Pyrogeographic analysis of drivers of lightning-ignited wildfires in Tasmania
Amila M. K. Wickramasinghe




A
B
Abstract
Recent studies have reported increasing frequency of lightning fires and associated burned area in Tasmania.
To identify factors driving the frequency and scale of lightning-induced fires in Tasmania.
We compiled datasets on lightning activity, lightning fire, vegetation and fuel moisture. Multi-model inference was used to evaluate predictors of lightning fire. We determined the number of days annually with fuel dry enough to be susceptible to lightning fires. We examined temporal trends in lightning, lightning fires and fuel moisture between 2005 and 2022.
Drier fuels and higher lightning density increased the probability of lightning fires, with areas of treeless vegetation being more susceptible than forests. Lightning fire-prone areas were concentrated in western and central Tasmania. However, no significant trends were found in lightning days or lightning fire days. There was a drying trend in fuel moisture in western and southern Tasmania, and a increasing wetness trend in northern and eastern regions.
Lightning fires are most likely in treeless vegetation with dry fuels and those exposed to intense lightning activity. The short record limits detecting temporal trends. High-resolution data on fuel dryness, lightning and ignitions are crucial for understanding lightning fire dynamics.
Keywords: climate change, dry lightning, forest fire, fuel dryness, Fuel Moisture Index, lightning, lightning density, Tasmania, Tasmanian Wilderness World Heritage area, treeless vegetation.
Introduction
Lightning originating from thunderstorms with minimal rainfall (‘dry lightning’) (Rorig and Ferguson 1999) is an important cause of wildfire globally (Scott et al. 2013; Flannigan et al. 2016; Pineda and Rigo 2017; Pérez-Invernón et al. 2021; Menezes et al. 2022). Such lightning-ignited wildfires (henceforth lightning fires) tend to occur in remote locations (Massada et al. 2012; Penman et al. 2012), so that accessing and controlling them can be difficult, often resulting in more extensive burned areas compared with anthropogenic wildfires (Flannigan and Wotton 1991). For instance, in western boreal and sub-alpine forests in the USA and Canada, approximately 75–90% of areas burned by wildfire are attributed to lightning fires (Nash and Johnson 1996; Abatzoglou et al. 2016; Nagy et al. 2018). Lightning fires are responsible for more than 50% of the area burned in some regions of Australia (Canadell et al. 2021). In the Australian island state of Tasmania, lightning fires were responsible for 53% of the area burned by wildfires between 2011 and 2019, while the number of lightning fires is significantly lower than of anthropogenic ones (Nampak et al. 2021). The low population density of most of Tasmania, especially the Tasmania Wilderness World Heritage Area, may contribute to delays in the detection and suppression of lightning fires, resulting in a larger burned area.
Despite the sensitivity of the paleoendemic species, Tasmania also contains some of the most fire-prone temperate forests in the world (Wood et al. 2011). The associated diversity of ecosystems and contrasting fire regimes make the island an excellent geographical setting for studying wildfires (Bowman et al. 2022). A strong precipitation gradient from the wet west coast (1500–2500 mm mean annual precipitation (MAP)) to the drier east of Tasmania (600–1000 mm MAP) (Bureau of Meteorology 2024), combined with varied geology and terrains ranging from coastal to low mountainous regions (highest point 1617 m above sea level), creates distinct vegetation patterns including rainforests, wet and dry eucalypt forests, grasslands, moorlands and heathlands. At local geographic scales (approximately 100 km2), there are mosaics of highly flammable vegetation (sedgelands and eucalypt savannas and forests) and fire-sensitive communities (including alpine vegetation and rainforest). The interplay of environmental gradients and vegetation diversity creates diverse fire regimes, ranging from frequent, low-severity surface fires to infrequent and intense forest fires (Fox-Hughes et al. 2014; Grose et al. 2014).
The southwestern part of the island is conserved in the Tasmanian Wilderness World Heritage Area (TWWHA) (Fig. 1a). The TWWHA covers approximately 15,800 km2 or nearly 25% of Tasmania’s landmass and is one of the last remaining expanses of temperate wilderness worldwide. Uncontrolled fires can destroy ecosystems that support endemic biotas that evolved in Gondwana, known as palaeoendemics (Bowman et al. 2021). The primary method used to mitigate the risk of wildfires in this area is prohibiting park visitors from setting outdoor camp fires, and using prescribed burning to reduce fuels in flammable vegetation types, particularly treeless sedgeland areas (Styger et al. 2018). Lightning fires, however, remain a threat to fire-sensitive ecosystems and hence their detection and control present a major management challenge.
Broad vegetation types and bioregions for the Australian island of Tasmania: (a) forest, treeless, and other vegetation types categorised within grid cells (0.05° × 0.05°) and boundary of the TWWHA (black line); (b) boundaries of Tasmania’s bioregions. Geographic data from the Department of Natural Resources and Environment Tasmania (2021).
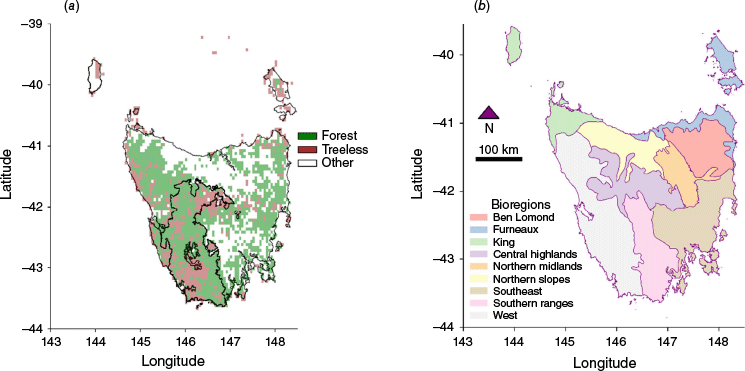
Styger et al. (2018) found evidence of a rise in the number lightning fires, the extent of land affected by these fires and the proportion of wildfires ignited by lightning within the TWWHA since 1990. However, their analysis did not consider trends in lightning fires in Tasmania more broadly. Additionally, Bowman et al. (2022) showed the area burned by unplanned fires, including lightning fires, across Tasmania has been increasing since 1990, with a notable rise in the percentage of Tasmania’s total area affected by lightning fires over this period. In the last decade, Tasmania has experienced large wildfires caused by lightning (Marsden-Smedley 2014; Love et al. 2019; Bowman et al. 2021; Wardlaw 2021; Ozaki et al. 2023). In 2013, several fires were ignited by lightning strikes and grew rapidly owing to subsequent dry, hot and windy conditions, collectively burning 0.8% of Tasmania’s land area (Tasmanian Government 2013; Marsden-Smedley 2014). Dry lightning storms in mid and late January 2016, following an extremely dry spring, resulted in multiple fires that burned approximately 123,000 ha across Tasmania, including 20,100 ha (~1.3%) of the TWWHA (Love et al. 2019). Similarly, in mid-January 2019, southern Tasmania experienced a massive dry lightning storm, resulting in over 2000 lightning strikes1 (Wardlaw 2021), which initiated about 70 fires in remote locations. Some of those lightning fires coalesced to form the Riveaux Road fire (Wardlaw 2021; Ozaki et al. 2023), which burned approximately 64,000 ha area encompassing tall eucalypt forests, silviculturally regenerated eucalypt forests, eucalypt plantations and buttongrass moorlands (Wardlaw 2021).
The observed increase in lightning fire activity within the TWWHA has been putatively attributed to a rise in the frequency of dry lightning storms, droughts and increasing fuel dryness (DPIPWE 2016; Styger et al. 2018). This trend raises concerns about a heightened risk of lightning fires occurring more often in the future (Coogan et al. 2020; Janssen et al. 2023), particularly because fire weather is projected to worsen owing to a warming and drying future climate (Keeley and Syphard 2016; Clarke and Evans 2019; Bowman et al. 2020; Li et al. 2020). Synoptic patterns conducive to extreme fire weather conditions across Tasmania are predicted to increase by the year 2100 (Grose et al. 2014), leading to an increase in overall fire danger, especially during the spring. The number and extent of lightning fires are likely determined by many factors including lightning occurrence, whether it is accompanied by rainfall, type of vegetation, fuel load and moisture content, weather conditions, presence of organic soils and firefighting response (Latham and Williams 2001; Rorig and Ferguson 2002; Evarts 2010; Abdollahi et al. 2019; Santoso et al. 2019; Nampak et al. 2021; Kalashnikov et al. 2023; Rao et al. 2023; Wickramasinghe et al. 2024). Lightning occurrence is highly variable in space and time (Kuleshov et al. 2006), and reliable, consistently recorded lightning data are available only since automatic detection systems became available in ~1995 (Christian et al. 2000; Virts et al. 2013; Peterson et al. 2021). Such a short record makes it difficult to determine whether there has been a significant recent increase in the number of lightning strikes. Therefore, it is important to examine temporal trends and spatial patterns in fuel moisture, which is likely one of the underlying drivers of the observed increases in lightning fires in Tasmania.
The overarching aim of our study is to determine whether the temporal increases in lightning fire activity observed in the TWWHA extend to Tasmania more broadly, and to identify the drivers of the increases. In this study, we describe the spatial and seasonal trends of lightning fires across Tasmania, identifying the lightning fire season. We also determine a fuel moisture threshold conducive to lightning fires and quantify the number of dry lightning days, dry fuel days, and days with coincident dry fuel and dry lightning during the lightning fire season. Additionally, we assess the probability of lightning fires in relation to forest and treeless vegetation types, fuel dryness and the number of lightning strikes (lightning density) within this fire season.
Data and methods
Lightning and lightning-induced fire data
Lightning data for the state of Tasmania were provided by the World Wide Lightning Location Network (WWLLN) (Jacobson et al. 2006; Hutchins et al. 2012), and include the date and time (Coordinated Universal Time, UTC) and the ground location coordinates of detected strikes, with high detection efficiency (~70–80%) for strokes over 40 kA peak current, and efficiencies (15–25%) for all cloud-to-ground strokes, based on data from over five WWLLN stations (World Wide Lightning Location Network 2023). Spanning January 2005 to December 2019, the dataset comprises 44,735 lightning strikes recorded in Tasmania. We also used lightning fire data from the Department of Natural Resources and Environment Tasmania (2024), which includes fires ignited by lightning, as well as information on the fire start dates and the mapped fire-affected areas (area polygons). This dataset has been collated from remotely sensed data and field data collected by the Tasmanian Fire Service, Tasmania Parks and Wildlife Service and the former Forestry Tasmania, and its reliability and consistency have improved with time with the increasing availability of high-resolution remotely sensed burned area data. Both data sources were used to examine the monthly distribution of: (i) total number of lightning strikes; (ii) dry lightning strikes, which we operationally defined as lightning strikes on days with less than 2.5 mm precipitation during a 24-h period (from 00:00 to 23:59 hours) (SILO 2023) following the approach of Rorig and Ferguson 1999); and (iii) number of lightning fires. We defined the fire season based on the months with the highest frequency of lightning fires across the state. Subsequent analyses were based on this defined fire season and were conducted for both the entire state of Tasmania and within the TWWHA, to explore potential drivers of the increased lightning fire activity reported within the TWWHA by Styger et al. (2018).
The lightning strike and fire data were mapped to grid cells measuring 0.05 × 0.05 (approximately 5 × 5 km), for use in statistical analyses, as well as to visualise the frequency of fuel dryness and incidence of dry lightning. This grid size was chosen to align with the spatial accuracy of the WWLLN lightning measurements, which has a mean spatial accuracy of 4.3 km (median: 3.0 km) (Hutchins et al. 2012; World Wide Lightning Location Network 2023). The 0.05 × 0.05 resolution ensures that the grid size is consistent with the uncertainty in the lightning data while avoiding oversmoothing of vegetation classes. We considered that more intense dry lightning storms are more likely to lead to fire, so we also calculated ‘lightning density’ as the number of strikes per grid cell on each dry lightning day.
Fuel moisture
We used the Fuel Moisture Index (FMI) of Sharples et al. (2009), a dimensionless linear index, as a proxy for the moisture content of litter fuels. The daily average FMI data, with a resolution of 0.05 × 0.05, were computed from climate data from the BARRA-TA (Bureau of Meteorology Atmospheric high-resolution Regional Reanalysis for Tasmania (TA)) (Su et al. 2021) downscaled regional reanalysis product. Vegetation data, sourced from TASVEG, the digital vegetation map of Tasmania (Department of Primary Industries Parks Water and Environment 2022), were similarly resampled to a 0.05 × 0.05 resolution. The dominant vegetation type within each grid cell was used to classify the vegetation as either treeless or forest. The spatial distribution of vegetation in Tasmania, classified into forest, treeless and other vegetation types (Department of Primary Industries Parks Water and Environment 2022), is presented in Fig. 1a.
Statistical analyses
We used statistical analyses to understand the correlates of lightning-ignited fires. Our data were suspected to be spatially correlated, so we initially tested how much this affected our findings using a generalised additive model (GAM) to explain fire occurrence that included the spatial terms latitude and longitude, along with FMI and fuel type. This was compared with the corresponding GAM without the spatial terms to quantify the spatial effect for model selection. Although the model containing the spatial terms was superior to the one without (Akaike’s Information Criterion (AIC) was lower by 238; Moran’s I was 0.22), results were similar, so our subsequent analyses were based on log-binomial regression models, which addresses the issue of potential bias in prevalence ration estimation and were easier to interpret.
We used multi-model inference and model selection based on AIC to evaluate predictors of the probability of fire occurring in a grid cell if dry lightning was recorded in that grid cell on the same day during the defined fire season. To evaluate statistical importance of the explanatory variables, the library ‘itertools’ of the Python package (Lott 2018) was used to generate a candidate set of models containing all possible combinations of explanatory variables, without interactions, and calculate their AIC weight. Continuous variables were scaled, so the magnitude of effects could be compared. Weighted model average coefficients and 95% confidence intervals were calculated from the AIC weights. To assess the model’s generalisability and reduce overfitting, we performed five-fold cross-validation, ensuring a more reliable estimate of performance given the limited number of fire occurrences. We used full model averaging, whereby coefficients were set to zero in models that did not contain the term (Galipaud et al. 2017). Variables were considered statistically important if these intervals did not overlap zero (Galipaud et al. 2017).
In our log-binomial regression model, we considered only those days and grid cells in which dry lightning was recorded; each grid cell and day with dry lightning represented one observation. The binary response variable was ‘Fire occurrence’; grid cells within fire polygons on days when lightning fires occurred were coded as 1, and grid cells outside these polygons as 0. The explanatory variables were FMI, vegetation type (forest, treeless) and lightning density, all at a grid cell resolution of 0.05 × 0.05. There were 15,138 non-fire occurrences (zeroes) compared with only 408 fire occurrences (ones).
We considered a 5% probability of fire arising from lightning strikes within a grid cell as representing a significant fire risk, consistent with probabilities observed in similar studies (Dorph et al. 2022) in the nearby southeast Australian state of Victoria (3–15%) for lightning-induced fires. The FMI corresponding to this 5% probability of lightning ‘fire occurrence’ was calculated separately for treeless and forest vegetation, then averaged to indicate the FMI threshold conducive to lightning fires. This operationally defined threshold was used in the mapping of fire risk from dry lightning strikes (Nolan et al. 2016; Argañaraz et al. 2018; Ellis et al. 2022).
Mapping the frequency of favourable conditions for lightning fires
Utilising the defined FMI threshold and the identified fire season, we calculated the mean number of ‘dry fuel days’ – days below the threshold – per year over the 2783 days that span 18 fire seasons, for each grid cell across Tasmania. We then generated maps illustrating the lightning density expressed as the number of strikes per grid cell during the fire seasons for the period of 2005 to 2020 inclusive and mean number of dry fuel days, along with the mean number of precipitation days, lightning days and dry lightning days per year. We also created a composite map that highlights regions susceptible to a higher frequency of dry fuel conditions and elevated incidence of dry lightning, taking into account factors such as lightning activity, precipitation and fuel dryness.
FMI distributions and relationship to lightning fires
An alternative way of investigating the bias in lightning fire occurrence towards dry fuels was to examine the percentage frequency distributions of FMI in (i) all grid cells on all days during the defined fire season; (ii) grid cells where dry lightning occurred and that were within lightning fire polygons (Department of Primary Industries Parks Water and Environment 2023); and (iii) grid cells where dry lightning occurred, but that were not inside fire polygons. This approach is less sensitive to missing data than is the binomial modelling based on presence or absence of fire in a defined space–time domain (Prior et al. 2024).
Examining temporal variations of the days of fuel dryness and lightning occurrence
We used the non-parametric Mann–Kendall test to examine the monotonic trends in the frequency of days with (i) lightning, (ii) lightning and dry fuel, and (iii) lightning, dry fuel and fire occurrences across both Tasmania and the TWWHA. Additionally, we analysed the proportion of dry fuel grid cells across Tasmania and the TWWHA. The predictor variable was the fire season, defined as the months of January, February, March, November and December of each year from 2005 to 2020, while the response variables were the totals for each metric within each fire season. We analysed the temporal trends of lightning fires using data from the Department of Natural Resources and Environment Tasmania (2024) across Tasmania and the TWWHA from 2005 to 2019 using the Mann–Kendall test. Although lightning fire records date back to the 1980s, data from earlier years were inconsistently collected, and records after 2019 were unavailable. Consequently, although our main analysis of the number of lightning fires is restricted to the period between 2005 and 2019, we did an additional analysis using the full time period from 1980 to 2019. We used a generalised linear model to test for a trend in fuel moisture trend during the lightning fire season in the period from 2004 and 2020 for individual grid cells at a spatial resolution of 0.05 × 0.05. FMI was the response variable and month and year were predictor variables. Grid cells exhibiting statistically significant trends (P ≤ 0.05) were used to identify drying regions (negative gradients) and increasingly wet regions (positive gradients) across Tasmania.
Results
Fire season in Tasmania
There was considerable monthly variation in lightning strikes across Tasmania overall, and within the TWWHA. Lightning was most frequent from November through January, with these 3 months accounting for 71% of the 31,636 strikes recorded in Tasmania between 2005 and 2019 inclusive (Fig. 2a). The number of lightning strikes then decreased to a minimum in June, before gradually increasing to January. Similar trends were observed in the TWWHA (Fig. 2b). The incidence of lightning fires mirrors the trend in total lightning strikes (Fig. 2c, d), with peak occurrences occurring from November to March, comprising approximately 97% of lightning fires in Tasmania and 99% in the TWWHA. The period from November to March was therefore identified as the primary fire season for both Tasmania and the TWWHA in our analysis, given the heightened occurrence of lightning and the corresponding increase in lightning fires.
The total number of lightning strikes recorded in each month from January 2005 to December 2019 is presented for (a) Tasmania, and (b) the TWWHA. The dark blue bars indicate elevated lightning activity from November to March, compared with the rest of the year, represented by light blue bars. The corresponding total number of lightning fires during this period is shown in (c) Tasmania, and (d) the TWWHA. A similar trend is observed in the occurrence of lightning-caused fires, with a significant number of incidents during the November to March period in both Tasmania and TWWHA.
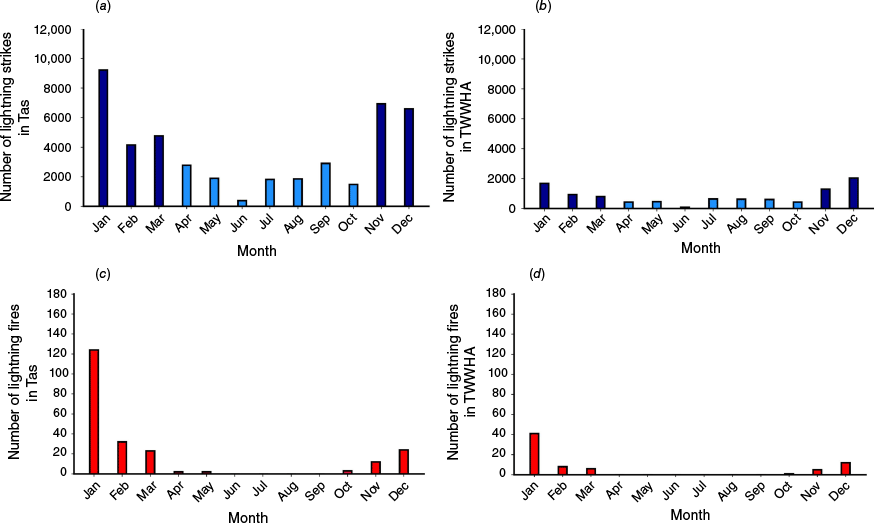
Dry lightning strikes and fires within the fire season
Over the study period, only one-fourth of dry lightning days (precipitation <2.5 mm day−1) coincided with dry fuel conditions across Tasmania, and in the TWWHA, this was even less frequent, with 1 in every 5.9 dry lightning days occurring alongside dry fuels, indicating that these conditions rarely coincide, particularly in the TWWHA. The analysis of dry lightning strike days revealed that most grid cells (75%) experienced only one dry strike per day, whereas grid cells with five or more strikes in a single day were uncommon, representing only 3.3% of the total number of cases. The maximum daily lightning density recorded was 32 strikes in a single grid cell. The number of lightning fires relative to lightning strikes was also low. January had the highest percentage at 1.34% in Tasmania and 2.43% in the TWWHA, compared with February’s 0.77% in Tasmania and 0.86% in the TWWHA. The percentage from November to March was 0.68% for Tasmania and 1.07% for the TWWHA.
Lightning fires burned a total of 445,686 ha across Tasmania during the defined fire seasons between January 2005 and December 2019, with 318,049 ha of the affected area located within the TWWHA. Thus, the TWWHA, which covers 23.1% of Tasmania’s area, experienced 21.4% of the total lightning strikes, but accounted for approximately 71.4% of the total area burned from lightning in the state.
Predictors of lightning fire
As expected, the probability of fires following dry lightning strikes was influenced by FMI, lightning density and vegetation, with the log-binomial regression model incorporating FMI, lightning density and vegetation as predictors showing the best performance, with the lowest AIC score, highest accuracy (97%) and highest ROC-AUC (receiver operating characteristic–area under the curve) value (0.762), indicating its superior ability to distinguish between fire and non-fire occurrences (Supplementary Table S1). All predictors in this model were statistically supported, with weighted average confidence intervals not including zero. The five-fold cross-validation results show consistent accuracy (~95%) across both vegetation types, with slight variation in AUC, indicating strong model performance in distinguishing fire occurrence.
The probability of dry lightning igniting a fire increased strongly as fuels became drier, particularly in treeless vegetation (Fig. 3a, b). The effect of treeless versus forest vegetation was comparable in magnitude with that of FMI, although it was associated with a wider confidence interval. The FMI associated with a 5% of probability of lightning fire occurrence is 11.56 for forests and 16.38 for treeless vegetation. We used the average of these FMIs (FMI = 14) as the threshold fuel moisture content signifying the likelihood of fire initiation during the lightning fire season in Tasmania. The binomial model predictions aligned well with the observed data for FMI values above 10, though slight overfitting occurred for lower FMIs, for which there were fewer data points. The probability of fire occurrence also increased with lightning density, although this had a weaker effect than did vegetation or FMI (Fig. 3e). The effect of lightning density was most apparent when it exceeded approximately 15; however, such incidents were rare, occurring in only ~0.1% of our dataset.
(a) Probability of dry lightning causing fire in relation to FMI in (a) forest, and (b) treeless vegetation. Bars show actual data, and the line the probability predicted from weighted averaged coefficients. Note the different scales on the y-axes. For presentation, FMI was binned into 10-unit classes, but actual data were used in the modelling. Probability of dry lightning igniting fires in relation to lightning density for selected FMI values, for (c) forest, and (d) treeless vegetation. The error bars represent 5% margin of error. (e) AIC weighted averaged model coefficients, and 95% confidence intervals of the predictor variables used in the binomial regression models. The ‘Treeless’ coefficient is relative to ‘Forest’. Continuous variables were standardised so the magnitude of effects can be directly compared. All predictors in the model showed statistical significance, as the weighted average confidence intervals did not encompass zero.
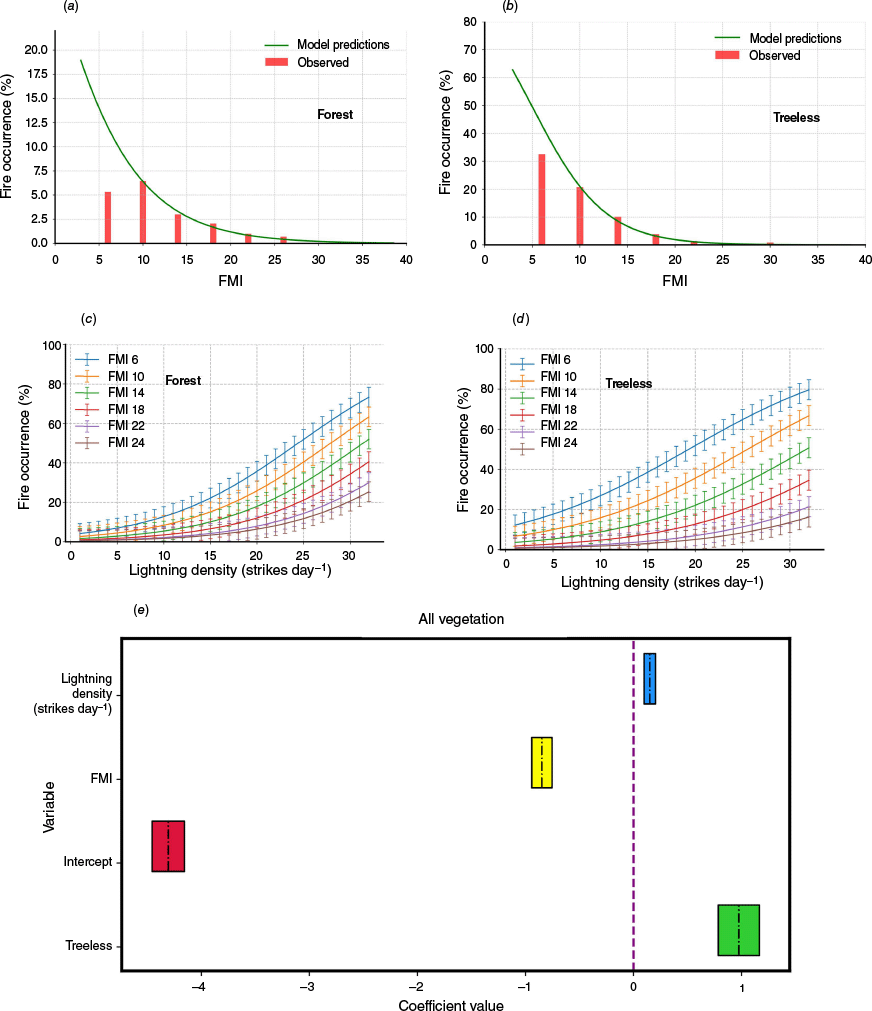
Maps of fuel dryness and dry-lightning days
The spatial variation of lightning density (strikes grid cell−1) (Fig. 4a) indicates that most lightning strikes occurred in the central highlands, western region, parts of the northern slopes and the northeast, including the Ben Lomond bioregion (Department of Natural Resources and Environment Tasmania 2021). The spatial distribution of days with less than 2.5 mm of precipitation in Tasmania exhibits a steep gradient from west to east, with the northern midlands bioregion experiencing up to approximately 135 dry days year−1 (Fig. 4b). Similarly, the northern midlands bioregion (Department of Natural Resources and Environment Tasmania 2021) sees up to approximately 80 dry fuel days year−1 (Fig. 4c). By contrast, the west of Tasmania, particularly within the TWWHA, receives more than 2.5 mm of precipitation on most days, and regions such as the northwest, south, Flinders Island (Furneaux bioregion) and King Island (King bioregion) exhibit less than 10 days of significant fuel dryness annually. Without accounting for precipitation (Fig. 4d), the northeast of the state (Ben Lomond bioregion) (Fig. 1b) has the highest lightning occurrence, of up to 1.2 days year−1. Other areas, including western sedgelands and central to eastern parts of the state, also experience frequent lightning days. However, this pattern changes for dry lightning days (Fig. 4e), which occur most often in the central to eastern parts of the state (up to 0.7 days year−1). The composite map (Fig. 4f), representing days with dry lightning while the FMI is below 14, highlights the central region (northern midlands, central highlands and Derwent valley bioregions) (Department of Natural Resources and Environment Tasmania 2021) of Tasmania as being particularly susceptible to lightning fires, with up to 0.4 days year−1 of such conditions. Except for the south most of the TWWHA (Fig. 1a) also shows a medium to high number of days with dry lightning and dry fuel conditions.
Geographic patterns of lightning, lightning fires and fuel dryness. (a) Lightning density represented as the number of strikes per grid cell for the period 2005–2020. Remaining panels indicate the number of days per year during the fire season in Tasmania with the following conditions: (b) ‘dry weather’, with precipitation <2.5 mm; (c) ‘dry fuel’ days with FMI <14; (d) days with lightning occurrence; (e) ‘dry lightning’ days with lightning occurrence and precipitation <2.5 mm; (f) ‘dry lightning days with dry fuel’ (lightning occurrence + precipitation <2.5 mm + FMI <14).
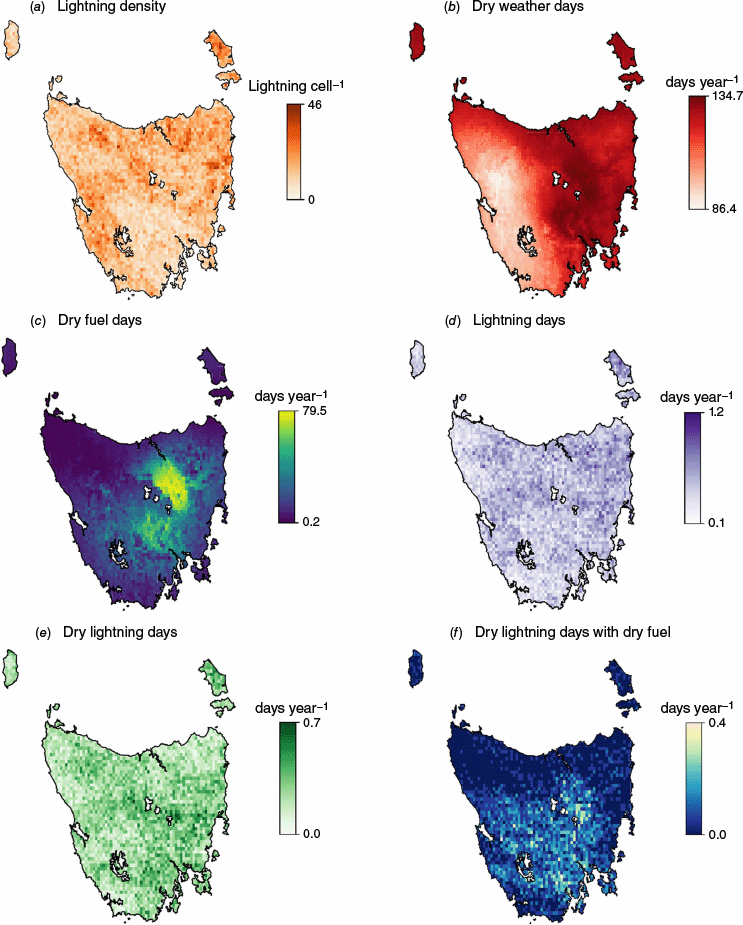
Frequency distribution of dry lightning days with and without fire
Across Tasmania, the median FMI for the dry lightning days with fire was 15.2, substantially lower than the value of 19.7 for the days without fire (Fig. 5). For context, the median FMI value for all days within the fire seasons in Tasmania was 18.4.
Trends in dry lightning days and fuel moisture
There was considerable variation in the number of lightning days across Tasmania and within the TWWHA (Fig. 6a). Lightning days were relatively frequent in 2014 and 2019, whereas in 2017 and 2020, there were very few in either the TWWHA, or Tasmania as a whole. Mann–Kendall test revealed that there were no statistically significant (P > 0.05) temporal trends in lightning days (Fig. 6a), days combining lightning and dry fuels (Fig. 6b), days combining lightning fires and dry fuels (Fig. 6c) (P > 0.05), reflecting high inherent variability and the limited period for which data are available. The strength and direction of the monotonic trends in the data were evaluated using the Mann–Kendall test with the tau (τ) statistic. The results indicate a weak negative trend (τ = −0.05) for lightning days, a weak to moderate positive trend (τ = 0.52) for lightning and fire days, and weak positive trends (τ = 0.12 and τ = 0.09) for lightning days with dry fuels in both Tasmania and the TWWHA. However, there was an upward trend in the number of lightning fires during the fire season across Tasmania and the TWWHA, which was not significant in the 2005–2019 period (Fig. 6e; P = 0.45 and 0.79 and τ = 0.14, 0.05 respectively), but it was highly significant during the longer 1980–2019 period (P < 0.001 and P = 0.02 and τ = 0.49 and 0.27 respectively; Fig. 6f). The geographical analysis showed contrasting trends in fuel moisture across the state during the period 2004–2020. There was a general drying trend in the central west and south, and a wetting trend in a band extending from the northwest and to the central east (Fig. 6g).
Annual trends in lightning, fuel dryness and lightning fire for Tasmania and the TWWHA during the fire season (Jan, Feb, March, Nov, Dec). Shading indicates 95% confidence intervals. (a) Number of lightning days; (b) number of lightning days with dry fuel (FMI < 14); (c) number of lightning fire days with dry fuel; (d) proportion of grid cells with dry fuel. Also shown are the number of lightning fires for the periods of (e) 2005–2019, and (f) 1980–2019. Overlapping markers in the early years indicate that the number of lightning fires in Tasmania and the TWWHA were identical during this period in (f). Note that reliability of these data has improved with time (see methods). (g) Map showing trends in FMI across Tasmania between 2004 and 2005, derived from a generalised linear model. Colours indicate areas with statistically significant (P ≤ 0.05) wetting trends (green) and drying trends (pink).
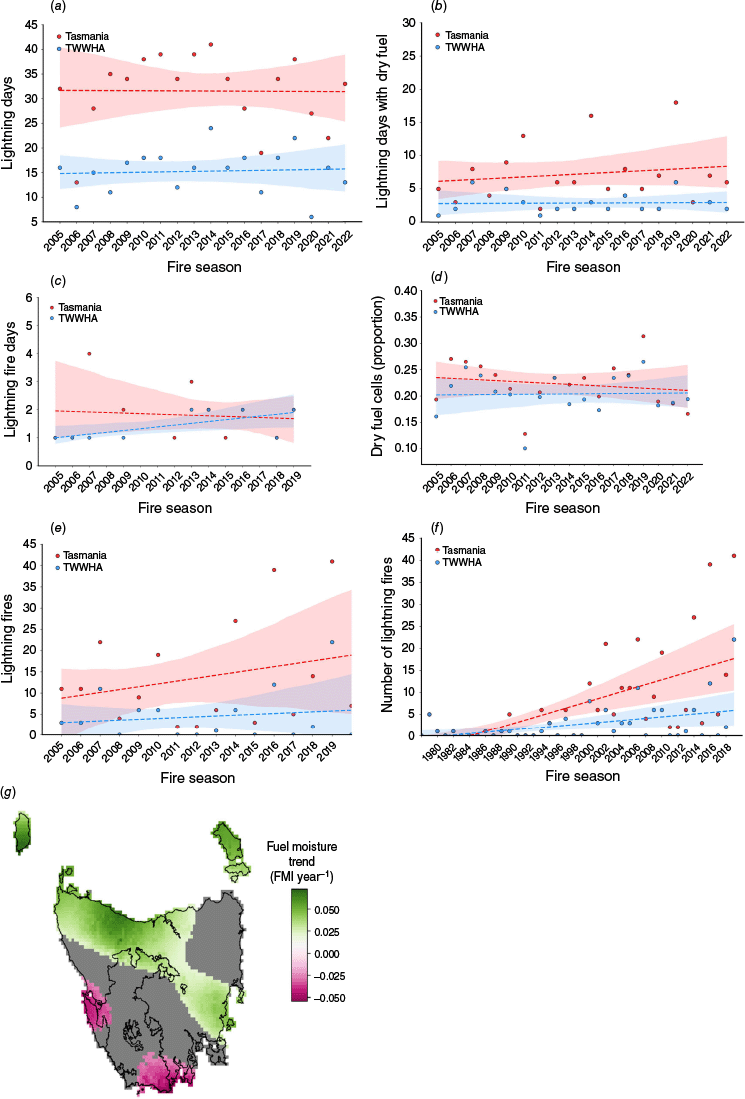
Discussion
The overarching aim of our study was to determine the drivers of the increasing number of lightning fires in Tasmania and increased area burned that has been reported for Tasmania (Styger et al. 2018; Bowman et al. 2022). Our pyrogeographic analyses show that while lightning strikes are fairly common, only a small fraction (2.7%) ignite fires. Further, our statistical analyses identified the critical role of fuel moisture, vegetation structure (forest vs treeless vegetation) and lightning density in driving lightning-ignited wildfires, supporting the findings of Styger et al. (2018) that fire incidence and area burned were correlated with extremes of soil dryness. We did not detect a robust statistically significant temporal trend in the number of lightning fires between 2005 and 2019, the period for which consistently collected data were available, but there was a significant increase between 1980 and 2019 (Fig. 6f), concordant with findings of Styger et al. (2018) for the period from 1980 to 2016. This underscores the importance of long-term, consistently collected data. We discuss our findings in relation to related research around the world, and consider the implication of climate change and identify opportunities to improve the predictive capacity of lightning fire risk modelling.
Our analysis revealed a distinct seasonal pattern in lightning activity, with a pronounced peak during the fire season when fuels are dry and fire weather frequently occurs (November to March, especially January), consistent with previous research (Bowman and Williamson 2021; Nampak et al. 2021). Previous spatial and temporal analyses of lightning fires in Tasmania have shown that only a small fraction of lightning strikes result in fire ignition, indicating the likely importance of dry fuel conditions combined with hot, windy weather (Marsden-Smedley; Caccamo et al. 2012; Wickramasinghe et al. 2024). Similar environmental constraints on lightning ignitions have been reported from Canada’s Central Cordillera (Wierzchowski et al. 2002). Note that some authors have used the metric ‘lightning ignition efficiency’ (LIE), or the number of ignitions per lightning strike, to compare results from different environments (Nampak et al. 2021). However, given the uncertainty in the location of recorded lightning in our dataset, we were unable to attribute fires to individual lightning strikes, so we could not calculate LIE.
We found that increased lightning density was associated with an increased probability of lightning fires (Nampak et al. 2021; Wickramasinghe et al. 2024). It is important to note that lightning is relatively uncommon in Tasmania, with far fewer annual lightning days than most of mainland Australia, especially northern Australia (Krause et al. 2014), and fewer high-density lightning storms (Dowdy and Kuleshov 2014; Peterson et al. 2021). However, the rare, high-density lightning storms are responsible for a disproportionate number of lightning strikes; for example, 15% of all lightning strikes (wet and dry combined) occurred on just 0.47% of the 1068 lightning days recorded between 2004 and 2018 in Tasmania (Wickramasinghe et al. 2024). This pattern mirrors observations in other dry lightning-prone regions globally; for example, Kalashnikov et al. (2022) noted that in California, a significant portion of lightning-induced wildfires resulted from rare, large dry lightning outbreaks. The comparative rarity of lightning, and its clustering in particularly intense storms, makes it difficult to discern clear trends in the available short record (2005–2022), despite previously reported upward trends in ignitions and area burned by lightning (Styger et al. 2018; Bowman et al. 2022).
Increased fuel dryness has consistently been linked to higher probabilities of dry lightning fires, as evidenced by studies in southeastern Australia and California (Dowdy and Mills 2012; Penman et al. 2012; Dorph et al. 2022; Rao et al. 2023). Our study confirms these findings, identifying specific fuel moisture thresholds that vary by vegetation type and align with established thresholds in both Australian and global contexts (Latham and Williams 2001; Larjavaara 2005; Nolan et al. 2016).
We found that treeless landscapes had a higher likelihood of lightning fire than did forests, especially for fuel moisture in the FMI range of 5–15, which is also consistent with Rodrigues et al. (2023), where the probability of fire was approximately four times higher in treeless vegetation than in forest. This agrees with other studies (Latham and Williams 2001; Nampak et al. 2021), including one in Tasmania showing a particularly high likelihood of fire in buttongrass moorlands (Nampak et al. 2021). Treeless landscapes like buttongrass moorlands (sedgelands) and grasslands present a higher fire hazard due to a drier microclimate than the more shaded, cooler forested areas (Styger et al. 2018). Dense canopy forests, including rainforests and wet eucalypt forests, maintain more stable microclimates, reducing their susceptibility to dry lightning fires despite high fuel loads (Leonard et al. 2014; Furlaud et al. 2021). For example, Bowman et al. (2024) found that sedgeland had six times more days per year when fine fuels are available to burn than adjacent forests. The high prevalence of buttongrass moorlands in western Tasmania, including the TWWHA, further amplifies fire risk in this region under dry conditions (Whinam and Hope 2005; French et al. 2016; Bowman et al. 2023).
The risk of lightning fires is the product of complex geographic patterns in lightning occurrence and rainfall across Tasmania. Rainfall is substantially higher in the west than the east of the state, but lightning occurrence is also generally higher in the central west and northeast. The overall result is a lower risk of lightning fires in the north and the far southwest of Tasmania than the rest of the state. Furthermore, we can attribute the absence of an overall trend in fuel moisture for Tasmania to geographically divergent trends across the state. The geographic patterns in fuel moisture that we detected align with observed changes in climate, whereby western Tasmania is receiving less rainfall and warmer temperatures, while eastern Tasmania is receiving more intense rainfall events (Grose et al. 2010).
Climate models offer varied projections on the impacts of warming on lightning activity, with some forecasting a 10% global increase in lightning frequency for every degree Celsius of warming (Price 2009), while others anticipate a decrease due to shifts in tropospheric ozone and methane levels (Finney et al. 2018). However, these projections are subject to significant uncertainty owing to differences in lightning parameterisation methods, with some models predicting up to a 45% increase in lightning flash density under future warming, whereas others forecast a decrease of up to 6.7% (Clark et al. 2017; Etten‐Bohm et al. 2021). There can also be large differences in regional trends. For example, in recent decades, central and northern Australia have experienced a decrease in dry lightning days (Canadell et al. 2021), whereas there has been a significant increase in dry lightning days along the southeast Australian coast, strongly linked to larger areas of forest being burned and a heightened risk of lightning-ignited wildfires (Canadell et al. 2021; Pérez-Invernón et al. 2023). The trends in southeastern Australia signify a potential increase in lightning fire risks in Tasmania due to climate-driven changes. Here, climate change is expected to lead to hotter conditions, more erratic rainfall and often drier fuels (Grose et al. 2014; Bowman et al. 2021), which may further exacerbate these risks. This underscores the importance of integrating current climate projections to better predict and mitigate future lightning-induced wildfires.
Incorporating vegetation traits such as fuel load, regrowth rates, dead fuel moisture content, Topographic Wetness Index (TWI) and time since the last fire into predictive models, alongside climate change projections, flash polarity and the peak current of lightning strikes (Schultz et al. 2019) could improve the accuracy of lightning fire risk assessments. Predictive modelling provides valuable insights into lightning fire risks but faces significant limitations in data availability and quality. Enhanced real-time monitoring has been achieved through the extensive deployment of lightning detection sensors across Tasmania, which offer precise geolocation data to better capture lightning events (NSSN 2023) and corresponding lightning ignition locations. However, collection of high-resolution fuel data is essential to accurately represent vegetation patterns and improve the precision of statistical models. Challenges persist in monitoring and forecasting live fuel moisture, especially in remote areas like the TWWHA. Adopting high-resolution Sentinel image time series (Ban et al. 2020) and integrating past fire severity data can greatly refine model accuracy for predicting lightning fires.
Conclusion
Our findings indicate that the highest lightning fire risk occurs during the November to March period in both Tasmania and the TWWHA. Our statistical analyses demonstrated that drier fuels and higher lightning density significantly increase the probability of lightning fires, especially in treeless vegetation compared with forests. We found that areas in western and central Tasmania are susceptible to dry lightning fires on more days per year during the fire season than other parts of the island. However, we were unable to demonstrate a significant historical trend in lightning activity or dry fuels days annually, reflecting the short record and high interannual variability of these data. Continued monitoring of fuel dryness and lightning activity, especially during the peak fire season, is crucial to further improve predictive models of the probability of lightning fire under current and projected future climates.
Data availability
The data that support this study will be shared on reasonable request to the corresponding author.
Declaration of funding
This research was funded by the Australian Research Council; FL220100099 awarded to D. M. J. S. B. and DP220100795 awarded to M. M. B., D. M. J. S. B., G. J. W.
Acknowledgement
The authors acknowledge the financial support provided by the Australian Research Council.
References
Abatzoglou JT, Kolden CA, Balch JK, Bradley BA (2016) Controls on interannual variability in lightning-caused fire activity in the western US. Environmental Research Letters 11(4), 045005.
| Crossref | Google Scholar |
Abdollahi M, Dewan A, Hassan QK (2019) Applicability of remote sensing-based vegetation water content in modeling lightning-caused forest fire occurrences. ISPRS International Journal of Geo-Information 8(3), 143.
| Crossref | Google Scholar |
Argañaraz JP, Landi MA, Scavuzzo CM, Bellis LM (2018) Determining fuel moisture thresholds to assess wildfire hazard: a contribution to an operational early warning system. PLoS One 13(10), e0204889.
| Crossref | Google Scholar | PubMed |
Ban Y, Zhang P, Nascetti A, Bevington AR, Wulder MA (2020) Near real-time wildfire progression monitoring with Sentinel-1 SAR time series and deep learning. Scientific Reports 10(1), 1322.
| Crossref | Google Scholar | PubMed |
Bowman DM, Williamson GJ (2021) River flows are a reliable index of forest fire risk in the temperate Tasmanian wilderness World Heritage Area, Australia. Fire 4(2), 22.
| Crossref | Google Scholar |
Bowman DM, Kolden CA, Abatzoglou JT, Johnston FH, van der Werf GR, Flannigan M (2020) Vegetation fires in the Anthropocene. Nature Reviews Earth & Environment 1(10), 500-515.
| Google Scholar |
Bowman DM, Rodriguez-Cubillo D, Prior LD (2021) The 2016 Tasmanian wilderness fires: fire regime shifts and climate change in a Gondwanan biogeographic refugium. In ‘Ecosystem Collapse and Climate Change’. (Eds JG Canadell, RB Jackson) pp. 133–153. (Springer) 10.1007/978-3-030-71330-0_6
Bowman DM, Kolden CA, Williamson GJ (2022) Bushfires in Tasmania, Australia: an introduction. Fire 5(2), 33.
| Crossref | Google Scholar |
Bowman DM, Ondei S, Lucieer A, Foyster S, Prior LD (2023) Forest–sedgeland boundaries are historically stable and resilient to wildfire at Blakes Opening in the Tasmanian Wilderness World Heritage Area, Australia. Landscape Ecology 38(1), 205-222.
| Crossref | Google Scholar |
Bowman DM, Prior LD, Foyster SM, Williamson GJ, Hua Q, Ondei S (2024) Edaphic factors control fire-prone sedgeland and Eucalyptus forest mosaics in southwestern Tasmania. Catena 242, 108114.
| Crossref | Google Scholar |
Bureau of Meteorology (2024) Average annual, seasonal and monthly rainfall maps, Vol. 2024. Average annual rainfall 30-year climatology (1991 to 2020). (Commonwealth of Australia) Available at http://www.bom.gov.au/climate/maps/averages/rainfall/?period=an®ion=ta
Caccamo G, Chisholm LA, Bradstock RA, Puotinen ML (2012) Using remotely sensed fuel connectivity patterns as a tool for fire danger monitoring. Geophysical Research Letters 39(1), L01302.
| Crossref | Google Scholar |
Canadell JG, Meyer C, Cook GD, Dowdy A, Briggs PR, Knauer J, Pepler A, Haverd V (2021) Multi-decadal increase of forest burned area in Australia is linked to climate change. Nature Communications 12(1), 6921.
| Crossref | Google Scholar | PubMed |
Clark SK, Ward DS, Mahowald NM (2017) Parameterization‐based uncertainty in future lightning flash density. Geophysical Research Letters 44(6), 2893-2901.
| Crossref | Google Scholar |
Clarke H, Evans JP (2019) Exploring the future change space for fire weather in southeast Australia. Theoretical and Applied Climatology 136, 513-527.
| Crossref | Google Scholar |
Coogan SC, Cai X, Jain P, Flannigan MD (2020) Seasonality and trends in human-and lightning-caused wildfires ≥2 ha in Canada, 1959–2018. International Journal of Wildland Fire 29(6), 473-485.
| Crossref | Google Scholar |
Department of Natural Resources and Environment Tasmania (2021) Tasmania’s Wetlands. (Tasmanian Government: Hobart, Tasmania) Available at https://nre.tas.gov.au/conservation/flora-of-tasmania/tasmanias-wetlands#Tasmania’sBioregions
Department of Natural Resources and Environment Tasmania (2024) Fire History. (Hobart, Tasmania) Available at https://listdata.thelist.tas.gov.au/opendata/
Department of Primary Industries Parks Water and Environment (2022) TASVEG 4.0 Fire Attributes. (Hobart, Tasmania) Available at https://www.thelist.tas.gov.au/app/content/data/geo-meta-data-record?detailRecordUID=8d982040-7cd5-45e0-97fd-2b07d5f8e503 [verified 22 November 2024]
Department of Primary Industries Parks Water and Environment (2023) Fire History. (Hobart, Tasmania) Available at https://listdata.thelist.tas.gov.au/opendata/ [verified 22 November 2024]
Dorph A, Marshall E, Parkins KA, Penman TD (2022) Modelling ignition probability for human-and lightning-caused wildfires in Victoria, Australia. Natural Hazards and Earth System Sciences 22(10), 3487-3499.
| Crossref | Google Scholar |
Dowdy AJ, Kuleshov Y (2014) Climatology of lightning activity in Australia: spatial and seasonal variability. Australian Meteorological and Oceanographic Journal 64(2), 103-108.
| Crossref | Google Scholar |
Dowdy AJ, Mills GA (2012) Atmospheric and fuel moisture characteristics associated with lightning-attributed fires. Journal of Applied Meteorology and Climatology 51(11), 2025-2037.
| Crossref | Google Scholar |
DPIPWE (2016) Tasmanian wilderness world heritage area (TWWHA) management plan 2016. (Department of Primary Industries, Water and Environment: Hobart, Australia) Available at https://increate.med-ina.org/static/assets/uploads/share/Step4-tools/DPIPWE-Tasmanian-Wilderness-Management-Plan-2016-part-1.pdf
Ellis TM, Bowman DM, Jain P, Flannigan MD, Williamson GJ (2022) Global increase in wildfire risk due to climate‐driven declines in fuel moisture. Global Change Biology 28(4), 1544-1559.
| Crossref | Google Scholar | PubMed |
Etten‐Bohm M, Yang J, Schumacher C, Jun M (2021) Evaluating the relationship between lightning and the large‐scale environment and its use for lightning prediction in global climate models. Journal of Geophysical Research: Atmospheres 126(5), e2020JD033990.
| Crossref | Google Scholar |
Finney DL, Doherty RM, Wild O, Stevenson DS, MacKenzie IA, Blyth AM (2018) A projected decrease in lightning under climate change. Nature Climate Change 8(3), 210-213.
| Crossref | Google Scholar |
Flannigan M, Wotton B (1991) Lightning-ignited forest fires in northwestern Ontario. Canadian Journal of Forest Research 21(3), 277-287.
| Crossref | Google Scholar |
Flannigan M, Wotton B, Marshall G, De Groot W, Johnston J, Jurko N, Cantin A (2016) Fuel moisture sensitivity to temperature and precipitation: climate change implications. Climatic Change 134, 59-71.
| Crossref | Google Scholar |
Fox-Hughes P, Harris R, Lee G, Grose M, Bindoff N (2014) Future fire danger climatology for Tasmania, Australia, using a dynamically downscaled regional climate model. International Journal of Wildland Fire 23(3), 309-321.
| Crossref | Google Scholar |
French BJ, Prior LD, Williamson GJ, Bowman DM (2016) Cause and effects of a megafire in sedge-heathland in the Tasmanian temperate wilderness. Australian Journal of Botany 64(6), 513-525.
| Crossref | Google Scholar |
Furlaud JM, Prior LD, Williamson GJ, Bowman DM (2021) Fire risk and severity decline with stand development in Tasmanian giant Eucalyptus forest. Forest Ecology and Management 502, 119724.
| Crossref | Google Scholar |
Galipaud M, Gillingham MA, Dechaume‐Moncharmont FX (2017) A farewell to the sum of Akaike weights: the benefits of alternative metrics for variable importance estimations in model selection. Methods in Ecology and Evolution 8(12), 1668-1678.
| Crossref | Google Scholar |
Grose MR, Fox-Hughes P, Harris RM, Bindoff NL (2014) Changes to the drivers of fire weather with a warming climate – a case study of southeast Tasmania. Climatic Change 124, 255-269.
| Crossref | Google Scholar |
Hutchins ML, Holzworth RH, Rodger C, Heckman S, Brundell JB (2012) WWLLN absolute detection efficiencies and the global lightning source function. Proceedings of Geophysical Research Abstracts 14, 12917.
| Google Scholar |
Jacobson AR, Holzworth R, Harlin J, Dowden R, Lay E (2006) Performance assessment of the world wide lightning location network (WWLLN), using the Los Alamos sferic array (LASA) as ground truth. Journal of Atmospheric and Oceanic Technology 23(8), 1082-1092.
| Crossref | Google Scholar |
Janssen TA, Jones MW, Finney D, Van der Werf GR, van Wees D, Xu W, Veraverbeke S (2023) Extratropical forests increasingly at risk due to lightning fires. Nature Geoscience 16(12), 1136-1144.
| Crossref | Google Scholar |
Kalashnikov DA, Abatzoglou JT, Nauslar NJ, Swain DL, Touma D, Singh D (2022) Meteorological and geographical factors associated with dry lightning in central and northern California. Environmental Research: Climate 1(2), 025001.
| Google Scholar |
Kalashnikov DA, Abatzoglou JT, Loikith PC, Nauslar NJ, Bekris Y, Singh D (2023) Lightning‐ignited wildfires in the western United States: ignition precipitation and associated environmental conditions. Geophysical Research Letters 50(16), e2023GL103785.
| Crossref | Google Scholar |
Keeley JE, Syphard AD (2016) Climate change and future fire regimes: examples from California. Geosciences 6(3), 37.
| Crossref | Google Scholar |
Krause A, Kloster S, Wilkenskjeld S, Paeth H (2014) The sensitivity of global wildfires to simulated past, present, and future lightning frequency. Journal of Geophysical Research: Biogeosciences 119(3), 312-322.
| Crossref | Google Scholar |
Kuleshov Y, Mackerras D, Darveniza M (2006) Spatial distribution and frequency of lightning activity and lightning flash density maps for Australia. Journal of Geophysical Research: Atmospheres 111(D19), D19105.
| Crossref | Google Scholar |
Latham D, Williams E (2001) Lightning and forest fires. In ‘Forest Fires’. (Eds EA Johnson, K Miyanishi) pp. 375–418. (Elsevier) 10.1016/B978-012386660-8/50013-1
Leonard SW, Bennett AF, Clarke MF (2014) Determinants of the occurrence of unburnt forest patches: potential biotic refuges within a large, intense wildfire in south-eastern Australia. Forest Ecology and Management 314, 85-93.
| Crossref | Google Scholar |
Li Y, Mickley LJ, Liu P, Kaplan JO (2020) Trends and spatial shifts in lightning fires and smoke concentrations in response to 21st century climate over the national forests and parks of the western United States. Atmospheric Chemistry and Physics 20(14), 8827-8838.
| Crossref | Google Scholar |
Love P, Remenyi T, Harris R, Bindoff N (2019) Tasmanian Wilderness World Heritage Area Climate Change and Bushfire Research Initiative. (The Antarctic Climate & Ecosystems Cooperative Research Centre: Hobart, Tasmania, Australia) Available at https://figshare.utas.edu.au/articles/report/Tasmanian_Wilderness_World_Heritage_Area_Climate_Change_and_Bushfire_Research_Initiative/23254919/1
Marsden-Smedley J. Lightning fires and climate change in the Tasmanian Wilderness World Heritage Area. (Department of Natural Resources and Environment Tasmania) Available at https://nre.tas.gov.au/Documents/RTI%20041%20-%202019%20-%2020%20(Active%20Disclosure).pdf
Marsden-Smedley J (2014) Tasmanian Wildfires January February 2013: Forcett, Dunalley, Repulse, Bicheno, Giblin River, Montumana, Molesworth and Gretna. (BCR Centre: East Melbourne, Victoria) Available at https://www.bushfirecrc.com/sites/default/files/managed/resource/taswildfires2013_final_reduced_sizel.pdf
Massada AB, Syphard AD, Stewart SI, Radeloff VC (2012) Wildfire ignition-distribution modelling: a comparative study in the Huron–Manistee National Forest, Michigan, USA. International Journal of Wildland Fire 22(2), 174-183.
| Crossref | Google Scholar |
Menezes LS, de Oliveira AM, Santos FL, Russo A, de Souza RA, Roque FO, Libonati R (2022) Lightning patterns in the Pantanal: untangling natural and anthropogenic-induced wildfires. Science of The Total Environment 820, 153021.
| Crossref | Google Scholar | PubMed |
Nagy RC, Fusco E, Bradley B, Abatzoglou JT, Balch J (2018) Human-related ignitions increase the number of large wildfires across US ecoregions. Fire 1(1), 4.
| Crossref | Google Scholar |
Nampak H, Love P, Fox-Hughes P, Watson C, Aryal J, Harris RM (2021) Characterizing spatial and temporal variability of lightning activity associated with wildfire over Tasmania, Australia. Fire 4(1), 10.
| Crossref | Google Scholar |
Nash C, Johnson E (1996) Synoptic climatology of lightning-caused forest fires in subalpine and boreal forests. Canadian Journal of Forest Research 26(10), 1859-1874.
| Crossref | Google Scholar |
Nolan RH, Boer MM, Resco de Dios V, Caccamo G, Bradstock RA (2016) Large‐scale, dynamic transformations in fuel moisture drive wildfire activity across southeastern Australia. Geophysical Research Letters 43(9), 4229-4238.
| Crossref | Google Scholar |
NSSN (NSW Smart Sensing Network) (2023) Promising sensors detect high-risk lightning strikes which help avoid catastrophic bushfires. (NSW Smart Sensing Network) Available at https://www.nssn.org.au/news/2023/10/11/promising-sensors-detect-high-risk-lightning-strikes-which-help-avoid-catastrophic-bushfires
Ozaki M, Williamson G, Fox-Hughes P, Love P, Aryal J (2023) Gell River fire driven by forced channeling and lateral fire in 2018–19 summer, Tasmania. Preprints 2023, 2023071502.
| Crossref | Google Scholar |
Penman T, Bradstock RA, Price O (2012) Modelling the determinants of ignition in the Sydney Basin, Australia: implications for future management. International Journal of Wildland Fire 22(4), 469-478.
| Crossref | Google Scholar |
Pérez-Invernón FJ, Huntrieser H, Soler S, Gordillo-Vázquez FJ, Pineda N, Navarro-González J, Reglero V, Montanyà J, van der Velde O, Koutsias N (2021) Lightning-ignited wildfires and long continuing current lightning in the Mediterranean Basin: preferential meteorological conditions. Atmospheric Chemistry and Physics Discussions 21(23), 17529-17557.
| Crossref | Google Scholar |
Pérez-Invernón FJ, Gordillo-Vázquez FJ, Huntrieser H, Jöckel P (2023) Variation of lightning-ignited wildfire patterns under climate change. Nature Communications 14(1), 739.
| Crossref | Google Scholar | PubMed |
Peterson M, Mach D, Buechler D (2021) A global LIS/OTD climatology of lightning flash extent density. Journal of Geophysical Research: Atmospheres 126(8), e2020JD033885.
| Crossref | Google Scholar |
Pineda N, Rigo T (2017) The rainfall factor in lightning-ignited wildfires in Catalonia. Agricultural and Forest Meteorology 239, 249-263.
| Crossref | Google Scholar |
Price C (2009) Thunderstorms, lightning and climate change. In ‘Lightning: Principles, Instruments and Applications’. (Eds HD Betz, U Schumann, P Laroche) (Springer: Dordrecht, Netherlands) 10.1007/978-1-4020-9079-0_24
Prior LD, Storey K, Williamson GJ, Bowman DM (2024) When soil becomes fuel: identifying a safe window for prescribed burning of Tasmanian vegetation growing on organic soils. International Journal of Wildland Fire 33(6), WF24061.
| Crossref | Google Scholar |
Rao K, Williams AP, Diffenbaugh NS, Yebra M, Bryant C, Konings AG (2023) Dry live fuels increase the likelihood of lightning‐caused fires. Geophysical Research Letters 50(15), e2022GL100975.
| Crossref | Google Scholar |
Rodrigues M, Jiménez-Ruano A, Gelabert PJ, de Dios VR, Torres L, Ribalaygua J, Vega-García C (2023) Modelling the daily probability of lightning-caused ignition in the Iberian Peninsula. International Journal of Wildland Fire 32(3), 351-362.
| Crossref | Google Scholar |
Rorig ML, Ferguson SA (1999) Characteristics of lightning and wildland fire ignition in the Pacific Northwest. Journal of Applied Meteorology and Climatology 38(11), 1565-1575.
| Crossref | Google Scholar |
Rorig ML, Ferguson SA (2002) The 2000 fire season: lightning-caused fires. Journal of Applied Meteorology and Climatology 41(7), 786-791.
| Crossref | Google Scholar |
Schultz CJ, Nauslar NJ, Wachter JB, Hain CR, Bell JR (2019) Spatial, temporal and electrical characteristics of lightning in reported lightning-initiated wildfire events. Fire 2(2), 18.
| Crossref | Google Scholar | PubMed |
Sharples JJ, McRae RH, Weber R, Gill AM (2009) A simple index for assessing fuel moisture content. Environmental Modelling & Software 24(5), 637-646.
| Crossref | Google Scholar |
SILO (Scientific Information for Land Owners) (2023) SILO - Australian climate data from 1889 to yesterday. Available at https://www.longpaddock.qld.gov.au/silo/about/climate-variables/
Styger J, Marsden-Smedley J, Kirkpatrick J (2018) Changes in lightning fire incidence in the Tasmanian Wilderness World Heritage Area, 1980–2016. Fire 1(3), 38.
| Crossref | Google Scholar |
Su C-H, Eizenberg N, Jakob D, Fox-Hughes P, Steinle P, White CJ, Franklin C (2021) BARRA v1.0: kilometre-scale downscaling of an Australian regional atmospheric reanalysis over four midlatitude domains. Geoscientific Model Development 14(7), 4357-4378.
| Crossref | Google Scholar |
Tasmanian Government (2013) 2013 Tasmanian Bushfires Inquiry. Available at https://www.dpac.tas.gov.au/__data/assets/pdf_file/0016/30751/1.Tasmanian_Bushfires_Inquiry_Report.pdf
Virts KS, Wallace JM, Hutchins ML, Holzworth RH (2013) Highlights of a new ground-based, hourly global lightning climatology. Bulletin of the American Meteorological Society 94(9), 1381-1391.
| Crossref | Google Scholar |
Wardlaw T (2021) Measuring a fire. The story of the January 2019 fire told from measurements at the Warra Supersite, Tasmania. Fire 4(2), 15.
| Crossref | Google Scholar |
Whinam J, Hope G (2005) The peatlands of the Australasian region. Stapfia 85, 397-433.
| Google Scholar |
Wickramasinghe AM, Boer MM, Cunningham CX, Nolan RH, Bowman DM, Williamson GJ (2024) Modeling the probability of dry lightning‐induced wildfires in Tasmania: a machine learning approach. Geophysical Research Letters 51(16), e2024GL110381.
| Crossref | Google Scholar |
Wierzchowski J, Heathcott M, Flannigan MD (2002) Lightning and lightning fire, Central Cordillera, Canada. International Journal of Wildland Fire 11(1), 41-51.
| Crossref | Google Scholar |
Wood SW, Murphy BP, Bowman DM (2011) Firescape ecology: how topography determines the contrasting distribution of fire and rain forest in the south‐west of the Tasmanian Wilderness World Heritage Area. Journal of Biogeography 38(9), 1807-1820.
| Crossref | Google Scholar |
World Wide Lightning Location Network (2023) ‘WWLLN: World Wide Lightning Location Network.’ (University of Washington) 10.5281/zenodo.15054997