Coloma PM, Avillach P, Salvo F, Schuemie MJ, Ferrajolo C, Pariente A, Fourrier-Réglat A, Molokhia M, Patadia V, van der Lei J, Sturkenboom M, Trifirò G. A reference standard for evaluation of methods for drug safety signal detection using electronic healthcare record databases. Drug Saf 2013; 36 13–23.
| A reference standard for evaluation of methods for drug safety signal detection using electronic healthcare record databases.Crossref | GoogleScholarGoogle Scholar | 1:CAS:528:DC%2BC3sXhsl2ns7bI&md5=1ed01f0b8f19e148ab31571901437ef8CAS | 23315292PubMed |
[40] Wallmann R, Llorca J, Gomez-Acebo I, Ortega AC, Roldan FR, Dierssen-Sotos T. Prediction of 30-day cardiac-related-emergency-readmissions using simple administrative hospital data. Int J Cardiol 2013; 164 193–200.
| Prediction of 30-day cardiac-related-emergency-readmissions using simple administrative hospital data.Crossref | GoogleScholarGoogle Scholar | 21775001PubMed |
[41] Brown JR, Conley SM, Niles NW. Predicting readmission or death after acute ST-elevation myocardial infarction. Clin Cardiol 2013; 36 570–5.
| 23754777PubMed |