Comparing two methods to measure oxidative pyrolysis gases in a wind tunnel and in prescribed burns
David R. Weise







A USDA Forest Service, Pacific Southwest Research Station, Riverside, CA 92507, USA.
B Pacific Northwest National Laboratory, Richland, WA 99352, USA.
C USDA Forest Service, Rocky Mountain Research Station, Missoula, MT 59808, USA.
D Department of Computer Sciences, Applied Mathematics and Statistics, University of Girona, Girona, Spain.
International Journal of Wildland Fire 32(1) 56-77 https://doi.org/10.1071/WF22079
Submitted: 24 May 2022 Accepted: 28 October 2022 Published: 30 November 2022
© 2023 The Author(s) (or their employer(s)). Published by CSIRO Publishing on behalf of IAWF. This is an open access article distributed under the Creative Commons Attribution 4.0 International License (CC BY).
Abstract
Background: Fire models use pyrolysis data from ground samples and environments that differ from wildland conditions. Two analytical methods successfully measured oxidative pyrolysis gases in wind tunnel and field fires: Fourier transform infrared (FTIR) spectroscopy and gas chromatography with flame-ionisation detector (GC-FID). Compositional data require appropriate statistical analysis.
Aims: To determine if oxidative pyrolysis gas composition differed between analytical methods and locations (wind tunnel and field).
Methods: Oxidative pyrolysis gas sample composition collected in wind tunnel and prescribed fires was determined by FTIR and GC/FID. Proportionality between gases was tested. Analytical method and location effects on composition were tested using permutational multivariate analysis of variance and the Kruskal–Wallis test.
Key results: Gases proportional to each other were identified. The FTIR composition differed between locations. The subcomposition of common gases differed between analytical methods but not between locations. Relative amount of the primary fuel gases (CO, CH4) was not significantly affected by location.
Conclusions: Composition of trace gases differed between the analytical methods; however, each method yielded a comparable description of the primary fuel gases.
Implications: Both FTIR and GC/FID methods can be used to quantify primary pyrolysis fuel gases for physically-based fire models. Importance of the trace gases in combustion models remains to be determined.
Keywords: compositional data analysis, Fourier transform infrared spectroscopy, FTIR, gas chromatography/flame ionisation detector, gas composition, GC/FID, log-ratio, Pinus palustris.
Introduction
Wildland fire is a complex phenomenon of chemical and physical processes. Two of the chemical processes which are key to wildland fire are pyrolysis and combustion (Shafizadeh 1984; Ward 2001). During pyrolysis, a solid wildland fuel is heated and breaks down into constituent parts consisting of gases, tars and a solid material called char (Shafizadeh and Fu 1973; Shafizadeh 1982; Di Blasi 2008). During combustion, gaseous pyrolysis products react with oxygen releasing energy (heat) and a large assortment of gaseous and solid chemical compounds (e.g. Andreae and Merlet 2001; Akagi et al. 2011; May et al. 2014). Oxidation reactions involving atmospheric gases such as the oxides of nitrogen occur (Lobert et al. 1990; Crutzen and Brauch 2016).
The primary components of wildland fuels are cellulose, hemicellulose and lignin (Shafizadeh 1982; Collard and Blin 2014). These components decompose thermally by a variety of pathways which are (a) temperature dependent (Shafizadeh 1982; Neves et al. 2011; Sekimoto et al. 2018) and (b) affected by the presence/absence of O2 (e.g. Senneca et al. 2007). During thermal decomposition, mass is conserved, meaning that the total mass of pyrolysis products will not exceed the sum of the beginning biomass and any O2 which reacts with the pyrolysis products. An increase in the production of one product results in a concomitant decrease in one or more of the other products. Earlier works describing pyrolysis products from the foliar component of wildland fuels typically focused on determining the amount of combustible gas produced by heating and did not report the chemical composition (e.g. Susott et al. 1975, 1979; Susott 1982a, 1982b; Rogers et al. 1986). Other early works focused on other parameters such as moisture content, crude fat content and fibre content (e.g. Richards 1940; Philpot 1969, 1971). More recent flammability-related work isolated particular components of the mixtures (e.g. Alessio et al. 2008; Chetehouna et al. 2009). In fact, many gases comprise the pyrolytic mixture and both the plant species and heating rate/mode affect the compositional makeup (e.g. Tihay and Gillard 2010; Safdari et al. 2018; Amini et al. 2019a, 2019b; Safdari et al. 2019, 2020; Weise et al. 2022b). These mixtures reported in our recent work contained CO, CO2, CH4, H2 and phenol in relatively large amounts; most of the other gases occurred in relatively small amounts as trace gases.
A wide variety of methods and instruments are available to identify and quantify the hundreds of gaseous and particulate compounds produced by the heating and combustion of wildland fuels. The methods and instrumentation have been compared in several studies (e.g. Fehsenfeld et al. 1987; Christian et al. 2004; Warneke et al. 2011; Haase et al. 2012; Kajos et al. 2015; Tasoglou et al. 2018; Li et al. 2019; Pistone et al. 2019). They have also been used in a complementary fashion to increase the detection and quantification of as large a suite of compounds as possible (e.g. Yokelson et al. 2007, 2013; Burling et al. 2010). Ward and Radke (1993) compared and contrasted different methods of describing smoke emissions from bench-scale measurement to aircraft and satellite measurement.
Linking laboratory results to field-scale phenomena has long been a topic of interest and the field of wildland fire and smoke emissions is no exception (Ward and Radke 1993; Yokelson et al. 2013). One potential measure to link results across scales is combustion efficiency η, defined as follows:

where CO2a and CO2t are the actual amount released by a fire and the theoretical amount if all carbon was converted to CO2, respectively, and ‘C’ denotes the amount of carbon contained in each of the smoke components (HC, hydrocarbons; PM, particulate matter). The η parameter based on CO2 was closely correlated with η based on the ratio of the amount of heat released by oxidation and the heat of combustion (Ward and Radke 1993). Further development of this concept led to the use of the CO/CO2 ratio and modified combustion efficiency (MCE, ΔCO2/[ΔCO2 + ΔCO]) as descriptors of a fire and as ‘independent’ predictors of other smoke components (Ward and Hao 1991; Yokelson et al. 1996) because MCE was often ‘well-correlated’ with other emission components. The problematic use of correlation measures on proportional relative data has long been known (Pearson 1897; Aitchison 1982). Thus, the widely-assumed convention that CO/CO2, and thus MCE, is a completely independent predictor for other smoke components has been shown to be incorrect (Weise et al. 2020b). While a suitable replacement for MCE has yet to be developed, Weise et al. (2020b) used a compositional linear trend to estimate trace gas composition over a range of combustion efficiency and reduced prediction error by 4% compared to linear regressions using MCE.
Much of the work describing the composition of pyrolysis products has occurred in bench-scale experiments and the results have been assumed to be applicable to wildland fires (e.g. Susott et al. 1979; Dimitrakopoulos 2001). However, we found no literature supporting the assumption that bench-scale experiments are applicable to the wildland fire setting. As a result, the U.S. Strategic Environmental Research and Development Program funded a series of studies designed to examine the linkages between bench-scale experiments and the wildland fire setting (Weise et al. 2022a). To date, we have used compositional data analysis (CoDA) techniques that consider the multivariate relative nature of these data to analyse the effects of plant species, heating mode and moisture content on non-oxidative pyrolysis products at bench-scale (Weise et al. 2022b). Using CoDA, we compared gases produced during flaming combustion and oxidative pyrolysis in a series of wind tunnel and field fires that were quantified using a gas chromatograph equipped with a flame ionisation detector (GC/FID) instrument (Weise et al. 2022c). The objective of the present manuscript is to compare the composition of oxidative pyrolysis gases measured in these same fires using GC/FID and Fourier transform infrared (FTIR) spectroscopy to determine if the methods produce comparable results. Both techniques have been used extensively in bench-scale pyrolysis experiments and are accepted methods to quantify the quantity and composition of pyrolysis gases.
Methods
Oxidative pyrolysis gas composition was determined by two analytical methods (GC/FID and FTIR) in wind tunnel and field settings (Fig. 1). The complete GC/FID data have been analysed and presented elsewhere (Weise et al. 2022c). We first present an analysis of the FTIR data which includes reanalysis of the field fire data (Scharko et al. 2019a) and then examine the subcomposition (subset) of gases measured by both methods. The approach to analysing the collected gases consisted of several steps. The composition of oxidative pyrolysis gases measured using FTIR was compared between wind tunnel and field experiments. The relative amounts of the subcomposition of common gases in the GC/FID and FTIR datasets (CO, CO2, CH4, C2H2, C2H4, C3H6, C4H6 and isobutene) for the wind tunnel and field data were then compared to determine if the subcomposition was affected by the analytical method or the sampling location (wind tunnel and field).
Wind tunnel fires
Eighty-eight fuel beds 2.4 m long and approximately 0.8 m wide composed of longleaf pine (Pinus palustris Mill.) needles and various combinations of fetterbush (Lyonia lucida (Lam.) K. Koch), sparkleberry (Vaccinium arboreum L.), blueberry (V. darrowii Camp) and inkberry (Ilex glabra (L.) A. Gray) (Table 1) were burned in the Riverside Fire Laboratory (RFL) wind tunnel. Wind tunnel burns were carried out under ‘no wind’ and 1 m s−1 conditions. Fuel beds were constructed by uniformly distributing 1000 g (wet weight) of needles over the entire fuel bed in sections based on a well-established procedure (Anderson and Rothermel 1965; Schuette 1965; Rothermel and Anderson 1966) and then placing the plants in predetermined locations. Composition and characteristics of the fuel bed components are available (Safdari et al. 2018; Matt et al. 2020). Fuel moisture content, fuel loading, ambient temperature and relative humidity in the wind tunnel varied between experiments. Pine needle dry mass ranged from 862 to 943 g, uniformly distributed over the fuel bed (Schuette 1965). The plants were placed in the fuel bed approximately 5 min before lighting the fire. Fuel beds were ignited with a line fire which spread the length of the fuel bed.
Two separate oxidative pyrolysis gas sampling setups were used. An array of eight stainless-steel sample tubes along the length of the fuel bed filled a sample canister with multiple small aliquots of oxidative pyrolysis gases resulting from the volatile matter for the GC/FID analysis. A separate sample tube and canister upwind of the oxidative pyrolysis tubes captured flaming emissions for comparison. Further detail on the experiments and measurement methods can be found elsewhere (Weise et al. 2022c). The flaming combustion samples are not included in this analysis. For the FTIR samples, two approaches were used (Scharko et al. 2019a; Banach et al. 2021). In the wind tunnel, a fixed stainless-steel probe located in the vicinity of the sample tube array captured gases emitted by the pyrolysing plant and pine needle fuels prior to the arrival of any combustion gases or the flame front. The gases were pumped into a long-path White cell (White 1942) housed inside the sample compartment of a Bruker Tensor 37 FTIR spectrometer. Two different sampling modes (dynamic - continuous gas flow through the White cell; static - gas flow into the White cell for a fixed time which was then valved shut) were used. Only the static samples (Banach et al. 2021) were used in the present analysis. Analysis of the dynamic samples is currently incomplete and will be the focus of a future analysis. Canister samples for FTIR analysis were collected in the field fires as described below.
Field fires
Five 0.1 ha experimental plots located at Ft. Jackson, SC in longleaf pine–slash pine forests (P. elliottii Engelm.) were burned in May 2018. The dominant understory shrub in these plots was sparkleberry (Hudak et al. 2020; Herzog et al. 2022). Further descriptions of the plots, fuel measurements and experimental burns are available (Scharko et al. 2019a; Hudak et al. 2020; Weise et al. 2022c). Real-time measurement of oxidative pyrolysis gases in an open-path environment using FTIR (Akagi et al. 2014) was not feasible so we collected canister samples. A 2.5 m gas sampling probe of 6 mm stainless-steel tubing with flexible stainless-steel tubing connecting to the sampling package captured the gases (Fig. 1), which we assume resulted from the volatilisation of the forest fuels. The field sampling package consisted of a swing Piston KNF Neuberger Pump,1 12-V gel cell rechargeable battery, stainless-steel tubing to a pressure relief valve and gauge. The flow rate to fill the canisters was 250 mL s−1. There were two identical canister sampling packages: one for 0.85 L SUMMA® canisters (172 kPa) for GC/FID analysis, and the second for 3 L SUMMA® canisters (138 kPa) for FTIR analysis (Scharko et al. 2019a). The sampling strategy was to identify plants along the edge of the plot that had enough foliage to ignite and allow the chance of sampling oxidative pyrolysis. As the flame front advanced, the probe was positioned ahead of the flame front as it approached. Air samples were acquired in close proximity to the base of the leading edge of approaching flame, taking short interval sample aliquots when it was likely that oxidative pyrolysis was occurring.
FTIR analysis of oxidative pyrolysis gases
In the static mode used for the field data, the gas was brought to the laboratory (typically on the same day), pumped from the SUMMA® canister and isolated in the White cell; the inlet/outlet valves were simultaneously closed such that the emitted gases were isolated in the cell at a desired pressure (~98.7–93.3 kPa for high pressures, and 57.3–53.3 kPa for lower pressure measurements). The goal of the static mode was to obtain a higher fidelity spectral ‘snapshot’ for a given point in time of the burn; more interferograms were averaged at higher spectral resolution thus allowing for detection of more gaseous species with higher sensitivity (Scharko et al. 2019a).
Twenty FTIR static samples were thus obtained (10 each from the wind tunnel and the field). The static experiment spectra were recorded using the full resolution of the spectrometer (0.6 cm−1), a 2 mm Jacquinot stop and double sided, forward–backward acquisition. The Tensor 37 FTIR was outfitted with a Ge/KBr beamsplitter, glow bar source and mercury cadmium telluride detector, providing data acquisition yielding spectra from 7500 to 500 cm−1. Due to the higher resolution requiring a smaller aperture and thereby lower light throughput, acquisition time was extended by averaging multiple scans for a full 30 min, resulting in vastly improved signal/noise ratios. For analysis of such complicated gas-phase mixtures, infrared spectral resolution of 1.0 cm−1 or better has been demonstrated to be advantageous (Burling et al. 2010; Akagi et al. 2014; Scharko et al. 2019a). The gas cell path-length was verified using purified isopropanol with a total of 10 spectra with neat gas pressures (MKS KF15 pressure transducer) ranging from 0.6 to 10.5 Torr. Resulting spectra were integrated from 3290 to 3515 cm−1 and using known cross-sections a Beer–Lambert plot was created from which the cell path-length was determined to be 6.5 ± 0.2 m. All infrared data were reduced using MALT5 software (Griffith 1996; Griffith et al. 2012) in which the experimental spectrum is fitted to a simulated spectrum; the mean-squared residual between the two is minimised, resulting in calculated absorption coefficients of each input species. These are quantified by comparing the cross sections to quantitative databases, such as the HITRAN line-by-line dataset (Gordon et al. 2017) or the PNNL 50°C gas-phase reference spectra (Sharpe et al. 2004; Kochanov et al. 2019) as input libraries. Using different spectral ‘microwindows’ for each species and taking care that none of the measured bands were saturated, the software is used to calculate a vapour-phase mixing ratio for each gas (Scharko et al. 2019b). In addition to the static samples, 10 dynamic samples were collected for the wind tunnel also; some results from the dynamic samples are presented elsewhere (Banach et al. 2021).
Gas chromatography of 0.85 L canisters
Background air samples were taken during the wind tunnel and field experiments in 0.85 L canisters and analysed, so the reported gas concentrations are excess mixing ratios (Ward and Radke 1993; Urbanski et al. 2008). The canister emission samples were analysed using EPA method TO-14A (EPA 1999) for CO2, CO, CH4 and C2 to C5 hydrocarbon gases with an Agilent model 7890 GC configured with two columns running simultaneously. A 3.175 mm diameter, 2 m Carbosphere™ packed column with a nickel catalyst methaniser was used for analysis of CO2 and CO using FIDs. The second column, a 0.53 mm diameter × 50 m length Agilent Al/S column, separated hydrocarbons and methane. Both columns went to FIDs and were run simultaneously. Chromatogram data were collected and processed using Agilent OpenLab software. A multipoint set of three standards bracketing the sample concentrations was analysed with each set of samples to construct a standard curve for each compound. Based on the integrated peak areas, the sample concentrations were calculated from the standard curves and written into a spreadsheet for analysis. Chromatograms of National Institute of Standards and Technology standard reference material gas standards for CO, CO2, CH4 and propane were acquired each day to validate the standard curve. Duplicate GC runs of canisters were performed for each sixth sample. A Trace Analytical RGA3 reduction gas analyser was used to measure H2 concentrations – this is a chromatographic instrument with a molecular sieve column and a UV mercuric oxide detector that provides highly sensitive precise measurement of trace level H2. The target range is 0–10 ppm H2; most samples were diluted with ultra-high purity nitrogen to be in this range. An H2 standard (Scott Specialty Gases) was used for calibration. Chromatograms of H2 from this instrument were collected and integrated with Agilent OpenLab software interfaced to the instrument. On a subset of canisters with significant detectable oxidative pyrolysis gases, GC/MS analysis was performed using an Agilent 6890 GC with a HP-5 0.320 mm × 30 m column, helium carrier gas and Agilent 4590N MS detector. The composition of the gases in 204 canisters was analysed for the wind tunnel and field burns.
Statistical methods
For the FTIR data, a combination of software was used for the post-acquisition spectral analysis and confirmation of the species observed during the campaign. The original MALT software (Multiple Atmospheric Layer Transmission) used classic least squares to create linear combinations of single-component spectra that minimised the difference between the measured and simulated spectra by adjusting mixing ratios (Griffith 1996). The MALT5 version uses a nonlinear least-squares algorithm (Griffith et al. 2012) and both HITRAN line-by-line data (Gordon et al. 2017) and the PNNL 50°C gas-phase reference spectra (Johnson et al. 2006, 2010; Kochanov et al. 2019) as input libraries to identify and quantify vapour-phase chemicals in the spectra. Spectra were compiled into parameter files and analysed using parameters including pressure, temperature, pathlength, resolution, as well as estimated initial values for chemical mixing ratios. To confirm that the chemical species were actually present, each spectrum generated by MALT5 was input to OPUS (Bruker Corporation LLC 2020) and subtracted from the measured spectrum; the target compound was purposefully omitted from the subtraction process to visually inspect if the omitted compound was present in the spectrum.
Atmospheric chemistry data which include smoke and pyrolysis gases are compositional data (e.g. Bandeen-Roche 1994; Engle et al. 2011; Jarauta-Bragulat et al. 2016; Gibergans-Baguena et al. 2020; Weise et al. 2020a, 2020b) and were analysed as such using the R system for statistical computing v4.1.3 (https://www.R-project.org/). The CoDA relies on log-ratio coordinate representations of the data and, hence, missing and zero values in the data set are problematic. The data were preprocessed using the multLN function in the zCompositions R package to impute random values below the minimum observed concentrations (Palarea-Albaladejo and Martín-Fernández 2013, 2015) for those instances where the gas concentration was below the detection limit (BDL) of the measuring instrument because this method provided results comparable to more complex imputation methods. Summary measures are defined for compositional data such as the centre (mean), total variance, log-ratio mean and variance (van den Boogaart and Tolosana-Delgado 2013). We calculated the log-ratio means for all gas pairs because the log-ratio can be used to examine the proportionality between each pair of gases (Lovell et al. 2015; Egozcue et al. 2018).
A proportionality measure ρ for compositional data, similar to the more familiar Pearson correlation coefficient r, ranges over [−1, 1] with one indicating perfect proportionality (Erb and Notredame 2016). Proportionality between each pair of gases was estimated for the FTIR and GC/FID data using the propr R package (Lovell et al. 2015; Erb and Notredame 2016; Quinn et al. 2017, 2018, 2019). Because ρ is the same for absolute (original) and relative forms of the gas compositions, it is a precise measure of correlation in the original data. Only positive values of ρ were considered to indicate proportionality based on the approach proposed by Egozcue et al. (2018). Since proportionality between D(D − 1)/2 gas pairs, where D is the number of gases, was tested for significance, the value of ρ was adjusted using the procedure updateCutoffs in propr to keep the false discovery rate (α) less than 0.05 (Benjamini and Hochberg 1995).
Seventeen scientifically meaningful comparisons between subgroups of gases were represented by so-called log-ratio balances, or simply balances, which are a special class of log-ratio coordinates where the log-ratio term compares the geometric mean of rk parts in one subset (in the numerator, indicated with + symbol) with the geometric mean of sk parts in another subset (in the denominator, indicated with − symbol). The general expression of the kth balance is given as follows:
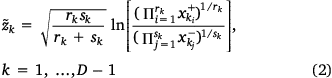
The preceding multiplicative factor is a normalisation factor formally required to ensure comparability of the balances and ensure orthonormality of the resulting coordinate system. A simpler expression as the normalised difference between the means of log-transformed data is given as follows:

Scientifically meaningful balances were formulated by defining an adequate sequential binary partition of the original composition (Egozcue and Pawlowsky-Glahn 2005) (Table A1).
The FTIR dataset consisted of 27 gases identified by FTIR and 27 observations (22 wind tunnel and five field), which yielded a dataset of 27 observations and 26 balances. The assumption of multivariate normality is required for many multivariate methods and several different tests have been developed. Similarly, many univariate tests require univariate normality. We applied several multivariate normality tests to see if there was agreement between the different tests. The Mardia, Henze–Zirkler, Royston and Energy tests for multivariate normality as implemented in the MVN R package (Korkmaz et al. 2014) indicated that distribution of these balances was not multivariate normal (Table A2). The univariate Shapiro–Wilk (Royston 1982) and Kolmogorov–Smirnov (Conover 1999) tests indicated that several of the distributions of the individual balances were not normal (Table A3). Tests of equality of the covariance matrices for the wind tunnel and field as implemented in the covTestR R package (Barnard and Young 2018) yielded contradictory results: two tests rejected the null hypothesis of equality (Schott 2007; Srivastava et al. 2014) and two did not (Srivastava 2007; Srivastava and Yanagihara 2010). For these reasons, permutational multivariate analysis of variance (PERMANOVA) (Anderson 2001, 2017) was used to statistically test the potential effect of sampling location (wind tunnel and field) on the mean relative composition of gases using the adonis function in the vegan R package (Oksanen et al. 2020). The Kruskal–Wallis rank sum test (Hollander et al. 2014), a nonparametric analogue of univariate ANOVA, compared the average balances between wind tunnel and field. The resulting P-values were adjusted to account for the multiple testing using the method of Benjamini and Hochberg (1995).
Seven balances were derived for the subcomposition of common gases. The univariate tests indicated that six of the seven balances were not normally distributed. Tests of equality of the covariance matrices for the subcomposition were also contradictory as above. As a group, the seven balances were not multivariate normal. The univariate Shapiro–Wilk test showed that six of seven balances were not univariate normal (Table A4). The PERMANOVA tested the effects of analytical technique, location (wind tunnel, field) and their interaction on the subcomposition. The Kruskal–Wallis test compared the average balances between location and analytical technique (FTIR and GC/FID) individually. The resulting P-values were also adjusted to account for the multiple testing (Benjamini and Hochberg 1995).
Results
Summary statistics
To facilitate meaningful comparison of the two analytical techniques, all gases that were missing from more than 60% of the observations were removed from the GC/FID and FTIR compositions. This resulted in the removal of ethanol, N2O, benzene, crotonaldehyde, isocyanic acid, NO, NO2 and sulfur dioxide from the FTIR samples and hexane, 1-hexene, 1-heptane, benzene, toluene, furan and furfural from the GC/FID samples (Table 1). The wind tunnel and field fires yielded 88 and seven GC/FID samples containing 22 gases, respectively. These same fires yielded 22 wind tunnel and five field FTIR samples containing 27 gases, respectively. Water vapour and CO2 were the two gases with the largest concentrations in the FTIR samples (Table 1); CO2 also dominated the GC/FID samples followed by CO. The geometric mean concentrations estimated using FTIR for CO2 were 15 272 and 46 229 ppm in the RFL wind tunnel and at Ft. Jackson, respectively. The GC/FID CO2 concentrations were an order of magnitude smaller. The concentrations of the other gases measured by GC/FID were generally smaller than the concentrations measured by FTIR. The original concentrations were summarised as geometric mean ⋇ geometric standard deviation. The geometric counterparts to the ordinary ‘arithmetic’ measures are adequate when the data are ratios to some reference amount (Fleming and Wallace 1986). The geometric standard deviation is related to the geometric mean by multiplication and division (Butler et al. 2020) indicated by ‘⋇’.
FTIR data
The sign of a log-ratio indicates which part of the ratio is relatively larger: negative values indicate the numerator is smaller than the denominator. The relative dominance of H2O, CO2, CO and CH4 in the wind tunnel and field compositions is indicated by their log-ratios with other gases being negative (Table 2) meaning that the relative amount of the other gas is smaller. In the CoDA context, perfect proportionality indicates that the log-ratio between two parts of a composition is constant, meaning that the relative amounts of the two parts are constant. The hypothesis of perfect proportionality between FTIR gas pairs was not rejected for 13 pairs in the wind tunnel data and a single pair for the Ft. Jackson samples, suggesting that these pairs may be proportional. Allene was proportional to acetylene in the field data, but not in the wind tunnel data. In the wind tunnel, HONO was proportional to both CO2 and CO while CO was also proportional to acetylene and ethene. The last observation is consistent because the presence of CO is usually associated with incomplete combustion. Isoprene was proportional with propene and 1,3-butadiene; also understandable as all three alkenes are found in concomitant ratios before combustion occurs (Brauer et al. 2014). For comparison, in the GC/FID dataset, the test for perfect proportionality was not rejected for 59 pairs of gases (Table 3) in the wind tunnel; only a single test was not rejected in the field data. Acetylene and 1,3-butadiene were each proportional with nine other gases. As with ordinary correlation, the statistical test of the null hypothesis of perfect proportionality is affected by the variance of the log-ratio, so increased variation makes proportionality (and correlation) more difficult to detect.
![]() |
![]() |
The PERMANOVA on log-ratio coordinates indicated a significant difference in mean FTIR gas compositions between the wind tunnel and the field (Table 4). Note that the results from this overall test are by construction independent of the log-ratio coordinate representation chosen for the original composition. However, to understand where the differences may arise, the 17 median values of the balances resulting from the sequential binary partition of the composition are shown in Fig. 2 and the details of the gases contained in the balances are found in Table A1. In the following sections the term ‘amount’ will be used for convenience – it is important to recall that the comparisons of what were originally mixing ratios are conducted in the space of log-ratio coordinates.
![]() |
![]() |
The amount of H2O and CO2 relative to all other gases and the amount of H2O relative to CO2 were greater in the wind tunnel than the field. The reported concentrations were already adjusted to account for the ambient atmosphere, so this difference in H2O is not due to differences in atmospheric humidity between the two locations, i.e. it is the excess H2O due to the fire, similarly for CO and CO2. Longleaf pine needle fuel moisture-content was comparable between wind tunnel and field fires and the live foliage moisture-content was approximately 100% and 200% in the wind tunnel and field fires, respectively (Weise et al. 2022c). The cause of the differences in the relative amounts may be proximity of the pyrolysing foliage to the sample probe (0.1 m in the wind tunnel versus 1 m in the field fires) or due to the size difference of the nursery and field plants.
More CO and light hydrocarbons were observed at Ft. Jackson relative to other pyrolysis gases. However, the relative amounts of CO and light hydrocarbons (alkanes, alkenes and alkynes) did not differ between the wind tunnel and Ft. Jackson, suggesting that the greater amount of CO and light hydrocarbons at Ft. Jackson is due to smaller relative amounts of the other gases. There were no significant differences in the relative amounts within the light hydrocarbon gases between the wind tunnel and Ft. Jackson fires.
The amount of nitrogen-bearing gases (methyl nitrite, NH3, HCN and HONO) relative to the other volatile organic compounds (VOC, not including light hydrocarbons) was smaller at Ft. Jackson than in the wind tunnel. More HONO and NH3 relative to HCN and methyl nitrite were observed in the wind tunnel fires. While we previously speculated that methyl nitrite’s presence at Ft. Jackson may have been associated with munitions (Scharko et al. 2019a), the amount of methyl nitrite relative to HCN did not differ significantly between the wind tunnel and field. It is important to note that 40% of the observations of methyl nitrite were BDL in contrast to 6% of HCN observations (Table 1). The relative amount of nitrogen present in the fuels may have differed because nursery plants were used in the wind tunnel as opposed to the native vegetation combusted at Ft. Jackson; this is even more likely if the nursery plants were fertilised. Similarly, the nitrogen content of the longleaf pine needles may have varied between the locations where the pine needles were harvested and the stands at Ft. Jackson. Fertilisation with nitrogen is a practice used in longleaf pine sites that are managed for pine straw production (Ludovici et al. 2018). No elemental analysis of the different fuels was performed so this is purely speculative. More HONO relative to NH3 was observed at Ft. Jackson. Sekimoto et al. (2018) associated HONO with a high-temperature pyrolysis profile and NH3 with a low-temperature pyrolysis profile. Based on this, our results suggest that more of the Ft. Jackson data resulted from high-temperature oxidative pyrolysis than the wind tunnel data. Maximum fuel temperatures observed below the FTIR probe in the wind tunnel ranged within 132–660°C and leaf temperatures at Ft. Jackson were 231–648°C (Weise et al. 2022c), which does not support differing pyrolysis temperature profiles in these data.
The amount of phenol relative to the other VOCs was greater in the wind tunnel (Banach et al. 2021). We previously reported difficulty in observing phenol and phenolic compounds at Ft. Jackson due to their infrared bands being somewhat weak and obscured by a number of other species, namely acetic acid, carbon dioxide, acetylene and hydrogen cyanide (Scharko et al. 2019a). This difficulty was reflected in the small balance value for Ft. Jackson. Results from bench-scale measurements of phenol produced in pyrolysis by different heating modes showed that after CO2, H2 and CO, more phenol relative to the remaining 84 gases was measured and plant species and moisture content affected the amount of tars relative to phenol (Weise et al. 2022b). More furan relative to naphthalene and more acetaldehyde relative to formaldehyde was observed in the oxidative pyrolysis gases at Ft. Jackson compared to the wind tunnel. The ratio of furan to acetylene has also been suggested as a possible measure to distinguish between high- and low-temperature pyrolysis factors (Sekimoto et al. 2018). Sekimoto et al. defined two factors that accounted for ‘much of the observed variability in VOCs’ as high- and low-temperature pyrolysis factors; air temperature was measured by an FTIR instrument at a sampling inlet after cooling and mixing with ambient air occurred. Our earlier analysis of this ratio suggested that high-temperature pyrolysis was the dominant process associated with the generation of gases at Ft. Jackson (Scharko et al. 2019a). That work and the work of Sekimoto et al. (2018) did not analyse the gas measurements as compositional data. Testing the furan to acetylene ratio could occur in the context of a different set of balances. We did not test whether this specific ratio differed between the wind tunnel and field. The log-ratios were very similar (Table 2), suggesting the possibility of no difference between the wind tunnel and Ft. Jackson. It may be possible to further explore this using the wind tunnel dynamic FTIR data coupled with heat flux and temperature data as in Banach et al. (2021).
Common gases
The gases common to the GC/FID and FTIR samples were CO, CO2, CH4, C2H2, C2H4, C3H6, C4H6 and isobutene (C4H8). Bearing in mind that the aliquots of gases were collected close in time, but not from the same aliquot of air, the trends in the relative amounts of the gases by the two methods were quite similar (Fig. 3). However, the relative amount of CO measured by FTIR at Ft. Jackson was an order of magnitude larger than the wind tunnel or GC/FID measurements. The PERMANOVA showed that the GC/FID and FTIR methods had a significant effect on the relative composition of the common gases (Table 5) but the location and interaction effects were not significant.
![]() |
![]() |
The median values of the four combinations of location and method and the overall median were calculated for each balance (Fig. 4) for the common gases. The analytical method significantly affected six of the seven balances as indicated by the Kruskal–Wallis test. The first two balances containing CO2 were smaller for the FTIR measurements at Ft. Jackson; relatively less CO2 and more CO and CH4 were measured, resulting in smaller balances. The FTIR measured more CO relative to CH4 than GC/FID; however, relatively more CH4 was measured by GC/FID in the field burns. The FTIR balance values were smaller than GC/FID for alkenes vs acetylene. The amount of ethene relative to the other alkenes did not differ between analytical methods. However, the amount of 1,3-butadiene relative to the C4 alkenes was greater in the FTIR samples. The propene vs isobutene balance suggested that more propene relative to isobutene was present in the FTIR samples compared to the GC/FID.
![]() |
Discussion
In the air pollution and smoke communities, emission ratios (ERs) are commonly analysed measures, perhaps starting with the work of Darley et al. (1966). Early air pollution and biomass smoke studies used linear regression to adjust laboratory and field gas measurements to common CO2 values in order to compare other gas concentrations (Boubel et al. 1969). The use of linear regression in this instance assumed a correlative relationship between the gases. More recent work has used ERs with CO to link laboratory and field experiments (Yokelson et al. 2013). In seminal work, Crutzen et al. (1979) examined CO2 ERs and reported ‘uniform CO/CO2’ ratios and that other ERs did not deviate by ‘more than a factor of six’. A ‘factor of six’ suggests that the ER may not be constant, i.e. the concentrations of the two gases may not be proportional or correlated. At the base of the wealth of literature that has examined ERs is the assumption that correlation is an appropriate statistical measure to use on these data and that ratios are an appropriate transformation to apply to the original data. The use of ERs comes close to the statistically appropriate handling of these data. If an entire composition of gases (x) is expressed as ER relative to CO2, i.e. , taking the logarithm of these ratios is equivalent to applying the so-called additive log-ratio (alr) transformation (Aitchison 1986; Weise et al. 2022b) and has been used in other atmospheric chemistry studies (Jarauta-Bragulat et al. 2016; Gibergans-Baguena et al. 2020). Although useful in parametric statistical modelling, the alr is not particularly adequate when distances, angles or shapes are involved (e.g. when performing clustering analysis or multidimensional scaling) as it deforms these (Aitchison 1986; Aitchison et al. 2000; van den Boogaart and Tolosana-Delgado 2013). An ER can be derived from any log-ratio x contained in Tables 2 and 3 by exp(x); for instance ERCH4(C2H2) = exp(−1.5) = 0.22 for FTIR in the wind tunnel (Table 2).
Correlation and linear regression are frequently used to examine the internal relationships between different gases or between the gases and external variables (such as fire phase, fuel type and fuel moisture). Ideally, the relative relationships between pyrolysis gases should not be affected by the method used to identify the gases. Gases that are proportional in one method should be proportional in another method. If the proportionality between a pair of gases is known, it would be possible to only measure one of the pair of gases. In the present study, only a small fraction (13/351) of the possible pairs of gases measured in the wind tunnel using FTIR were identified as proportional; only five gases were proportional with CO or CO2. This may be a surprising result based on previous studies that correlated many gases with CO or CO2. It is important to recall that in those previous studies, the use of correlation to examine the relationships ignored the relative nature of the data. The data from those studies could be reanalysed using proportionality to examine the relationships of combustion gases with CO and CO2. In the field measurements at Ft. Jackson, only one of 351 pairs was proportional. For the GC/FID oxidative pyrolysis data, 25% (59/231) and 1/231 of the pairs were proportional in the wind tunnel and Ft. Jackson data, respectively. Of the 59 pairs, 36 were proportional in the wind tunnel GC/FID flaming combustion data reported previously (Weise et al. 2022c). Proportionality is a measure based on the variability of the log-ratio variance; relatively large variance does not support proportionality. Fewer proportional pairs might suggest that the data measured using FTIR were more variable than the GC/FID data and that the Ft. Jackson field measurements were more variable than the wind tunnel measurements. However, this observation is based on gas compositions that have only nine gases in common. The variability could also be a natural characteristic of the gases emitted during the oxidative pyrolysis process. The potential increase in variability from the wind tunnel setting to the field setting is not surprising. Dimitrakopoulos (2001) argued for the use of tightly controlled bench-scale non-oxidative pyrolysis measurements due to the chaotic variability present in the fire environment. It is currently not possible to compare the current data to other data from similar studies to assess the quality of the data as these are the first such data describing pyrolysis gases in a fire setting. We sought to control as much variability as possible through the use of common sampling methods, replication of experiments, simplification of fuels and similar environmental conditions to produce quality data. Future scientists can use these data as a point of comparison to determine what the natural variability of gas composition is. It is important that physically-based fire models also incorporate this variability in composition instead of assuming that the composition is constant. However, we have recently shown using CoDA techniques that measurements made in simplified and controlled wind tunnel experiments can be related to field measurements (Weise et al. 2022c).
The balances associated with the FTIR data from the wind tunnel and Ft. Jackson suggested some differences in the relative amounts of various gases. The cause for the differences between the wind tunnel and Ft. Jackson may be due to the sampling probe location differences, insufficient sample size or greater natural variability. The relative amount of nitrogen content in the fuels may have differed due to different cultural practices used in commercial nurseries, pine stands managed for pine straw production and pine stands managed by the Department of Defence.
Direct comparison of gas measurements in a fire environment produced with different instruments is challenging and influenced by many factors. In prior work and the current study, 57 gases associated with wildland fire (Johnson et al. 2010; Weise et al. 2015; Scharko et al. 2019b) were added to the PNNL spectral library. Although 57 is small compared to the hundreds of compounds that can be identified using other methods such as GC-FID, FTIR is a viable technique for wildland fire. Different instruments and analytical techniques may measure the same gases with differing resolution or are unable to detect the same compounds (Ward and Radke 1993). A wide variety of instrumentation is often deployed to measure a large suite of compounds (e.g. Yokelson et al. 2013). We previously noted that different methods influence the relative abundance of measured emissions (Weise et al. 2015) even though CoDA was not used in this earlier study.
The present study used two methods readily adapted to field use. The inherent spatial, temporal and compositional variability in fuels as well as the non-steady conditions under which the fuels were heated can affect the composition of the pyrolysis and combustion products. Some of this variability can be controlled by using a common sample line or assuming that sample collection points in proximity are true replicates. The wind tunnel fuel beds were constructed so that the amount and depth of longleaf pine needles was uniform and the density of the uniformly distributed plants was increased due to the plants’ small size in order to produce a detectable concentration of gases. The distribution of both pine needles and live plants in the field was not uniform and contained additional fuel types. Using more uniform field sites such as pine plantations instead of natural stands in future experiments may reduce variability. Using more sensitive instrumentation that is field-hardened and sampling closer to the live foliage may improve measurements; however, the mixture of gases sampled in the wind tunnel resulted from live and dead fuels in close proximity which may be difficult to achieve when measuring mature plants in a field setting. Performing elemental analysis to enable carbon and nitrogen balances is another improvement that could be used in future experiments. As the elemental makeup of plants is also compositional data, log-ratios of the different elements should be used.
In the present study, the assumption was made that samples taken in proximity to each other in wind tunnel fuel beds or in small prescribed burns in natural fuels each represented true replicates. In three of the four (location × method) combinations, samples were collected in canisters for subsequent analysis, either later in the day (FTIR) or several weeks after collection (GC/FID). The wind tunnel FTIR spectroscopic measurements were collected in real time. The SUMMA® canisters used for sample collection and storage have been shown to be reliable storage containers for up to 32 weeks and are used routinely to collect fire gas samples (Evans et al. 1998; Austin et al. 2001; Wang et al. 2014; Strand et al. 2016).
While all these factors may affect the absolute values for the oxidative pyrolysis gases collected, it is the relative values that provide the relevant information since these are compositional data. Based on the subcomposition of gases measured by both FTIR and GC/FID, the analytical method did significantly affect relative composition of oxidative pyrolysis gases based on the PERMANOVA while the fire location (wind tunnel versus field) effect was not significant. The dominance of the primary pyrolysis gases (CO2, CO and CH4) relative to the light hydrocarbon trace gases differed between methods. Future experiments using instrumentation with a greater number of common gases could be used to further evaluate the applicability of wind-tunnel scale results to field fires. In pyrolysis models within physics-based fire models, these three primary gases are the ones often used (e.g. Westbrook and Dryer 1981; Zhou and Mahalingam 2001; Di Blasi 2008). The balance between CO2 and the fuel gases (CO and CH4) and the relative amount of CO to CH4 did not differ between locations. This potential lack of difference for the dominant pyrolysis gases is encouraging and may support the use of relatively simple oxidative pyrolysis models in physics-based wildland fire models as in Borujerdi et al. (2022). Application of common statistical methods to meaningful log-ratio coordinate representations of compositional data conveying relative information ensures that the results are not an artifact of the presence or absence of a gas in the composition. A future manuscript will compare the composition of pyrolysis gases to determine if it is possible to link the non-oxidative bench-scale results with the oxidative pyrolysis fire-scale results. Lastly, another future manuscript will compare the changes in composition of gases measured over time using dynamic FTIR as described in Banach et al. (2021).
Conclusions
Oxidative pyrolysis gases were successfully measured in a wind tunnel and in small field prescribed burns using two different methods: FTIR-spectroscopy and GC/FID analysis. Using CoDA techniques for the FTIR data, the composition of wind tunnel and field oxidative pyrolysis gases differed. In the wind tunnel setting, use of FTIR enabled the measurement of instantaneous samples of gases that were not measured by the GC/FID setup used. Although not presented here, FTIR can also provide dynamic description of the changing composition of gases as fuels heat, pyrolyse and combust.
Gases potentially proportional to each other were identified for both methods; however, the number of proportional pairs differed markedly due to the observed variability in part. The subcomposition of gases measured by both methods showed that the compositions were affected by the measurement method but not by location. The subcomposition differed in the relative amounts of the trace gases. The relative amount of the two primary fuel gases (CO and CH4) was significantly affected by the analytical method. Although the composition of trace gases differed between the analytical methods, from a fire modelling perspective, each method yielded comparable description of the primary fuel gases, providing independent verification of measurements. While the importance of the trace gases in combustion models remains to be determined, complementary measurement methods such as FTIR and GC/FID can provide characterisation of a larger set of compounds than each method separately. This study provided measurements of the compositional variability associated with oxidative pyrolysis gases that can be used in future comparisons.
Data availability
Once approved for release by the sponsor of this study (SERDP), the data will be available through the Forest Service Research Data Archive https://www.fs.usda.gov/rds/archive/.
Conflicts of interest
The authors declare no conflicts of interest.
Declaration of funding
This work was supported by the DOD/DOE/EPA Strategic Environmental Research and Development Program project RC-2640. JPA was supported by the project PID2021-123833OB-I00, funded by the Spanish Ministry of Science and Innovation (MCIN/AEI/10.13039/501100011033) and by ERDF A way of making Europe. USDA Forest Service annual research appropriations provided in-kind support from 2016 to 2022.
Acknowledgements
This article has been contributed to by US Government employees as part of their official duties and accordingly, their work is in the public domain in the USA.
References
Aitchison J (1982) The statistical analysis of compositional data. Journal of the Royal Statistical Society: Series B (Methodological) 44, 139–160.| The statistical analysis of compositional data.Crossref | GoogleScholarGoogle Scholar |
Aitchison J (1986) ‘The statistical analysis of compositional data.’ (Chapman and Hall: London, New York)
Aitchison J, Barceló-Vidal C, Martín-Fernández JA, Pawlowsky-Glahn V (2000) Logratio analysis and compositional distance. Mathematical Geology 32, 271–275.
| Logratio analysis and compositional distance.Crossref | GoogleScholarGoogle Scholar |
Akagi SK, Yokelson RJ, Wiedinmyer C, Alvarado MJ, Reid JS, Karl T, Crounse JD, Wennberg PO (2011) Emission factors for open and domestic biomass burning for use in atmospheric models. Atmospheric Chemistry and Physics 11, 4039–4072.
| Emission factors for open and domestic biomass burning for use in atmospheric models.Crossref | GoogleScholarGoogle Scholar |
Akagi SK, Burling IR, Mendoza A, Johnson TJ, Cameron M, Griffith DWT, Paton-Walsh C, Weise DR, Reardon J, Yokelson RJ (2014) Field measurements of trace gases emitted by prescribed fires in southeastern US pine forests using an open-path FTIR system. Atmospheric Chemistry and Physics 14, 199–215.
| Field measurements of trace gases emitted by prescribed fires in southeastern US pine forests using an open-path FTIR system.Crossref | GoogleScholarGoogle Scholar |
Alessio GA, Peñuelas J, Llusià J, Ogaya R, Estiarte M, De Lillis M (2008) Influence of water and terpenes on flammability in some dominant Mediterranean species. International Journal of Wildland Fire 17, 274
| Influence of water and terpenes on flammability in some dominant Mediterranean species.Crossref | GoogleScholarGoogle Scholar |
Amini E, Safdari M-S, DeYoung JT, Weise DR, Fletcher TH (2019a) Characterization of pyrolysis products from slow pyrolysis of live and dead vegetation native to the southern United States. Fuel 235, 1475–1491.
| Characterization of pyrolysis products from slow pyrolysis of live and dead vegetation native to the southern United States.Crossref | GoogleScholarGoogle Scholar |
Amini E, Safdari M-S, Weise DR, Fletcher TH (2019b) Pyrolysis kinetics of live and dead wildland vegetation from the southern United States. Journal of Analytical and Applied Pyrolysis 142, 104613
| Pyrolysis kinetics of live and dead wildland vegetation from the southern United States.Crossref | GoogleScholarGoogle Scholar |
Anderson MJ (2001) A new method for non-parametric multivariate analysis of variance. Austral Ecology 26, 32–46.
| A new method for non-parametric multivariate analysis of variance.Crossref | GoogleScholarGoogle Scholar |
Anderson MJ (2017) Permutational Multivariate Analysis of Variance (PERMANOVA). In ‘Wiley StatsRef: Statistics Reference Online’. (Eds N Balakrishnan, T Colton, B Everitt, W Piegorsch, F Ruggeri, JL Teugels) pp. 1–15. (John Wiley & Sons, Ltd: Chichester, UK)
| Crossref |
Anderson HE, Rothermel RC (1965) Influence of moisture and wind upon the characteristics of free-burning fires. Symposium (International) on Combustion 10, 1009–1019.
| Influence of moisture and wind upon the characteristics of free-burning fires.Crossref | GoogleScholarGoogle Scholar |
Andreae MO, Merlet P (2001) Emission of trace gases and aerosols from biomass burning. Global Biogeochemical Cycles 15, 955–966.
| Emission of trace gases and aerosols from biomass burning.Crossref | GoogleScholarGoogle Scholar |
Austin CC, Wang D, Ecobichon DJ, Dussault G (2001) Characterization of volatile organic compounds in smoke at experimental fires. Journal of Toxicology and Environmental Health, Part A 63, 191–206.
| Characterization of volatile organic compounds in smoke at experimental fires.Crossref | GoogleScholarGoogle Scholar |
Banach CA, Bradley AM, Tonkyn RG, Williams ON, Chong J, Weise DR, Myers TL, Johnson TJ (2021) Dynamic infrared gas analysis from longleaf pine fuel beds burned in a wind tunnel: observation of phenol in pyrolysis and combustion phases. Atmospheric Measurement Techniques 14, 2359–2376.
| Dynamic infrared gas analysis from longleaf pine fuel beds burned in a wind tunnel: observation of phenol in pyrolysis and combustion phases.Crossref | GoogleScholarGoogle Scholar |
Bandeen-Roche K (1994) Resolution of additive mixtures into source components and contributions: a compositional approach. Journal of the American Statistical Association 89, 1450–1458.
| Resolution of additive mixtures into source components and contributions: a compositional approach.Crossref | GoogleScholarGoogle Scholar |
Barnard B, Young D (2018) covTestR: Covariance Matrix Tests. Available at https://CRAN.R-project.org/package=covTestR
Benjamini Y, Hochberg Y (1995) Controlling the false discovery rate: a practical and powerful approach to multiple testing. Journal of the Royal Statistical Society: Series B (Methodological) 57, 289–300.
| Controlling the false discovery rate: a practical and powerful approach to multiple testing.Crossref | GoogleScholarGoogle Scholar |
Borujerdi PR, Shotorban B, Mahalingam S, Weise DR (2022) Influence of pyrolysis gas composition and reaction kinetics on leaf-scale fires. Combustion Science and Technology
| Influence of pyrolysis gas composition and reaction kinetics on leaf-scale fires.Crossref | GoogleScholarGoogle Scholar |
Boubel RW, Darley EF, Schuck EA (1969) Emissions from burning grass stubble and straw. Journal of the Air Pollution Control Association 19, 497–500.
| Emissions from burning grass stubble and straw.Crossref | GoogleScholarGoogle Scholar |
Brauer CS, Blake TA, Guenther AB, Sharpe SW, Sams RL, Johnson TJ (2014) Quantitative infrared absorption cross sections of isoprene for atmospheric measurements. Atmospheric Measurement Techniques 7, 3839–3847.
| Quantitative infrared absorption cross sections of isoprene for atmospheric measurements.Crossref | GoogleScholarGoogle Scholar |
Bruker Corporation LLC (2020) OPUS software, v. 5.5. (Billerica, MA, USA)
Burling IR, Yokelson RJ, Griffith DWT, Johnson TJ, Veres P, Roberts JM, Warneke C, Urbanski SP, Reardon J, Weise DR, Hao WM, de Gouw J (2010) Laboratory measurements of trace gas emissions from biomass burning of fuel types from the southeastern and southwestern United States. Atmospheric Chemistry and Physics 10, 11115–11130.
| Laboratory measurements of trace gas emissions from biomass burning of fuel types from the southeastern and southwestern United States.Crossref | GoogleScholarGoogle Scholar |
Butler BM, Palarea-Albaladejo J, Shepherd KD, Nyambura KM, Towett EK, Sila AM, Hillier S (2020) Mineral–nutrient relationships in African soils assessed using cluster analysis of X-ray powder diffraction patterns and compositional methods. Geoderma 375, 114474
| Mineral–nutrient relationships in African soils assessed using cluster analysis of X-ray powder diffraction patterns and compositional methods.Crossref | GoogleScholarGoogle Scholar |
Chetehouna K, Barboni T, Zarguili I, Leoni E, Simeoni A, Fernandez-Pello AC (2009) Investigation on the emission of volatile organic compounds from heated vegetation and their potential to cause an accelerating forest fire. Combustion Science and Technology 181, 1273–1288.
| Investigation on the emission of volatile organic compounds from heated vegetation and their potential to cause an accelerating forest fire.Crossref | GoogleScholarGoogle Scholar |
Christian TJ, Kleiss B, Yokelson RJ, Holzinger R, Crutzen PJ, Hao WM, Shirai T, Blake DR (2004) Comprehensive laboratory measurements of biomass-burning emissions: 2. First intercomparison of open-path FTIR, PTR-MS, and GC-MS/FID/ECD. Journal of Geophysical Research 109, D02311
| Comprehensive laboratory measurements of biomass-burning emissions: 2. First intercomparison of open-path FTIR, PTR-MS, and GC-MS/FID/ECD.Crossref | GoogleScholarGoogle Scholar |
Collard F-X, Blin J (2014) A review on pyrolysis of biomass constituents: mechanisms and composition of the products obtained from the conversion of cellulose, hemicelluloses and lignin. Renewable and Sustainable Energy Reviews 38, 594–608.
| A review on pyrolysis of biomass constituents: mechanisms and composition of the products obtained from the conversion of cellulose, hemicelluloses and lignin.Crossref | GoogleScholarGoogle Scholar |
Conover WJ (1999) ‘Practical nonparametric statistics.’ (Wiley: New York)
Crutzen PJ, Brauch HG (Eds) (2016) ‘Paul J. Crutzen: a pioneer on atmospheric chemistry and climate in the anthropocene.’ (Springer: Zürich)
Crutzen PJ, Heidt LE, Krasnec JP, Pollock WH, Seiler W (1979) Biomass burning as a source of atmospheric gases CO, H2, N20, NO, CH3Cl and COS. Nature 282, 253–256.
| Biomass burning as a source of atmospheric gases CO, H2, N20, NO, CH3Cl and COS.Crossref | GoogleScholarGoogle Scholar |
Darley EF, Burleson FR, Mateer EH, Middleton JT, Osterli VP (1966) Contribution of Burning of Agricultural Wastes to Photochemical Air Pollution. Journal of the Air Pollution Control Association 16, 685–690.
| Contribution of Burning of Agricultural Wastes to Photochemical Air Pollution.Crossref | GoogleScholarGoogle Scholar |
Di Blasi C (2008) Modeling chemical and physical processes of wood and biomass pyrolysis. Progress in Energy and Combustion Science 34, 47–90.
| Modeling chemical and physical processes of wood and biomass pyrolysis.Crossref | GoogleScholarGoogle Scholar |
Dimitrakopoulos AP (2001) Thermogravimetric analysis of Mediterranean plant species. Journal of Analytical and Applied Pyrolysis 60, 123–130.
| Thermogravimetric analysis of Mediterranean plant species.Crossref | GoogleScholarGoogle Scholar |
Egozcue JJ, Pawlowsky-Glahn V (2005) Groups of parts and their balances in compositional data analysis. Mathematical Geology 37, 795–828.
| Groups of parts and their balances in compositional data analysis.Crossref | GoogleScholarGoogle Scholar |
Egozcue JJ, Pawlowsky-Glahn V, Gloor GB (2018) Linear association in compositional data analysis. Austrian Journal of Statistics 47, 3
| Linear association in compositional data analysis.Crossref | GoogleScholarGoogle Scholar |
Engle M, Martín-Fernández JA, Geboy N, Olea RA, Peucker-Ehrenbrink B, Kolker A, Krabbenhoft D, Lamothe P, Bothner M, Tate M (2011) Source apportionment of atmospheric trace gases and particulate matter: comparison of log-ratio and traditional approaches. In ‘Proceedings of the 4th International Workshop on Compositional Data Analysis’, Girona, Spain. p. 10. (Universitat de Girona: Girona, Spain) Available at http://hdl.handle.net/10256/13626
EPA (1999) Compendium Method TO-14A Determination Of Volatile Organic Compounds (VOCs) In Ambient Air Using Specially Prepared Canisters With Subsequent Analysis By Gas Chromatography. In ‘Compendium of Methods for the Determination of Toxic Organic Compounds in Ambient Air’. 86pp. (Environmental Protection Agency: Cincinnati, OH) Available at https://www.epa.gov/sites/production/files/2019-11/documents/to-14ar.pdf
Erb I, Notredame C (2016) How should we measure proportionality on relative gene expression data. Theory in Biosciences 135, 21–36.
| How should we measure proportionality on relative gene expression data.Crossref | GoogleScholarGoogle Scholar |
Evans JC, Huckaby JL, Mitroshkov AV, Julya JL, Hayes JC, Edwards JA, Sasaki LM (1998) 32-week holding-time study of SUMMA polished canisters and triple sorbent traps used to sample organic constituents in radioactive waste tank vapor headspace. Environmental Science & Technology 32, 3410–3417.
| 32-week holding-time study of SUMMA polished canisters and triple sorbent traps used to sample organic constituents in radioactive waste tank vapor headspace.Crossref | GoogleScholarGoogle Scholar |
Fehsenfeld FC, Dickerson RR, Hübler G, Luke WT, Nunnermacker LJ, Williams EJ, Roberts JM, Calvert JG, Curran CM, Delany AC, Eubank CS, Fahey DW, Fried A, Gandrud BW, Langford AO, Murphy PC, Norton RB, Pickering KE, Ridley BA (1987) A ground-based intercomparison of NO, NOx, and NOy measurement techniques. Journal of Geophysical Research 92, 14710
| A ground-based intercomparison of NO, NOx, and NOy measurement techniques.Crossref | GoogleScholarGoogle Scholar |
Fleming PJ, Wallace JJ (1986) How not to lie with statistics: the correct way to summarize benchmark results. Commun ACM 29, 218–221.
| How not to lie with statistics: the correct way to summarize benchmark results.Crossref | GoogleScholarGoogle Scholar |
Gibergans-Baguena J, Hervada-Sala C, Jarauta-Bragulat E (2020) The quality of urban air in Barcelona: a new approach applying compositional data analysis methods. Emerging Science Journal 4, 113–121.
| The quality of urban air in Barcelona: a new approach applying compositional data analysis methods.Crossref | GoogleScholarGoogle Scholar |
Gordon IE, Rothman LS, Hill C, Kochanov RV, Tan Y, Bernath PF, Birk M, Boudon V, Campargue A, Chance KV, Drouin BJ, Flaud J-M, Gamache RR, Hodges JT, Jacquemart D, Perevalov VI, Perrin A, Shine KP, Smith M-AH, Tennyson J, Toon GC, Tran H, Tyuterev VG, Barbe A, Császár AG, Devi VM, Furtenbacher T, Harrison JJ, Hartmann J-M, Jolly A, Johnson TJ, Karman T, Kleiner I, Kyuberis AA, Loos J, Lyulin OM, Massie ST, Mikhailenko SN, Moazzen-Ahmadi N, Müller HSP, Naumenko OV, Nikitin AV, Polyansky OL, Rey M, Rotger M, Sharpe SW, Sung K, Starikova E, Tashkun SA, Auwera JV, Wagner G, Wilzewski J, Wcisło P, Yu S, Zak EJ (2017) The HITRAN2016 molecular spectroscopic database. Journal of Quantitative Spectroscopy and Radiative Transfer 203, 3–69.
| The HITRAN2016 molecular spectroscopic database.Crossref | GoogleScholarGoogle Scholar |
Griffith DWT (1996) Synthetic calibration and quantitative analysis of gas-phase FT-IR spectra. Applied Spectroscopy 50, 59–70.
| Synthetic calibration and quantitative analysis of gas-phase FT-IR spectra.Crossref | GoogleScholarGoogle Scholar |
Griffith DWT, Deutscher NM, Caldow C, Kettlewell G, Riggenbach M, Hammer S (2012) A Fourier transform infrared trace gas and isotope analyser for atmospheric applications. Atmospheric Measurement Techniques 5, 2481–2498.
| A Fourier transform infrared trace gas and isotope analyser for atmospheric applications.Crossref | GoogleScholarGoogle Scholar |
Haase KB, Keene WC, Pszenny AAP, Mayne HR, Talbot RW, Sive BC (2012) Calibration and intercomparison of acetic acid measurements using proton-transfer-reaction mass spectrometry (PTR-MS. Atmospheric Measurement Techniques 5, 2739–2750.
| Calibration and intercomparison of acetic acid measurements using proton-transfer-reaction mass spectrometry (PTR-MS.Crossref | GoogleScholarGoogle Scholar |
Herzog MM, Hudak AT, Weise DR, Bradley AM, Tonkyn RG, Banach CA, Myers TL, Bright BC, Batchelor JL, Kato A, Maitland JS, Johnson TJ (2022) Point cloud based mapping of understory shrub fuel distribution, estimation of fuel consumption and relationship to pyrolysis gas emissions on experimental prescribed burns Fire 5, 118
| Point cloud based mapping of understory shrub fuel distribution, estimation of fuel consumption and relationship to pyrolysis gas emissions on experimental prescribed burnsCrossref | GoogleScholarGoogle Scholar |
Hollander M, Wolfe DA, Chicken E (2014) ‘Nonparametric statistical methods.’ (John Wiley & Sons, Inc: Hoboken, NJ)
Hudak AT, Kato A, Bright BC, Loudermilk EL, Hawley C, Restaino JC, Ottmar RD, Prata GA, Cabo C, Prichard SJ, Rowell EM, Weise DR (2020) Towards spatially explicit quantification of pre- and postfire fuels and fuel consumption from traditional and point cloud measurements. Forest Science 66, 428–442.
| Towards spatially explicit quantification of pre- and postfire fuels and fuel consumption from traditional and point cloud measurements.Crossref | GoogleScholarGoogle Scholar |
Jarauta-Bragulat E, Hervada-Sala C, Egozcue JJ (2016) Air Quality Index revisited from a compositional point of view. Mathematical Geosciences 48, 581–593.
| Air Quality Index revisited from a compositional point of view.Crossref | GoogleScholarGoogle Scholar |
Johnson TJ, Masiello T, Sharpe SW (2006) The quantitative infrared and NIR spectrum of CH2I2 vapor: vibrational assignments and potential for atmospheric monitoring. Atmospheric Chemistry and Physics 6, 2581–2591.
| The quantitative infrared and NIR spectrum of CH2I2 vapor: vibrational assignments and potential for atmospheric monitoring.Crossref | GoogleScholarGoogle Scholar |
Johnson TJ, Profeta LTM, Sams RL, Griffith DWT, Yokelson RL (2010) An infrared spectral database for detection of gases emitted by biomass burning. Vibrational Spectroscopy 53, 97–102.
| An infrared spectral database for detection of gases emitted by biomass burning.Crossref | GoogleScholarGoogle Scholar |
Kajos MK, Rantala P, Hill M, Hellén H, Aalto J, Patokoski J, Taipale R, Hoerger CC, Reimann S, Ruuskanen TM, Rinne J, Petäjä T (2015) Ambient measurements of aromatic and oxidized VOCs by PTR-MS and GC-MS: intercomparison between four instruments in a boreal forest in Finland. Atmospheric Measurement Techniques 8, 4453–4473.
| Ambient measurements of aromatic and oxidized VOCs by PTR-MS and GC-MS: intercomparison between four instruments in a boreal forest in Finland.Crossref | GoogleScholarGoogle Scholar |
Kochanov RV, Gordon IE, Rothman LS, Shine KP, Sharpe SW, Johnson TJ, Wallington TJ, Harrison JJ, Bernath PF, Birk M, Wagner G, Le Bris K, Bravo I, Hill C (2019) Infrared absorption cross-sections in HITRAN2016 and beyond: Expansion for climate, environment, and atmospheric applications. Journal of Quantitative Spectroscopy and Radiative Transfer 230, 172–221.
| Infrared absorption cross-sections in HITRAN2016 and beyond: Expansion for climate, environment, and atmospheric applications.Crossref | GoogleScholarGoogle Scholar |
Korkmaz S, Goksuluk D, Zararsiz G (2014) MVN: An R Package for assessing multivariate normality. The R Journal 6, 151–162.
| MVN: An R Package for assessing multivariate normality.Crossref | GoogleScholarGoogle Scholar |
Li H, Lamb KD, Schwarz JP, Selimovic V, Yokelson RJ, McMeeking GR, May AA (2019) Inter-comparison of black carbon measurement methods for simulated open biomass burning emissions. Atmospheric Environment 206, 156–169.
| Inter-comparison of black carbon measurement methods for simulated open biomass burning emissions.Crossref | GoogleScholarGoogle Scholar |
Lobert JM, Scharffe DH, Hao WM, Crutzen PJ (1990) Importance of biomass burning in the atmospheric budgets of nitrogen-containing gases. Nature 346, 552–554.
| Importance of biomass burning in the atmospheric budgets of nitrogen-containing gases.Crossref | GoogleScholarGoogle Scholar |
Lovell D, Pawlowsky-Glahn V, Egozcue JJ, Marguerat S, Bähler J (2015) Proportionality: a valid alternative to correlation for relative data. PLoS Computational Biology 11, e1004075
| Proportionality: a valid alternative to correlation for relative data.Crossref | GoogleScholarGoogle Scholar |
Ludovici K, Eaton R, Zarnoch S (2018) Longleaf pine site response to repeated fertilization and forest floor removal by raking and prescribed burning. e-Research Paper RP-SRS-60. (USDA Forest Service Southern Research Station: Asheville, NC) Available at https://www.fs.usda.gov/treesearch/pubs/55619
Matt FJ, Dietenberger MA, Weise DR (2020) Summative and ultimate analysis of live leaves from southern U.S. forest plants for use in fire modeling. Energy & Fuels 34, 4703–4720.
| Summative and ultimate analysis of live leaves from southern U.S. forest plants for use in fire modeling.Crossref | GoogleScholarGoogle Scholar |
May AA, McMeeking GR, Lee T, Taylor JW, Craven JS, Burling I, Sullivan AP, Akagi S, Collett JL, Flynn M, Coe H, Urbanski SP, Seinfeld JH, Yokelson RJ, Kreidenweis SM (2014) Aerosol emissions from prescribed fires in the United States: A synthesis of laboratory and aircraft measurements Journal of Geophysical Research: Atmospheres 119, 11,826–11,849.
| Aerosol emissions from prescribed fires in the United States: A synthesis of laboratory and aircraft measurementsCrossref | GoogleScholarGoogle Scholar |
Neves D, Thunman H, Matos A, Tarelho L, Gómez-Barea A (2011) Characterization and prediction of biomass pyrolysis products. Progress in Energy and Combustion Science 37, 611–630.
| Characterization and prediction of biomass pyrolysis products.Crossref | GoogleScholarGoogle Scholar |
Oksanen J, Blanchet FG, Friendly M, Kindt R, Legendre P, McGlinn D, Minchin PR, O’Hara RB, Simpson GL, Solymos P, Stevens MHH, Szoecs E, Wagner H (2020) vegan: Community Ecology Package. Available at https://CRAN.R-project.org/package=vegan
Palarea-Albaladejo J, Martín-Fernández JA (2013) Values below detection limit in compositional chemical data. Analytica Chimica Acta 764, 32–43.
| Values below detection limit in compositional chemical data.Crossref | GoogleScholarGoogle Scholar |
Palarea-Albaladejo J, Martín-Fernández JA (2015) zCompositions — R package for multivariate imputation of left-censored data under a compositional approach. Chemometrics and Intelligent Laboratory Systems 143, 85–96.
| zCompositions — R package for multivariate imputation of left-censored data under a compositional approach.Crossref | GoogleScholarGoogle Scholar |
Pearson K (1897) Mathematical contributions to the theory of evolution.—On a form of spurious correlation which may arise when indices are used in the measurement of organs. Proceedings of the Royal Society of London 60, 489–498.
| Mathematical contributions to the theory of evolution.—On a form of spurious correlation which may arise when indices are used in the measurement of organs.Crossref | GoogleScholarGoogle Scholar |
Philpot CW (1969) Seasonal changes in heat content and ether extractive content of chamise. Research Paper INT-61. (USDA Forest Service, Intermountain Forest and Range Experiment Station: Ogden, UT) Available at https://ia601005.us.archive.org/16/items/seasonalchangesi61phil/seasonalchangesi61phil.pdf
Philpot CW (1971) The pyrolysis products and thermal characteristics of cottonwood and its components. Research Paper INT-107. (USDA Forest Service, Intermountain Forest and Range Experiment Station: Ogden, UT).
Pistone K, Redemann J, Doherty S, Zuidema P, Burton S, Cairns B, Cochrane S, Ferrare R, Flynn C, Freitag S, Howell SG, Kacenelenbogen M, LeBlanc S, Liu X, Schmidt KS, Sedlacek III AJ, Segal-Rozenhaimer M, Shinozuka Y, Stamnes S, van Diedenhoven B, Van Harten G, Xu F (2019) Intercomparison of biomass burning aerosol optical properties from in situ and remote-sensing instruments in ORACLES-2016. Atmospheric Chemistry and Physics 19, 9181–9208.
| Intercomparison of biomass burning aerosol optical properties from in situ and remote-sensing instruments in ORACLES-2016.Crossref | GoogleScholarGoogle Scholar |
Quinn TP, Richardson MF, Lovell D, Crowley TM (2017) propr: An R-package for identifying proportionally abundant features using compositional data analysis. Scientific Reports 7, 16252
| propr: An R-package for identifying proportionally abundant features using compositional data analysis.Crossref | GoogleScholarGoogle Scholar |
Quinn TP, Erb I, Richardson MF, Crowley TM (2018) Understanding sequencing data as compositions: an outlook and review (J Wren, Ed.). Bioinformatics 34, 2870–2878.
| Understanding sequencing data as compositions: an outlook and review (J Wren, Ed.).Crossref | GoogleScholarGoogle Scholar |
Quinn TP, Erb I, Gloor G, Notredame C, Richardson MF, Crowley TM (2019) A field guide for the compositional analysis of any-omics data. GigaScience 8, giz107
| A field guide for the compositional analysis of any-omics data.Crossref | GoogleScholarGoogle Scholar |
Richards LW (1940) Effect of certain chemical attributes of vegetation on forest inflammability. Journal of Agricultural Research 60, 833–838.
Rogers JM, Susott RA, Kelsey RG (1986) Chemical composition of forest fuels affecting their thermal behavior. Canadian Journal of Forest Research 16, 721–726.
| Chemical composition of forest fuels affecting their thermal behavior.Crossref | GoogleScholarGoogle Scholar |
Rothermel RC, Anderson HE (1966) Fire spread characteristics determined in the laboratory. Research Paper INT-30. (USDA Forest Service, Intermountain Forest and Range Experiment Station: Ogden, UT) Available at http://www.fs.fed.us/rm/pubs_int/int_rp030.pdf
Royston JP (1982) An extension of Shapiro and Wilk’s W test for normality to large samples. Applied Statistics 31, 115–124.
| An extension of Shapiro and Wilk’s W test for normality to large samples.Crossref | GoogleScholarGoogle Scholar |
Safdari M-S, Rahmati M, Amini E, Howarth JE, Berryhill JP, Dietenberger M, Weise DR, Fletcher TH (2018) Characterization of pyrolysis products from fast pyrolysis of live and dead vegetation native to the Southern United States. Fuel 229, 151–166.
| Characterization of pyrolysis products from fast pyrolysis of live and dead vegetation native to the Southern United States.Crossref | GoogleScholarGoogle Scholar |
Safdari M-S, Amini E, Weise DR, Fletcher TH (2019) Heating rate and temperature effects on pyrolysis products from live wildland fuels. Fuel 242, 295–304.
| Heating rate and temperature effects on pyrolysis products from live wildland fuels.Crossref | GoogleScholarGoogle Scholar |
Safdari M-S, Amini E, Weise DR, Fletcher TH (2020) Comparison of pyrolysis of live wildland fuels heated by radiation vs. convection. Fuel 268, 117342
| Comparison of pyrolysis of live wildland fuels heated by radiation vs. convection.Crossref | GoogleScholarGoogle Scholar |
Scharko NK, Oeck AM, Myers TL, Tonkyn RG, Banach CA, Baker SP, Lincoln EN, Chong J, Corcoran BM, Burke GM, Ottmar RD, Restaino JC, Weise DR, Johnson TJ (2019a) Gas-phase pyrolysis products emitted by prescribed fires in pine forests with a shrub understory in the southeastern United States. Atmospheric Chemistry and Physics 19, 9681–9698.
| Gas-phase pyrolysis products emitted by prescribed fires in pine forests with a shrub understory in the southeastern United States.Crossref | GoogleScholarGoogle Scholar |
Scharko NK, Oeck AM, Tonkyn RG, Baker SP, Lincoln EN, Chong J, Corcoran BM, Burke GM, Weise DR, Myers TL, Banach CA, Griffith DWT, Johnson TJ (2019b) Identification of gas-phase pyrolysis products in a prescribed fire: first detections using infrared spectroscopy for naphthalene, methyl nitrite, allene, acrolein and acetaldehyde. Atmospheric Measurement Techniques 12, 763–776.
| Identification of gas-phase pyrolysis products in a prescribed fire: first detections using infrared spectroscopy for naphthalene, methyl nitrite, allene, acrolein and acetaldehyde.Crossref | GoogleScholarGoogle Scholar |
Schott JR (2007) A test for the equality of covariance matrices when the dimension is large relative to the sample sizes. Computational Statistics & Data Analysis 51, 6535–6542.
| A test for the equality of covariance matrices when the dimension is large relative to the sample sizes.Crossref | GoogleScholarGoogle Scholar |
Schuette RD (1965) Preparing reproducible pine needle fuel beds. Research Note INT-36. (USDA Forest Service, Intermountain Forest and Range Experiment Station: Ogden, UT)
Sekimoto K, Koss AR, Gilman JB, Selimovic V, Coggon MM, Zarzana KJ, Yuan B, Lerner BM, Brown SS, Warneke C, Yokelson RJ, Roberts JM, de Gouw J (2018) High- and low-temperature pyrolysis profiles describe volatile organic compound emissions from western US wildfire fuels. Atmospheric Chemistry and Physics 18, 9263–9281.
| High- and low-temperature pyrolysis profiles describe volatile organic compound emissions from western US wildfire fuels.Crossref | GoogleScholarGoogle Scholar |
Senneca O, Ciaravolo S, Nunziata A (2007) Composition of the gaseous products of pyrolysis of tobacco under inert and oxidative conditions. Journal of Analytical and Applied Pyrolysis 79, 234–243.
| Composition of the gaseous products of pyrolysis of tobacco under inert and oxidative conditions.Crossref | GoogleScholarGoogle Scholar |
Shafizadeh F (1982) Introduction to pyrolysis of biomass. Journal of Analytical and Applied Pyrolysis 3, 283–305.
| Introduction to pyrolysis of biomass.Crossref | GoogleScholarGoogle Scholar |
Shafizadeh F (1984) The chemistry of pyrolysis and combustion. In ‘The Chemistry of Solid Wood’. (Ed. R Rowell) Advances in chemistry. pp. 489–529. (American Chemical Society: Washington, D.C.)
| Crossref |
Shafizadeh F, Fu YL (1973) Pyrolysis of cellulose. Carbohydrate Research 29, 113–122.
| Pyrolysis of cellulose.Crossref | GoogleScholarGoogle Scholar |
Sharpe SW, Johnson TJ, Sams RL, Chu PM, Rhoderick GC, Johnson PA (2004) Gas-phase databases for quantitative infrared spectroscopy. Appl Spectrosc 58, 1452–1461.
| Gas-phase databases for quantitative infrared spectroscopy.Crossref | GoogleScholarGoogle Scholar |
Srivastava MS (2007) ‘Testing the equality of two covariance matrices and independence of two sub-vectors with fewer observations than the dimension.’ (University of North Carolina at Greensboro: Greensboro, NC)
Srivastava MS, Yanagihara H (2010) Testing the equality of several covariance matrices with fewer observations than the dimension. Journal of Multivariate Analysis 101, 1319–1329.
| Testing the equality of several covariance matrices with fewer observations than the dimension.Crossref | GoogleScholarGoogle Scholar |
Srivastava MS, Yanagihara H, Kubokawa T (2014) Tests for covariance matrices in high dimension with less sample size. Journal of Multivariate Analysis 130, 289–309.
| Tests for covariance matrices in high dimension with less sample size.Crossref | GoogleScholarGoogle Scholar |
Strand T, Gullett B, Urbanski S, O’Neill S, Potter B, Aurell J, Holder A, Larkin N, Moore M, Rorig M (2016) Grassland and forest understorey biomass emissions from prescribed fires in the south-eastern United States – RxCADRE 2012. International Journal of Wildland Fire 25, 102–113.
| Grassland and forest understorey biomass emissions from prescribed fires in the south-eastern United States – RxCADRE 2012.Crossref | GoogleScholarGoogle Scholar |
Susott RA (1982a) Characterization of the thermal properties of forest fuels by combustible gas analysis. Forest Science 28, 404–420.
| Characterization of the thermal properties of forest fuels by combustible gas analysis.Crossref | GoogleScholarGoogle Scholar |
Susott RA (1982b) Differential scanning calorimetry of forest fuels. Forest Science 28, 839–851.
| Differential scanning calorimetry of forest fuels.Crossref | GoogleScholarGoogle Scholar |
Susott RA, DeGroot WF, Shafizadeh F (1975) Heat content of natural fuels. Journal of Fire and Flammability 6, 311–325.
Susott RA, Shafizadeh F, Aanerud TW (1979) A quantitative thermal analysis technique for combustible gas detection. Journal of Fire and Flammability 10, 94–103.
Tasoglou A, Subramanian R, Pandis SN (2018) An inter-comparison of black-carbon-related instruments in a laboratory study of biomass burning aerosol. Aerosol Science and Technology 52, 1320–1331.
| An inter-comparison of black-carbon-related instruments in a laboratory study of biomass burning aerosol.Crossref | GoogleScholarGoogle Scholar |
Tihay V, Gillard P (2010) Pyrolysis gases released during the thermal decomposition of three Mediterranean species. Journal of Analytical and Applied Pyrolysis 88, 168–174.
| Pyrolysis gases released during the thermal decomposition of three Mediterranean species.Crossref | GoogleScholarGoogle Scholar |
Urbanski SP, Hao WM, Baker S (2008) Chemical composition of wildland fire emissions. In ‘Wildland Fires and Air Pollution’. (Eds A Bytnerowicz, MJ Arbaugh, AR Riebau, C Andersen) Developments in Environmental Science. pp. 79–107. (Elsevier: Amsterdam, The Netherlands)
| Crossref |
van den Boogaart KG, Tolosana-Delgado R (2013) ‘Analyzing compositional data with R.’ (Springer: Heidelberg)
Wang H, Lou S, Huang C, Qiao L, Tang X, Chen C, Zeng L, Wang Q, Zhou M, Lu S, Yu X (2014) Source profiles of volatile organic compounds from biomass burning in Yangtze River Delta, China. Aerosol and Air Quality Research 14, 818–828.
| Source profiles of volatile organic compounds from biomass burning in Yangtze River Delta, China.Crossref | GoogleScholarGoogle Scholar |
Ward DE (2001) Combustion chemistry and smoke. In ‘Forest Fires: Behavior and Ecological Effects’. (Eds EA Johnson, K Miyanishi) pp. 55–77. (Academic Press: San Diego, CA)
| Crossref |
Ward DE, Hao WM (1991) ‘Projections of emissions from burning of biomass for use in studies of global climate and atmospheric chemistry.’ p. 19. (Air and Waste Management Association: Vancouver, British Columbia, Canada) Available at http://www.treesearch.fs.fed.us/pubs/43258
Ward DE, Radke LF (1993) Emissions measurement from vegetation fires: a comparative evaluation of methods and results. In ‘Fire in the environment: the ecological, atmospheric, and climatic importance of vegetation fires: report of the Dahlem Workshop, held in Berlin, 15–20 March 1992’. (Eds PJ Crutzen, JG Goldammer) pp. 53–76. (John Wiley & Sons Ltd) Available at http://www.fs.fed.us/rm/pubs_other/rmrs_1993_ward_d001.pdf
Warneke C, Roberts JM, Veres P, Gilman J, Kuster WC, Burling I, Yokelson R, de Gouw JA (2011) VOC identification and inter-comparison from laboratory biomass burning using PTR-MS and PIT-MS. International Journal of Mass Spectrometry 303, 6–14.
| VOC identification and inter-comparison from laboratory biomass burning using PTR-MS and PIT-MS.Crossref | GoogleScholarGoogle Scholar |
Weise DR, Johnson TJ, Reardon J (2015) Particulate and trace gas emissions from prescribed burns in southeastern U.S. fuel types: Summary of a 5-year project. Fire Safety Journal 74, 71–81.
| Particulate and trace gas emissions from prescribed burns in southeastern U.S. fuel types: Summary of a 5-year project.Crossref | GoogleScholarGoogle Scholar |
Weise DR, Jung H, Palarea-Albaladejo J, Cocker DR (2020a) Compositional data analysis of smoke emissions from debris piles with low-density polyethylene. Journal of the Air & Waste Management Association 70, 834–845.
| Compositional data analysis of smoke emissions from debris piles with low-density polyethylene.Crossref | GoogleScholarGoogle Scholar |
Weise DR, Palarea‐Albaladejo J, Johnson TJ, Jung H (2020b) Analyzing wildland fire smoke emissions data using compositional data techniques. Journal of Geophysical Research: Atmospheres 125, e2019JD032128
| Analyzing wildland fire smoke emissions data using compositional data techniques.Crossref | GoogleScholarGoogle Scholar |
Weise DR, Fletcher TH, Johnson TJ, Hao W, Dietenberger MA, Princevac M, Butler BW, McAllister S, O’Brien JJ, Loudermilk EL, Ottmar RD, Hudak AT, Kato A, Shotorban B, Mahalingam S, Mell WE, Boardman CR, Myers TL, Baker SP, Bright BC, Restaino JC (2022a) Fundamental measurements and modeling of prescribed fire behavior in the naturally heterogeneous fuel beds of southern pine forests. Final Report RC-2640. (USDA Forest Service, Pacific Southwest Research Station: Albany, CA) Available at https://apps.dtic.mil/sti/pdfs/AD1180629.pdf
Weise DR, Fletcher TH, Safdari M-S, Amini E, Palarea-Albaladejo J (2022b) Application of compositional data analysis to determine the effects of heating mode, moisture status and plant species on pyrolysates. International Journal of Wildland Fire 31, 24–45.
| Application of compositional data analysis to determine the effects of heating mode, moisture status and plant species on pyrolysates.Crossref | GoogleScholarGoogle Scholar |
Weise DR, Hao WM, Baker S, Princevac M, Aminfar A-H, Palarea-Albaladejo J, Ottmar RD, Hudak AT, Restaino J, O’Brien JJ (2022c) Comparison of fire-produced gases from wind tunnel and small field experimental burns. International Journal of Wildland Fire 31, 409–434.
| Comparison of fire-produced gases from wind tunnel and small field experimental burns.Crossref | GoogleScholarGoogle Scholar |
Westbrook CK, Dryer FL (1981) Simplified reaction mechanisms for the oxidation of hydrocarbon fuels in flames. Combustion Science and Technology 27, 31–43.
| Simplified reaction mechanisms for the oxidation of hydrocarbon fuels in flames.Crossref | GoogleScholarGoogle Scholar |
White JU (1942) Long optical paths of large aperture. Journal of the Optical Society of America 32, 285–288.
| Long optical paths of large aperture.Crossref | GoogleScholarGoogle Scholar |
Yokelson RJ, Griffith DWT, Ward DE (1996) Open-path Fourier transform infrared studies of large-scale laboratory biomass fires. Journal of Geophysical Research: Atmospheres 101, 21067
| Open-path Fourier transform infrared studies of large-scale laboratory biomass fires.Crossref | GoogleScholarGoogle Scholar |
Yokelson RJ, Karl T, Artaxo P, Blake DR, Christian TJ, Griffith DWT, Guenther A, Hao WM (2007) The Tropical Forest and Fire Emissions Experiment: overview and airborne fire emission factor measurements. Atmospheric Chemistry and Physics 7, 5175–5196.
| The Tropical Forest and Fire Emissions Experiment: overview and airborne fire emission factor measurements.Crossref | GoogleScholarGoogle Scholar |
Yokelson RJ, Burling IR, Gilman JB, Warneke C, Stockwell CE, de Gouw J, Akagi SK, Urbanski SP, Veres P, Roberts JM, Kuster WC, Reardon J, Griffith DWT, Johnson TJ, Hosseini S, Miller JW, Cocker III DR, Jung H, Weise DR (2013) Coupling field and laboratory measurements to estimate the emission factors of identified and unidentified trace gases for prescribed fires. Atmospheric Chemistry and Physics 13, 89–116.
| Coupling field and laboratory measurements to estimate the emission factors of identified and unidentified trace gases for prescribed fires.Crossref | GoogleScholarGoogle Scholar |
Zhou X, Mahalingam S (2001) Evaluation of reduced mechanism for modeling combustion of pyrolysis gas in wildland fire. Combustion Science and Technology 171, 39–70.
| Evaluation of reduced mechanism for modeling combustion of pyrolysis gas in wildland fire.Crossref | GoogleScholarGoogle Scholar |
1 The use of trade or firm names in this publication is for reader information and does not imply endorsement by the U.S. Department of Agriculture of any product or service.
Appendix
![]() |
![]() |
![]() |
![]() |