Remote sensing applications for prescribed burn research
Anna LoPresti





A
B
C
D
Abstract
Prescribed burning is a key management strategy within fire-adapted systems, and improved monitoring approaches are needed to evaluate its effectiveness in achieving social-ecological outcomes. Remote sensing provides opportunities to analyse the impacts of prescribed burning, yet a comprehensive understanding of the applications of remote sensing for prescribed burn research is lacking. We conduct a literature review of 120 peer-reviewed publications to synthesise the research aims, methodologies, limitations and future directions of remote sensing for the analysis of prescribed fire. Studies evaluating management outcomes found prescribed burning effective for wildfire risk reduction, yet few analysed co-benefits or trade-offs with other management goals. Most studies use passive, spaceborne, low spatial resolution sensors, characterised in the literature as consistent and accessible data sources but limited in detecting small, low-severity and short-duration fires characteristic of prescribed burns. In contrast, active remote sensing approaches including LiDAR are less frequently employed, but show promise for highly accurate, spatially explicit 3D vegetation and fuel load mapping. Remote sensing advances toward higher spatial resolution, more frequent revisit, denser spectral sampling and more data across the electromagnetic spectrum are critical to advancing prescribed fire research, addressing current methodological gaps, and improving fuels and fire management capacity.
Keywords: burnt area mapping, controlled burning, Earth observation, fire detection, fire ecology, fuels reduction, LiDAR, prescribed fire, satellite imagery, wildfire management.
Introduction
Fire plays a critical role in maintaining the health and functioning of fire-adapted ecosystems around the world. The disruption of fire regimes due to anthropogenic factors including fire suppression, climate change and land-use change negatively impacts fire-prone social-ecological systems (Bowman et al. 2009). Prescribed burning – the intentional application of fire to the landscape to achieve management goals – is recognised as a means of reintroducing or maintaining fire regimes within fire-adapted ecosystems, thus promoting ecosystem resilience (Stephens et al. 2012; Ryan et al. 2013; Hunter and Robles 2020). Additionally, prescribed burning is a key component of wildfire management, reducing hazardous fuel loads and altering wildfire risk (Fernandes and Botelho 2003; McWethy et al. 2019). As climate change continues to drive larger and more frequent wildfires in some parts of the globe (Jolly et al. 2015; Abatzoglou and Williams 2016; Pausas and Keeley 2021), the research community has advocated for increased prescribed burning as a hazard reduction strategy with ecological co-benefits (Kolden 2019). Given growing interest in scaling up hazardous fuels treatments and renewed policy attention, research is needed to evaluate and implement prescribed burning as a multi-objective conservation and climate change adaptation strategy.
To effectively evaluate prescribed burn outcomes, monitoring across multiple spatial and temporal scales is often necessary owing to the heterogeneity and long-term impacts of fire on the landscape (Turner et al. 1994; Webster and Halpern 2010). These factors impact the feasibility of in situ measurements, hindering data acquisition and analysis. In response, remote sensing has become increasingly common in fire ecology and management, serving as an important data source for evaluating landscape-scale impacts of fire. Using radiation absorbed or reflected passively from the Earth’s surface or from active pulses emitted from sensors, remote sensing has been used for a range of pre-fire, active-fire and post-fire research applications. These include active fire detection (Xu and Zhong 2017), burn severity assessment (White et al. 1996; Eidenshink et al. 2007; Parks et al. 2014; Veraverbeke et al. 2014), smoke and emissions monitoring (Li et al. 2015), carbon monitoring (Xiao et al. 2019), fuels (Saatchi et al. 2007; Stavros et al. 2018) and fuel moisture content analysis (Qi et al. 2012; Yebra et al. 2013; Xie et al. 2022), and post-fire vegetation recovery monitoring (van Leeuwen et al. 2010; Kibler et al. 2019).
Although studies have synthesised the applications of remote sensing for wildfire (Leblon et al. 2016; Veraverbeke et al. 2018; Szpakowski and Jensen 2019), defined as unintentional fires that escape management, similar efforts for prescribed fire are lacking. As discussed by Hiers et al. (2020), differences between wildfire and prescribed burning, such as physical characteristics of the burn and the resulting effect on the landscape, imply unique monitoring needs. For example, in regions including the US West, Canada and Australia, prescribed burns tend to be smaller and shorter-duration burns than unmanaged wildfire (Knapp et al. 2009), leading to differences in remote sensing detectability (Wooster et al. 2021) and the temporal scale of post-fire recovery trajectories (Volkova et al. 2019). Often, prescribed fire results in lower burn severity – defined here as the loss of or change in aboveground and belowground organic matter, while recognising that individual studies use various metrics to measure severity (Keeley 2009). Prescribed burns may have multiple ignition points (Molina et al. 2022) and may be implemented when conditions are optimal for containment (Baijnath-Rodino et al. 2022), potentially leading to different duration, seasonality, spatial patterns and progression than wildfires (Boer et al. 2009). As a result of unique physical characteristics, prescribed burns and wildfire differ in their landscape-scale effects. Wildfires may result in severe ecological damage leading to type conversions (Syphard et al. 2019; Coop et al. 2020; Davis et al. 2020), loss of human life and impacts to health (Kochi et al. 2010) and damaged infrastructure (Mietkiewicz et al. 2020), whereas prescribed fires are intended to result in hazardous fuel reduction (Fernandes and Botelho 2003) and protection of endangered species habitat and biodiversity through refugia, corridors and variable stand ages on the landscape (Kennedy et al. 2008; Ryan et al. 2013; Meddens et al. 2018). Therefore, the assumption that remote sensing approaches for wildfire research are appropriate for prescribed burn research requires further investigation.
To address this gap, we ask: (1) which remote sensing methods and data streams have been applied in prescribed burn research? (2) What are the research aims of studies using remote sensing to analyse prescribed burns? (3) What do remote sensing studies conclude about the impacts of prescribed burning? (4) What do studies conclude about the effectiveness of remote sensing products and sensors for prescribed burn research? The objective of this paper is to highlight current knowledge, identify gaps and outline paths forward to improve understanding of prescribed burn impacts on the landscape as well as remote sensing methods to monitor multiple management goals. We find that remote sensing methods that are deemed fully operational for wildfire research have important limitations for prescribed burn research owing to reduced detection of small, short-duration and low-severity fires. We highlight that the ideal combination of measurement objectives for prescribed burn research may require a unique constellation of platforms to improve science and management.
Methods
Remote sensing context
There are several remote sensing instruments that provide publicly available and commercial data to observe fire and its environmental effects. Government-owned and freely available data can come from aircraft instruments, the International Space Station (ISS) or free-flying satellites. Aircraft or unmanned aerial systems (UAS) can host both active and passive instruments including Light Detection and Ranging (LiDAR), context cameras, imaging spectrometers such as AVIRIS (Airborne Visible/infrared Imaging Spectrometer) (Green et al. 1998; Chapman et al. 2019) and thermal radiometers including HyTES (Johnson et al. 2011) and MASTER (Hook et al. 2001). There are instruments hosted on the ISS such as NASA’s ECOSTRESS (Fisher et al. 2020) and EMIT (Green et al. 2020). ‘Free-flying’ spacecrafts and instruments include the US Geological Survey (USGS) Landsat Sustained Land Imagers (National Research Council 2013), NASA’s Aqua and Terra Moderate Resolution Imaging Spectroradiometers (MODIS; Barnes et al. 2003), NOAA’s Geostationary Operational Environmental Satellites (GOES; Davis 2007), and Visible Infrared Imaging Radiometer Suite (VIIRS; Murphy et al. 2006), and the European Space Agency’s Sentinel series of active and passive sensors (Showstack 2014).
Although the suite of these instruments, among many others, has been used to assess pre-, active and post-fire conditions, the utility of these instruments for prescribed fire research has been relatively less explored than their utility for wildfire research. Because each remote sensing mission has unique measurement objectives and operations, spanning a range of spatial resolutions, temporal revisit, sensitivity to pre- or post-fire conditions and mission duration, there is a need to better understand the specific capabilities and limitations of remote sensing for prescribed burn applications.
Review procedure
We compiled peer-reviewed journal articles using the following keyword search under ‘TOPIC’ within Web of Science (all databases) on 9 February 2022: (‘prescribed burn*’ OR ‘prescribed fire*’ OR ‘controlled burn*’ OR ‘cultural burn*’ OR ‘hazard reduction burn*’) AND (‘remote sensing’ OR ‘remotely sensed’). The search returned 268 studies published between 1993 and 2022 (Fig. 1). We acknowledge that the keywords may bias our results to English-speaking countries (Trisos et al. 2021), and that focusing on peer-reviewed literature omits relevant information from sources including Traditional Ecological Knowledge that is often not written (Shuman et al. 2022), and grey literature that is less accessible. Given our objective to evaluate research aims and outcomes specifically using remote sensing data, we chose to limit the scope of the review to peer-reviewed studies.
Flowchart of literature review procedure. Following keyword searches in Web of Science databases and a pre-screening process, a full screening and content analysis of 120 studies was conducted.
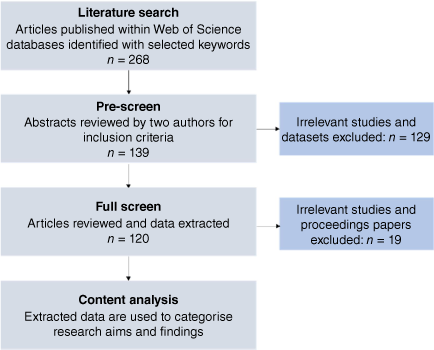
For this review, we define remote sensing broadly as a terrestrial (i.e. tower or tripod), airborne (i.e. aircraft or UAS), or spaceborne (i.e. free flying or on the ISS) platform that acquires information about an object without making physical contact. By applying the limited search query ‘remote sensing’ or ‘remotely sensed’, we constrained the review to studies that self-identified as using remote sensing approaches, avoiding the inclusion of specific search terms (e.g. Landsat, LiDAR) that could bias the results toward these methods. We included cultural burns in our search terms, a component of Indigenous fire management distinct from the Western tradition of prescribed burning (Adlam et al. 2022). Despite key differences in their history and practice, research has begun to investigate collaborations between Western land managers and Indigenous fire practitioners using cultural burning to reduce wildfire impacts (Lake et al. 2017; Marks-Block and Tripp 2021).
Two authors independently reviewed each abstract to determine whether the inclusion criteria were met: (1) the study utilised remote sensing, and (2) the remote sensing approach was applied to a prescribed burn. The authors agreed on inclusion criteria for 245 abstracts (intercoder reliability = 91.4%), and consensus was reached for the remaining 23 papers through discussion. The abstract review resulted in the inclusion of 139 studies, which were randomly assigned to one author for full review (Fig. 1). Based on the full reading, an additional 19 papers were excluded because they did not meet the criteria or were conference proceeding papers, which were excluded for consistency. Our review procedure resulted in full coding and analysis of 120 studies (see Data sources in the Supplementary material).
Data extraction
For each study, we extracted the following data for all remote sensing methods that contributed to the analysis of prescribed burning: study area (country, geographic location, land use type), remote sensing characteristics (data product, data type, spatial and spectral resolution, temporal revisit time), study aim(s) and study findings. Additionally, we extracted information on the prescribed burn feature analysed, which describes the characteristic of the prescribed fire about which the remote sensing platform acquired information (see Supplementary Table S1 for a full list of data extraction categories and their descriptions).
To address Research Question 1, we categorised and coded information to facilitate cross-study comparison. Remote sensing platforms were classified as terrestrial, airborne, or spaceborne, while sensors were categorised as active (providing their own energy source for illumination) or passive (measuring energy that is naturally available) (Horning 2008). The passive sensors were further categorised as recording reflectance in the visible (400–700 nm), near-infrared (NIR: 700–1400 nm), short-wave infrared (SWIR: 1400–3000 nm), mid-wave infrared (MIR: 3000–5000 nm), or thermal or long-wave infrared (TIR: 8000–14,000 nm) portions of the electromagnetic spectrum. Spatial resolution was categorised as very high (<0.5 m), high (0.5–2 m), moderate (2–29.9 m) and low (≥30 m) (Navulur 2006). Land-use types were determined based on two existing classifications: global biomes (Olson et al. 2001) and anthropogenic biomes (Ellis and Ramankutty 2008) to encompass both natural and human-dominated land use types respectively. The prescribed burn features analysed with remote sensing approaches were coded into five categories: active fire, burned area, vegetation characteristics, smoke and soil features (Table S2).
Content analysis
To address Research Questions 2–4, we conducted a content analysis of study research aims and findings only including those research aims that were addressed with the use of remote sensing. Whereas some studies utilised remote sensing to address research aims related to prescribed burning (e.g. using satellite imagery to evaluate prescribed fire severity), other studies had research aims related to the remote sensing methodologies themselves (e.g. comparing LiDAR data of post-fire vegetation structure with field-based observations). Given this diversity, we developed two subgroups to facilitate comparison of comparable content: ‘prescribed burn research aims’ and ‘remote sensing research aims’. Partitioning allowed for subgroup analysis, a meta-analytic methodology to characterise differences by dividing studies into characteristic groups (Mikolajewicz and Komarova 2019).
We applied an inductive analysis approach to the research findings in each subgroup, grouping qualitative data into a reduced number of emergent categories (Kyngäs 2020). For research aims evaluating the impact of prescribed burning on a management goal, the results were categorised as: ‘positive impact’, ‘neutral impact’ or ‘negative impact’ for the respective management goal (e.g. wildfire risk reduction). For research aims evaluating remote sensing performance, the results were categorised as: ‘effective, ‘limited effectiveness’, or ‘ineffective’. In each case, the categorisation was based on analysis of the direct language used in the studies to characterise their findings.
Results
Spatiotemporal trends
Peer-reviewed studies utilising remote sensing for the analysis of prescribed burns have substantially increased in number over the past three decades. Whereas the earliest study that met our inclusion criteria was published in 1993, half of the included studies were published in 2016 or later (Fig. 2). Published study sites span 21 countries (with one additional study conducted at an intercontinental scale of 32 countries) but are highly unevenly distributed (Fig. 3). The United States is the most represented country (n = 72), followed by Australia (n = 26) and Brazil (n = 4).
Annual publications using remote sensing for prescribed burn research. Total number of publications has significantly increased since the first identified study in 1993. More studies use passive remote sensing than active remote sensing or combinations of active and passive remote sensing. Note that only studies published prior to our search (9 February) are included for 2022.
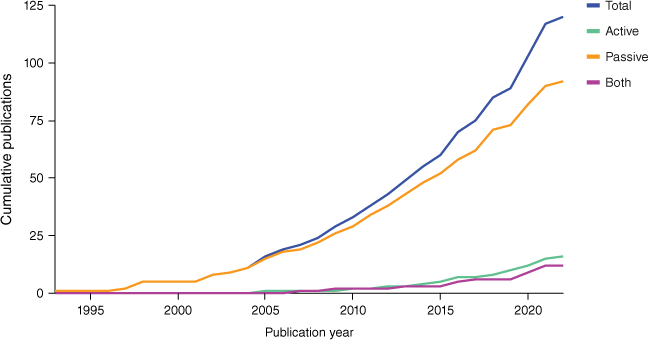
Map of the number of studies by country. Most included countries had coverage by a single study. The countries with the most coverage are the USA (n = 72), Australia (n = 26) and Brazil (n = 4). The total number of studies by country sums to more than the number of included studies as some studies span multiple countries.
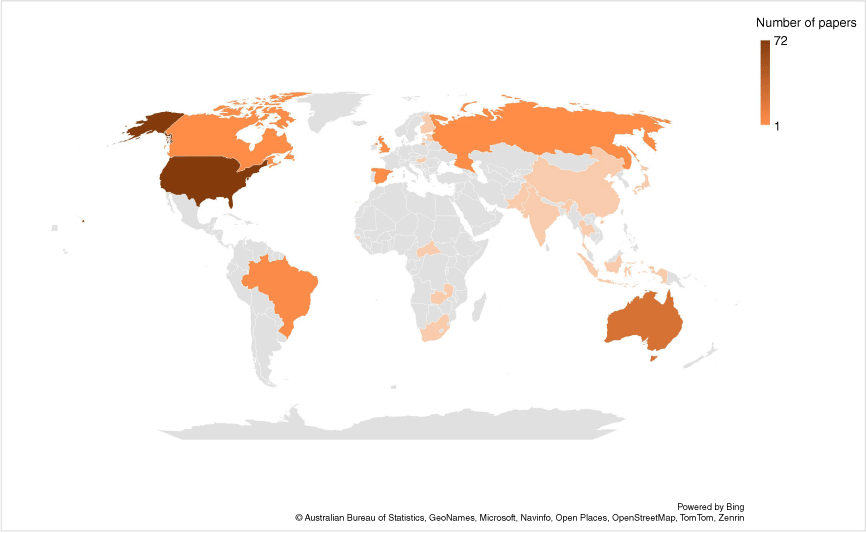
There was less representation of anthropogenic land use types (27.5% of studies) compared with natural land use types (94.2% of the studies). The anthropogenic biomes (‘anthromes’) represented were rangelands (25.0% of studies), croplands (14.2% of studies) and urban areas (0.8% of studies). The 120 studies analysed covered all natural terrestrial biomes except mangroves; however, nearly half (46.7%) covered temperate conifer forests. At a coarser spatial resolution, forests were the most represented land cover type: 74.2% of studies covered biomes that included forested land.
Remote sensing trends
Within the 120 studies, we identified 192 remote sensing methods, as 41 studies used multiple methods to analyse prescribed burns. Spaceborne platforms were the most common (n = 122), followed by airborne (n = 38) and terrestrial (n = 32) platforms. The most frequently used spaceborne platforms were Landsat (n = 44) and Aqua and Terra using the MODIS instrument (n = 30). The majority of sensors had a low spatial resolution of ≥30 m (n = 112), in comparison with numbers for high resolution in the range of 0.5–2 m (n = 54) or moderate resolution sensors between 2 and 29.9 m (n = 26).
Passive sensors (n = 159) were much more commonly used than active sensors (n = 33) for prescribed burn research applications. Passive remote sensing data were most frequently collected in the visible and NIR wavelengths. Data collected in the SWIR were also relatively common, but fewer studies utilised data collected in the MIR and TIR (Fig. 4). LiDAR was the most frequently used active remote sensing method (n = 24), followed by radio detection and ranging (radar) (n = 6) and sonic detection and ranging (sodar) (n = 3). LiDAR is a remote sensing method that uses a pulsed laser to measure distances, which can generate three-dimensional data on the height, shape and structure of surface characteristics including vegetation. Active remote sensors tended to be mounted on terrestrial (n = 16) or airborne platforms (n = 13). Spaceborne active remote sensors were much less common (n = 4) and included Sentinel-1 Synthetic Aperture Radar (SAR) and PALSAR-2.
Coverage and spatial resolution of passive remote sensing approaches in the literature. The reviewed studies utilised data from sensors with variable spatial resolutions and spectral coverages, resulting in different applications for prescribed burn (PB) research. Here, remote sensors are categorised based on their spatial resolutions (high, 0.5–2 m; moderate, 2–29.9 m; low, ≥30 m) and spectral coverage (visible, 400–700 nm; NIR, 700–1400 nm; SWIR, 1400–3000 nm; MWIR, 3000–5000 nm, thermal, 8000–14,000 nm), and primary applications for sensors at each resolution level are detailed. Grey bars and associated values represent the number of sensors in each category, with the most common outlined in black. Many sensors span multiple spectral regions, resulting in row totals greater than the number of low, medium and high-resolution sensors.
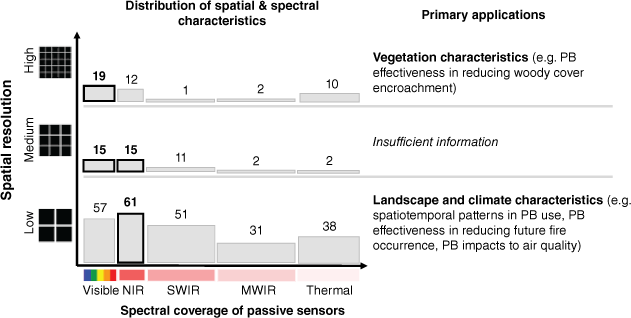
Remote sensing platforms and sensors have been used to evaluate many distinct features of prescribed burns, which we categorised into five feature classes: active fire, burned area, vegetation characteristics, smoke and soil features. Some remote sensing approaches were more commonly applied for observing particular feature classes, resulting in identifiable trends. For example, 57% of active remote sensing instruments were used to analyse vegetation characteristics including canopy height profiles and fuel loads, primarily driven by the frequent use of LiDAR for three-dimensional vegetation data. Although uncommonly utilised, all three instances of sodar identified in the literature were applied to the analysis of smoke characteristics such as plume height profiles and pyroconvection owing to the ability to measure wind and atmospheric turbulence (Charland and Clements 2013; Arreola Amaya and Clements 2020). Radar was most frequently used for burned area analyses, but also applied to smoke and vegetation features. Passive remote sensors were relatively evenly applied toward burned area features (n = 59), active fire features (n = 54) and vegetation features (n = 49), and much less commonly used for observing smoke and soil characteristics.
Depending on the research aims and prescribed burn feature of interest within each study, remote sensing can be used pre-burn, during burn, post-burn, or across the burn cycle. Of the 192 remote sensing applications used across studies, 70 were used during a burn. Unsurprisingly, these applications were primarily used to evaluate active fire and smoke features. A total of 64 sensors were used at multiple time steps across the burn cycle to compare pre- and post-fire conditions or to evaluate landscape change over time, from seasonal to decadal scales. Studies using a combination of pre- and post-burn data predominantly focused on vegetation and burned area features, often using remote sensing derived-indices. For example, the differenced Normalized Burn Ratio (dNBR) was commonly used as a metric of burn severity (e.g. Soverel et al. 2011; Arkle et al. 2012; Petrakis et al. 2018; Srivastava et al. 2020). Whereas the Normalized Burn Ratio (NBR) is defined as the normalised difference ratio in reflectance between the NIR and SWIR portions of the electromagnetic spectrum, dNBR is calculated from the difference between post-fire NBR and pre-fire NBR and therefore requires multiple remote sensing acquisition time steps. Although sensors were frequently used at multiple stages of the fire cycle, 47 sensors were used only post-burn and eight were used only pre-burn. The pre-burn remote sensing applications were exclusively used to evaluate vegetation features, such as canopy height profiles (Kiefer et al. 2014) or land cover and fuel classifications (Clark et al. 2009; Wiens et al. 2009; Miao et al. 2011; Loudermilk et al. 2014; Liu et al. 2021).
Study aims and findings: prescribed burning
Seventy-three studies included research aims related to prescribed burning. These studies used remote sensing to evaluate impacts on social-ecological management objectives (Impacts) or to improve understanding of prescribed burn patterns and processes (Understanding). Our inductive content analysis revealed 14 discrete research aims within the categories of Impacts and Understanding (Table 1).
Category | Prescribed burn research aim | Description | Studies citation number | Platform trends | Sensor trends (% passive) | |
---|---|---|---|---|---|---|
Impact | Wildfire risk reduction | Evaluates the impact of prescribed fire on fuel loads or subsequent wildfire behaviour (severity, intensity, extent) | 5, 12, 14, 17, 25, 27, 55, 70, 74, 75, 78, 87, 90, 92, 106, 108, 110, 111, 116 | ![]() | ![]() | |
Woody encroachment reduction | Evaluates the impact of prescribed fire on woody encroachment of grassland systems | 4, 12, 60, 79, 81, 85, 86, 99, 105 | ![]() | ![]() | ||
Vegetation | Evaluates the impact of prescribed fire on vegetation features including vegetation change and recovery | 12, 39, 48, 93, 100, 107, 112 | ![]() | ![]() | ||
Air quality | Evaluates the impact of prescribed fire on air quality from smoke/emissions | 9, 40, 73, 89, 118, 119 | ![]() | ![]() | ||
Wildlife habitat | Evaluates the impact of prescribed fire on fauna habitat quality or suitability for a particular species | 47, 65, 77, 78 | ![]() | ![]() | ||
Forage production | Evaluates the impact of prescribed fire on forage provisioning services such as pasture availability or carrying capacity | 54, 94 | ![]() | ![]() | ||
Invasive species management | Evaluates the impact of prescribed fire on invasive species management or restoration of invaded ecosystems | 54, 105 | ![]() | ![]() | ||
Watershed management | Evaluates the impact of prescribed fire on watershed condition or recharge | 31, 91 | ![]() | ![]() | ||
Carbon storage | Evaluates the impact of prescribed fire on carbon storage or fluxes | 59, 86 | ![]() | ![]() | ||
Soil | Evaluates the impact of prescribed fire on soil properties or patterns | 85, 86 | ![]() | ![]() | ||
Understanding | Spatiotemporal patterns | Aims to understand where and/or when prescribed fire occurs on the landscape | 1, 11, 12, 20, 50, 51, 68, 69, 76, 102, 103 | ![]() | ![]() | |
Smoke plumes | Aims to understand prescribed fire smoke plume characteristics, dynamics, or structure | 7, 8, 16, 18, 42, 43, 58, 114, 115 | ![]() | ![]() | ||
Fire dynamics | Aims to understand prescribed fire behaviour or progression, such as rate and direction of spread | 7, 15, 22, 57, 115 | ![]() | ![]() | ||
Fuel dynamics | Aims to understand prescribed fire fuel consumption patterns or combustion properties | 36, 37, 53, 88, 93 | ![]() | ![]() |
Figure includes remote sensing trends for each research aim, including platform trends (blue, spaceborne; orange, airborne; grey, terrestrial) and sensor trends (% of sensors that are passive). The remote sensing trends include all sensors used for the research aim, which may sum to a total greater than the number of studies in cases where studies used multiple remote sensors. The citation numbers refer to the Data Sources in the supplementary material, which lists the 120 studies included in the review analysis.
Effectiveness of prescribed burning for management goals
Forty-seven studies evaluated the impact of prescribed burning on environmental management goals. Of these, the most frequently assessed goal was wildfire risk reduction (n = 19). All studies in this category found prescribed burning reduced wildfire risk, with the most common finding being reduced burn severity of subsequent wildfires. Primarily using passive, spaceborne platforms such as Landsat and indices such as the NBR or dNBR to evaluate burn characteristics, these studies found that prescribed burning reduced the incidence of late-season wildfires (Price et al. 2012), reduced post-wildfire changes in vegetation (Petrakis et al. 2018), and reduced the extent and severity of subsequent wildfires (Arkle et al. 2012; Radford et al. 2020; Cansler et al. 2022). Studies evaluating fuels reduction used active remote sensing approaches such as LiDAR to assess vegetation characteristics (e.g. vertical structure of ladder fuels at multiple fuel heights). These studies found that prescribed burning increases fuel load fragmentation (Franke et al. 2018) and reduces fine fuel loads (Wells et al. 2021), fuel height (Skowronski et al. 2007) and ladder fuels (Clark et al. 2009).
Whereas remote sensing methods found prescribed burning effective for wildfire risk reduction, results differed for air quality impacts. Six studies evaluated air quality using passive, low-resolution, spaceborne approaches during active burns, with all but one finding negative air quality impacts from prescribed burning. However, Schweizer et al. (2019) identified prescribed burning as having a net positive impact on air quality, when considering the mitigated exposure resulting from avoided wildfire emissions. Active fire characteristics (e.g. hotspot detections) or smoke characteristics (e.g. smoke density, smoke-influenced days) were used to compute pollutant emissions or exposure levels, often in combination with air quality models.
For the remaining management goals, impacts of prescribed burning were inconsistent across studies. Seven studies found prescribed burning effective in reducing woody encroachment, whereas one study found the practice partially effective – slowing the increase of woody encroachment, but not reducing it (Ansley et al. 2010) – and one found it ineffective (Bucini and Lambin 2002). Impacts of prescribed fire on vegetation were highly variable and dependent on ecosystem type, burn severity and seasonality. Similarly, the impacts on habitat quality depended on the study’s focal species. Few studies used remote sensing to investigate the effectiveness of prescribed burning on invasive species management (n = 2), forage production (n = 2), water management (n = 2), carbon sequestration and storage (n = 2), or soil (n = 2).
Understanding prescribed burning
The second category of research aims addressed gaps in understanding prescribed burn physical patterns or processes. Understanding prescribed burn spatiotemporal patterns was the most common aim in this category (n = 11), with studies applying remote sensing methods to determine where and when prescribed burns occur on the landscape. For example, studies described the occurrence of prescribed burns at different times of year (Tian et al. 2013), or in relation to human settlement patterns (Bucini and Lambin 2002; Temudo et al. 2020). To do so, 50% of the remote sensors within this research aim were used to detect active fire features during burns to quantify prescribed fire counts or area. The evaluation of prescribed burn spatiotemporal patterns was primarily conducted over large geographic scales (i.e. international, national, or regional scales) using passive low-resolution spaceborne sensors (but see Allen et al. 2016).
Study aims and findings: remote sensing
While some studies utilised remote sensing to address research questions about prescribed burning as detailed in the previous section, 82 studies contained research aims related to the remote sensing methods themselves (Table 2). As shown in Table 2, the vast majority evaluated the performance of the remote sensing methodology for its intended prescribed fire application (Performance evaluation), with the most common aim being evaluation of a product or sensor. To explore the effectiveness of remote sensing products or sensors for prescribed fire research, we focus on this research aim for the remaining analysis. However, some studies evaluated the performance of other aspects of remote sensing methodologies including indices (n = 15) such as the NBR and Normalized Difference Vegetation Index (NDVI). The same number of studies (n = 15) evaluated the performance of a remote sensing algorithm or predictive model, including image classification techniques. Few studies addressed other topics including using remote sensing data to validate a model (validation), describing a remote sensing approach (descriptive), or assessing the usability of remote sensing for stakeholders or land managers (usability).
Category | Remote sensing research aim | Description | Studies citation number | |
---|---|---|---|---|
Performance evaluation | Remote sensing product/sensor | Evaluates whether a remote sensing product or sensor performed positively for its intended purpose | 3, 8, 10, 11, 21, 23, 26, 27, 30, 31, 33, 35, 36, 37, 40, 41, 49, 52, 54, 55, 57, 58, 61, 63, 66, 67, 68, 71, 72, 73, 80, 82, 83, 84, 86, 88, 90, 92, 95, 98, 102, 103, 108, 109, 111, 118, 119, 120 | |
Remote sensing index | Evaluates whether a remote sensing index (e.g. NBR, NDVI) performed positively for its intended purpose | 6, 19, 28, 34, 48, 62, 64, 71, 96, 97, 98, 100, 101, 105, 107 | ||
Remote sensing algorithm or predictive model | Evaluates whether a remote sensing algorithm or predictive model performed positively for its intended purpose | 15, 16, 30, 38, 44, 45, 46, 53, 56, 61, 83, 91, 96, 104, 107 | ||
Remote sensing platform | Evaluates whether a remote sensing platform (the structure or vehicle on which the instrument is mounted) performed positively for its intended purpose | 21, 117 | ||
Model using remote sensing inputs | Evaluates whether a model that uses remote sensing data as an input performed positively for its intended purpose | 43 | ||
Validation | Model validation | Uses remote sensing as observational data to compare or validate model outputs | 18, 24 | |
Descriptive | Remote sensing approach | Describes a remote sensing approach, such as the history, context, or applications of the remote sensor | 76, 81 | |
Spectral features | Describes spectral features or identifies changes in the electromagnetic radiation (EMR) related to prescribed burning | 32 | ||
Usability | Stakeholder usability | Evaluates the usability or utility of a remote sensing approach for stakeholders including natural resource or emergency service managers | 2, 13, 29, 113 |
The citation numbers refer to the Data Sources supplementary material, which lists the 120 studies included in the review analysis.
Performance evaluation of remote sensing products and sensors
The most common research aim we identified in the literature was the performance evaluation of a remote sensing product or sensor. The 48 studies in this category included 78 products/sensors. However, performance findings were unavailable for five sensors, resulting in 73 analysed sensors (12 active, 61 passive). In the results that follow, effective performance indicates the remote sensing approach was deemed fit for use for its intended purpose. Limited effectiveness indicates the study identified benefits and limitations, while ineffective performance indicates that the study determined the remote sensing method was not fully operational for its intended purpose owing to significant limitations.
Approximately 40% of the evaluated products or sensors were identified as effective for their prescribed burn research uses (n = 29). Notably, 83.3% of active sensors were deemed effective, compared with only 31.1% of passive sensors (Fig. 5). Of the active remote sensors, LiDAR was effective for identifying fire-induced forest understorey change (Gupta et al. 2015), producing spatially explicit high-resolution fuel bed height measurements (Rowell et al. 2015) and estimating fuel consumption (Hudak et al. 2020). A key advantage of LiDAR in comparison with passive remote sensing approaches was the ability to map three-dimensional vegetation structure. Radar was found, in multiple instances, to perform positively for smoke plume analysis (McCarthy et al. 2018; Aydell and Clements 2021).
Remote sensing performance for products and sensors. All remote sensors identified in the ‘Performance evaluation of a remote sensing product or sensor’ research aim were evaluated to determine whether performance was effective, limited, or ineffective for their intended prescribed fire application. Performance outcomes are shown for multiple aspects of the remote sensor including: which prescribed burn feature was being analysed (prescribed burn features), the spatial resolution category of the sensor (spatial resolution), whether the sensor is active or passive (sensor) and whether the sensor is mounted on a spaceborne, airborne, or terrestrial platform (platform).
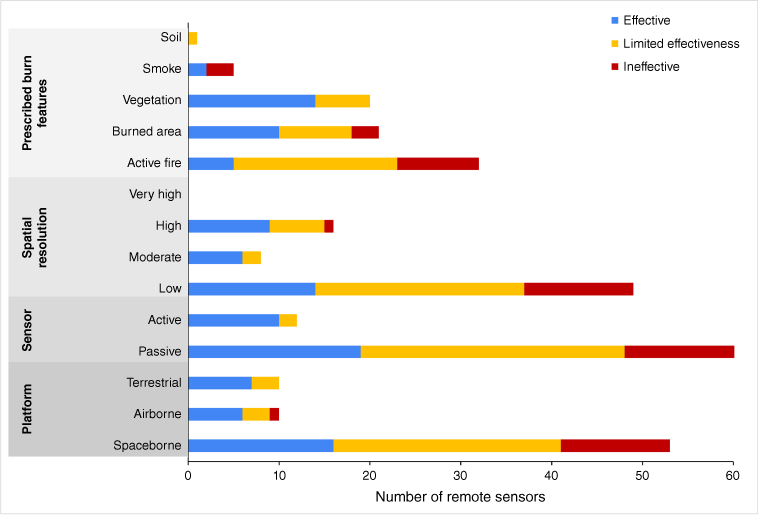
A variety of low-resolution, passive spaceborne sensors also performed effectively for their prescribed burn applications. Landsat was able to differentiate between wildfire and prescribed fire disturbance with 80% accuracy (Nguyen et al. 2018), contribute to useful fuel maps for management (Franke et al. 2018) and detect vegetation cover changes resulting from prescribed burns (Goodrich et al. 2020). However, Landsat (along with MODIS) is a near-polar orbiting satellite, and thus provides more limited temporal coverage. Geostationary satellites, such as GOES, provide continuous coverage of a specific area over time and thus may be advantageous for prescribed fire monitoring. Indeed, in one study comparing GOES and MODIS, GOES outperformed MODIS for detection of small fires (Soja et al. 2009). Some spaceborne sensors (e.g. Sentinel-2A) performed positively in combination with additional data sources such as in situ fuel load data (Wells et al. 2021), or to supplement bottom–up data sources such as prescribed burn inventories (Zeng et al. 2016).
Approximately 42% of sensors had limited effectiveness for their prescribed fire applications (n = 31); 16.7% of active sensors analysed were identified in this category, compared with 41.4% of passive sensors. This group is predominantly characterised by low-resolution, spaceborne, passive sensors (n = 23), which performed well for some aspects of the study research aims (acceptable accuracy and large spatial coverage) but also faced important constraints in their utility for prescribed burn research (omission errors for small or low-severity burns). Passive satellite remote sensing can provide coverage over large spatial scales and in data-scarce areas in which in situ data are particularly challenging to collect. However, limitations accompanying large spatial coverage include potential trade-offs with spatial detail. For example, Rauste et al. (1997) discuss that despite the coverage advantages of spaceborne remote sensing approaches for fire applications, they cannot entirely replace the function or benefit of aerial monitoring. Satellite instruments in this category tended to perform well for capturing a large proportion of fires (Soja et al. 2009) but were specifically limited in their detection of smaller and less severe fires. Although studies tended to reflect positively on the overall levels of accuracy in satellite-based fire detection, many noted that omission errors for small low-severity burns primarily impact detection of prescribed fire rather than wildland fire (e.g. Pouliot et al. 2008). Despite important aspects of positive performance noted across studies, the limited ability of such sensors to capture smaller, lower-severity fire disproportionately impacts their utility for prescribed burn detection and research applications.
Relatively few products or sensors were found ineffective for their intended applications (n = 13). Of the passive sensors analysed, 25.9% were found ineffective, while no active sensors were identified in this category. Common reasons for poor performance included those similar to the limitations noted above. However, their placement in the ‘ineffective’ category rather than the ‘limited effectiveness’ category reflects that the limitation is more severe or more fundamental to the aim of the study, or the remote sensing approach lacks a benefit that outweighs or justifies the limitation. In such cases, the remote sensing instrument was deemed ineffective for its intended use. Significant underestimations of burned area, unacceptably high errors of omission or commission of active fire, and lack of calibration with ground data were common shortcomings. For example, one study by Huang et al. (2018) compared two remote sensing-derived products (Blended Polar Geo Biomass Burning Emissions Product and Global Fire Emissions Database) with prescribed burn permit data, finding that both products significantly underestimated prescribed burns. Their study specifies two common underlying causes of inaccurate fire detections that apply to many other studies: mismatches between the small size of prescribed burns and the spatial resolution of the sensor, and mismatches between the short duration of prescribed burns and the temporal resolution (frequency of overpass time) of the sensor. Most sensors in this category (n = 9) were used to analyse active fire features (i.e. fire radiative power, fire radiative energy), suggesting gaps remain in prescribed burn hotspot detection.
Discussion
Informing prescribed fire management with remote sensing
Interest in using and evaluating remote sensing for prescribed burn research has substantially increased in recent years, and applications continue to expand. Most studies have used passive, low-resolution, spaceborne remote sensing, with a smaller number of studies applying active or high-resolution sensors in recent years. Studies relying on remotely sensed data found prescribed burning effective for several management goals, at least in the limited regions and land cover types where studies were conducted, such as forests in the USA and Australia. The geographic skew may be a result of reviewing studies published in the English language. However, the USA (McWethy et al. 2019) and Australia (Johnston et al. 2021) have faced record-breaking fire seasons in the past several years that may result in relatively higher interest in prescribed burn research in these locations. Additionally, the overrepresentation of forest ecosystems in the literature may be due to prioritisation of mitigating severe wildfire in forested lands, or difficulties characterising vegetation with remote sensing in grasslands or other short-statured vegetation communities (Franke et al. 2018).
The most common prescribed burn research aims were (1) evaluating the effectiveness of prescribed fire for wildfire risk reduction, and (2) understanding spatiotemporal patterns of prescribed fire. These findings reflect current priorities within prescribed fire research and management and highlight existing gaps in the ability to effectively monitor prescribed fire. The focus in the literature on wildfire risk reduction aligns with recent policy and planning efforts in fire-adapted landscapes such as the Western US. For example, the recently released USDA Forest Service Wildfire Crisis Strategy aims for an additional 20 million acres (80937 square kilometers) of fuel reduction treatments on National Forest lands in the US West to achieve wildfire risk reduction goals (USDA 2022). The findings of studies included in this review highlight that remote sensing methods contribute to the growing body of evidence on the effectiveness of prescribed fire in reducing and altering subsequent wildfire risk. All studies that evaluated prescribed burn effectiveness for wildfire risk reduction found the treatment to be effective, which aligns with results from studies utilising in situ data (e.g. Fernandes 2015; Prichard et al. 2010; Weston et al. 2022). However, the fact that the second most common research aim is understanding spatiotemporal patterns points to a continued interest and need for accurate, consistent remote sensing methods for prescribed burn detection across large spatial scales. Accurate and timely detection of prescribed fire is a critical prerequisite for monitoring treatment effectiveness and impacts at the landscape scale, including for wildfire risk reduction goals.
Although many studies used remote sensing to evaluate the effectiveness of prescribed burning for management goals, very few (n = 6) considered prescribed burning impacts on multiple management goals, resulting in a lack of information on potential co-benefits and trade-offs between priorities. Prescribed fires can affect various management objectives, including air quality, carbon storage and habitat for wildlife, among others. In the US, these objectives have legal implications for compliance with the Endangered Species Act and air quality levels set by regional regulatory bodies (Schultz et al. 2019; Stephens et al. 2019). The potential for trade-offs and co-benefits between different management objectives highlights the importance of developing robust methods for assessing the socio-environmental impacts of prescribed burning. Within this review, we identified studies using remote sensing methods to evaluate a wide range of management goals including: wildfire risk reduction, forage production, invasive species management, watershed management, woody encroachment reduction, air quality, habitat quality, carbon, soil and vegetation management. Given the diverse applications of remote sensing for prescribed burn research demonstrated in existing studies, using remote sensing to evaluate multiple management goals is possible, albeit currently underutilised. In addition to limited coverage of multiple impacts, some individual impacts were infrequently assessed in the literature. Studies using remote sensing to evaluate prescribed fire impacts on invasive species management, forage production, watershed management, soil and carbon were particularly limited. Gaps may be due to the relative difficulty of remotely sensing these impacts. For example, challenges in accurately quantifying biomass may affect the number of studies remotely sensing impacts of prescribed fire on carbon stocks. However, recent remote sensing advances such as spaceborne LiDAR may aid future efforts to evaluate prescribed fire impacts on carbon (Leite et al. 2022) and other understudied management priorities.
When using remote sensing to evaluate the effectiveness of prescribed burning, selecting appropriate counterfactuals is critical. Most studies compared the impacts of prescribed burning with a counterfactual of ‘no fire’ (for example, comparing wildlife habitat quality within a prescribed fire with habitat quality in an unburned area). However, the assumed lack of wildfire may be an inaccurate counterfactual in locations likely to experience frequent and/or severe wildfires. In such cases, explicitly accounting for the probability of severe fire – as well as the use of prescribed burning to reduce subsequent wildfire severity – may be necessary for estimating net impacts. For example, only one study assessing air quality impacts compared emissions from a prescribed burn with a high-intensity wildfire, concluding that prescribed burning mitigates net smoke exposure (Schweizer et al. 2019). The latter study highlights that although prescribed burns are not without risks or trade-offs, alternative scenarios such as unmitigated wildfire also present risks that must be evaluated. There may be trade-offs in short-term impacts (e.g. reduced air quality, carbon emissions) from prescribed fire that need to be weighed against long-term impacts when more severe fires occur with greater fuel buildup (Cary et al. 2017). For example, in fire-adapted systems, forests can re-sequester carbon within 7 years following low-severity fire expected from prescribed burning, whereas carbon re-sequestration can take over a century after a stand-replacing fire (Kashian et al. 2006). Remote sensing of multiple metrics and for multiple management goals can aid in the integration of public health and climate mitigation considerations into prescribed fire research and management.
Informing remote sensing mission requirements for prescribed fire
The most common research aim among studies in the review was the performance evaluation of a remote sensing product or sensor, reflecting ongoing interest and effort in developing, evaluating and applying remote sensing methods to the analysis of prescribed fire. Although remote sensing approaches have been used in fire research for decades, applications tailored to prescribed burns are still in a stage of refinement and validation.
We find that remote sensing methods typically applied to wildfire detection and analysis are also frequently applied to prescribed burns. The most common remote sensing data source identified in the literature is Landsat, which provides the longest continuous record of space-based satellite imagery (Wulder et al. 2019). The long-term temporal continuity, which facilitates consistent analyses pre- and post-fire, may partially explain its widespread use within the literature. Using Landsat, Aqua/Terra MODIS, GOES and other optical and thermal satellite imagery has the advantage of spatial coverage, public access and consistent availability relative to airborne and terrestrial remote sensing, which contributes to their utility for research and management. In addition to the convenience and accessibility of these methods, a number of studies found satellite data can detect prescribed burns at an accuracy level appropriate for management. However, what constitutes an appropriate level of accuracy likely depends on management goals, risk tolerance, technical capabilities and institutional capacity to act on available data.
Whereas some studies found that commonly used passive spaceborne remote sensors were effective for analysing prescribed burns, others identified limitations as illustrated in Fig. 5. A constraint of using low spatial resolution satellite data for fire detection and impact evaluation is high errors of omission for smaller, shorter-duration and less severe fires, which disproportionately impacts prescribed burn detection and monitoring. We highlight this commonly identified constraint in Fig. 6, which demonstrates the use of Landsat 8 OLI imagery for burn severity analysis based on dNBR (Parks et al. 2018). The three treatments (broadcast burns between 100 and 200 acres, or 0.4 and 0.8 square kilometers,in Colorado, USA) show a range of detected severities from entirely unburned (Row 1) to moderate–high severity fire (Row 3). Although a systematic analysis would be needed to determine the proportion of prescribed fires detected and undetected by dNBR, Fig. 6 provides visual examples of both and, therefore, demonstrates the mixed levels of effectiveness found in the reviewed literature.
Example of differenced Normalized Burn Ratio for fire severity assessment: the following figure demonstrates the use of 30-m Landsat 8 OLI to calculate the differenced Normalized Burn Ratio (dNBR) for three prescribed burns and one wildfire in Colorado, USA. dNBR was calculated using Google Earth Engine code from Parks et al. (2018). The burn perimeters for each fire are outlined in white. Rows 1–3 depict examples of prescribed burns of average size for the state of Colorado (100–200 acres) and the same treatment type (broadcast burning) with varying levels of detection. Row 4 comparatively depicts dNBR for a wildfire. All dNBR values are scaled by 103 and reclassified based on a proposed severity classification from the US Geological Survey. All four burns occurred in forested land cover classes, visualised by the pre-fire false colour composite vegetation imagery obtained from Landsat. Impacts to vegetation from each burn can be seen in the post-fire false colour composite vegetation imagery, with denser vegetation cover represented as red and less dense vegetation cover represented as green. In Row 1, the prescribed burn (112 acres, completed 28 October 2021) was undetected based on the dNBR classification, and resulted in little to no detectable change in vegetation. In Row 2, the prescribed burn (104 acres, completed 13 September 2014) was partially detected as a mix of unburned and low-severity pixels, with slight vegetation change. In Row 3, the prescribed burn (159 acres, completed 19 August 2019) was well detected with a mix of unburned, low and moderate severity pixels and resulted in visually reduced density of vegetation. In Row 4, the East Canyon Fire (2905 acres, contained 27 June 2020) burned a relatively larger area at higher severity that the prescribed fires, resulting in clear reduction of vegetation density within the wildfire boundary. The three prescribed burns shown here provide examples of the mixed effectiveness of dNBR for prescribed fire detection and burned area assessment, compared with larger and more severe wildfire.
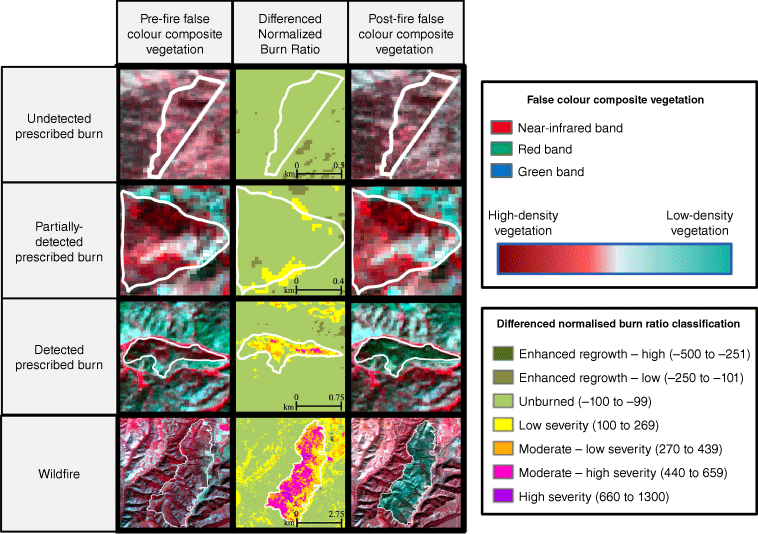
Additionally, Fig. 6 highlights the utility of supplementary information to contextualise prescribed fire effects on the landscape; for instance, we show pre-and post-fire false-colour composite Landsat imagery of vegetation to visualise the vegetation density reduction resulting from each burn. As can be seen in Row 1, the prescribed burn classified as unburned had negligible impacts on overstorey vegetation, whereas the wildfire in Row 4 resulted in a visible reduction of vegetation density within the wildfire boundary. Further, the burn size, duration and severity may interact with other environmental and management-related characteristics, such as seasonality, cloud cover, vegetation type, canopy cover, or treatment heterogeneity. Therefore, additional remote sensing information such as pre- and post-fire vegetation or in situ data such as burn implementation method may inform remotely sensed burn severity analysis. Remote sensing approaches that extend beyond the applications demonstrated in Fig. 6 are needed to better account for the unique characteristics of prescribed fire. For example, considering specific fire effects metrics such as understorey biomass loss using multi-temporal terrestrial laser scanning (TLS) may supplement spectral indices derived from satellite imagery. Although spatial coverage of TLS data remains limited, efforts such as the Interagency LiDAR Monitoring and Research Applications (IntELiMon) program in the US aim to scale up automated processing and analysis. Existing methods from wildfire research that aim to advance burn severity analyses and impact evaluation by combining spectral indices with active remote sensing data (e.g. Wulder et al. 2009; McCarley et al. 2017; Fernández-Guisuraga et al. 2022) could be evaluated for their utility in prescribed fire contexts.
Although all studies included in this review remotely sensed prescribed burns, some research aims were broadly interested in detecting or analysing multiple types of fire on the landscape, including wildfire. In such cases, studies may have found the use of spaceborne, low-resolution, passive remote sensing to detect fire with an acceptable level of accuracy when considering all fire types. The limited ability to detect prescribed fire may not be of particular concern in cases where wildfire detection is the primary aim. However, for prescribed burn research specifically, the results reveal key trade-offs between remote sensors with large spatial or temporal coverage (typically, passive spaceborne sensors) and those with high spatial resolution (typically, active airborne/terrestrial sensors).
Although we identified fewer instances of active remote sensing in the literature, active approaches show promise for enhancing prescribed burn research. Over 80% of analysed active sensors were found to perform positively, with LiDAR especially noted for the ability to map three-dimensional vegetation features pre- and post-fire. Active remote sensing data were generally described as highly accurate and spatially explicit. In some cases, LiDAR was found more effective than in situ measurements owing to the ability to better capture landscape heterogeneity (Shrestha et al. 2021) and non-destructively estimate fuel consumption (Hudak et al. 2020).
Additionally, airborne remote sensing platforms can provide high spatial detail and have multi-sensor capacity that may be advantageous for prescribed fire applications. Of the 38 airborne applications identified in our review, 28 (74%) had high-resolution data, 25 (66%) carried passive sensors and 13 (34%) carried active sensors. Although fewer airborne remote sensing applications (n = 10) were evaluated for their performance than spaceborne sensors (n = 53), 60% performed positively. In one study evaluating the effectiveness of airborne remote sensing approaches, UAS platforms carrying passive sensors were evaluated as having limited performance but were found to be more effective when their data were combined with active sensors to create multispectral–LiDAR and hyperspectral–LiDAR fusion products (Sankey et al. 2021). The fusion of multiple forms of data, including active–passive and terrestrial–airborne, presents unique opportunities to better align remote sensing data with prescribed burn research aims.
However, there are also barriers to the application of current active remote sensing approaches for prescribed fire research, despite effective performances. The spatial and temporal coverage of studies using active sensors tended to be more limited than studies using passive spaceborne imagery. Spaceborne active remote sensing (e.g. Sentinel, PALSAR-2) was uncommon in the literature compared with terrestrial and airborne sensors. Although sodar and radar tended to perform effectively in the literature, the low number of studies using these methods limits generalisations about their utility. Additionally, studies often did not address the high resource intensiveness or financial cost associated with the use of active remote sensing methods, which may present barriers to research and management communities. Although such barriers often apply to remote sensing generally, the accessibility and familiarity of more common passive platforms like Landsat – and their integration with cloud-based computing platforms such as Google Earth Engine (Gorelick et al. 2017) – may offer advantages in terms of the relative ease of conducting research. Expanding the applications of active remote sensing for prescribed burn research has the potential to complement the use of passive imagery by providing increased accuracy (e.g. higher spatial resolution) and detail (e.g. vegetation structure) (Stavros et al. 2018).
Most sensors deemed effective for their prescribed burn applications analysed vegetation features (e.g. land cover, fuel height) while a majority of ineffective sensors analysed active fire features (e.g. fire radiative power). This suggests that remote sensing applications for vegetation monitoring are more well established compared with applications particular to prescribed burn monitoring and detection. Active remote sensing methods for vegetation analysis appear transferable to prescribed burn research, whereas tailored fire applications may require additional development to increase accuracy. The emitted spectral radiance from flaming fires peaks in the MIR spectral region, making it advantageous for hotspot detection (Leblon et al. 2016). Given the relatively low number of sensors that performed positively for active fire features in this review, expanding the use of MIR sensors may advance analysis of active fire features in prescribed burn research. Additionally, active remote sensing tends to be applied to different prescribed burn features and research questions than passive remote sensing. Most research aims that addressed the effectiveness of prescribed burning for fuels reduction goals used LiDAR to evaluate post-fire vegetation. For features such as fuel heights and fine fuel levels, high-resolution airborne or terrestrial remote sensing appear to be best suited for analyses. Active remote sensing was also commonly applied for analyses of smoke features such as plume dynamics or height. In contrast, passive remote sensing tended to be used to analyse burned area features such as burn severity, or active fire features such as hotspot detection, over large geographic areas.
Comparing wildfire and prescribed fire remote sensing needs
Trade-offs between the strengths and weaknesses of remote sensing methods reveal important distinctions between remote sensing applications that are optimised for wildfire and those that are optimised for prescribed fire. Within the last decade, multiple reviews have been published evaluating the use of remote sensing for fire research. Comparing findings from these reviews with results from our analyses highlights when existing wildfire remote sensing approaches may be appropriate for prescribed burn research, and where prescribed burn research may require distinct approaches. Leblon et al. (2016) in their review of wildfire remote sensing identified MODIS as ‘the standard sensor for active fire monitoring’ owing to its spatial resolution, fire detection capacity and global spatial coverage. Additionally, they found that the frequent revisit time of MODIS burned area products compensated for its lower spatial resolution compared with other sensors. However, our review reveals that in the case of prescribed burn detection, the choice between high temporal revisit time and high spatial resolution is less obvious. Because detection of small burns is critical to prescribed fire monitoring, the importance of high spatial resolution is outsized for prescribed fire research. Leblon et al. (2016) and Szpakowski and Jensen (2019) both highlight MODIS limitations for small fire detection. Therefore, although MODIS may be the standard for wildfire monitoring, it is not necessarily optimised for prescribed fire. Ultimately, Leblon et al. (2016) conclude that fire detection with remote sensing can be considered fully operational. Although many sensors evaluated in this review were effective for their prescribed burn applications, 60% were found ineffective or limited in effectiveness, with limitations predominantly related to passive remote sensing of active fire features. Therefore, gaps remain in remote sensing for prescribed fire detection, despite the operationality of remote sensing for fire detection more broadly.
However, there are also trends in wildfire remote sensing that mirror or align with prescribed burn research needs. Szpakowski and Jensen (2019), reviewing applications of remote sensing for fire ecology, discuss the rise in the use of LiDAR over the past few decades. In alignment with our findings, they highlight the advantages of LiDAR for analysing vegetation structural properties and for supplementing other remote sensing data sources such as multispectral imagery. Although the use of active remote sensing such as LiDAR is beneficial for fire research generally, it presents particular opportunities for advancing prescribed fire research. In social-ecological contexts where prescribed fire occurs as a low-severity surface fire under a forest canopy, fire detection and burn analyses can be difficult or infeasible using common passive remote sensing methods. From the perspective of a passive airborne or spaceborne sensor, the canopy may mask understorey impacts of prescribed fire. For example, Franke et al. (2018) specify that fuel maps of the Brazilian cerrado based on Sentinel-2 and Landsat data have greater uncertainties in locations with higher canopy cover compared with open savanna. Therefore, the use of LiDAR may have a immense benefit within prescribed fire research.
Our review reveals that despite differences between wildfire and prescribed fire research needs, similar remote sensing approaches tend to be used. Methods that are optimised for large fires such as MODIS, Landsat and other passive spaceborne sensors are frequently used in prescribed burn research. Although the benefits of active remote sensing such as LiDAR are recognised, these are less frequently utilised but their use is expanding quickly.
Future directions for prescribed fire research
Low spatial resolution passive spaceborne remote sensing approaches are the most frequently used methods for prescribed burn research to date and will continue to play an important role owing to their utility for analyses at large spatiotemporal scales. However, the decommissioning of NASA’s Aqua and Terra missions, after 20 years despite an initial 6‐year mission, will require users to shift to data from other missions, such as the NASA Preparatory Project (NPP) mission. The NPP mission has similar spectral bands and spatial resolution, but has only an afternoon overpass time, similarly to Aqua, and thus leaves a gap in morning/ante meridiem fire detection, or in atmospheric conditions when cloud cover is lower. A paucity of MIR (3000–5000 nm) studies exists in the literature, despite its importance in detecting active fire (Cawse-Nicholson et al. 2021). Future missions such as NASA’s Surface Biology and Geology mission will have thermal imager instrumentation to better retrieve information in this wavelength (Stavros et al. 2022). Although VIIRS is commonly used for wildfire detection, and VIIRS 375-m data have been found to have active fire detection sensitivity approximately 10 times that of MODIS (Wooster et al. 2021), it was infrequently used in studies included in the present review (n = 2). Both studies using VIIRS discussed limitations related to cloud obscuration, a common constraint for satellite sensors (Dickinson et al. 2015; Li et al. 2020). This constraint is compounded by the fairly short duration of prescribed fires, which limits the window of time available to obtain a cloud-free image of the active burn. Therefore, additional research on the applications of VIIRS for prescribed burn detection is needed.
Active and high-resolution remote sensing approaches show promise for prescribed burn research. Commercial imagery (i.e. Planet, Maxar) and new constellations or harmonisation of public-space missions (i.e. Landsat-Next, Sentinel Next Generation) will help advance observations for high spatial resolution and more frequent revisit times. As active remote sensing data from the Global Ecosystem Dynamics Investigation (GEDI), the Ice, Cloud, and Land Elevation Satellite-2 (ICESat-2), the Multi-angle Imaging SpectroRadiometer (MISR) and other radars (e.g. Sentinel-1, BIOMASS, NISAR) continue to expand, so too will the ability to monitor and evaluate a wider variety of prescribed burn management goals and impacts. For example, the GEDI joint mission between NASA and the University of Maryland launched in 2018 provides three-dimensional forest data derived from LiDAR observations collected globally between 51.6°N and 51.6°S latitudes. This information on forest height and structure can contribute to the estimation of carbon impacts from wildfire and prescribed fire, as well as pre-fire fuel loads. Determining when existing remote sensing products, tools, or approaches designed for wildfire research can be effectively applied to prescribed burn research – and when they cannot – will be critical to building an improved knowledge base for prescribed fire science. Our review shows that high spatial resolution, with combined spectral bands in the visible, mid-wave infrared and active LiDAR, provide synergistic applications for prescribed fire remote sensing. Emerging satellite missions from both public and commercial sectors, and international partnerships providing harmonised products, will provide to some extent the high-spatial resolution, spectral band placement and revisit requirements for prescribed fire research. However, observation gaps remain in future LiDAR missions, with GEDI now offline until resuming sometime in 2024 and the need for sub-daily observations of active fire at high to moderate resolution not currently met. The affordability and accessibility of emergent remote sensing approaches will play an important role in their integration into prescribed burning management and research.
Prescribed burning is an important management action and climate adaptation strategy in fire-adapted systems, and remote sensing offers the opportunity to monitor and evaluate fire at unprecedented spatial and temporal scales. As the field of prescribed burn research advances, it will be critical to recognise the strengths and limitations of current methods, make explicit the trade-offs between wildfire and prescribed fire applications, and scale up the use of remote sensing optimised for prescribed fire. Our review contributes to these goals by synthesising trends, research aims and findings of studies using remote sensing for prescribed burn research in order to elucidate gaps and future directions within this growing field of research.
Data availability
All data included in the literature review are published, peer-reviewed studies. For a full list of the studies included in the analysis, see Supplementary Material.
Declaration of funding
K. S. acknowledges support from a NOAA Climate and Global Change Postdoctoral Fellowship. A. L. acknowledges support from the University of Colorado Boulder Graduate School, and the Joint Fire Science Program Graduate Research Innovation Award. B. P. and L. D. acknowledge support from NASA’s Terrestrial Ecology program. A. L., L. D., K. S. and N. S. acknowledge support from NASA Research Opportunities in Space and Earth Science (Award Number 80NSSC23K0397) and N. S. acknowledges support from WKID Solutions, LLC. L. D. and A. L. acknowledge support from the Morpho Initiative at the National Center for Ecological Analysis and Synthesis. The supporting sources were notinvolved in the preparation of the data or manuscript, or in the decision to submit for publication.
Acknowledgements
The authors acknowledge and thank Dr Miguel Villarreal for his contributions to the design of and data processing support for Fig. 6.
Author contributions
A. L. conceived the ideas and conducted the literature search. A. L. and M. H. designed methodology; A. L., M. H. and K. S. collected data (literature coding and data extraction); A. L. analysed the data (content analysis); A. L. and M. H. produced the figures; A. L., B. P., N. S. and L. D. synthesised literature and distilled key findings; A. L. led the writing of the manuscript with input from all authors, particularly N. S. and L. D. All authors contributed critically to the drafts and gave final approval for publication.
References
Abatzoglou JT, Williams AP (2016) Impact of anthropogenic climate change on wildfire across western US forests. Proceedings of the National Academy of Sciences 113(42), 11770-11775.
| Crossref | Google Scholar | PubMed |
Adlam C, Almendariz D, Goode RW, Martinez DJ, Middleton BR (2022) Keepers of the flame: supporting the revitalization of Indigenous cultural burning. Society & Natural Resources 35(5), 575-590.
| Crossref | Google Scholar |
Allen KA, Denelle P, Ruiz FMS, Santana VM, Marrs RH (2016) Prescribed moorland burning meets good practice guidelines: a monitoring case study using aerial photography in the Peak District, UK. Ecological Indicators 62, 76-85.
| Crossref | Google Scholar |
Ansley RJ, Pinchak WE, Teague WR, Kramp BA, Jones DL, Barnett K (2010) Integrated grazing and prescribed fire restoration strategies in a mesquite savanna: II. Fire behavior and mesquite landscape cover responses. Rangeland Ecology and Management 63(3), 286-297.
| Crossref | Google Scholar |
Arkle RS, Pilliod DS, Welty JL (2012) Pattern and process of prescribed fires influence effectiveness at reducing wildfire severity in dry coniferous forests. Forest Ecology and Management 276, 174-184.
| Crossref | Google Scholar |
Arreola Amaya M, Clements CB (2020) Evolution of plume core structures and turbulence during a wildland fire experiment. Atmosphere 11(8), 842.
| Crossref | Google Scholar |
Aydell TB, Clements CB (2021) Mobile Ka-Band polarimetric Doppler radar observations of wildfire smoke plumes. Monthly Weather Review 149(5), 1247-1264.
| Crossref | Google Scholar |
Baijnath-Rodino JA, Li S, Martinez A, Kumar M, Quinn-Davidson LN, York RA, Banerjee T (2022) Historical seasonal changes in prescribed burn windows in California. Science of The Total Environment 836, 155723.
| Crossref | Google Scholar | PubMed |
Barnes WL, Xiong X, Salomonson VV (2003) Status of Terra MODIS and Aqua MODIS. Advances in Space Research 32(11), 2099-2106.
| Crossref | Google Scholar |
Boer MM, Sadler RJ, Wittkuhn RS, McCaw L, Grierson PF (2009) Long-term impacts of prescribed burning on regional extent and incidence of wildfires – Evidence from 50 years of active fire management in SW Australian forests. Forest Ecology and Management 259(1), 132-142.
| Crossref | Google Scholar |
Bowman DMJS, Balch JK, Artaxo P, Bond WJ, Carlson JM, Cochrane MA, D’Antonio CM, DeFries RS, Doyle JC, Harrison SP, Johnston FH, Keeley JE, Krawchuk MA, Kull CA, Marston JB, Moritz MA, Prentice IC, Roos CI, Scott AC, Swetnam TW, Van Der Werf GR, Pyne SJ (2009) Fire in the Earth System. Science 324(5926), 481-484.
| Crossref | Google Scholar | PubMed |
Bucini G, Lambin EF (2002) Fire impacts on vegetation in central Africa: a remote-sensing-based statistical analysis. Applied Geography 22(1), 27-48.
| Crossref | Google Scholar |
Cansler CA, Kane VR, Hessburg PF, Kane JT, Jeronimo SMA, Lutz JA, Povak NA, Churchill DJ, Larson AJ (2022) Previous wildfires and management treatments moderate subsequent fire severity. Forest Ecology and Management 504, 119764.
| Crossref | Google Scholar |
Cary GJ, Davies ID, Bradstock RA, Keane RE, Flannigan MD (2017) Importance of fuel treatment for limiting moderate-to-high intensity fire: findings from comparative fire modelling. Landscape Ecology 32(7), 1473-1483.
| Crossref | Google Scholar |
Cawse-Nicholson K, Townsend PA, Schimel D, Assiri AM, Blake PL, Buongiorno MF, Campbell P, Carmon N, Casey KA, Correa-Pabón RE, Dahlin KM, Dashti H, Dennison PE, Dierssen H, Erickson A, Fisher JB, Frouin R, Gatebe CK, Gholizadeh H, Gierach M, Glenn NF, Goodman JA, Griffith DM, Guild L, Hakkenberg CR, Hockberg EJ, Holmes TRH, Hu C, Hulley G, Huemmrich KF, Kudela RM, Kokaly RF, Lee CM, Martin R, Miller CE, Moses WJ, Muller-Karger FE, Ortiz JD, Otis DB, Pahlevan N, Painter TH, Pavlick R, Poulter B, Qi Y, Realmuto VJ, Roberts D, Schaepman ME, Schneider FD, Schwandner FM, Serbin SP, Shiklomanov AN, Stavros EN, Thompson DR, Torres-Perez JL, Turpie KR, Tzortziou M, Ustin S, Yu Q, Yusup Y, Zhang Q, , the SBG Algorithms Working Group (2021) NASA’s surface biology and geology designated observable: a perspective on surface imaging algorithms. Remote Sensing of Environment 257, 112349.
| Crossref | Google Scholar |
Chapman JW, Thompson DR, Helmlinger MC, Bue BD, Green RO, Eastwood ML, Geier S, Olson-Duvall W, Lundeen SR (2019) Spectral and radiometric calibration of the next generation Airborne Visible Infrared Spectrometer (AVIRIS-NG). Remote Sensing 11(18), 2129.
| Crossref | Google Scholar |
Charland AM, Clements CB (2013) Kinematic structure of a wildland fire plume observed by Doppler lidar. Journal of Geophysical Research: Atmospheres 118(8), 3200-3212.
| Crossref | Google Scholar |
Clark KL, Skowronski N, Hom J, Duveneck M, Pan Y, Tuyl SV, Cole J, Patterson M, Maurer S (2009) Decision support tools to improve the effectiveness of hazardous fuel reduction treatments in the New Jersey Pine Barrens. International Journal of Wildland Fire 18(3), 268-277.
| Crossref | Google Scholar |
Coop JD, Parks SA, Stevens-Rumann CS, Crausbay SD, Higuera PE, Hurteau MD, Tepley A, Whitman E, Assal T, Collins BM, Davis KT, Dobrowski S, Falk DA, Fornwalt PJ, Fulé PZ, Harvey BJ, Kane VR, Littlefield CE, Margolis EQ, North M, Parisien MA, Prichard S, Rodman KC (2020) Wildfire-driven forest conversion in western North American landscapes. BioScience 70(8), 659-673.
| Crossref | Google Scholar | PubMed |
Davis GK (2007) History of the NOAA satellite program. Journal of Applied Remote Sensing 1(1), 012504.
| Crossref | Google Scholar |
Davis KT, Higuera PE, Dobrowski SZ, Parks SA, Abatzoglou JT, Rother MT, Veblen TT (2020) Fire-catalyzed vegetation shifts in ponderosa pine and Douglas-fir forests of the western United States. Environmental Research Letters 15(10), 1040b8.
| Crossref | Google Scholar |
Dickinson MB, Hudak AT, Zajkowski T, Loudermilk EL, Schroeder W, Ellison L, Kremens RL, Holley W, Martinez O, Paxton A, Bright BC, O’Brien JJ, Hornsby B, Ichoku C, Faulring J, Gerace A, Peterson D, Mauceri J (2015) Measuring radiant emissions from entire prescribed fires with ground, airborne and satellite sensors – RxCADRE 2012. International Journal of Wildland Fire 25(1), 48-61.
| Crossref | Google Scholar |
Eidenshink J, Schwind B, Brewer K, Zhu Z-L, Quayle B, Howard S (2007) A project for monitoring trends in burn severity. Fire Ecology 3(1), 3-21.
| Crossref | Google Scholar |
Ellis EC, Ramankutty N (2008) Putting people in the map: anthropogenic biomes of the world. Frontiers in Ecology and the Environment 6(8), 439-447.
| Crossref | Google Scholar |
Fernandes PM (2015) Empirical support for the use of prescribed burning as a fuel treatment. Current Forestry Reports 1(2), 118-127.
| Crossref | Google Scholar |
Fernandes PM, Botelho HS (2003) A review of prescribed burning effectiveness in fire hazard reduction. International Journal of Wildland Fire 12(2), 117-128.
| Crossref | Google Scholar |
Fernández-Guisuraga JM, Suárez-Seoane S, Fernandes PM, Fernández-García V, Fernández-Manso A, Quintano C, Calvo L (2022) Pre-fire aboveground biomass, estimated from LiDAR, spectral and field inventory data, as a major driver of burn severity in maritime pine (Pinus pinaster) ecosystems. Forest Ecosystems 9, 100022.
| Crossref | Google Scholar |
Fisher JB, Lee B, Purdy AJ, Halverson GH, Dohlen MB, Cawse-Nicholson K, Wang A, Anderson RG, Aragon B, Arain MA, Baldocchi DD, Baker JM, Barral H, Bernacchi CJ, Bernhofer C, Biraud SC, Bohrer G, Brunsell N, Cappelaere B, Castro-Contreras S, Chun J, Conrad BJ, Cremonese E, Demarty J, Desai AR, De Ligne A, Foltýnová L, Goulden ML, Griffis TJ, Grünwald T, Johnson MS, Kang M, Kelbe D, Kowalska N, Lim J-H, Maïnassara I, McCabe MF, Missik JEC, Mohanty BP, Moore CE, Morillas L, Morrison R, Munger JW, Posse G, Richardson AD, Russell ES, Ryu Y, Sanchez-Azofeifa A, Schmidt M, Schwartz E, Sharp I, Šigut L, Tang Y, Hulley G, Anderson M, Hain C, French A, Wood E, Hook S (2020) ECOSTRESS: NASA’s next generation mission to measure evapotranspiration from the International Space Station. Water Resources Research 56, e2019WR026058.
| Crossref | Google Scholar |
Franke J, Barradas ACS, Borges MA, Menezes Costa M, Dias PA, Hoffmann AA, Orozco Filho JC, Melchiori AE, Siegert F (2018) Fuel load mapping in the Brazilian Cerrado in support of integrated fire management. Remote Sensing of Environment 217, 221-232.
| Crossref | Google Scholar |
Goodrich DC, Wei H, Burns IS, Guertin DP, Spaeth K, Hernandez M, Holifield-Collins C, Kautz M, Heilman P, Levick LR, Ponce G, Carrillo E, Tiller R (2020) Evaluation of conservation effects assessment project grazing lands conservation practices on the Cienega Creek watershed in southeast Arizona with AGWA/RHEM modeling tools. Journal of Soil and Water Conservation 75(3), 304-318.
| Crossref | Google Scholar |
Gorelick N, Hancher M, Dixon M, Ilyushchenko S, Thau D, Moore R (2017) Google Earth Engine: planetary-scale geospatial analysis for everyone. Remote Sensing of Environment 202, 18-27.
| Crossref | Google Scholar |
Green RO, Eastwood ML, Sarture CM, Chrien TG, Aronsson M, Chippendale BJ, Faust JA, Pavri BE, Chovit CJ, Solis M, Olah MR, Williams O (1998) Imaging spectroscopy and the Airborne Visible/Infrared Imaging Spectrometer (AVIRIS). Remote Sensing of Environment 65(3), 227-248.
| Crossref | Google Scholar |
Green RO, Mahowald N, Ung C, Thompson DR, Bator L, Bennet M, Bernas M, Blackway N, Bradley C, Cha J, Clark P, Clark R, Cloud D, Diaz E, Ben Dor E, Duren R, Eastwood M, Ehlmann BL, Fuentes L, Ginoux P, Gross J, He Y, Kalashnikova O, Kert W, Keymeulen D, Klimesh M, Ku D, Kwong-Fu H, Liggett E, Li L, Lundeen S, Makowski MD, Mazer A, Miller R, Mouroulis P, Oaida B, Okin GS, Ortega A, Oyake A, Nguyen H, Pace T, Painter TH, Pempejian J, Garcia-Pando CP, Pham T, Phillips B, Pollock R, Purcell R, Realmuto V, Schoolcraft J, Sen A, Shin S, Shaw L, Soriano M, Swayze G, Thingvold E, Vaid A, Zan J (2020) The Earth Surface Mineral Dust Source Investigation: An Earth Science Imaging Spectroscopy Mission. In ‘2020 IEEE Aerospace Conference’. Presented at the 2020 IEEE Aerospace Conference. pp. 1–15. (Institute of Electrical and Electronics Engineers)
Gupta V, Reinke KJ, Jones SD, Wallace L, Holden L (2015) Assessing metrics for estimating fire-induced change in the forest understorey structure using terrestrial laser scanning. Remote Sensing 7(6), 8180-8201.
| Crossref | Google Scholar |
Hiers JK, O’Brien JJ, Varner JM, Butler BW, Dickinson M, Furman J, Gallagher M, Godwin D, Goodrick SL, Hood SM, Hudak A, Kobziar LN, Linn R, Loudermilk EL, McCaffrey S, Robertson K, Rowell EM, Skowronski N, Watts AC, Yedinak KM (2020) Prescribed fire science: the case for a refined research agenda. Fire Ecology 16(1), 11.
| Crossref | Google Scholar |
Hook SJ, Myers JJ, Thome KJ, Fitzgerald M, Kahle AB (2001) The MODIS/ASTER airborne simulator (MASTER) – A new instrument for earth science studies. Remote Sensing of Environment 76(1), 93-102.
| Crossref | Google Scholar |
Huang R, Zhang X, Chan D, Kondragunta S, Russell AG, Odman MT (2018) Burned area Ccmparisons between prescribed burning permits in southeastern United States and two satellite-derived products. Journal of Geophysical Research: Atmospheres 123(9), 4746-4757.
| Crossref | Google Scholar |
Hudak AT, Kato A, Bright BC, Loudermilk EL, Hawley C, Restaino JC, Ottmar RD, Prata GA, Cabo C, Prichard SJ, Rowell EM, Weise DR (2020) Towards spatially explicit quantification of pre- and post-fire fuels and fuel consumption from traditional and point cloud measurements. Forest Science 66(4), 428-442.
| Crossref | Google Scholar |
Hunter ME, Robles MD (2020) Tamm review: the effects of prescribed fire on wildfire regimes and impacts: a framework for comparison. Forest Ecology and Management 475, 118435.
| Crossref | Google Scholar |
Johnston FH, Borchers-Arriagada N, Morgan GG, Jalaludin B, Palmer AJ, Williamson GJ, Bowman DMJS (2021) Unprecedented health costs of smoke-related PM2.5 from the 2019–20 Australian megafires. Nature Sustainability 4(1), 42-47.
| Crossref | Google Scholar |
Jolly WM, Cochrane MA, Freeborn PH, Holden ZA, Brown TJ, Williamson GJ, Bowman DMJS (2015) Climate-induced variations in global wildfire danger from 1979 to 2013. Nature Communications 6(1), 7537.
| Crossref | Google Scholar | PubMed |
Kashian DM, Romme WH, Tinker DB, Turner MG, Ryan MG (2006) Carbon storage on landscapes with stand-replacing fires. BioScience 56(7), 598-606.
| Crossref | Google Scholar |
Keeley JE (2009) Fire intensity, fire severity and burn severity: a brief review and suggested usage. International Journal of Wildland Fire 18(1), 116-126.
| Crossref | Google Scholar |
Kennedy MC, Ford ED, Singleton P, Finney M, Agee JK (2008) Informed multi-objective decision-making in environmental management using Pareto optimality. Journal of Applied Ecology 45(1), 181-192.
| Crossref | Google Scholar |
Kibler CL, Parkinson A-ML, Peterson SH, Roberts DA, D’Antonio CM, Meerdink SK, Sweeney SH (2019) Monitoring post-fire recovery of chaparral and conifer species using field surveys and Landsat time series. Remote Sensing 11(24), 2963.
| Crossref | Google Scholar |
Kiefer MT, Heilman WE, Zhong S, Charney JJ, Bian X, Skowronski NS, Hom JL, Clark KL, Patterson M, Gallagher MR (2014) Multiscale simulation of a prescribed fire event in the New Jersey Pine Barrens using ARPS-CANOPY. Journal of Applied Meteorology and Climatology 53(4), 793-812.
| Crossref | Google Scholar |
Kochi I, Donovan GH, Champ PA, Loomis JB, Kochi I, Donovan GH, Champ PA, Loomis JB (2010) The economic cost of adverse health effects from wildfire-smoke exposure: a review. International Journal of Wildland Fire 19(7), 803-817.
| Crossref | Google Scholar |
Kolden CA (2019) We’re not doing enough prescribed fire in the Western United States to mitigate wildfire risk. Fire 2(2), 30.
| Crossref | Google Scholar |
Lake FK, Wright V, Morgan P, McFadzen M, McWethy D, Stevens-Rumann C (2017) Returning fire to the land: celebrating traditional knowledge and fire. Journal of Forestry 115(5), 343-353 Pmmcwethydstevens-rumannc.
| Crossref | Google Scholar |
Leite RV, Silva CA, Broadbent EN, do Amaral CH, Liesenberg V, de Almeida DRA, Mohan M, Godinho S, Cardil A, Hamamura C, de Faria BL, Brancalion PHS, Hirsch A, Marcatti GE, Dalla Corte AP, Zambrano AMA, da Costa MBT, Matricardi EAT, da Silva AL, Goya LRRY, Valbuena R, de Mendonça BAF, Silva Junior CHL, Aragão LEOC, García M, Liang J, Merrick T, Hudak AT, Xiao J, Hancock S, Duncason L, Ferreira MP, Valle D, Saatchi S, Klauberg C (2022) Large scale multi-layer fuel load characterization in tropical savanna using GEDI spaceborne lidar data. Remote Sensing of Environment 268, 112764.
| Crossref | Google Scholar |
Li F, Zhang X, Kondragunta S, Schmidt CC, Holmes CD (2020) A preliminary evaluation of GOES-16 active fire product using Landsat-8 and VIIRS active fire data, and ground-based prescribed fire records. Remote Sensing of Environment 237, 111600.
| Crossref | Google Scholar |
Li X, Song W, Lian L, Wei X (2015) Forest fire smoke detection using back-propagation neural network based on MODIS data. Remote Sensing 7(4), 4473-4498.
| Crossref | Google Scholar |
Liu T, Mickley LJ, McCarty JL (2021) Global search for temporal shifts in fire activity: potential human influence on southwest Russia and north Australia fire seasons. Environmental Research Letters 16(4), 044023.
| Crossref | Google Scholar |
Loudermilk EL, Achtemeier GL, O’Brien JJ, Hiers JK, Hornsby BS (2014) High-resolution observations of combustion in heterogeneous surface fuels. International Journal of Wildland Fire 23(7), 1016-1026.
| Crossref | Google Scholar |
Marks-Block T, Tripp W (2021) Facilitating prescribed fire in northern California through Indigenous governance and interagency partnerships. Fire 4(3), 47.
| Crossref | Google Scholar |
McCarley TR, Kolden CA, Vaillant NM, Hudak AT, Smith AMS, Wing BM, Kellogg BS, Kreitler J (2017) Multi-temporal LiDAR and Landsat quantification of fire-induced changes to forest structure. Remote Sensing of Environment 191, 419-432.
| Crossref | Google Scholar |
McCarthy N, McGowan H, Guyot A, Dowdy A (2018) Mobile X-Pol Radar: a new tool for investigating pyroconvection and associated wildfire meteorology. Bulletin of the American Meteorological Society 99(6), 1177-1195.
| Crossref | Google Scholar |
McWethy DB, Schoennagel T, Higuera PE, Krawchuk M, Harvey BJ, Metcalf EC, Schultz C, Miller C, Metcalf AL, Buma B, Virapongse A, Kulig JC, Stedman RC, Ratajczak Z, Nelson CR, Kolden C (2019) Rethinking resilience to wildfire. Nature Sustainability 2(9), 797-804.
| Crossref | Google Scholar |
Meddens AJH, Kolden CA, Lutz JA, Smith AMS, Cansler CA, Abatzoglou JT, Meigs GW, Downing WM, Krawchuk MA (2018) Fire refugia: what are they, and why do they matter for global change? BioScience 68(12), 944-954.
| Crossref | Google Scholar |
Miao Z, Lathrop RG, Xu M, La Puma IP, Clark KL, Hom J, Skowronski N, Van Tuyl S (2011) Simulation and sensitivity analysis of carbon storage and fluxes in the New Jersey Pinelands. Environmental Modelling & Software 26(9), 1112-1122.
| Crossref | Google Scholar |
Mietkiewicz N, Balch JK, Schoennagel T, Leyk S, St Denis LA, Bradley BA (2020) In the line of fire: consequences of human-ignited wildfires to homes in the US (1992–2015). Fire 3(3), 50.
| Crossref | Google Scholar |
Mikolajewicz N, Komarova SV (2019) Meta-analytic methodology for basic research: a practical guide. Frontiers in Physiology 10, 203.
| Crossref | Google Scholar | PubMed |
Molina JR, Ortega M, Rodríguez y Silva F (2022) Fire ignition patterns to manage prescribed fire behavior: application to Mediterranean pine forests. Journal of Environmental Management 302, 114052.
| Crossref | Google Scholar | PubMed |
Nguyen TH, Jones SD, Soto-Berelov M, Haywood A, Hislop S (2018) A spatial and temporal analysis of forest dynamics using Landsat time-series. Remote Sensing of Environment 217, 461-475.
| Crossref | Google Scholar |
Olson DM, Dinerstein E, Wikramanayake ED, Burgess ND, Powell GVN, Underwood EC, D’amico JA, Itoua I, Strand HE, Morrison JC, Loucks CJ, Allnutt TF, Ricketts TH, Kura Y, Lamoreux F, Wettengel WW, Hedao P, Kassem KR (2001) Terrestrial ecoregions of the world: a new map of life on Earth: a new global map of terrestrial ecoregions provides an innovative tool for conserving biodiversity. BioScience 51(11), 933-938.
| Crossref | Google Scholar |
Parks SA, Dillon GK, Miller C (2014) A new metric for quantifying burn severity: the relativized burn ratio. Remote Sensing 6(3), 1827-1844.
| Crossref | Google Scholar |
Parks SA, Holsinger LM, Voss MA, Loehman RA, Robinson NP (2018) Mean composite fire severity metrics computed with Google Earth Engine offer improved accuracy and expanded mapping potential. Remote Sensing 10, 879.
| Crossref | Google Scholar |
Pausas JG, Keeley JE (2021) Wildfires and global change. Frontiers in Ecology and the Environment 19(7), 387-395.
| Crossref | Google Scholar |
Petrakis RE, Villarreal ML, Wu Z, Hetzler R, Middleton BR, Norman LM (2018) Evaluating and monitoring forest fuel treatments using remote sensing applications in Arizona, USA. Forest Ecology and Management 413, 48-61.
| Crossref | Google Scholar |
Pouliot GA, Pace TG, Biswadev R, Pierce T, Mobley D (2008) Development of a biomass burning emissions inventory by combining satellite and ground-based information. Journal of Applied Remote Sensing 2(1), 021501.
| Crossref | Google Scholar |
Price OF, Russell-Smith J, Watt F (2012) The influence of prescribed fire on the extent of wildfire in savanna landscapes of western Arnhem Land, Australia. International Journal of Wildland Fire 21, 297-305.
| Crossref | Google Scholar |
Prichard SJ, Peterson DL, Jacobson K (2010) Fuel treatments reduce the severity of wildfire effects in dry mixed conifer forest, Washington, USA. Canadian Journal of Forest Research 40(8), 1615-1626.
| Crossref | Google Scholar |
Qi Y, Dennison PE, Spencer J, Riaño D (2012) Monitoring live fuel moisture using soil moisture and remote sensing proxies. Fire Ecology 8(3), 71-87.
| Crossref | Google Scholar |
Radford IJ, Woolley L-A, Corey B, Vigilante T, Hatherley E, Fairman R, Carnes K, Start AN, Wunambal Gaambera Aboriginal Corporation (2020) Prescribed burning benefits threatened mammals in northern Australia. Biodiversity and Conservation 29(9), 2985-3007.
| Crossref | Google Scholar |
Rauste Y, Herland E, Frelander H, Soini K, Kuoremaki T, Ruokari A (1997) Satellite-based forest fire detection for fire control in boreal forests. International Journal of Remote Sensing 18(12), 2641-2656.
| Crossref | Google Scholar |
Rowell EM, Seielstad CA, Ottmar RD, Rowell EM, Seielstad CA, Ottmar RD (2015) Development and validation of fuel height models for terrestrial lidar – RxCADRE 2012. International Journal of Wildland Fire 25(1), 38-47.
| Crossref | Google Scholar |
Ryan KC, Knapp EE, Varner JM (2013) Prescribed fire in North American forests and woodlands: history, current practice, and challenges. Frontiers in Ecology and the Environment 11(s1), e15-e24.
| Crossref | Google Scholar |
Saatchi S, Halligan K, Despain DG, Crabtree RL (2007) Estimation of forest fuel load from radar remote sensing. IEEE Transactions on Geoscience and Remote Sensing 45(6), 1726-1740.
| Crossref | Google Scholar |
Sankey JB, Sankey TT, Li J, Ravi S, Wang G, Caster J, Kasprak A (2021) Quantifying plant–soil–nutrient dynamics in rangelands: fusion of UAV hyperspectral-LiDAR, UAV multispectral-photogrammetry, and ground-based LiDAR-digital photography in a shrub-encroached desert grassland. Remote Sensing of Environment 253, 112223.
| Crossref | Google Scholar |
Schultz CA, McCaffrey SM, Huber-Stearns HR (2019) Policy barriers and opportunities for prescribed fire application in the western United States. International Journal of Wildland Fire 28(11), 874.
| Crossref | Google Scholar |
Schweizer D, Preisler HK, Cisneros R (2019) Assessing relative differences in smoke exposure from prescribed, managed, and full suppression wildland fire. Air Quality, Atmosphere & Health 12(1), 87-95.
| Crossref | Google Scholar |
Showstack R (2014) Sentinel satellites initiate new era in Earth observation. Eos, Transactions American Geophysical Union 95(26), 239-240.
| Crossref | Google Scholar |
Shrestha M, Broadbent EN, Vogel JG (2021) Using GatorEye UAV-Borne LiDAR to quantify the spatial and temporal effects of a prescribed fire on understory height and biomass in a pine savanna. Forests 12(1), 38.
| Crossref | Google Scholar |
Shuman JK, Balch JK, Barnes RT, Higuera PE, Roos CI, Schwilk DW, Stavros EN, Banerjee T, Bela MM, Bendix J, Bertolino S, Bililign S, Bladon KD, Brando P, Breidenthal RE, Buma B, Calhoun D, Carvalho LMV, Cattau ME, Cawley KM, Chandra S, Chipman ML, Cobian-Iñiguez J, Conlisk E, Coop JD, Cullen A, Davis KT, Dayalu A, De Sales F, Dolman M, Ellsworth LM, Franklin S, Guiterman CH, Hamilton M, Hanan EJ, Hansen WD, Hantson S, Harvey BJ, Holz A, Huang T, Hurteau MD, Ilangakoon NT, Jennings M, Jones C, Klimaszewski-Patterson A, Kobziar LN, Kominoski J, Kosovic B, Krawchuk MA, Laris P, Leonard J, Loria-Salazar SM, Lucash M, Mahmoud H, Margolis E, Maxwell T, McCarty JL, McWethy DB, Meyer RS, Miesel JR, Moser WK, Nagy RC, Niyogi D, Palmer HM, Pellegrini A, Poulter B, Robertson K, Rocha AV, Sadegh M, Santos F, Scordo F, Sexton JO, Sharma AS, Smith AMS, Soja AJ, Still C, Swetnam T, Syphard AD, Tingley MW, Tohidi A, Trugman AT, Turetsky M, Varner JM, Wang Y, Whitman T, Yelenik S, Zhang X (2022) Reimagine fire science for the Anthropocene. PNAS Nexus 1(3), 115.
| Crossref | Google Scholar | PubMed |
Skowronski N, Clark K, Nelson R, Hom J, Patterson M (2007) Remotely sensed measurements of forest structure and fuel loads in the Pinelands of New Jersey. Remote Sensing of Environment 108(2), 123-129.
| Crossref | Google Scholar |
Soja AJ, Al-Saadi JA, Giglio L, Randall D, Kittaka C, Pouliot GA, Kordzi JJ, Raffuse SM, Pace TG, Pierce T, Moore T, Roy B, Pierce B, Szykman JJ (2009) Assessing satellite-based fire data for use in the National Emissions Inventory. Journal of Applied Remote Sensing 3(1), 031504.
| Crossref | Google Scholar |
Soverel NO, Coops NC, Perrakis DDB, Daniels LD, Gergel SE (2011) The transferability of a dNBR-derived model to predict burn severity across 10 wildland fires in western Canada. International Journal of Wildland Fire 20, 518-531.
| Crossref | Google Scholar |
Srivastava SK, Lewis T, Behrendorff L, Phinn S (2020) Spatial databases and techniques to assist with prescribed fire management in the south-east Queensland bioregion. International Journal of Wildland Fire 30, 90-111.
| Crossref | Google Scholar |
Stavros EN, Coen J, Peterson B, Singh H, Kennedy K, Ramirez C, Schimel D (2018) Use of imaging spectroscopy and LIDAR to characterize fuels for fire behavior prediction. Remote Sensing Applications: Society and Environment 11, 41-50.
| Crossref | Google Scholar |
Stavros EN, Chrone J, Cawse-Nicholson K, Freeman A, Glenn NF, Guild L, Kokaly R, Lee C, Luvall J, Pavlick R, Poulter B, Uz SS, Serbin S, Thompson DR, Townsend PA, Turpie K, Yuen K, Thome K, Wang W, Zareh S-K, Nastal J, Bearden D, Miller CE, Schimel D (2022) Designing an observing system to study the Surface Biology and Geology (SBG) of the Earth in the 2020s. Journal of Geophysical Research: Biogeosciences 128(1), e2021JG006471.
| Crossref | Google Scholar | PubMed |
Stephens SL, McIver JD, Boerner REJ, Fettig CJ, Fontaine JB, Hartsough BR, Kennedy PL, Schwilk DW (2012) The effects of forest fuel-reduction treatments in the United States. BioScience 62(6), 549-560.
| Crossref | Google Scholar |
Stephens SL, Kobziar LN, Collins BM, Davis R, Fulé PZ, Gaines W, Ganey J, Guldin JM, Hessburg PF, Hiers K, Hoagland S, Keane JJ, Masters RE, McKellar AE, Montague W, North M, Spies TA (2019) Is fire “for the birds”? How two rare species influence fire management across the US. Frontiers in Ecology and the Environment 17(7), 391-399.
| Crossref | Google Scholar |
Syphard AD, Brennan TJ, Keeley JE (2019) Drivers of chaparral type conversion to herbaceous vegetation in coastal southern California. Diversity and Distributions 25(1), 90-101.
| Crossref | Google Scholar |
Szpakowski DM, Jensen JLR (2019) A review of the applications of remote sensing in fire ecology. Remote Sensing 11(22), 2638.
| Crossref | Google Scholar |
Temudo MP, Oom D, Pereira JM (2020) Bio-cultural fire regions of Guinea-Bissau: analysis combining social research and satellite remote sensing. Applied Geography 118, 102203.
| Crossref | Google Scholar |
Tian X, Zhao F, Shu L, Wang M (2013) Distribution characteristics and the influence factors of forest fires in China. Forest Ecology and Management 310, 460-467.
| Crossref | Google Scholar |
Trisos CH, Auerbach J, Katti M (2021) Decoloniality and anti-oppressive practices for a more ethical ecology. Nature Ecology & Evolution 5(9), 1205-1212.
| Crossref | Google Scholar | PubMed |
Turner MG, Hargrove WW, Gardner RH, Romme WH (1994) Effects of fire on landscape heterogeneity in Yellowstone National Park, Wyoming. Journal of Vegetation Science 5(5), 731-742.
| Crossref | Google Scholar |
van Leeuwen WJD, Casady GM, Neary DG, Bautista S, Alloza JA, Carmel Y, Wittenberg L, Malkinson D (2010) Monitoring post-wildfire vegetation response with remotely sensed time-series data in Spain, USA and Israel. International Journal of Wildland Fire 19(1), 75-93.
| Crossref | Google Scholar |
Veraverbeke S, Stavros EN, Hook SJ (2014) Assessing fire severity using imaging spectroscopy data from the Airborne Visible/Infrared Imaging Spectrometer (AVIRIS) and comparison with multispectral capabilities. Remote Sensing of Environment 154, 153-163.
| Crossref | Google Scholar |
Veraverbeke S, Dennison P, Gitas I, Hulley G, Kalashnikova O, Katagis T, Kuai L, Meng R, Roberts D, Stavros EN (2018) Hyperspectral remote sensing of fire: state-of-the-art and future perspectives. Remote Sensing of Environment 216, 105-121.
| Crossref | Google Scholar |
Volkova L, Weiss Aparicio AG, Weston CJ (2019) Fire intensity effects on post-fire fuel recovery in Eucalyptus open forests of south-eastern Australia. Science of The Total Environment 670, 328-336.
| Crossref | Google Scholar | PubMed |
Webster KM, Halpern CB (2010) Long-term vegetation responses to reintroduction and repeated use of fire in mixed-conifer forests of the Sierra Nevada. Ecosphere 1(5), 1-27.
| Crossref | Google Scholar |
Wells AG, Munson SM, Sesnie SE, Villarreal ML (2021) Remotely sensed fine-fuel changes from wildfire and prescribed fire in a semi-arid grassland. Fire 4(4), 84.
| Crossref | Google Scholar |
Weston CJ, Di Stefano J, Hislop S, Volkova L (2022) Effect of recent fuel reduction treatments on wildfire severity in southeast Australian Eucalyptus sieberi forests. Forest Ecology and Management 505, 119924.
| Crossref | Google Scholar |
White JD, Ryan KC, Key CC, Running SW (1996) Remote sensing of forest fire severity and vegetation recovery. International Journal of Wildland Fire 6(3), 125-136.
| Crossref | Google Scholar |
Wiens J, Sutter R, Anderson M, Blanchard J, Barnett A, Aguilar-Amuchastegui N, Avery C, Laine S (2009) Selecting and conserving lands for biodiversity: the role of remote sensing. Remote Sensing of Environment 113(7), 1370-1381.
| Crossref | Google Scholar |
Wooster MJ, Roberts GJ, Giglio L, Roy DP, Freeborn PH, Boschetti L, Justice C, Ichoku C, Schroeder W, Davies D, Smith AMS, Setzer A, Csiszar I, Strydom T, Frost P, Zhang T, Xu W, de Jong MC, Johnston JM, Ellison L, Vadrevu K, Sparks AM, Nguyen H, McCarty J, Tanpipat V, Schmidt C, San-Miguel-Ayanz J (2021) Satellite remote sensing of active fires: history and current status, applications and future requirements. Remote Sensing of Environment 267, 112694.
| Crossref | Google Scholar |
Wulder MA, White JC, Alvarez F, Han T, Rogan J, Hawkes B (2009) Characterizing boreal forest wildfire with multi-temporal Landsat and LIDAR data. Remote Sensing of Environment 113(7), 1540-1555.
| Crossref | Google Scholar |
Wulder MA, Loveland TR, Roy DP, Crawford CJ, Masek JG, Woodcock CE, Allen RG, Anderson MC, Belward AS, Cohen WB, Dwyer J, Erb A, Gao F, Griffiths P, Helder D, Hermosilla T, Hipple JD, Hostert P, Hughes MJ, Huntington J, Johnson DM, Kennedy R, Kilic A, Li Z, Lymburner J, McCorkel J, Pahlevan N, Scambos TA, Schaaf C, Schott JR, Sheng Y, Storey J, Vermote E, Vogelmann J, White JC, Wynne RH, Zhu Z (2019) Current status of Landsat program, science, and applications. Remote Sensing of Environment 225, 127-147.
| Crossref | Google Scholar |
Xiao J, Chevallier F, Gomez C, Guanter L, Hicke JA, Huete AR, Ichii K, Ni W, Pang Y, Rahman AF, Sun G, Yuan W, Zhang L, Zhang X (2019) Remote sensing of the terrestrial carbon cycle: a review of advances over 50 years. Remote Sensing of Environment 233, 111383.
| Crossref | Google Scholar |
Xie J, Qi T, Hu W, Huang H, Chen B, Zhang J (2022) Retrieval of live fuel moisture content based on multi-source remote sensing data and ensemble deep learning model. Remote Sensing 14(17), 4378.
| Crossref | Google Scholar |
Xu G, Zhong X (2017) Real-time wildfire detection and tracking in Australia using geostationary satellite: Himawari-8. Remote Sensing Letters 8(11), 1052-1061.
| Crossref | Google Scholar |
Yebra M, Dennison PE, Chuvieco E, Riaño D, Zylstra P, Hunt ER, Danson FM, Qi Y, Jurdao S (2013) A global review of remote sensing of live fuel moisture content for fire danger assessment: moving towards operational products. Remote Sensing of Environment 136, 455-468.
| Crossref | Google Scholar |
Zeng T, Liu Z, Wang Y (2016) Large fire emissions in summer over the southeastern US: satellite measurements and modeling analysis. Atmospheric Environment 127, 213-220.
| Crossref | Google Scholar |