Carbon-dioxide-driven increase in foliage projective cover is not the same as increased woody plant density: lessons from an Australian tropical savanna
Gabriel M. Crowley

A Artemis Nature Fund Inc., Malanda, Qld 4885, Australia.
B Research and Recovery of Endangered Species Group, School of Environmental and Earth Sciences, University of Queensland, Saint Lucia, Qld 4072, Australia.
The Rangeland Journal 45(2) 81-95 https://doi.org/10.1071/RJ23001
Submitted: 3 January 2023 Accepted: 22 May 2023 Published: 15 June 2023
© 2023 The Author(s) (or their employer(s)). Published by CSIRO Publishing on behalf of the Australian Rangeland Society. This is an open access article distributed under the Creative Commons Attribution-NonCommercial-NoDerivatives 4.0 International License (CC BY-NC-ND)
Abstract
Carbon accounting in tropical savannas relies on a good understanding of the effects of atmospheric carbon dioxide (CO2) and land management on foliage projective cover (FPC) and vegetation structure. We used generalised additive modelling to track changes in Autumn Persistent Green (APG, a satellite-image-derived measure of FPC) in six vegetation types on Cape York Peninsula, Australia, over an 18-year period, and examined the influence of fire and grazing land tenure. We then used field monitoring and variography (analysis of spatial autocorrelation) in a smaller study area to determine whether changes in APG reflected vegetation structural change. APG increased through the 18-year study period and was significantly influenced by vegetation type, recent fire history and grazing land tenure. Residual year-on-year increases suggest CO2 fertilisation was the main driver of APG increase. APG was reduced by fires in the previous year, with early dry season fires having greater impact than late dry season fires, particularly in grassland and rainforest. This is consistent with leaves being most fire sensitive early in the year, when they are actively growing, than in the late dry season, when they are dormant. As seedlings and suckers would be particularly fire-sensitive, early fires may therefore be more effective than late fires at preventing woody encroachment. We demonstrated that variography provides a good indication of whether APG increases are caused by increases in FPC alone, or by an increase in tree density. We found support for increased woody plant density in grasslands, and that this increase was most pronounced on grazing lands. Conversely, we found no support for stem density increases in the dominant eucalypt woodland, despite APG increases being highest in this vegetation type. Hence, increases in FPC cannot always be equated to increases in woody biomass, and may occur in their absence. This conclusion has serious implications for global carbon accounting.
Keywords: biophysical models, climate change and high CO2, fire vegetation patterns, grasslands, grazing pressure, remote sensing, savanna woodlands, vegetation structure.
Introduction
Potential effects of increasing atmospheric CO2 on vegetation include increases in foliage projective cover (FPC) and the size and density of woody plants (Hovenden and Williams 2010; Bond and Midgley 2012; Donohue et al. 2013). If FPC can be shown to influence woody plant size and number, then it can also be used as a measure of woody biomass. Although increases in both measures of foliage cover (Piao et al. 2020) and woody plant density (Stevens et al. 2017) have been observed in many landscapes over the last century, the nexus between the two is largely untested.
Australia is a key region for testing this relationship. Studies have shown an 11.7% increase in the normalised difference vegetation index (NDVI) across eastern Australia since 1982 (Rifai et al. 2022). This has been interpreted as indicating an increasing FPC as a result of CO2 fertilisation. Australian tropical savannas have also experienced structural vegetation change over the same period, with woody encroachment of grasslands, and the expansion of rainforests (Bowman and Fensham 1991; Neldner et al. 1997; Crowley and Garnett 1998; Russell-Smith et al. 2004a; Sharp and Bowman 2004). CO2 fertilisation is also likely to have had a role in these changes (Bond and Midgley 2012). In this study, we investigated changes in FPC and vegetation structure on Cape York Peninsula (CYP) in the Australian tropical savanna zone.
FPC may increase as a result of increases in the density of leaves within an existing canopy area; an expansion of the canopy of existing trees, or an increase in tree density (Fig. 1; Fisher et al. 2018). At least the first of these may occur without an increase in woody biomass. Rain-induced leaf flush is often transient, reversing with the onset of drought, and carbon assimilated by leaves may be lost to the atmosphere rather than converted to timber (Thomas and Martin 2012; Rahman et al. 2019).
(a, b) Components of vegetation that contribute to foliage projective cover; and how these are translated into foliage response to (c–e) increasing and (f–h) decreasing rainfall. Artwork © Mariska Marnane.
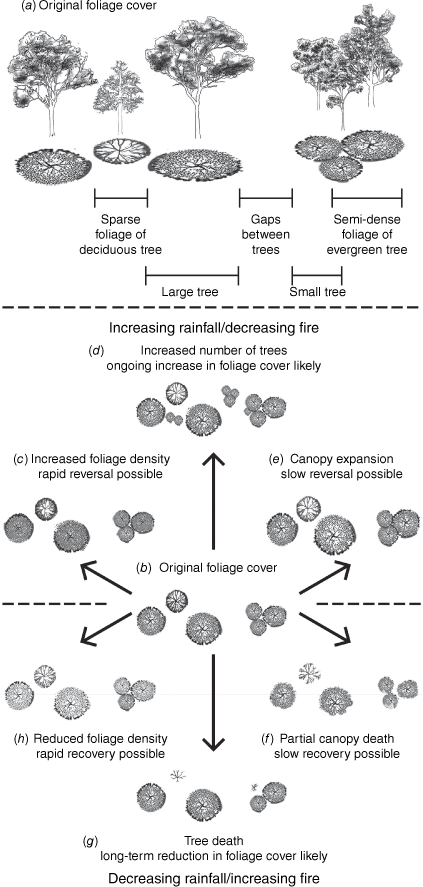
FPC increases and structural vegetation change may also have different drivers. Increase in woody stem density in savannas has been linked to fire and grazing regimes, rainfall variation and CO2 fertilisation (Bond and Midgley 2000; van Langevelde et al. 2003; Sharp and Bowman 2004; Prior et al. 2006; Crowley et al. 2009; Bond and Midgley 2012; Anderegg et al. 2013; Cook et al. 2015; Stevens et al. 2016). While FPC is likely to respond to the same drivers, it may not do so in the same way. Knowing the extent to which changes in FPC and woody stem density are linked is essential for global carbon accounting. Accurate assessment of carbon stocks also requires a landscape-scale understanding of how CO2 fertilisation interacts with fire and grazing regimes to affect vegetation structure (Bultan et al. 2022).
Linking vegetation change to causal factors is often challenging because of the limited timeframe of field studies or intermittent availability of aerial photographs, whereas global and continental assessments may be at too coarse a scale to identify vegetation structure or its relationship with land management (cf. Haverd et al. 2020). Recently, medium-scale (30 m resolution) vegetation products derived from Landsat imagery, combined with on-ground verification, has facilitated assessment of Australian vegetation structure at landscape, regional and continental scales (Flood et al. 2019; Liao et al. 2020). Of these products, Persistent Green (a satellite-image derived measure of FPC, see Supplementary Appendix S1 in the Supporting Information), has also been used to assess vegetation change (Scarth et al. 2015). The utility of this product may be enhanced by variography, the statistical description of the texture (spatial autocorrelation) of imagery (Solana-Gutiérrez and Merino-de-Miguel 2011), tied to on-ground observations of vegetation structure. In this study, we used Persistent Green to assess FPC change and the extent to which this has been translated into changes in vegetation structure. We also examined the role of CO2 fertilisation and fire and grazing land tenure in driving FPC change. Finally, we propose a method for further exploring vegetation change and its causes using variography tied to on-ground observations of vegetation structure.
Methods
Study areas
Our main study area extends over 274 000 km2 of CYP (Fig. 2; 140–145°E, 12–19°S), and constitutes 21% of Australia’s tropical savanna zone. It is within the area identified as having a spike in carbon sequestration in 2010–2011 (Ma et al. 2016). It has reliable monsoonal rainfall, with minimal interannual variation (Laughlin et al. 2003; Bureau of Meteorology 2019) and little vegetation clearance (Accad et al. 2021), so provides a key opportunity for assessing whether increasing CO2 levels have been translated into vegetation structural change in tropical savannas. Vegetation change documented in the study area from plot-based sampling and analysis of aerial photographs includes loss of grasslands; thickening of teatree woodlands; and expansion of rainforest into adjoining eucalypt woodlands (Neldner et al. 1997; Crowley and Garnett 1998; Russell-Smith et al. 2004a; Crowley et al. 2009).
The study area showing focal vegetation types. Map adapted from Broad Vegetation Group mapping by Department of Environment and Science (2018).
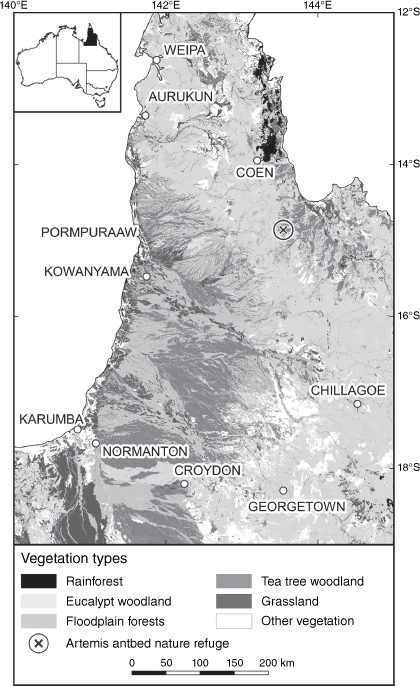
This study area is primarily in the tropical savanna climatic zone (Bureau of Meteorology 2006; Supplementary Appendix S2, Fig. S3). Between 1961 and 1990, mean temperatures averaged 24–30°C, minima 18–21°C, and maxima 24–40°C, with little change over the subsequent decades (Bureau of Meteorology 2022). Rainfall is concentrated between November and April (Fig. S2), and averaged 580–2400 mm a year between 1986 and 2015, with moderately low annual variability (Fig. S3; Bureau of Meteorology 2022). Rainfall in the 30 years to 2018 averaged 3% higher than in the previous 30 years (Bureau of Meteorology 2019). Spatially, interannual rainfall variability increased as rainfall decreased (Fig. S3). Soils are typically infertile, especially deficient in phosphorus and sulfur (Biggs and Philip 1995). Fifteen vegetation types (1:5 million scale Broad Vegetation Groups, BVGs; Queensland Herbarium 2018) have been mapped in the study area (Table S1, Fig S4). Eucalypt woodland is the most extensive (47.7%), followed by teatree woodland (18.8%) and floodplain forest (12.4%). Grassland covers 7.7% of the study area. First Nations Peoples have occupied CYP for at least 37 000 years (David and Lourandos 1997). Most land was removed from First Australians’ ownership for cattle grazing in the late 19th century, and continued to be grazed throughout the study period (Table S2, Fig. S5). Restoration of land to First Nations Peoples commenced in 1987, along with large areas being retired from grazing.
During the study period, highest fire frequency occurred along the western peninsula (excluding the low-lying alluvial fans), extending to the east coast in the central peninsula (Figs S6, S7). Large areas were burnt in at least 14 of the 18 years. Dedicated fire extension programs began in 2004, and the Australian Government’s Savanna Burning program in 2012 (Edwards et al. 2021). This program awards carbon credits for the use of low intensity early dry season (EDS: January–July) burning to reduce the extent of more intense late dry season (LDS: August–December) wildfires, thereby abating greenhouse gas emissions and sequestering carbon (Murphy et al. 2015).
We used Artemis Antbed Nature Refuge (AANR) – originally a 21 km2 area being managed for golden-shouldered parrot (Psephotus chrysopterygius Gould, 1857) recovery in central CYP – as a case study area for assessing vegetation structural change (Murphy et al. 2021). AANR is on Artemis cattle station, and has been used for grazing since 1911 (Crowley and Garnett 2000). It was fenced and dedicated as a Nature Refuge in 1994, although grazing continued until 2019. In 2022, AANR was expanded to >1000 km2. It is dominated by eucalypt woodland (56.4%) and teatree woodland (26.3%), with pockets of grassland (9.1%; Fig. S8). AANR and the surrounding area experienced teatree (Melaleuca viridiflora Sol. ex Gaertn) invasion of grasslands and thickening of teatree woodlands (Crowley and Garnett 1998; Crowley et al. 2009). Between 2001 and 2020, the 30 m pixels within AANR were burnt an average of 2.6 times (2.3 times by LDS fires), with no fires in either 2001 or 2020.
Data layers and study period
We used Autumn Persistent Green (APG; Department of Environment and Science 2022; 30 m pixels; coordinate reference system – EPSG: 3577, https://epsg.io/) as a measure of FPC, because autumn (March–May) imagery was the least affected by current-year fires (Supplementary Appendix S1). We used fire scar mapping from the North Australian Fire Information website. This was derived from satellite imagery from MODIS (the Moderate Resolution Imaging Spectroradiometer), VIIRS (the Visible Infrared Imaging Radiometer Suite) and NOAA (the National Oceanic and Atmospheric Administration) (Charles Darwin University 2021; 250 m pixels; EPSG: 4283). Annual EDS fire rasters were produced by combining all fires from January to July, inclusive, and LDS fire rasters by combining all fires from August to December, inclusive. We chose a study period (2001–2018) over which both Persistent Green imagery (1990–2018) and fire scar mapping (2000–2020) were available at the time of analysis, delaying the start year to 2001 to enable inclusion of previous-year fire occurrence in our modelling. We compiled a raster of grazing land tenure during the study period (Table S2, Fig. S5; 1:100 000; EPSG: 3577) for inclusion in all models.
We also sourced data layers of other variables with the potential to influence fire occurrence and vegetation structure (Supplementary Appendix S3, Table S3), i.e. vegetation type (Queensland Herbarium 2018; 1:100 000 resolution; EPSG:4283), rainfall (Bureau of Meteorology 2008; pixel size: 0.025°; EPSG:4283), and 1-, 2- or 3-year percentage rainfall anomalies (Bureau of Meteorology 2021; 0.05° pixels; EPSG:3577), as justified below. We did not include temperature, because vegetation change in savanna regions has been shown to be relatively insensitive to temperature (García Criado et al. 2020).
Vegetation type influences fire regime through variations in its structure and composition (Miller and Murphy 2017). Different vegetation types have also exhibited different forms of structural change that are – in turn – influenced by fire regime. Rainforest on CYP (BVG 1) has expanded into adjoining woodlands and grasslands (Bowman and Fensham 1991; Russell-Smith et al. 2004a, 2004b). The structure of Eucalypt-dominated woodland (BVG3) and floodplain forest (BVG4) appears to have remained fairly stable across northern Australia (Crowley and Garnett 1998; Murphy et al. 2014; Prior et al. 2020). Teatree woodland on CYP (BVG 8) has markedly thickened under a fire regime that has been influenced by cattle grazing (BVG 8, Crowley et al. 2009), and encroached on adjoining grasslands (BVG13; Neldner et al. 1997; Crowley and Garnett 1998). We therefore retained each of these vegetation types, and combined all remaining vegetation types into an unclassified vegetation type.
Seasonal and annual variations in rainfall influence fire occurrence (Felderhof and Gillieson 2006). They also drive leaf production and loss, and thus change in FPC (Fensham et al. 2009; Thomas and Martin 2012; Anderegg et al. 2013; Rahman et al. 2019). Extended wet and dry periods drive cyclical canopy thickening and thinning (Fensham and Holman 1999); with 3-year rainfall anomalies being shown to influence Persistent Green values in northern Australian savannas (Cowley 2020).
Modelling
Data management and modelling were undertaken in R (R Core Team 2020). We combined all data layers for 18 years of APG, 19 years of EDS and LDS fire extent, grazing land tenure, vegetation, rainfall, and 18 years of 1-, 2- and 3-year rainfall anomalies into a raster stack matching the extent of the main study area using the raster package (Hijmans and van Etten 2012). All layers were first either rasterised or disaggregated to a 30 m resolution, with no interpolation. Values from this stack were extracted at 30 525 stratified random points across the study area (1500–6000 per vegetation type) for use in analysis, reduced to 27 622 points (959–5742 per vegetation type; Fig. S9, Table S4) on the removal of missing values and locations whose grazing land tenure changed within the study period.
We used generalised additive modelling (GAM) for very large datasets (bam function in the mgcv package; Wood 2019): first, to characterise changes in fire occurrence in each vegetation type over time; and then, to assess the influence of fire and grazing land tenure on FPC in each vegetation type over time. To model EDS and LDS fire occurrence in each vegetation type over time, we used GAM with a binomial distribution and the logit link function using factor smooth and 18 knots (the number of joins between spline functions) corresponding to the 18 years of the study period as a fixed effect. Each model of fire occurrence also initially contained the following random effects: previous-year fire season (EDS, LDS, or unburnt), grazing land tenure (yes, no), mean annual rainfall, 1-, 2- or 3-yearly rainfall anomalies, geospatial location, and – for LDS fire occurrence alone – EDS burning in the current year. To clarify general trends in fire occurrence, we reran the best-fit model using factor smooth with three knots to produce a model with maximum smoothness.
To model changes in APG in each vegetation type over time, we used GAM with a Gaussian distribution and the identity link function (with factor smooth and 18 knots) as a fixed effect, initially with no other effects included in the model. To assess the influence of management on APG, we reran the model including year–vegetation type, previous-year season of burn, grazing land tenure and vegetation–grazing interactions as fixed effects; as well as average annual rainfall, 1-, 2- or 3-yearly rainfall anomalies, and geospatial location as random effects.
Inclusion of geospatial location in all models accounted for spatiotemporal variability, but we did not incorporate autocorrelation as we were not making predictions outside our sampled time periods or study area (Pawley and McArdle 2021). We adjusted each model by substituting or removing variables to identify the best-fit model that explained the highest level of deviance with the least effective degrees of freedom as determined by a deviance test ANOVA. Independent fixed and random effects were retained in the model if their contribution was significant at P < 0.05, or if the analysis of deviance test indicated that they strengthened the model.
Field observations and variography
We used variography tied to on-ground observations to identify modes of canopy change in AANR (Fig. 1). We extracted the woody plant data from seven 200 m2 permanently-marked field plots in and around AANR (Fig. S6) established in 1998–2001 in grasslands being invaded by teatree woodland, and remeasured in 2021 (Crowley and Garnett 1998; Crowley et al. 2009; Murphy et al. 2021). We grouped counts of woody plants into two size classes (1–3, and >3 m, which is the height above which lignotuberous plants can generally survive burning without height reduction; Bond and Midgley 2000). We compared the results from these plots with changes in the APG imagery between 2001 and 2020.
We compared APG variograms for AANR in 2001 and 2020 to assess whether on-ground vegetation structural change in the grassland/teatree woodland vegetation community could be detected using variography, as follows. Uniform grassland or woodland has low variation in FPC. Adding trees to grassland increases the variation; filling in canopy gaps in woodland reduces it. Variography captures these changes by measuring spatial autocorrelation, or how similar FPC at any one point is to that of the surrounding vegetation (Solana-Gutiérrez and Merino-de-Miguel 2011; Hamada et al. 2019). Variograms map semivariance (variance of observations that are lower than the mean) against distance (Supplementary Appendix S4). If spatial autocorrelation is present, influence declines with distance, and semivariance increases towards a maximum value (the sill; Fig. S10). The distance at which this occurs is called the range. Within-pixel semivariance is captured in the nugget (semivariance at zero distance). Within a vegetation type, semivariance usually follows a Gaussian, spherical or exponential form; and adjoining vegetation formations produce secondary and subsequent peaks (Kamal et al. 2014). Variation in cover within the canopy and between canopies is reflected in a variogram’s nugget and partial sill (sill minus nugget), respectively; the range reflects size of vegetation structural elements, and the form reflects the level of clustering within those elements (Hamada et al. 2019). Hence variogram parameters can be used to separate out structural elements of vegetation cover. Increase in foliage density within the canopy and infilling of canopy gaps smaller than the pixel size should reduce the nugget-to-partial sill ratio (Table S5). Woody invasion of grassland should increase the sill. We also applied variography to the eucalypt woodland in AANR using the same principles, but without the benefit of field observations.
We extracted APG for grassland/teatree woodland and eucalypt woodland from the 2001 and 2020 APG layers for use in separate variograms. As severe fragmentation interferes with variogram calculation, we selected a contiguous subset of 12 510 eucalypt woodland pixels for analysis, but were able to use all 8274 grassland/teatree woodland pixels. We constructed and analysed APG variograms for each year-vegetation combination using the R package gstat (Pebesma 2004). After discounting anisotropy (directional dependence), we constructed a single two-dimensional exponential variogram for each year-vegetation combination. We used a Kolmogorov–Smirnov test to assess whether there was a significant change in the shape of the variograms between years, and extracted the variogram parameters of nugget, sill, partial sill, and range for further comparison. We also used two-sample sign tests to compare APG values of grassland, teatree woodland and eucalypt woodland in AANR in 2001 and 2020.
Data limitations
The following data limitations were considered in our interpretation. Differences in raster resolution may have obscured any relationships between APG (30 m pixels), fire scars (250 m), rainfall (0.025°) and rainfall anomalies (0.05°), thus creating Type II, rather than Type I errors. Further blurring of relationships may have arisen from the smoothing of APG through the full time series to reduce the influence of seasonal changes in FPC (Department of Environment and Science 2022). Therefore, any immediate impacts of fire and rainfall on APG were likely to be underestimated.
Although, of the Persistent Green products, APG (March–May) would be least affected by current-year fires, high cloud cover at the start of Autumn would tend to push the selection of images towards the end of the season, at a time when fires are beginning to occur (Fig. S1). This could obscure the relationship between APG and previous-year fires. Conversely, images derived from the start of the season would have been extracted before the wet season rains stopped (Fig. S2). This could obscure the relationship between FPC and 1-year rainfall anomalies, and to a lesser extent, 2- and 3-year rainfall anomalies.
Cloud cover also possibly obscured storm-burns, the fires that are considered most effective at reducing woody encroachment (Crowley et al. 2009). Storm-burning is undertaken within a couple of days of the first heavy rains, usually in December or January. Wet soils usually mean that grass cover recovers rapidly after a storm-burn, recolonising fire scars by the time the sky clears enough to show in imagery. Storm-burns are therefore likely to have been under-mapped, and their temporal variability means that mapping of both EDS and LDS fires could have included storm-burns in some years. In summary, these data limitations are likely to have reduced the strength of real relationships, rather than fabricated relationships that do not exist.
Results
Fire
Between 2001 and 2018, an average of 28.0% of our study area was burnt each year; 5.1% by EDS fires and 22.9% by LDS fires (Fig. 3). The best-fit model explaining EDS fire occurrence included a smooth function of vegetation and year with 18 knots as a fixed effect, and previous-year fire season, grazing land tenure, 1-year rainfall anomalies and location as random effects (Supplementary Appendix S5). The best-fit model explaining LDS fire included a smooth function of vegetation and year with 18 knots as a fixed effect, and previous-year fire season, grazing land tenure, 3-year rainfall anomalies, EDS fire occurrence in the current year and location as random effects. These models explained a low proportion of the deviance of EDS and LDS fire probability (16.6 and 16.2%, respectively). The maximum-smooth models (three knots) containing the same significant fixed and random effects explained only 14.9 and 13.8% of the deviance, respectively. In both seasons, the probability of burning differed significantly between vegetation types, being lowest in rainforest and highest in teatree woodland. EDS fire occurrence varied little through the study period, with lowest extents in about 2008. LDS fire occurrence decreased until about 2010, and continued to do so in rainforest; but levelled out in eucalypt woodland, grassland and unclassified vegetation; and increased in teatree woodland and floodplain forest.
Generalised additive models for changes in fire occurrence in the (a) early and (b) late dry season in each of six vegetation types over time on Cape York Peninsula. Solid lines indicate the model explaining the most deviance with the least effective degrees of freedom for the relationship between fire occurrence and year in the six vegetation types, after accounting for random effects of grazing land tenure, average rainfall, rainfall variation and spatial variation, and, in (b) current-year early dry season fire. These models had 18 knots corresponding to the 18 years of the study period. Dashed lines show the maximum-smooth model, which has the same fixed and random effects as the best-fit model, but has been reduced to three knots to delineate the general trends. Confidence bands are in grey.
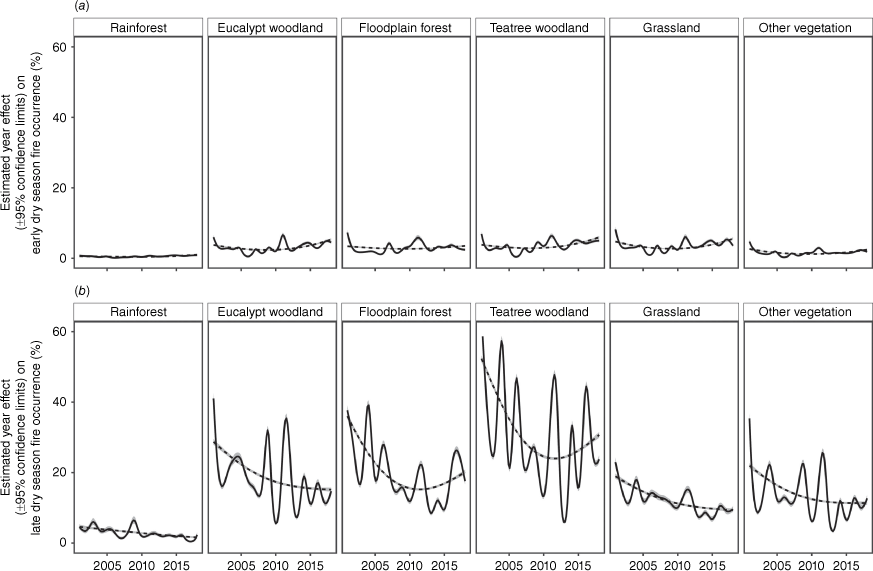
Foliage projective cover
Autumn Persistent Green increased by an average of 5.11 ± 0.04% through the study period, with the slope varying slightly between years (Fig. 4). It increased most in eucalypt woodland and least in grassland and rainforest (Table 1). The GAM model APG trend across vegetation types alone explained 37.6% of the deviance in APG (Supplementary Appendix S6). The best-fit model explaining APG deviance included the following fixed effects: a smooth function of vegetation type and year with 18 knots, and vegetation type, along with previous-year fire season and grazing land tenure, and their individual interactions with vegetation type; and the following random effects: average rainfall, 3-year rainfall anomalies and location. This model explained 58.6% of the deviance in APG, significantly more than the vegetation trend model (P = 0.0000; Fig. 4b). APG differed significantly between vegetation types, being highest in rainforest and lowest in grassland. Fire had a significant negative effect on APG in the subsequent autumn in all vegetation types, with the greatest effect in rainforest and least in eucalypt woodland (Fig. 5a). The impact of EDS fire on APG was consistently greater than that of LDS fire. Grazing land tenure had a significant positive impact on APG in grassland and teatree woodland, and a significant negative impact in all other vegetation types (Fig. 5b).
Generalised additive models for changes in Autumn Persistent Green in each of six vegetation types of over time on Cape York Peninsula (±95% confidence intervals) (a) before and (b) after accounting for interactions with previous-year fire occurrence and grazing land tenure, and the random effects of average rainfall, rainfall variation and spatial variation. Both models had 18 knots corresponding to the 18 years of the study period. Confidence bands are in grey.
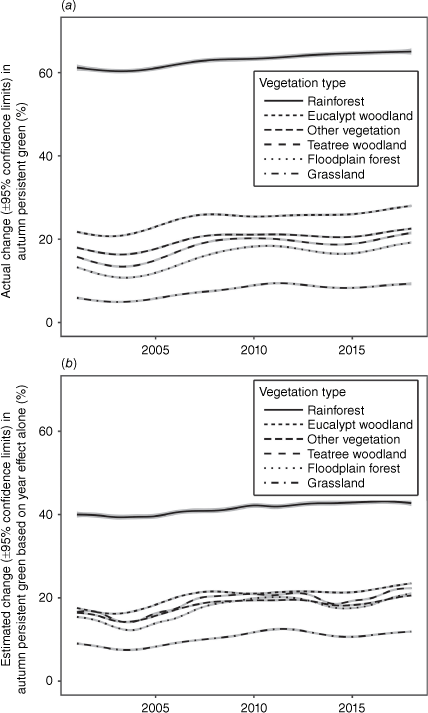
Vegetation type | n | Cape York Peninsula | AANR | ||
---|---|---|---|---|---|
Mean APG change | s.e.m. | Mean APG change | s.e.m. | ||
Rainforest | 959 | 3.69 | 0.31 | – | – |
Eucalypt woodland | 5246 | 6.17 | 0.08 | 7.88 | 0.04 |
Floodplain forest | 5742 | 5.87 | 0.08 | – | – |
Teatree woodland | 5591 | 5.70 | 0.08 | 9.64 | 0.06 |
Grassland | 4836 | 3.34 | 0.07 | 6.99 | 0.10 |
Other | 5248 | 4.50 | 0.09 | – | – |
Study area | 27 622 | 5.11 | 0.04 | – | – |
Vegetation structural change
Between 2001 and 2021, the number of small woody plants (1–3 m) in the monitoring plots in and around AANR increased five-fold, from an average of 586 ± 5 to 2921 ± 29 plants per hectare. The number of large woody plants (>3 m) increased four-fold in the same period, reaching an average of 1529 ± 23 plants per hectare. Two-sampled sign tests comparing the eight sets of plots in 2001 and 2021 indicated that the increases in the number of small and large plants were equally significant (S = 0; P = 0.0078; Fig. 6).
Changes in number of woody plants in eight 200 m2 monitoring plots in and around Artemis Antbed Nature Refuge between 2001 and 2021. Whiskers represent 1.5 times interquartile range.
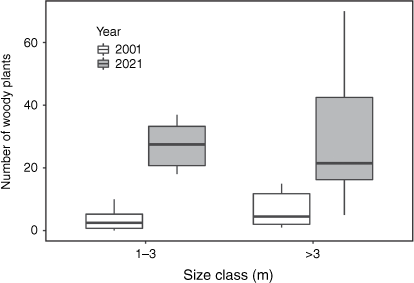
In each of the three vegetation types, APG increases in AANR between 2001 and 2018 were substantially higher than CYP averages for the same period, with those in grassland and teatree woodland being roughly twice as high (Table 1). Two-sample sign tests indicated that median APG significantly increased in grassland from 14 to 21 (S = 2073, P = 0.000), and in teatree woodland from 26 to 38 (S = 6136, P = 0.000; Fig. 7e). Woody encroachment within the grassland/teatree woodland complex between 2001 and 2021 (Fig 7a-b) was also evident in the APG images and variograms (Table 2, Fig. 7c-f). High sill values for this complex indicated a highly variable structure in 2001, and its increase of 48% by 2020 indicated patchy woodland encroachment, rather than a general thickening of the foliage of existing plants. Although eucalypt woodland had a significant APG increase of similar magnitude (from 35 to 42; S = 12 984, P = 0.000), variography suggested this was not caused by structural change. The low sill indicated a relatively uniform canopy; and low change-in-sill indicated moderate structural change over time. Low nugget-to-partial-sill ratios in grassland/teatree woodland suggested that – in both years – the main source of APG increase was an increase in canopy area between pre-existing patches of trees and patches of grasses. By contrast, higher nugget-to-partial-sill ratios in eucalypt woodland indicated a predominance of within-pixel (and therefore within-canopy) variation in FPC, and the drop in this metric by 2020 indicated increasing within-canopy uniformity. As we confined our measurements inside the two vegetation communities, neither the range (154 and 124 for grassland/teatree woodland; 185 and 145 for eucalypt woodland) nor form (all exponential) of either variogram changed substantially, and there were no secondary peaks. In summary, the variogram parameters are consistent with teatree invasion of grassland, as shown by the high and increasing sill; and that eucalypt woodland – rather than undergoing structural change – has experienced infilling of the existing canopy, as shown by the reduced nugget-to-partial-sill ratio.
Changes in Autumn Persistent Green (APG) in Artemis Antbed Nature Refuge. Monitoring plot AR03 in grassland/teatree woodland in (a) 2001 and (b) 2021; APG in (c) 2001 and (d) 2020; (e) quantile box plot of APG for grassland/teatree woodland and eucalypt woodland in 2001 and 2020 (whiskers represent 1.5 times interquartile range); and (f) variograms of APG for grassland/teatree woodland and eucalypt woodland in 2001 and 2020. Source of APG data: Department of Environment and Science (2022).
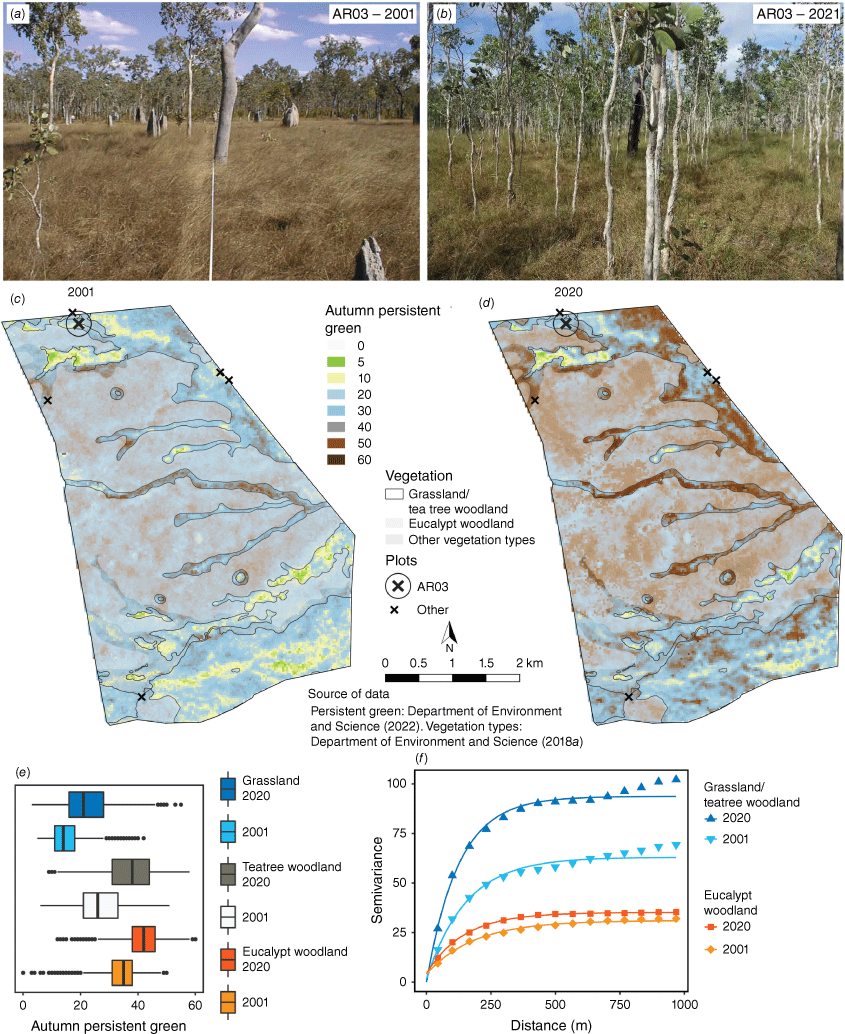
Habitat | Year | Variogram parametersA | Variogram comparisonsB | ||||||
---|---|---|---|---|---|---|---|---|---|
Nugget | Partial sill | Sill | Nugget/partial sill | Range | n | K–S statistic | P-value | ||
Grassland/teatree woodland | 2001 | 1.51 | 61.5 | 63.04 | 0.0239 | 154 | |||
Grassland/teatree woodland | 2020 | 0.00 | 93.7 | 93.71 | 0.0000 | 124 | 15 | 0.800 | 0.000 |
Eucalypt woodland | 2001 | 4.06 | 27.0 | 31.10 | 0.1305 | 185 | |||
Eucalypt woodland | 2020 | 4.35 | 30.8 | 35.16 | 0.1236 | 145 | 15 | 0.667 | 0.002 |
AFor definition of variogram parameters, see Supplementary Appendix S4.
BVariogram comparisons undertaken using Kolmogorov–Smirnov (K–S) tests.
Discussion
This study confirmed that FPC increased in our study area between 2001 and 2018, with APG averaging an increase of 5.11 ± 0.04%. This increase is commensurate with a CO2-driven 10–15% NDVI increase across a similar study area over the last 38 years found using two other satellite products (Surface Reflectance provided by NOAA’s AVHRR (Advanced Very High Resolution Radiometer) and the MODIS product provided by NASA (National Aeronautics and Space Administration); Rifai et al. 2022). The novelty of our study is that it begins to unpick the nature of this remotely-sensed greening. Our findings showed FPC increases in both woody vegetation and grassland, and our on-ground monitoring and variography linked these increases in grassland – but not in eucalypt woodland – to structural vegetation change (as per Fig. 1d). We were also able to identify the factors driving FPC increase.
Measuring changes in FPC and vegetation structure
The Persistent Green product, specifically designed to capture FPC at a fine (30 m) resolution, allowed us to separate out changes in canopy foliage from those in the grass layer. Other measures of primary productivity with a coarser resolution (500 m for MODIS and 0.5° for AVHRR), and that do not remove the effect of ground cover (e.g. NDVI), will provide less accurate measures of FPC.
When combined with variography, APG was also useful for tracking the conversion from grassland to woodland, and for assessing within-canopy changes in woodland. Vegetation structure was reflected in the variogram sill, which was higher in grassland in the process of being invaded by woodland than it was in the relatively uniform eucalypt woodland. The documented patchy transition from grassland to woodland was captured in the 48% increase in sill, while the reduction in the nugget-to-partial sill ratio suggested only within-canopy FPC increases in eucalypt woodland. Variography applied to coarser-scaled products would be less able to detect such changes, as within-pixel variation at a 500 m resolution would be less able to differentiate between within-canopy and between-canopy changes in FPC.
Our assessment of vegetation change from grassland to teatree woodland was greatly enhanced by access to permanently-marked vegetation plots that were first measured over two decades ago (Murphy et al. 2021). Site photographs (Supplementary Appendix S1) showed that woody invasion of grasslands between 2001 and 2021 was patchy, creating textural relief that was captured in the increasing sill. Several other sets of relocatable monitoring sites exist in the Australian tropical savannas, including the on-ground assessments made for the initial calibration of the Persistent Green Product (Scarth et al. 2015). Remeasurement of these plots should form the basis of assessing the relationship between APG and vegetation change, particularly of whether increases in APG have coincided with increases in tree number and basal area or just crown density, and whether these relationships have changed through time. However, a dearth of permanent plots in our study area means investment in new plots will be required to track structural change in most vegetation types into the future. Economic stringencies and insecurity of tenure make establishing long term monitoring plots difficult (Lindenmayer and Likens 2018). We propose that a network of camera traps, which are now being used as an economical means of canopy monitoring (Chianucci et al. 2021), could be established in key areas to capture changes in canopy cover and vegetation structure as an economical adjunct to physical tree monitoring, and that the results be linked to change in APG and its variography over periods of at least 10 years.
Relationship between increases in FPC and woody biomass
In theory, CO2-driven increases in foliage production should result in increases in woody biomass – and hence carbon stocks – in the form of tree recruitment, tree height and/or tree basal area (Cook et al. 2015). Such changes would be consistent with CO2 fertilisation improving water-use efficiency and releasing the trees from strong water limitation. While increases in APG could be linked to increases in stem density in grassland in our study area, the variography suggests that this relationship breaks down once a uniform woodland canopy is established. Eucalypt woodland appears to be the most stable vegetation type on CYP (Crowley and Garnett 1998). Indeed, there is little evidence of elevated tree recruitment in eucalypt woodland anywhere in Australian tropical savannas, even where fire has been excluded for up to 23 years (Murphy et al. 2015; Prior et al. 2020). Landholder perceptions to the contrary (Lankester 2006) are possibly based on selective memory of increases in the wetter parts of rainfall-driven thickening and thinning cycles (Fensham et al. 2009). Furthermore, CO2-enhanced growth was found in only one of the two savanna eucalypts tested in pot trials (Duff et al. 1994).
Variography may not differentiate between simple increases in leaf density and the production of new stems or branches that occurs without extending the existing canopy outline. However, in a global review of tree growth rates, Rahman et al. (2019) found no evidence of accelerated growth that could be attributed to CO2 fertilisation, and concluded either that this was because of a decoupling of leaf production from wood production, or that any enhanced sequestration was being directed underground. They concluded that the positive effects of CO2 on leaf growth may be offset by the adverse effects of associated temperature rise. Any extra carbon assimilated by the leaves may also be lost through respiration (Jiang et al. 2020b), or not converted to woody biomass when soil nutrients are limiting (Jiang et al. 2020a), as they are through much of CYP (Biggs and Philip 1995). Hence, both the variography and literature indicate that FPC increase is unlikely to indicate elevated carbon sequestration in the dominant eucalypt woodlands of northern Australia.
Increases in APG in our study may not even indicate increased leaf production, but simply result from the same biomass of leaves being retained in the canopy for longer, having less horizontal overlap, being held at a more horizontal angle, or having an increased surface area-to-mass ratio. Of these factors, extended period of leaf retention is known to occur under elevated CO2 (McCarthy et al. 2007). Eucalypts in the study area include a mix of semi-deciduous species, which regrow their leaves annually (Myers et al. 1998), and evergreen species, which can lose up to 40% of their leaves towards the end of the dry season (O’Grady et al. 2000). However, impacts of CO2 on leaf retention through the wet season have not been studied. The impact of CO2 on leaf overlap and leaf angle has only been studied in a single eucalypt species, with no apparent effect (Pisek et al. 2021). Leaf-area-to-mass ratio decreases under elevated CO2, but increases in response to the associated improvement to water-use efficiency (Whitehead and Beadle 2004; Rahman et al. 2019). Without detailed knowledge of these responses for the species in our study area, it is not possible to link the APG increases to increased leaf biomass or carbon sequestration (Jiang et al. 2020b). The predominance of recent NDVI increases in Australia may simply result from the foliage of sclerophyllous plants being most responsive to CO2 fertilisation (Niinemets et al. 2011), but nutrient limitations may prevent this advantage being converted to carbon sequestration (Jiang et al. 2020a). We therefore conclude that an increase in FPC cannot be equated to increases in tree number or size, or above-ground biomass. While FPC does increase as stem density increases in open vegetation, such as in the case of grassland conversion to teatree woodland, our variography shows that it can also increase at an even greater rate in the absence of vegetation structural change.
Drivers
CO2 fertilisation is considered to be the main driver of recent increases in FPC (Ma et al. 2016; Zhu et al. 2016). The year-on-year APG increase shown in our data (year effect after the removal of the effects of other driving processes) would suggest this is the case. Our model also showed that both fire and grazing tenure affected FPC, but had little impact on region-wide increases.
Fire in the previous year reduced APG, with EDS fires having a greater impact than LDS fires in all vegetation types. A negative effect of fire on APG was to be expected, as fires in Australian tropical savannas may scorch the canopy, or kill branches and whole trees to the point of elimination of fire sensitive species (Prior et al. 2009; Bowman et al. 2014; Murphy et al. 2014). Nevertheless, it seems counterintuitive that EDS fires had the greatest impact on foliage cover. Elsewhere in northern Australia, LDS fires are typically more severe than EDS fires, and thus more lethal to trees (Werner and Prior 2013; Murphy et al. 2015; Cowley 2020). However, milder weather in our study area, driven by a maritime climate, and a shorter period of extreme fire conditions (Stanton 1992; Perry et al. 2020) may limit the capacity of LDS fires to damage the canopy or kill trees. EDS fires may simply be more detrimental to foliage because they occur when the plants are actively growing (Miller 2000; Prior et al. 2006), whereas LDS fires occur when the canopy has thinned, and leaves are senescing (O’Grady et al. 2000). Fire-sensitivity of actively growing plants is the rationale behind using storm-burns to control woody encroachment. Storm-burns have been found to be effective at opening the vegetation structure in and around AANR (Crowley et al. 2009). Unfortunately, wet season cloud cover – and the fact that storm burns may be lit either side of the LDS-EDS boundary – mean APG imagery cannot assess their effectiveness at the regional level. Regardless of the effect of fire season, the overall decline in fire incidence through the study period is likely to have marginally facilitated the increase in APG, and the shift between LDS and EDS fires for carbon abatement (greatest in eucalypt woodland as a result of the Savanna Burning program; Edwards et al. 2021) has not been enough to reverse this effect.
APG was lower on grazing land than on non-grazing land in most vegetation types. This may also seem counterintuitive, as grazing should release trees from competition from grasses and reduce fire intensity (Archer 1995; Ash and Corfield 1998; Liedloff et al. 2001; Beringer et al. 2007; Riginos 2009; Pillay and Ward 2021). In rainforest, this difference may be explained by cattle browsing on seedlings and saplings reducing the recruitment of trees to the canopy (Ondei et al. 2017). In eucalypt woodland, the grazing land effect may result from tree health being adversely affected by cattle stripping bark (Guerreiro et al. 2015). It may also be an artefact of the most densely-vegetated country being preferentially relinquished from grazing land, or not selected as grazing land in the first place (Holmes 2010). This is particularly likely for rainforest, with the most luxuriant stands being conserved in protected areas. The reverse pattern of elevated APG on grazing land in grassland and – to a lesser extent – in teatree woodland is consistent with the woodland encroachment documented both in this study and elsewhere in grazed tropical savannas (Crowley and Garnett 1998; Sharp and Bowman 2004; Bond and Midgley 2012; Archer et al. 2017). Our results confirm that – regardless of other drivers – woody encroachment of grasslands is likely to be most extreme under cattle grazing.
Conclusions
All vegetation types in our study area experienced increases in APG over the period 2001 to 2018. Based on unexplained year-on-year increases in APG, CO2 fertilisation may be responsible for most of this increase. A review of the literature shows that sclerophylly makes the foliage of Australian plants most responsive to CO2 fertilisation, but the nutrient limited soils make them least able to convert this to biomass accumulation. Hence, FPC increase on CYP does not convincingly demonstrate elevated carbon sequestration. Nor does it indicate vegetation change, except in grassland, where both textural analysis of the APG imagery and on-ground plot monitoring demonstrated woodland invasion. While FPC increase in eucalypt woodland was double that in grassland, variography indicated no change in stem density in this vegetation type.
We demonstrated a clear link between fire regime and FPC, with EDS fires being more effective than LDS fires at restricting FPC increases into the subsequent autumn, particularly in grassland and rainforest. Though counterintuitive, this finding is consistent with foliage being most fire-sensitive when plants are actively growing. Given the differences in fire severity across northern Australia, this effect may not apply outside our study area. In grassland and teatree woodland, we also found that FPC was highest on grazing land, reflecting the role of grazing in driving woodland encroachment. That the reverse was true in all other vegetation types may be a true reflection of the impact of grazing on tree health, or an artefact of land-use decision making.
Untangling whether CO2-driven APG increases result from changes in leaf morphology, longevity, number or size; canopy density or extent; or tree number or size is essential for understanding the implications of APG changes for carbon accounting. Further, given the poor correspondence between changes in APG and vegetation structure, we caution against using remote sensing to assess changes of carbon stocks without the assistance of variography, or to determine the relative importance of drivers of structural vegetation change. However, the questions raised about the contribution of grazing to – and the relative impacts of EDS and LDS fires on – woodland encroachment are worthy of further investigation.
Data availability
The APG data layers are openly available in the TERN Data Discovery Portal at https://portal.tern.org.au. All code used in this study and the stratified sample data for Cape York Peninsula are available in GitHub at https://github.com/GM-Crowley/CYP. The code for extracting APG from this repository to produce the variograms for our case study area is also available at the above GitHub address.
Acknowledgements
We acknowledge the First Nations Peoples of Cape York Peninsula. We thank James Donaldson, Zoe Williams and Marcus Mulholland (GSNRM), Kerri Woodcock (Cape York NRM), Martine Maron (University of Queensland) and Leasie Felderhof and Linda Brosnan (Firescape Science) for their administrative support; Susan Shephard, Wendy Cooper and Rigel Jensen for assistance in the field; Al Healy, Robert Denham, Fiona Watson (Queensland Department of Environment and Science; DES) and Peter Scarth (University of Queensland) for producing the APG layers and advising on their application; Robyn Cowley for advice on calculating multi-year rainfall anomalies; Allan Burr for beta-testing our R code; Noam Ross for his excellent online GAM course, Mariska Marnane for drafting Fig. 1, and Andy Gillison (Center for Biodiversity Management), Jeremy Russell-Smith and Cameron Yates (Charles Darwin University), Fiona Watson (DES), Anna Richards (CSIRO), Paul Novelly, and an anonymous reviewer for constructive comments on the manuscript. We are most grateful to David Gillieson, who provided an exceptional level of advice and support throughout this study.
References
Accad A, Kelley JAR, Richter D, Neldner VJ, Li J (2021) ‘Remnant regional ecosystem vegetation in Queensland, analysis 1997-2019.’ (Queensland Department of Environment and Science: Brisbane) Available at https://www.qld.gov.au/environment/plants-animals/plants/ecosystems/remnant-vegetation [accessed 14 April 2023]
Anderegg WRL, Kane JM, Anderegg LDL (2013) Consequences of widespread tree mortality triggered by drought and temperature stress. Nature Climate Change 3, 30-36.
| Crossref | Google Scholar |
Archer S (1995) Herbivore mediation of grass-woody plant interactions. Tropical Grasslands 29, 218-235.
| Google Scholar |
Ash AJ, Corfield JP (1998) Influence of pasture condition on plant selection patterns by cattle: its implications for vegetation change in a monsoon tallgrass rangeland. Tropical Grasslands 32, 178-187.
| Google Scholar |
Beringer J, Hutley LB, Tapper NJ, Cernusak LA (2007) Savanna fires and their impact on net ecosystem productivity in North Australia. Global Change Biology 13, 990-1004.
| Crossref | Google Scholar |
Biggs AJW, Philip SR (1995) Soils of Cape York Peninsula. Land Resources Bulletin QV95001. (Department of Primary Industries: Mareeba) Available at https://www.publications.qld.gov.au/dataset/f8fbc5f1-3fa7-4849-b04d-3ab581c5d03d/resource/e90e1bcf-477a-4f22-96e2-f6d90f3a4163/download/cypqv95001soilsofcapeyorkpeninsula.pdf [accessed 14 April 2023]
Bond WJ, Midgley GF (2000) A proposed CO2-controlled mechanism of woody plant invasion in grasslands and savannas. Global Change Biology 6, 865-869.
| Crossref | Google Scholar |
Bond WJ, Midgley GF (2012) Carbon dioxide and the uneasy interactions of trees and savannah grasses. Philosophical Transactions of the Royal Society B: Biological Sciences 367, 601-612.
| Crossref | Google Scholar |
Bowman DMJS, Fensham RJ (1991) Response of a monsoon-forest savanna boundary to fire protection, Weipa, northern Australia. Australian Journal of Ecology 16, 111-118.
| Crossref | Google Scholar |
Bowman DMJS, MacDermott HJ, Nichols SC, Murphy BP (2014) A grass–fire cycle eliminates an obligate-seeding tree in a tropical savanna. Ecology and Evolution 4, 4185-4194.
| Crossref | Google Scholar |
Bultan S, Nabel JEMS, Hartung K, Ganzenmüller R, Xu L, Saatchi S, Pongratz J (2022) Tracking 21st century anthropogenic and natural carbon fluxes through model-data integration. Nature Communications 13, 5516.
| Crossref | Google Scholar |
Bureau of Meteorology (2006) Köppen climate classification (base climate related classification datasets). Available at http://www.bom.gov.au/climate/averages/climatology/gridded-data-info/metadata/md_koppen_classification.shtml [accessed 12 April 2023]
Bureau of Meteorology (2008) Australian average rainfall data from 1961 to 1990. Bioregional assessment source dataset. Available at https://data.gov.au/data/dataset/fd91f2d4-2cc8-4d5d-9f67-8fe8af1e2676 [accessed 12 April 2023]
Bureau of Meteorology (2019) Regional weather and climate guide: Cape York. Available at http://www.bom.gov.au/climate/climate-guides/guides/025-Cape-York-QLD-Climate-Guide.pdf [accessed 14 April 2023]
Bureau of Meteorology (2021) Rainfall variability (1900-2019). (Commonwealth of Australia) Available at http://www.bom.gov.au/jsp/ncc/climate_averages/rainfall-variability/index.jsp [accessed 12 April 2023]
Bureau of Meteorology (2022) Long-range weather and climate. Available at http://www.bom.gov.au/climate [accessed 11 April 2023]
Charles Darwin University (2021) NAFI: North Australian fire information website. (Charles Darwin University: Darwin, NT) Available at https://www.firenorth.org.au [accessed 12 April 2023]
Chianucci F, Bajocco S, Ferrara C (2021) Continuous observations of forest canopy structure using low-cost digital camera traps. Agricultural and Forest Meteorology 307, 108516.
| Crossref | Google Scholar |
Cook GD, Liedloff AC, Murphy BP (2015) Predicting the effects of fire management on carbon stock dynamics using statistical and process-based modelling. In ‘Carbon accounting and savanna fire management’. (Eds BP Murphy, AC Edwards, CP Meyer, J Russell-Smith) pp. 295–315. (CSIRO Publishing: Melbourne, Vic., Australia)
Cowley R (2020) Woody cover change in grazing lands of northern Australia since 1988. Range Management Newsletter. Vol. 20. Available at https://austrangesoc.com.au/woody-cover-change-in-grazing-lands-of-northern-australia-since-1988 [accessed 14 April 2020]
Crowley GM, Garnett ST (1998) Vegetation change in the grasslands and grassy woodlands of east-central Cape York Peninsula, Australia. Pacific Conservation Biology 4, 132-148.
| Crossref | Google Scholar |
Crowley GM, Garnett ST (2000) Changing fire management in the pastoral lands of Cape York Peninsula of northeast Australia, 1623 to 1996. Australian Geographical Studies 38, 10-26.
| Crossref | Google Scholar |
Crowley G, Garnett S, Shephard S (2009) Impact of storm-burning on Melaleuca viridiflora invasion of grasslands and grassy woodlands on Cape York Peninsula, Australia. Austral Ecology 34, 196-209.
| Crossref | Google Scholar |
David B, Lourandos H (1997) 37,000 years and more in tropical Australia: investigating long-term archaeological trends in Cape York Peninsula. Proceedings of the Prehistoric Society 63, 1-23.
| Crossref | Google Scholar |
Department of Environment and Science (2018) Pre-clearing Broad Vegetation Groups - Queensland Version 4.0 (14 Dec 2018). https://qldspatial.information.qld.gov.au/catalogue/ [accessed 22 June 2017, superseded by Version 6]
Department of Environment and Science (2022) Seasonal persistent green - Landsat, JRSRP algorithm Version 3.0, Australia coverage. Terrestrial Ecosystem Research Network. (Queensland Government: Brisbane) Available at https://portal.tern.org.au/metadata/TERN% 2Fdd359b61-3ce2-4cd5-bc63-d54d2d0e2509 [accessed 12 April 2023]
Donohue RJ, Roderick ML, McVicar TR, Farquhar GD (2013) Impact of CO2 fertilization on maximum foliage cover across the globe’s warm, arid environments. Geophysical Research Letters 40, 3031-3035.
| Crossref | Google Scholar |
Duff GA, Berryman CA, Eamus D (1994) Growth, biomass allocation and foliar nutrient contents of two Eucalyptus species of the wet-dry tropics of Australia grown under CO2 enrichment. Functional Ecology 8, 502-508.
| Crossref | Google Scholar |
Edwards A, Archer R, De Bruyn P, Evans J, Lewis B, Vigilante T, Whyte S, Russell-Smith J (2021) Transforming fire management in northern Australia through successful implementation of savanna burning emissions reductions projects. Journal of Environmental Management 290, 112568.
| Crossref | Google Scholar |
Felderhof L, Gillieson D (2006) Comparison of fire patterns and fire frequency in two tropical savanna bioregions. Austral Ecology 31, 736-746.
| Crossref | Google Scholar |
Fensham RJ, Holman JE (1999) Temporal and spatial patterns in drought-related tree dieback in Australian savanna. Journal of Applied Ecology 36, 1035-1050.
| Crossref | Google Scholar |
Fensham RJ, Fairfax RJ, Ward DP (2009) Drought-induced tree death in savanna. Global Change Biology 15, 380-387.
| Crossref | Google Scholar |
Fisher A, Scarth P, Armston J, Danaher T (2018) Relating foliage and crown projective cover in Australian tree stands. Agricultural and Forest Meteorology 259, 39-47.
| Crossref | Google Scholar |
Flood N, Watson F, Collett L (2019) Using a U-net convolutional neural network to map woody vegetation extent from high resolution satellite imagery across Queensland, Australia. International Journal of Applied Earth Observation and Geoinformation 82, 101897.
| Crossref | Google Scholar |
García Criado M, Myers-Smith IH, Bjorkman AD, Lehmann CER, Stevens N (2020) Woody plant encroachment intensifies under climate change across tundra and savanna biomes. Global Ecology and Biogeography 29, 925-943.
| Crossref | Google Scholar |
Guerreiro MF, Nicodemo MLF, Porfírio-da-Silva V (2015) Vulnerability of ten Eucalyptus varieties to predation by cattle in a silvopastoral system. Agroforestry Systems 89, 743-749.
| Crossref | Google Scholar |
Hamada Y, Szoldatits K, Grippo M, Hartmann HM (2019) Remotely sensed spatial structure as an indicator of internal changes of vegetation communities in desert landscapes. Remote Sensing 11, 1495.
| Crossref | Google Scholar |
Haverd V, Smith B, Canadell JG, Cuntz M, Mikaloff-Fletcher S, Farquhar G, Woodgate W, Briggs PR, Trudinger CM (2020) Higher than expected CO2 fertilization inferred from leaf to global observations. Global Change Biology 26, 2390-2402.
| Crossref | Google Scholar |
Hijmans RJ, van Etten J (2012) raster: Geographic analysis and modeling with raster data. R package version 2.0-12. Available at http://CRAN.R-project.org/package=raster/ [accessed 4 Jul 2022]
Holmes J (2010) The multifunctional transition in Australia’s tropical savannas: the emergence of consumption, protection and indigenous values. Geographical Research 48, 265-280.
| Crossref | Google Scholar |
Hovenden MJ, Williams AL (2010) The impacts of rising CO2 concentrations on Australian terrestrial species and ecosystems. Austral Ecology 35, 665-684.
| Crossref | Google Scholar |
Jiang M, Caldararu S, Zhang H, Fleischer K, Crous KY, Yang J, De Kauwe MG, Ellsworth DS, Reich PB, Tissue DT, Zaehle S, Medlyn BE (2020a) Low phosphorus supply constrains plant responses to elevated CO2: a meta-analysis. Global Change Biology 26, 5856-5873.
| Crossref | Google Scholar |
Jiang M, Medlyn BE, Drake JE, Duursma RA, Anderson IC, Barton CVM, Boer MM, Carrillo Y, Castañeda-Gómez L, Collins L, Crous KY, De Kauwe MG, dos Santos BM, Emmerson KM, Facey SL, Gherlenda AN, Gimeno TE, Hasegawa S, Johnson SN, Kännaste A, Macdonald CA, Mahmud K, Moore BD, Nazaries L, Neilson EHJ, Nielsen UN, Niinemets Ü, Noh NJ, Ochoa-Hueso R, Pathare VS, Pendall E, Pihlblad J, Piñeiro J, Powell JR, Power SA, Reich PB, Renchon AA, Riegler M, Rinnan R, Rymer PD, Salomón RL, Singh BK, Smith B, Tjoelker MG, Walker JKM, Wujeska-Klause A, Yang J, Zaehle S, Ellsworth DS (2020b) The fate of carbon in a mature forest under carbon dioxide enrichment. Nature 580, 227-231.
| Crossref | Google Scholar |
Kamal M, Phinn S, Johansen K (2014) Characterizing the spatial structure of mangrove features for optimizing image-based mangrove mapping. Remote Sensing 6, 984-1006.
| Crossref | Google Scholar |
Lankester, AJ (2006) Landholder experiences and perceptions of the extent, onset and causes of woody vegetation change: Northern Gulf scoping study. Final Report to the Tropical Savannas Cooperative Research Centre. (James Cook University: Townsville, Qld) Available at https://www.researchgate.net/profile/Ally_Lankester/publication/265162983_Landholder_perceptions_of_vegetation_change_in_the_Northern_Gulf_Australia/links/540172dc0cf23d9765a4964e.pdf [accessed 14 April 2023]
Laughlin GP, Zuo H, Walcott J, Bugg AL (2003) The rainfall reliability wizard—a new tool to rapidly analyse spatial rainfall reliability with examples. Environmental Modelling & Software 18, 49-57.
| Crossref | Google Scholar |
Liao Z, Van Dijk AIJM, He B, Larraondo PR, Scarth PF (2020) Woody vegetation cover, height and biomass at 25-m resolution across Australia derived from multiple site, airborne and satellite observations. International Journal of Applied Earth Observation and Geoinformation 93, 102209.
| Crossref | Google Scholar |
Liedloff AC, Coughenour MB, Ludwig JA, Dyer R (2001) Modelling the trade-off between fire and grazing in a tropical savanna landscape, northern Australia. Environment International 27, 173-180.
| Crossref | Google Scholar |
Ma X, Huete A, Cleverly J, Eamus D, Chevallier F, Joiner J, Poulter B, Zhang Y, Guanter L, Meyer W, Xie Z, Ponce-Campos G (2016) Drought rapidly diminishes the large net CO2 uptake in 2011 over semi-arid Australia. Scientific Reports 6, 37747.
| Crossref | Google Scholar |
McCarthy HR, Oren R, Finzi AC, Ellsworth DS, Kim H-S, Johnsen KH, Millar B (2007) Temporal dynamics and spatial variability in the enhancement of canopy leaf area under elevated atmospheric CO2. Global Change Biology 13, 2479-2497.
| Crossref | Google Scholar |
Murphy BP, Lehmann CER, Russell-Smith J, Lawes MJ (2014) Fire regimes and woody biomass dynamics in Australian savannas. Journal of Biogeography 41, 133-144.
| Crossref | Google Scholar |
Murphy SA, Shephard S, Crowley GM, Garnett ST, Webster P, Cooper W, Jensen R (2021) Pre-management actions baseline report for Artemis Antbed Parrot Nature Refuge. (National Environmental Science Program, Threatened Species Recovery Hub: Brisbane, Qld) Available at https://www.nespthreatenedspecies.edu.au/publications-and-tools/pre-management-actions-baseline-report-for-artemis-antbed-parrot-nature-refuge [accessed 14 April 2023]
Myers BA, Williams RJ, Fordyce I, Duff GA, Eamus D (1998) Does irrigation affect leaf phenology in deciduous and evergreen trees of the savannas of northern Australia? Australian Journal of Ecology 23, 329-339.
| Crossref | Google Scholar |
Neldner VJ, Fensham RJ, Clarkson JR, Stanton JP (1997) The natural grasslands of Cape York Peninsula, Australia: description, distribution and conservation status. Biological Conservation 81, 121-136.
| Crossref | Google Scholar |
Niinemets Ü, Flexas J, Peñuelas J (2011) Evergreens favored by higher responsiveness to increased CO2. Trends in Ecology & Evolution 26, 136-142.
| Crossref | Google Scholar |
O’Grady AP, Chen X, Eamus D, Hutley LB (2000) Composition, leaf area index and standing biomass of eucalypt open forests near Darwin in the Northern Territory, Australia. Australian Journal of Botany 48, 629-638.
| Crossref | Google Scholar |
Ondei S, Prior LD, Vigilante T, Bowman DMJS (2017) Fire and cattle disturbance affects vegetation structure and rain forest expansion into savanna in the Australian monsoon tropics. Journal of Biogeography 44, 2331-2342.
| Crossref | Google Scholar |
Pawley MDM, McArdle BH (2021) Inferences with spatial autocorrelation. Austral Ecology 46, 942-949.
| Crossref | Google Scholar |
Pebesma EJ (2004) Multivariable geostatistics in S: the gstat package. Computers & Geosciences 30, 683-691.
| Crossref | Google Scholar |
Perry JJ, Cook GD, Graham E, Meyer CP, Murphy HT, VanDerWal J (2020) Regional seasonality of fire size and fire weather conditions across Australia’s northern savanna. International Journal of Wildland Fire 29, 1-10.
| Crossref | Google Scholar |
Piao S, Wang X, Park T, Chen C, Lian X, He Y, Bjerke JW, Chen A, Ciais P, Tømmervik H, Nemani RR, Myneni RB (2020) Characteristics, drivers and feedbacks of global greening. Nature Reviews Earth & Environment 1, 14-27.
| Crossref | Google Scholar |
Pillay T, Ward D (2021) Grass competition is more important than fire for suppressing encroachment of Acacia sieberiana seedlings. Plant Ecology 222, 149-158.
| Crossref | Google Scholar |
Pisek J, Řezníčková L, Adamson K, Ellsworth DS (2021) Leaf inclination angle and foliage clumping in an evergreen broadleaf Eucalyptus forest under elevated atmospheric CO2. Australian Journal of Botany 69, 622-629.
| Crossref | Google Scholar |
Prior LD, Brook BW, Williams RJ, Werner PA, Bradshaw CJA, Bowman DMJS (2006) Environmental and allometric drivers of tree growth rates in a north Australian savanna. Forest Ecology and Management 234, 164-180.
| Crossref | Google Scholar |
Prior LD, Murphy BP, Russell-Smith J (2009) Environmental and demographic correlates of tree recruitment and mortality in north Australian savannas. Forest Ecology and Management 257, 66-74.
| Crossref | Google Scholar |
Prior LD, Whiteside TG, Williamson GJ, Bartolo RE, Bowman DMJS (2020) Multi-decadal stability of woody cover in a mesic eucalypt savanna in the Australian monsoon tropics. Austral Ecology 45, 621-635.
| Crossref | Google Scholar |
Queensland Herbarium (2018) Remnant 2017 Broad Vegetation Groups of Queensland (BVG) Version 4.0 (14 Dec 2018). (Department of Science, Information Technology and Innovation: Brisbane) Available at http://qldspatial.information.qld.gov.au/catalogue [accessed 4 July 2022, superceded by version 5.2]
Rahman M, Islam M, Gebrekirstos A, Bräuning A (2019) Trends in tree growth and intrinsic water-use efficiency in the tropics under elevated CO2 and climate change. Trees 33, 623-640.
| Crossref | Google Scholar |
R Core Team (2020) R: A language and environment for statistical computing. Version 4.1.3 (2020-10-10). (R Foundation for Statistical Computing: Vienna, Austria) Available at https://www.R-project.org/ [accessed 9 December 2020]
Rifai SW, De Kauwe MG, Ukkola AM, Cernusak LA, Meir P, Medlyn BE, Pitman AJ (2022) Thirty-eight years of CO2 fertilization has outpaced growing aridity to drive greening of Australian woody ecosystems. Biogeosciences 19, 491-515.
| Crossref | Google Scholar |
Riginos C (2009) Grass competition suppresses savanna tree growth across multiple demographic stages. Ecology 90, 335-340.
| Crossref | Google Scholar |
Russell-Smith J, Stanton PJ, Edwards AC, Whitehead PJ (2004a) Rain forest invasion of eucalypt-dominated woodland savanna, Iron Range, north-eastern Australia: II. Rates of landscape change. Journal of Biogeography 31, 1305-1316.
| Crossref | Google Scholar |
Russell-Smith J, Stanton PJ, Whitehead PJ, Edwards A (2004b) Rain forest invasion of eucalypt-dominated woodland savanna, Iron Range, north-eastern Australia: I. Successional processes. Journal of Biogeography 31, 1293-1303.
| Crossref | Google Scholar |
Scarth P, Armston J, Flood N, Denham R, Collett L, Watson F, Trevithick B, Muir J, Goodwin N, Tindall D, Phinn S (2015) Operational application of the landsat timeseries to address large area landcover understanding. The International Archives of the Photogrammetry, Remote Sensing and Spatial Information Sciences XL-3/W3, 571-575.
| Crossref | Google Scholar |
Sharp BR, Bowman DMJS (2004) Net woody vegetation increase confined to seasonally inundated lowlands in an Australian tropical savanna, Victoria River District, Northern Territory. Austral Ecology 29, 667-683.
| Crossref | Google Scholar |
Solana-Gutiérrez J, Merino-de-Miguel S (2011) A variogram model comparison for predicting forest changes. Procedia Environmental Sciences 7, 383-388.
| Crossref | Google Scholar |
Stanton JP (1992) J. P. Thomson oration: The neglected lands: recent changes in the ecosystem of Cape York Peninsula and the challenge of their management. Queensland Geographical Journal 7, 1-18.
| Google Scholar |
Stevens N, Erasmus BFN, Archibald S, Bond WJ (2016) Woody encroachment over 70 years in South African savannahs: overgrazing, global change or extinction aftershock? Philosophical Transactions of the Royal Society B: Biological Sciences 371, 20150437.
| Crossref | Google Scholar |
Stevens N., Lehmann C. E. R., Murphy B. P., Durigan G. (2017) Savanna woody encroachment is widespread across three continents. Global Change Biology 23(1), 235-244.
| Crossref | Google Scholar |
Thomas SC, Martin AR (2012) Carbon content of tree tissues: a synthesis. Forests 3, 332-352.
| Crossref | Google Scholar |
van Langevelde F, van de Vijver CADM, Kumar L, van de Koppel J, de Ridder N, van Andel J, Skidmore AK, Hearne JW, Stroosnijder L, Bond WJ, Prins HHT, Rietkerk M (2003) Effects of fire and herbivory on the stability of savanna ecosystems. Ecology 84, 337-350.
| Crossref | Google Scholar |
Werner PA, Prior LD (2013) Demography and growth of subadult savanna trees: interactions of life history, size, fire season, and grassy understory. Ecological Monographs 83, 67-93.
| Crossref | Google Scholar |
Whitehead D, Beadle CL (2004) Physiological regulation of productivity and water use in Eucalyptus: a review. Forest Ecology and Management 193, 113-140.
| Crossref | Google Scholar |
Wood SN (2019) mgcv: Mixed GAM Computation Vehicle with Automatic Smoothness Estimation. Version 1.8-40. Available at https://cran.r-project.org/web/packages/mgcv/index.html [accessed 7 June 2023]
Zhu Z, Piao S, Myneni RB, Huang M, Zeng Z, Canadell JG, Ciais P, Sitch S, Friedlingstein P, Arneth A, Cao C, Cheng L, Kato E, Koven C, Li Y, Lian X, Liu Y, Liu R, Mao J, Pan Y, Peng S, Peñuelas J, Poulter B, Pugh TAM, Stocker BD, Viovy N, Wang X, Wang Y, Xiao Z, Yang H, Zaehle S, Zeng N (2016) Greening of the Earth and its drivers. Nature Climate Change 6, 791-795.
| Crossref | Google Scholar |