Linking fire radiative power to land cover, fire history, and environmental setting in Alaska, 2003–2022
Jessica J. Walker
A
B
C
Abstract
Fire radiative power (FRP) shows promise as a diagnostic and predictive indicator of fire behavior and post-fire effects in Alaska, USA.
To investigate relationships between FRP, vegetation functional groups, and environmental settings in Alaska (2003–2022) under various fire history conditions.
We tested for distinctness of MODIS FRP distributions associated with vegetation classes and fire legacies (frequency and number of previous burns). We used a random forest model to examine relative importance of vegetation class for FRP versus bottom-up biophysical and temporal parameters.
FRP distributions are statistically distinct among vegetation functional groups with contrasting fuel biomass, or within functional groups with contrasting burn characteristics. Location and topography, which constrain vegetation class, strongly determine FRP, and fire history is of lesser importance over the 19-year analysis period.
FRP can be used to identify wildfire consumption in dissimilar vegetation classes but is highly conditioned by geographic location. The complex and evolving vegetation composition of post-fire boreal landscapes precludes a clear association of expected FRP at distinct stages of recovery.
These results can inform further study of FRP as an indicator of fire behavior and fuel consumption and for informing dynamics of post-fire recovery across Alaska.
Keywords: Alaska, black spruce, boreal, fire intensity, Fire radiative power (FRP), MODIS, post-fire, shrubland, tundra, white spruce, wildfire.
Introduction
The US state of Alaska (AK) has been warming faster than the global average for over half a century (Markon et al. 2018), consistent with observations of amplified warming in high-latitude regions of the northern hemisphere (Wendler and Shulski 2009; Screen and Simmonds 2010; Rantanen et al. 2022). During this time, the size, frequency (i.e. interval between fires), and ecological impacts of wildfires have increased across most Alaskan biomes (Kasischke and Turetsky 2006; Kasischke et al. 2010; Turetsky et al. 2011; French et al. 2015; Barrett et al. 2016; Masrur et al. 2018; Buma et al. 2022). Increases in the annual burned area and occurrence of large fire seasons have also been reported, although the statistical robustness and magnitude of trends varies with geographic extent, span of time, and source data (Kasischke and Turetsky 2006; Veraverbeke et al. 2017, 2018; York et al. 2020; Jones et al. 2022).
Intensified fire activity has potential to disrupt formerly predictable trajectories of post-fire vegetation succession across broad regions of northern boreal forest (Baltzer et al. 2021). In the forested boreal interior of Alaska, under historical climate and fire regimes, post-fire forest regeneration typically occurs as either self-replacement of the same dominant pre-fire tree species or as multiple successions of tree species that assume post-fire canopy dominance (Kurkowski et al. 2008). For example, stand-replacing crown fires in forests of black spruce (Picea mariana (Mill.) Britton, Sterns & Poggenb.), the dominant tree species in boreal North America (Baltzer et al. 2021), trigger a successional pattern characterized by recruitment of pre-fire overstorey species within a decade following fire (Johnstone et al. 2004), sometimes including a deciduous phase where sprouting trees (e.g. quaking aspen [Populus tremuloides Michx.] and paper birch [Betula papyrifera Marshall]) and willows (Salix spp.) dominate prior to closure of the black spruce canopy (Johnstone 2006; Mack et al. 2008). Climate-induced shifts in boreal fire regimes can lead to a third successional pathway – species replacement – where fires occurring with atypical frequency (hereafter, repeat fires) or with uncharacteristic severity result in a shift in species dominance from conifers to broadleaf deciduous species (Kurkowski et al. 2008; Johnstone et al. 2010). In recent decades, repeat fires and increased consumption of surface and belowground organic layers seem to be catalyzing a shift in forest dominance from black spruce to alternative broadleaf deciduous or mixed forests (Barrett et al. 2011; Johnstone et al. 2020; Baltzer et al. 2021). Anomalous climate and fire patterns are also driving succession changes in non-forested, high-latitude ecosystems. In tundra ecosystems, increasing wildfire activity (Jones et al. 2009) is predicted to lead to a proliferation or expansion of shrub cover (Lantz et al. 2013; Macander et al. 2022) though with considerable biophysically and environmentally mediated variation in response (e.g. Bret-Harte et al. 2013; Chen et al. 2021; Hollingsworth et al. 2021).
Changes in climate and fire regimes and concomitant shifts in vegetation composition, succession, and landscape vegetation patterns have far-reaching consequences for the structure, functioning, and ecological role of boreal ecosystems. Persistent rearrangements of vegetation communities, their productivity and flammability, and their relationships to fire can affect surface energy fluxes through changes in albedo and energy and carbon exchanges (Chapin et al. 2000; Turetsky et al. 2011) and influence global climate regulation via shifts in terrestrial–atmospheric exchange of water, energy, and biogenic carbon (Gauthier et al. 2015). At more local levels, biome shifts have important implications for biodiversity (Schindler and Lee 2010), wildlife habitat (Rupp et al. 2006; Gustine et al. 2014; Palm et al. 2022), game management (Beck et al. 2011), and the availability of subsistence resources for rural communities (Nelson et al. 2008).
In Alaska, where the remoteness and inaccessibility of many wildfire sites complicate ground-based investigations into fire behavior and ecological fire impacts, satellite imagery is an invaluable tool for assessing immediate and longer-term impacts of fires (Michalek et al. 2000). Imagery acquired by the Moderate Resolution Imaging Spectroradiometer (MODIS) sensors on the National Aeronautics and Space Administration (NASA) Earth Observing System satellites Aqua and Terra is of particular utility due to the sensors’ explicit design for active fire monitoring purposes (Kaufman et al. 1996) and high image acquisition rate (four times daily). The detection of radiances at mid-infrared channels by MODIS allows the calculation of fire radiative power (FRP), a quantitative measure of fire intensity at the time of measurement (Kaufman et al. 1996, 1998) that is proportional to the instantaneous rate of fuel consumption and pyrogenic emissions (Kaufman et al. 1998; Wooster 2002; Wooster and Zhang 2004; Wooster et al. 2005). FRP is thus a critical first-order metric and the source of additional derived fire parameters; the integration of FRP over the total duration of a fire yields fire radiative energy (FRE), which is linearly related to the cumulative amount of emissions and combusted biomass (Kaufman et al. 1996; Wooster 2002).
The statistically robust relationship between FRP and rates of fuel consumption (Wooster et al. 2005) has allowed researchers to explore FRP observations for insights into broad-scale ecological functioning and fire behavior. This relationship exists because the amount of radiant heat released per unit of dry matter consumed is relatively consistent across vegetation types (Wooster et al. 2005; Giglio et al. 2006), such that variations in FRP arise from temporal and spatial variability in the quantity and condition (e.g. density and moisture content) of biomass available to burn (Ichoku et al. 2008). For example, a global study of FRP across different vegetation classes found that in tropical ecosystems with high vegetation moisture content, FRP is inversely related to forest density and positively associated with the proportion of herbaceous vegetation, while the reverse is true in boreal regions (Giglio et al. 2006). Wooster and Zhang (2004) used FRP to distinguish between fire characteristics in boreal Siberia and North America. Lower mean FRP values in Siberia, combined with a decoupling from increasing percent tree cover, led to the inference that surface fires are dominant in Siberia while hotter canopy fires are more frequent in North America. Smith and Wooster (2005) classified savanna fires into head- or back-fires based on FRP values associated with relative proportions of smoldering or flaming emissions. Barrett and Kasischke (2013) attributed higher FRP values associated with late-season fires in boreal Alaskan forests to increasingly warm and dry fuels, as compared with early-season conditions. In circumpolar tundra systems, Masrur et al. (2018) documented similar seasonal FRP variability, which was correlated with antecedent (prior winter) and prevailing land evaporation, precipitation, surface temperature, and soil moisture levels.
The potential use of FRP information and the difficulty of collecting field data across Alaska incentivize the examination of how FRP, as a measure of fire intensity, can be used to infer characteristics and short- and long-term impacts of wildfires on ecosystems. Fire intensity may be a singularly useful metric of potential fire effects in boreal forests because it can be linked to consumption of both aboveground biomass and soil organic layers, resolving some of the issues in assigning ecological effects based on fire severity measures. Here, we distinguish fire intensity from fire severity. Fire intensity is the instantaneous measurement of a fire’s energetic output (Keeley 2009), while fire severity is the post hoc assessment of ecological change caused by a fire (Lentile et al. 2006). In Alaskan boreal forests, the high flammability of black spruce means that fire severity is largely decoupled from tree mortality or overstorey consumption (Kasischke et al. 2010). Fire severity is measured instead by depth of organic layer burn, which is the most important determinant of boreal ecosystem functioning (Barrett et al. 2010) and a critical factor in post-fire regrowth trajectories (Dyrness and Norum 1983; Viereck 1983; Kasischke et al. 2008; Gibson et al. 2016). Customary assessments of fire severity via interpretation of pre- and post-fire spectral reflectance (e.g. Holsinger et al. 2022) are less meaningful in boreal forests because the degree of soil layer consumption is not directly detectable via existing satellite sensors (Murphy et al. 2008; Barrett et al. 2010). FRP-derived intensity metrics can potentially be used as proxies of fire severity in boreal forests because the consumption of fuel biomass includes the organic soil layer. These metrics could be validated with field-based assessments of fire behavior and effects, including regrowth trajectories, and used in future active fires to predict possible ecological outcomes. Parameterizing FRP values across a range of boreal vegetation types and burn conditions helps associate active fire behavior with fire effects and identify the conditions likely to produce disrupted trajectories of post-fire vegetation succession.
To our knowledge, no previous studies have evaluated spatial and temporal patterns of FRP in Alaska across the length of the MODIS record. Here we leverage MODIS imagery and the computational power of a cloud-based geospatial analysis platform to explore wildfire FRP patterns in diverse Alaskan biomes from 2003 through 2022. Our overarching objective is to investigate the influence of vegetation composition and history of recent fire on detected fire intensity across Alaska by relating FRP data to an extensive spatial catalog of mapped fire perimeters since 1940 and a moderate-resolution (30-m) classified vegetation map. In this study, we aimed to: (1) examine the relationship of FRP to vegetation type; (2) examine the relationship of FRP to fire history (frequency and fire-free interval); and (3) evaluate the importance of other bottom-up parameters such as location and topography on patterns of FRP. Quantifying the relationships of FRP to vegetation and fire history provides information about fire effects in remote, high-latitude ecosystems where direct observations and field-based characterization of fire characteristics and impacts are difficult to obtain. An established link of FRP with vegetation classes and fire history parameters supports efforts to predict the effects of subsequent fires more accurately, contributing to better models of likely succession trajectories, post-fire landscape composition, and future fire regimes in Alaska.
Materials and methods
Study area
The study area is defined by wildfires detected by MODIS across Alaska, USA from 2003 through 2022 (Fig. 1). The majority of fires are in the forested boreal interior, which encompasses ~800,000 km2 between the Brooks Range in the north to the Alaska Range in the south (Dissing and Verbyla 2003; Wendler and Shulski 2009). Fires are less common in the northern and western tundra biome (Kasischke and Turetsky 2006; Rocha et al. 2020) and are rare along the southern coastal region. Because MODIS can fail to detect smaller, lower intensity, or subsurface fires, such as those in peatlands (Sirin and Medvedeva 2022), not all wildfires that occurred during the study time period are represented in the MODIS FRP dataset.
Study area in Alaska showing the extent of burned areas (1940–2022) derived from vector datasets of fire perimeters and overlaid on forested land cover (Ruefenacht et al. 2008). Fires recorded by MODIS satellites during this study (2003–2022) are color-coded according to the dominant consolidated existing vegetation type (EVT) within their perimeters; the color does not indicate 100% coverage of the labeled EVT. Vegetation classes not included in project analyses because of their small areal representation are grouped into ‘Other’.
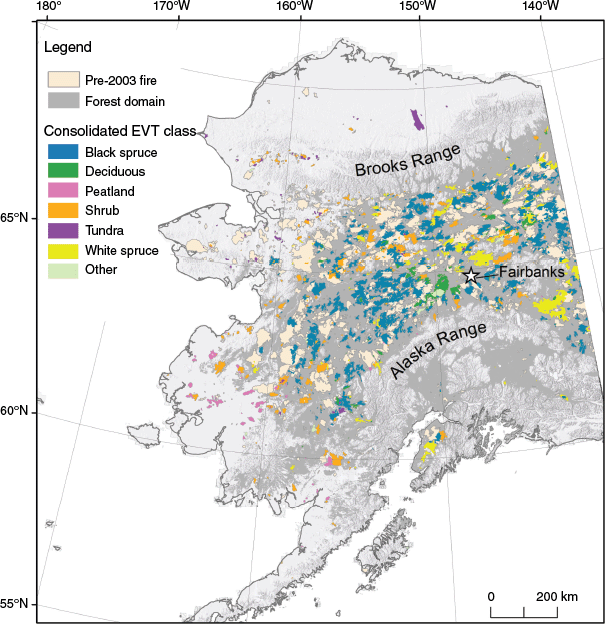
Strong moisture and temperature gradients exist across Alaska (Dissing and Verbyla 2003), with polar air masses dominating the north and oceanic air masses along the coast (Alexander et al. 2012). The interior climate is continental, with cold winters and relatively warm summers; the mean temperatures in January and July for Fairbanks are −23.2°C and 16.5°C, respectively (Shulski and Wendler 2007; Alaska Climate Research Center 2022a). Compared to 1981–2010 normals, 1991–2020 average annual temperatures have risen 0.6–1.7°C across the state (Alaska Climate Research Center 2022b).
Data sources
LANDFIRE data products (www.landfire.gov) provide geospatial information relevant for wildfire management and modeling at a 30-m spatial resolution (Rollins 2009). We reclassified LANDFIRE existing vegetation type (EVT), representative of the dominant plant community within a given area (Picotte et al. 2019), from 103 fine-scale vegetation units to 10 consolidated EVT classes that share broadly similar biogeographical and burn characteristics (Table 1 lists the consolidated set of variables; for the complete list of original classes, see Supplementary Table S1). All subsequent analyses used the consolidated classes. The 2001 LANDFIRE Alaska dataset pre-dates the fire events included in this study, ensuring that land cover classes represent ground-based conditions present when the study fires occurred. For Alaska, the 2001 LANDFIRE EVT layer was originally produced using predictive landscape models (Picotte et al. 2019). The vegetation map units are based on NatureServe’s terrestrial ecological systems classification (Comer et al. 2003), which is a nationally consistent set of plant community types that tend to co-occur within landscapes (Rollins 2009). We downloaded the AK 2001 [LF 1.0.5] EVT dataset (LANDFIRE 2011) from the LANDFIRE website and uploaded it to the cloud-computing environment Google Earth Engine (GEE) (Gorelick et al. 2017). This version addressed inconsistencies in the original 2001 product by incorporating ancillary Landsat-based data sources, expert knowledge, topographic filtering, and aggregation of vegetation classes (Connot 2014).
Consolidated EVT class | Proportion of consolidated EVT classes contained within MODIS 1-km active fire pixels in Alaska from 2003 to 2022 (%) | |
---|---|---|
Black spruce | 40.3 | |
White spruce | 21.6 | |
Deciduous (aspen, balsam poplar, birch) | 15.3 | |
Shrubland | 11.9 | |
Tundra | 2.9 | |
Wetland | 2.7 | |
Peatland | 2.6 | |
Other (agriculture, barren, developed, snow, water) | 1.5 | |
Grassland | 1.2 | |
Non-spruce conifer (e.g. hemlock, sitka spruce) | <0.1 |
The area and proportion of each class burned were derived by aggregating the EVT composition across all MODIS 1-km active fire pixels in Alaska from 2003 to 2022.
We retrieved all MODIS Thermal Anomalies and Fire Daily 1-km Collection 6 data (Terra: MOD14A1.006; Aqua: MYD14A1.006) from January 2003 to November 2022 over Alaska in GEE. This dataset provides daily fire detection bands and a maximum FRP product at 1-km resolution. Given the offset launch dates of the Terra (December 1999) and Aqua (May 2002) satellites, we excluded 2001 and 2002 from our analysis to maintain consistency of data availability (e.g. Cattau et al. 2020). Terra and Aqua acquire images at different times of the day (local equatorial crossing at 1000/2200 and 1330/0130, respectively), which can lead to slightly higher FRP values for Aqua (Giglio et al. 2006). We retained nominal- and high-confidence fire pixels and reduced any overlapping images by selecting the MODIS pixel with the highest FRP value. We removed fires <404 ha to match the size threshold we set in the Bureau of Land Management (BLM) Alaska Interagency Coordination Center (AICC) Fire History geospatial database (Bureau of Land Management Alaska Interagency Coordination Center 2021), as described in the Fire History section. We then calculated the area of individual LANDFIRE EVT classes within each pixel boundary. Datasets were assembled for each calendar year and exported with the FRP value (megawatts, MW) of each fire pixel, as well as its centroid location (latitude and longitude), acquisition viewing angle (degrees), and area of each constituent EVT class (m2).
We obtained mapped wildfire perimeters in Alaska for 1940–2021 from the BLM AICC Fire History database. Perimeters are derived from a variety of sources and AICC mapping standards have changed over time due to the refinement of imaging capabilities and mapping precision; we limited our study to fires ≥404 ha, which is the smallest fire size consistently mapped throughout the database period. The resulting dataset included all non-prescribed fires in Alaska recorded from 1940 to 2021 larger than 404 ha. Because the compilation of AICC 2022 fire perimeters was not available at the time of our study, we obtained perimeters for 2022 from the National Interagency Fire Center (NIFC) Wildland Fire Interagency Geospatial Services (WFIGS) Group (National Interagency Fire Center 2022). The same selection criteria were applied to the WFIGS set of fire perimeters in Alaska before merging the datasets to create a single file of 1940–2022 polygons. In a GIS environment (ESRI ArcMap ver. 10.5.1; Python ver. 2.7.12), we converted all overlapping fire extents to discrete, non-overlapping polygons with distinct burn histories. Each polygon was attributed with information about all contributing fires, including start date, burn duration, and fire size. We exported the polygon dataset to GEE and the tabular dataset to R (ver. 4.0.5) (R Core Team 2021), where we derived aggregate fire characteristics by ecoregion, geographic location, and fire return interval. The compilation of the extent and number of fires over time demonstrates the high interannual variability in Alaska fire seasons since 1940 (Fig. 2).
Fire history records in Alaska from 1940 to 2022 show temporal variability in (top) number of fires and (bottom) area burned, with right-aligned 10-year moving average lines. Fire perimeters are derived from the Alaska Interagency Coordination Center and the Wildland Fire Interagency Geospatial Services Group.
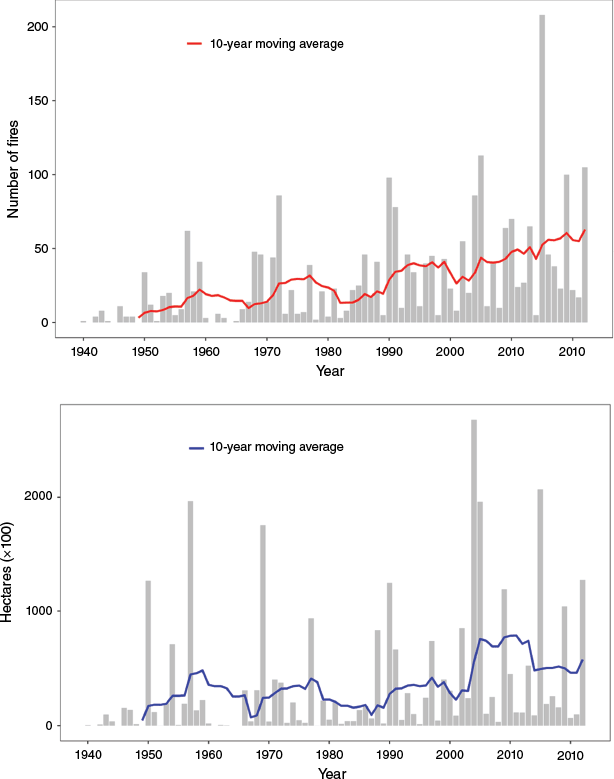
The resolution difference between MODIS pixels (1 km) and EVT land cover data (30 m) means that each FRP value potentially represents a wide variety of land cover (Fig. 3). To associate each pixel with a single land cover type, we assigned it the consolidated EVT class representing the largest proportion of the pixel area (Fig. 3d, top). This ‘winner-takes-all’ approach subsumes existing land cover heterogeneity, so we retained the original fractional EVT classes to set minimum thresholds of land cover coverage for analysis purposes (Fig. 3d, bottom). No pixels experienced intervening fires between the LANDFIRE data collected in 2001 and the date of FRP observation, ensuring that the consolidated EVT classes represented the vegetation type burned.
Attribution of MODIS pixels with fire radiative power (FRP), fire history, and consolidated existing vegetation type (EVT), illustrated with a single fire example. (a) MODIS active fire pixels associated with a fire perimeter were retained for analysis if (b) the centroid of the pixel was within the fire boundary. (c) The FRP value in each retained pixel was associated with the consolidated EVT classes within its extent, summarized as (d, top) majority EVT type and (d, bottom) individual proportions. (e) The timing of overlapping wildfires was similarly associated with pixels that had centroids within the burn perimeter.
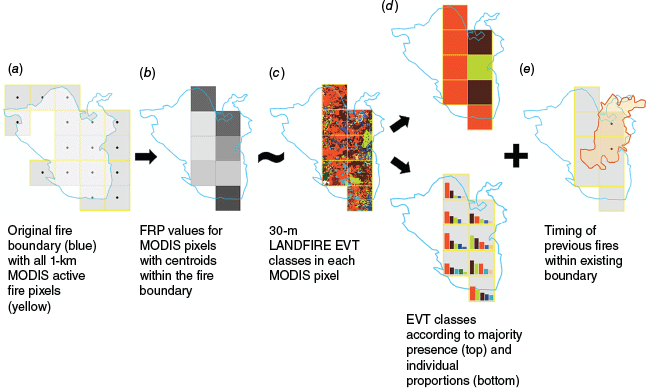
We derived topographic information from the US Geological Survey (USGS) 3D Elevation Program (3DEP) 10-m raster digital elevation model (U.S. Geological Survey 2020). We calculated mean slope, aspect, and elevation with the Terrain Analysis in Google Earth Engine (TAGEE) package (Safanelli et al. 2020) for a 1-km2 area around each centroid point. The DEM was smoothed with a bilinear Gaussian filter prior to deriving terrain attributes and resampled to 90-m resolution to facilitate computation within GEE. We calculated mean aspect using the arctangent of pixel-wise sine and cosine values (Fisher 1993) and grouped results into 45° classes: north (0°–22.5°, 337.5°–360°); north-east (22.5°–67.5°); east (67.5°–112.5°); south-east (112.5°–157.5°); south (157.5°–202.5°); south-west (202.5°–247.5°); west (247.5°–292.5°); and north-west (292.5°–337.5°).
Processing of raster and vector data
Not all fire polygons were uniformly covered by active fire pixels either because MODIS sensors failed to detect an active fire or because they accurately recorded the absence of fire. Neither fire history database differentiates between burned and unburned areas within a fire perimeter, so a fire polygon may contain a mosaic of both (French et al. 2004). To associate FRP data with fire perimeters, we removed pixels with a centroid outside a fire boundary; we associated remaining pixels with the fire history at their centroid location (Fig. 3b). For each pixel with multiple fire occurrences throughout the data record, we calculated the maximum number of times burned (Burn Number) and the intervening time between fires (Burn Interval). We compiled the annual sum of area burned (Annual Area Burned) to represent fire activity levels. We additionally retrieved the day of year (DOY) of fire onset for each burn. Portions of the processing workflow were completed on the USGS Yeti supercomputer (Falgout and Gordon 2021) provided by the USGS Core Science Analytics, Synthesis, & Libraries (CSASL) Advanced Research Computing (ARC) group.
Each MODIS swath encompasses ±55°. Due to longer line-of-sight distances and the Earth’s curvature, at high scan angles, the pixel ground footprint increases in the along-track direction until pixels overlap by almost 50% at the scan edge; i.e. the ‘bow-tie’ effect (Wolfe et al. 1998). The duplicated area over which the values are retrieved results in proportionally higher minimum detectable FRP values (Freeborn et al. 2011; Kumar et al. 2011). To minimize the effect on our analysis, we removed all pixels with acquisition angles >24°, which reduced the number of included pixels from 117,542 to 27,727 (a 76% decrease).
Statistical analysis
We tested for FRP differences among consolidated EVT classes using non-parametric Kruskal–Wallis analysis of variance (Kruskal and Wallis 1952) followed by Dunn’s tests (Dunn 1964) with Bonferroni–Holm correction (Holm 1979) for post hoc assessment of significant pairwise differences (R package dunn.test, Dinno 2017). Non-parametric tests were applied because the data did not meet parametric assumptions for data normality and equal class sizes and variances. To isolate the effects of discrete vegetation class on detected FRP, we included only EVT classes in which at least 10 active fire pixels contained a high-proportion majority (≥75%) of that land cover type. In this and other tests, the requirement of a minimum number of pixels with a given EVT proportion balanced two goals: to reduce the possibility that burning of other vegetation types influenced the detected FRP and to provide an adequate number of samples for statistical inference purposes.
We used Kruskal–Wallis rank sum tests to assess differences in FRP by Burn Number and Burn Interval. For tests relating to Burn Number, we again included classes where ≥10 pixels contained ≥75% of a single consolidated EVT. For Burn Interval we binned the elapsed time into 20-years intervals, which required a reduction of the criteria to n ≥ 10 pixels with ≥65% of the EVT class because fewer qualifying samples existed across the intervals. We used Kruskal–Wallis tests with Dunn’s z-test approximation to evaluate differences within class across time and between classes at discrete time intervals.
We used a random forest regression model (RF; Breiman 2001) to investigate the relative importance of vegetation class, fire history, and selected ancillary variables on FRP (Table 2). Independent model variables included dominant vegetation type, Burn Number, and Burn Interval with metrics representing fire seasonality (DOY of fire onset), location (latitude and longitude of pixel centroid), topography (mean aspect, elevation, and slope), and annual area burned. We used consolidated vegetation class as a categorical variable, with a required minimum EVT proportion of 50% in each pixel. We lowered the threshold EVT percentage to ensure sufficient pixels were distributed across the range of each explanatory variable because models can underperform when input data are sparse (e.g. Coulston et al. 2016). We removed pixels with Burn Number >3 because few samples existed in the highest burn classes and centered and scaled all numeric data.
Variable | Definition | Units | |
---|---|---|---|
Annual area burned | Total area burned each calendar year across all 1-km MODIS pixels used in the vegetation analysis | km2 | |
Burn interval | Elapsed time between successive fires in a MODIS pixel | Years | |
Burn number | Number of times a MODIS pixel has burned since 1940 | NA | |
Fire seasonality | Date of fire start reported in the fire perimeter datasets | Day of year | |
Aspect | Mean orientation of pixels clockwise from north within a 1-km2 area around the pixel centroid | Cardinal directions in 45° intervals (N, NE, E, SE, S, SW, W, NW) | |
Elevation | Mean height of terrain above sea level within a 1-km2 area around the pixel centroid | m | |
Slope | Mean percent change of elevation within a 1-km2 area around the pixel centroid | % | |
Latitude | Latitude of MODIS pixel centroid | ° | |
Longitude | Longitude of MODIS pixel centroid | ° |
Two of the more important parameters in RF models are: (1) mtry, which is the number of randomly selected predictor variables evaluated at each split; and (2) ntree, which is the total number of decision trees generated. To evaluate the optimal values for each, we conducted a 10-fold cross validation across candidate values and assessed model root mean squared error (RMSE) and coefficient of determination (R2). Based on the results, we trained the model with mtyr = 4 and ntree = 400. We evaluated variable importance with the impurity metric, which is the variance of the responses (Wright and Ziegler 2017); i.e. how much the mean-squared error of the model would increase if the predictor in question were randomized (Breiman 2001). To assist with model interpretation, we generated partial dependence plots, which show the effect of individual predictor variables on the response while holding all other predictors at their average value (Cutler et al. 2007). RF models, construction, and interpretation were conducted with R packages ranger (Wright and Ziegler 2017), tidymodels (Kuhn and Wickham 2020), and DALEX (Biecek 2018).
Results
Fire intensity varies among some vegetation classes
Kruskal–Wallis tests showed a significant difference (χ2 = 122.9, d.f. = 5, P < 0.001) in FRP among the six consolidated EVT classes that met the criteria for this analytical step (≥10 pixels containing ≥75% of the EVT) (Fig. 4; Table S2). Pairwise tests for significant differences indicated significantly higher (P < 0.001) FRP for white spruce (Picea glauca (Moench) Voss) (median 157.6; standard error [s.e.] 23.7) than for all other classes (Table 3). The FRP for black spruce was not significantly different (P > 0.05, median 89.4; s.e. 7.7) than the FRP for deciduous vegetation but was significantly higher (P < 0.05) than the FRP for all other EVT classes excluding white spruce. The FRP values for peatland (median 47.4; s.e. 7.8), shrubland (median 68.9; s.e. 17.6), and tundra (median 76.1; s.e. 17.6) were not significantly different from each other. Among all EVT classes, black spruce was distinguished by a large number of monospecific pixels available for analysis (3111) and numerous fire detections in the upper intensity range (Fig. 4; Fig. S1).
Boxplots of fire radiative power distributions derived from MODIS active fire pixels in Alaska from 2003 to 2022, grouped by consolidated existing vegetation type (EVT). Shown are only those EVT classes in which ≥10 pixels have a high-proportion majority (≥75%) of that class. The vertical line is the median, the triangle is the mean, boxes encompass the interquartile range (IQR), and whiskers extend 1.5× IQR beyond the lowest and highest IQR value. Boxplots labeled with the same letters indicate that the means do not differ significantly (P < 0.05). Individual points show the underlying distribution of the data, with the number of included pixels listed along the y-axis.
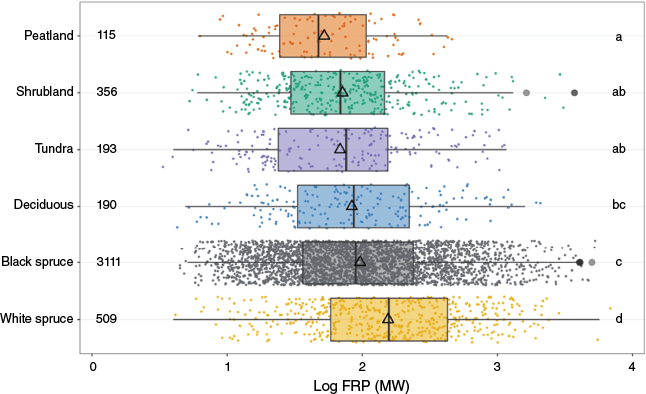
EVT comparison | Z | P-value | |
---|---|---|---|
Black spruce – Deciduous | 1.26 | 0.410 | |
Black spruce – Peatland | 4.92 | <0.001 | |
Black spruce – Shrubland | 4.09 | 0.004 | |
Black spruce – Tundra | 3.20 | 0.011 | |
Black spruce – White spruce | −7.56 | <0.001 | |
Deciduous – Peatland | 3.16 | 0.011 | |
Deciduous – Shrubland | 1.50 | 0.538 | |
Deciduous – Tundra | 1.40 | 0.487 | |
Deciduous – White spruce | −5.36 | <0.001 | |
Peatland – Shrubland | −2.23 | 0.156 | |
Peatland – Tundra | −1.96 | 0.253 | |
Peatland – White spruce | −8.03 | <0.001 | |
Shrubland – Tundra | 0.09 | 0.925 | |
Shrubland – White spruce | −8.55 | <0.001 | |
Tundra – White spruce | −7.08 | <0.001 |
Fire history metrics are weakly linked to FRP
Burn Number had little effect on FRP values over the 19-year period of available data. Shrubland (χ2 = 4.23, d.f. = 1, P = 0.034) and tundra (χ2 = 6.94, d.f. = 1, P = 0.0084) were the only classes where FRP was significantly lower (P < 0.05) for repeat fires (Fig. 5, lower FRP for Burn Number >1 vs Burn Number = 1). Although repeat burns were common in several consolidated EVT classes, few of the fires occurred in pixels with highly monospecific (≥75%) land cover composition, which was the qualifying threshold for this analysis. Black spruce was the only EVT class for which more than 10 monospecific pixels existed with Burn Number >2 (Fig. 5).
Fire radiative power (FRP) associated with the number of burns in consolidated existing vegetation type (EVT) classes. Each category represents the highest burn number recorded for a MODIS pixel and thus comprises independent sets of data. Shown are only those EVT classes containing n ≥ 10 pixels with ≥75% of that class. Different letters represent a significant (P < 0.05) difference in FRP. In each plot, the horizontal line is the median, the triangle is the mean, boxes encompass the interquartile range (IQR), and whiskers extend 1.5× IQR beyond the lowest and highest IQR value. Points show outliers beyond 1.5× IQR and the number of included pixels is shown along the x-axis.
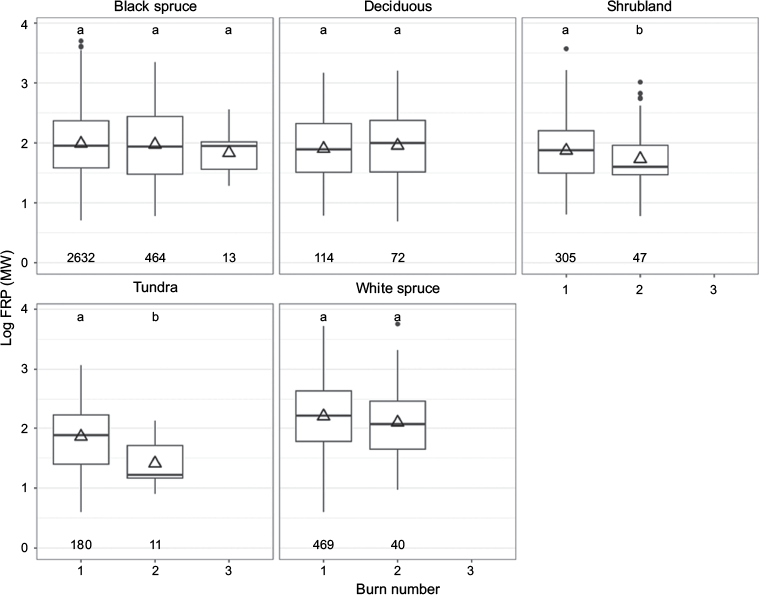
The analysis of the effect of fire-free interval was constrained to four classes that had pixels with an EVT proportion of ≥65% in more than one 20-years interval (Table S3). FRP patterns varied among the classes according to the length of fire-free interval but showed few statistically significant differences (Fig. 6). Only black spruce FRP values were statistically distinct among Burn Intervals, with the 20–40 years FRP significantly lower than FRP in the 1–20 years (Z = −2.52, P = 0.046), 40–60 years (Z = −4.08, P < 0.001), and 60–80 years (Z = −3.14, P = 0.008) intervals. Significant differences between vegetation classes existed within the 20–40 years interval only, where deciduous FRP was higher than black spruce (Z = 2.87, P = 0.028), shrubland (Z = 3.03, P = 0.02) and tundra (Z = 3.27, P = 0.01), and white spruce FRP was higher than tundra (Z = 3.06, P = 0.02).
Fire radiative power (FRP) dynamics over time grouped by consolidated existing vegetation type (EVT). Shown are mean FRP values within 20-year intervals of elapsed time since the previous documented fire. Each temporal bin comprises n ≥ 10 pixels, each containing ≥65% of the given EVT. The tundra class contains ≥10 pixels in only one temporal bin (20–40 years). Vertical bars indicate ±1 s.e.
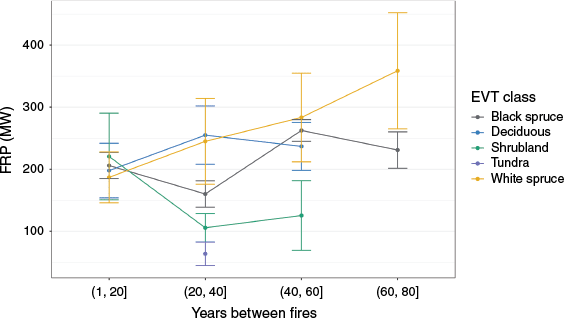
Geography and seasonality are important predictors of FRP
In the RF model, which had an expanded number of classes due to the requirement of a ≥50% EVT proportion (Table S4), variables other than vegetation class and fire history provided the highest explanatory power for FRP: longitude, latitude, DOY, elevation, slope, and aspect (Fig. 7). Burn Interval and Burn Number had minor relative influence, while the vegetation class for each burned pixel was least important. Total out-of-bag variance explained by the model was 46.1% with a RMSE of 264.6.
Variable importance in the random forest regression model of fire radiative power (FRP). Data are derived from MODIS active fire pixels in which ≥50% of the consolidated existing vegetation type (EVT) consists of a single class.
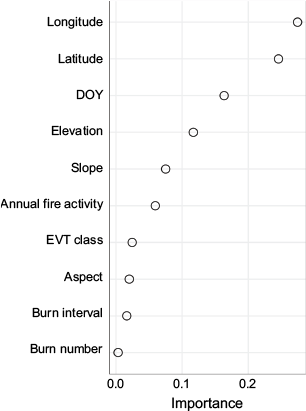
Partial dependence plots show the importance of predictor variables on FRP for individual vegetation types (Fig. 8), largely grouped by forest vs non-forest (shrubland, tundra, peatland) class. For forest vegetation classes, timing of fire onset was positively related to FRP such that FRP increased with increasing DOY until approximately mid-June (DOY 170), after which timing of fire onset was largely associated with lower FRP. In contrast, onset of fire in non-forest vegetation types showed an earlier peak, then a decreasing influence on FRP that was countered by a slight increasing effect on FRP from early July (~DOY 190) through mid-August (DOY 230). Aspect had the largest influence on FRP for peatland and tundra classes, with a pronounced increase for more southern aspects, while FRP increased slightly for white spruce on north-facing slopes. For all vegetation types, FRP increased with elevation until ~200 m and with slope until angles of 3°–5°. Geographic effects included a sharp increase of FRP for latitudes above 66°N for forest vegetation, a decrease in FRP with higher latitudes for non-forest vegetation, and an increase in FRP from west to east for all classes. For white spruce and black spruce, FRP increased with longer fire-free intervals; other vegetation types showed little trend. There was no evident, consistent relationship between FRP and annual area burned.
Partial dependence plots for input variables to the random forest model, by consolidated existing vegetation type (EVT). Each plot shows the marginal effect of the explanatory variable (x axes) on predicted fire radiative power (ŷ; log FRP; y axes) when all other variables are held constant at their mean value. Included pixels contain ≥50% of each EVT.
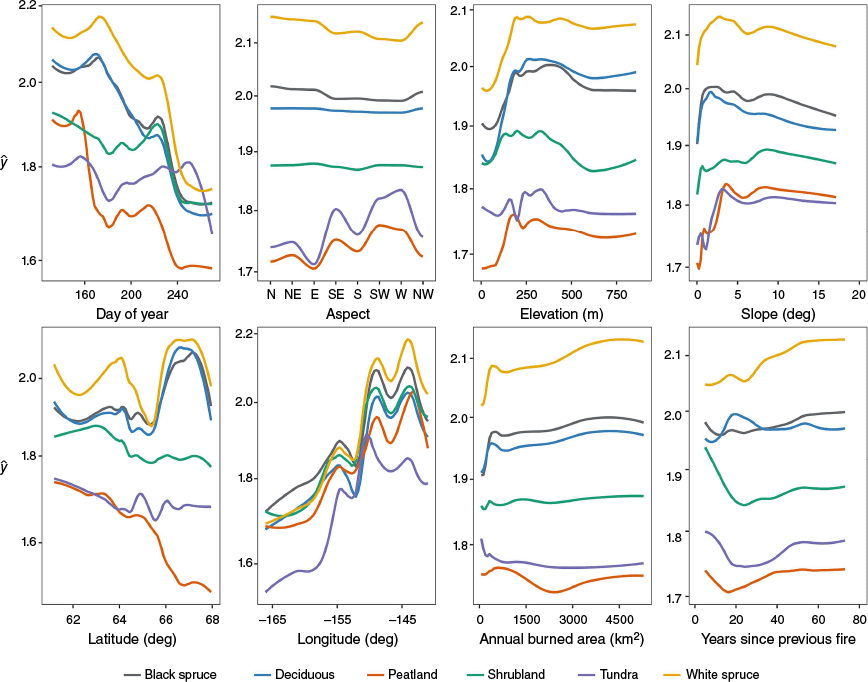
Discussion
FRP is different among vegetation functional types but not individual vegetation classes
Our results show that FRP is different between forest and non-forest land cover across Alaska. In ecosystems with lower amounts of aboveground fuel (peatland, shrubland, tundra), fires were generally less intense than those in tree-dominated classes (black spruce, white spruce, deciduous). This positive relationship with aboveground biomass is consistent with observations of FRP differentiability among other land cover types (Giglio et al. 2006, 2016).
Similar to (Wooster and Zhang 2004), we found that FRP can also differentiate among boreal forest classes. Here, fires in pixels dominated by black spruce and broadleaf deciduous stands had statistically lower FRP distributions than those in white spruce-dominated pixels. This finding highlights the control of canopy, surface, and belowground biomass properties on fire intensity. Broadleaf deciduous systems are highly productive, but have elevated moisture content and low flammability (Van Cleve et al. 1983; Cumming 2001; Kasischke and Hoy 2012). Black spruce forests, in contrast, are the least productive of the boreal forest types, with carbon allocation heavily weighted towards storage in belowground organic layers (Kasischke and French 1997; Alexander and Mack 2016), yielding low aboveground biomass (Van Cleve et al. 1983; Rignot et al. 1994). Wildfires in boreal black spruce forests tend to kill overstorey trees and remove most aboveground vegetation (Van Cleve et al. 1983; Viereck 1983) and can consume surface organic layers and belowground organic matter (Kasischke et al. 2010). The low flammability/high productivity of deciduous stands offsets the high flammability/low productivity of black spruce, such that their FRP distributions are statistically indistinguishable in this study. However, white spruce combines high aboveground biomass and net primary production (Van Cleve et al. 1983; Gower et al. 2001) with lower moisture content and a greater propensity to crown fires (Vanderlinden 1996; Ascoli et al. 2020). The additive effects of these characteristics resulted in statistically higher FRP distributions (Fig. 4).
Despite significant differences in FRP between vegetation functional groups, individual classes had limited discriminatory power in the random forest model. We attribute this result to the substantial overlap of low- to mid-range FRP values among EVT classes. Although classes with varying aboveground fuel loads showed general differentiation at the high end of their FRP ranges, they broadly shared lower value ranges due to the numerous factors that suppress observed fire intensity, such as overstorey vegetation canopy, fuel conditions, cloud cover, thick smoke, and rain (Eckmann et al. 2008; Ichoku et al. 2008; Freeborn et al. 2014). Thus, a low-range FRP value could represent a fire in a tundra ecosystem, or it could equally be retrieved from a wet black spruce system with heavy cloud cover. Because no single value conclusively indicates a particular class, discrimination among FRP distributions might be possible only in an aggregate manner if ancillary information about the landscape is unavailable. A more effective bottom-up analysis of FRP patterns could incorporate other vegetation-specific parameters to increase the separability of the distributions.
FRP is weakly associated with fire history metrics
We found little association between FRP and fire history metrics for most EVT classes. Burn Number influenced FRP only in shrub- or tundra-dominated areas, where fires in previously burned pixels were significantly less intense than fires in pixels with no recorded history of fire. In the RF model, Burn Number and Burn Interval were not important predictors of FRP. Burn Interval was not significantly different among most EVT classes and decadal intervals; the only clear distinction occurred in the 20–40 years time interval, in which all EVT classes were separable. Black spruce FRP values in that interval were also statistically distinct from all other time intervals.
In tundra and shrub-dominated systems, we observed a decrease in FRP with increasing Burn Number that likely reflects the complexity and variability of tundra fire recovery. Tundra fires can lead to divergent pathways of vegetation response, with fire severity promoting shrub presence (Lantz et al. 2013), suppressing it (Hollingsworth et al. 2021), or having only a site-mediated positive effect (Chen et al. 2021), all of which could affect subsequent fire intensity. The significant reduction of FRP in reburned tundra pixels is consistent with documentation of multi-decadal post-burn recovery tracks (Frost et al. 2020). The relatively abbreviated tundra fire return interval in this study (mean 32.9 ± 12.6 years) places most reburned pixels within a recovery timeframe. The results are less aligned with other studies that have shown more rapid recovery of surface and vegetation properties; tundra surface properties quantified by remote sensing metrics achieved parity with pre-burned areas within 5 years (Zhou et al. 2019) to one decade (Rocha et al. 2020). The decrease in FRP may also be indicative of an atypical vegetation recovery pathway because repeated burns within anomalously short intervals can disrupt normal recovery trajectories (Racine et al. 1987; Hayes and Buma 2021). The dynamics of shrubs in tundra ecosystems may inform the post-fire dynamics of shrubland-only ecosystems. Shrub abundance and density have been positively linked to fire occurrence (Lantz et al. 2010), while other studies have observed contradictory relationships, in which high-severity fire led to an increase in grass dominance (Hollingsworth et al. 2021).
The lack of a more prominent role of fire legacy in determining the FRP of subsequent fires in forested settings was unexpected given trends in boreal ecosystem recovery. Model predictions of relationships among succession, fuel conditions, and fire behavior in black spruce forests (Cronan and Jandt 2008) lead to the expectation of a commensurate association of FRP with fire history, as does the critical role of time since fire as a driver of boreal vegetation composition and abundance (Jorgensen et al. 2023). One explanation may be the overlap of recovery timelines and fire return intervals. Under a post-disturbance replacement scenario in black spruce stands, deciduous growth dominates until the development of a black spruce canopy within 25–30 years (Viereck 1973), after which biomass gradually increases until stand maturity at 45–70 years (Viereck et al. 1983; Cronan and Jandt 2008). For black spruce-dominated pixels, the mean interval between consecutive fires in this study (44.7 ± 18.0 years) coincides with the onset of stand maturity, which could allow for biomass accumulation that supports fire intensities indistinguishable from those of an initial burn. A similar rationale could also explain the lack of burn number effect for deciduous stands (return interval: 42.5 ± 11.0 years). In contrast, white spruce ecosystems are dominated by aspen and birch for up to 100 years after a fire (Viereck and Little 1972; Cumming 2001). The white spruce FRI in this study (46.2 ± 16.6 years) placed any reburn within a mid-successional deciduous phase, particularly for pixels with two or more recorded fires. No effect of prior fire is evident despite the clear separability of fire intensity values between deciduous and white spruce.
Non-vegetation factors play a key role in determining FRP
The strongest predictors of FRP were factors linked to vegetation distributions, such as latitude, longitude, and topography, as well as DOY of fire onset. We attribute the influence of latitude and longitude to the tight connection between vegetation aboveground biomass and geography across Alaska. The pronounced east-west moisture and temperature gradient (Dissing and Verbyla 2003) determines primary production across the longitudinal range, with forest in the comparatively temperate interior giving way to tundra and peatland along the western coast. Similarly, the climatic gradient along the broad latitudinal expanse (~15°) dictates the progression northward from interior boreal forest to herbaceous-dominated tundra, which also varies with latitude (Walker 2000). FRP patterns in Alaska are thus most consistently determined by general fire location; substantial variations in fire intensity are less likely within interior Alaska forests, for instance, than they are between the interior and the Arctic north.
Date of fire onset substantially influences FRP because it serves as an integrated measure of the ground and weather conditions that control fire ignition throughout the year. Dryness of available fuel influences the degree of combustion, which affects FRP retrieval (Ichoku et al. 2008). Fire intensity increases throughout the spring and early summer as wetter conditions caused by thawing ground ice and melting snow cover become progressively drier (Kasischke and French 1997; Barrett et al. 2016) before seasonal rains arrive later in July. For forest-dominated pixels, FRP peaks in early July and decreases thereafter, while shrubland, peatland, and tundra classes steadily increase from July onward before a later-season decrease. Because small and large fire activity years can exhibit differences in burned vegetation composition (Kasischke and Hoy 2012; Dash et al. 2016), fire severity (Barrett et al. 2011), and consumption of organic layer (Turetsky et al. 2011), we also generated random forest models stratified by extremes of fire activity (low: <25% quartile; high: >75% quartile). High activity years showed the influence of atypically late-season forest fires fostered by exceptionally warm and dry conditions (Bureau of Land Management Alaska Interagency Coordination Center 2004), but in low-activity years, patterns in non-forested ecosystems resembled the decreasing FRP patterns seen in forested environments (Supplementary Fig. S2). The high relevance of date of fire onset points to the importance of weather conditions as a top-down control on fine-scale variations in fire intensity.
Topographic factors of aspect, elevation, and slope moderately influenced FRP; these parameters, like geographical location, are highly associated with vegetation distributions (Rignot et al. 1994; Turetsky et al. 2011) and fire behavior (Kasischke et al. 2002; Duffy et al. 2007; Roland et al. 2019). Our expectation was for a stronger correlation given the documented interactions between fire occurrence and terrain parameters in tundra, at least (Masrur et al. 2022). Here the coarse spatial resolution of the MODIS data is likely a contributing factor because topographic parameters have less importance at scales >1 km2 (Dissing and Verbyla 2003).
Uncertainties and limitations
The results highlight an intrinsic limitation of the retrospective nature of this analysis; we do not have comprehensive information about the vegetation and burn characteristics of fires prior to the MODIS era. For a pixel to be classified as majority white spruce at the time of the EVT dataset production, earlier fires were likely low severity or patchy enough to allow for broad-scale persistence of white spruce communities. Our imposition of vegetation proportional thresholds likely excluded prior white spruce-dominated pixels that experienced more severe or widespread fires and are currently within the deciduous phase of a replacement trajectory. The lack of detailed information about prior fire events and landscape composition prevents accurately tracking fire intensity of all vegetation possibilities along a succession timeline. Similarly, environmental factors such as soil moisture play a key role in mediating plant recovery (Jorgensen et al. 2023), emphasizing the difficulty in analyzing the influence of fire history parameters without information about local conditions.
Much of the difficulty in parameterizing FRP values can be attributed to the intractable problem of reducing a complex interplay of highly dynamic factors to a single representative value. The extreme variability of wildfire in space and time contributes to the uncertainty of what is burning and where it is located at the time of image acquisition. Various types of fires can exist simultaneously across a MODIS 1-km2 pixel; an active fire pixel likely contains a spatially heterogeneous mix of smoldering, flaming, or non-burned areas that contribute different amounts of radiative energy (Kaufman et al. 1998; Wooster et al. 2005). The type and growth status of vegetation play a prominent role in determining fire intensity. Of the pixels examined here, 15,133 (55%) have three or more vegetation types that each cover >10% of the pixel area. For pixels with mosaics of vegetation types rather than monoculture stands, the detected FRP reflects the combination of their burn characteristics. Changing environmental conditions, such as weather, smoke cover, and cloud cover, also control FRP retrieval. The combination of these highly variable conditions means that FRP is poorly represented by a single discrete measurement in time; even the 15-min sampling interval of geostationary satellites may not characterize FRP adequately (Boschetti and Roy 2009). Moreover, because FRP is an integrated summation of radiative events within a discrete area, a pixel that encompasses a tundra fire may have the same FRP as a highly localized burn in a forest setting (Eckmann et al. 2008).
Compounding the factors that dynamically amplify or attenuate fire intensity is uncertainty associated with each of the three primary sources of data used in this study: (1) vegetation type; (2) fire history; and (3) FRP. The accuracy of the original LANDFIRE 2002 vegetation map was low (DeVelice 2012) prior to its refinement in 2016, but even recent error analyses of the revised LANDFIRE EVT dataset in the Pacific Northwest returned accuracies of only 52% (Picotte et al. 2019). Our consolidation of classes to broad functional types may have minimized some inaccuracies. The estimation of the proportion of black spruce burned from 2003 to 2022 (40.8%) is here close to the overall representation of the land cover class in AK forests (44%) (Van Cleve et al. 1983), and within annual ranges found in other studies (32.3–45.1%) (Kasischke and Hoy 2012).
Data availability and the various methods used to compile fire history perimeters over time undoubtedly affected observed patterns in FRP dynamics. Where fire return intervals are measurably longer than the available fire history record—for example, in tundra ecosystems that historically had extended fire-free intervals of 150 years or longer (Higuera et al. 2011)—previous fires may not be detectable in this analysis. For instance, although few of the tundra-dominated pixels had fire-free intervals longer than 40–50 years, fire-free intervals of 10–40 years were well represented. The pattern of documentation is presumably due to the extreme remoteness of the tundra region in Alaska and the lack of Landsat Thematic Mapper data prior to 1982, which could complicate the identification and accurate delineation of fire extents (e.g. Baughman 2024). The uncertain reliability of the fire perimeter record is also a likely factor in the lack of separability of FRP values in the 40–60 years and 60–80 years ranges (Fig. 6). Although the EVT classes could have potentially exhibited the same burn intensity given their individual regrowth trajectories, fires 40–80 years prior to our study time frame would have occurred between 1942 and 1982, which again largely predates satellite-based fire delineation.
As with any remote sensing platform, orbital and sensor constraints result in imperfect observational records. These limitations affect the accuracy and completeness of the MODIS FRP datasets. MODIS fails to detect active fires that burn between overpasses (Giglio 2007) or are blocked by atmospheric contamination (e.g. clouds, smoke cover) or overstory vegetation (Barrett et al. 2016). Although there is no theoretical upper bound on the fire size or intensity that MODIS sensors can detect, there is a practical lower limit. Small (<100 m2) and low-intensity (<10 MW) fires may not emit enough radiance to be detected (Giglio et al. 2003; Freeborn et al. 2014; Sperling et al. 2020), and these minimum thresholds increase with scan angle (Freeborn et al. 2011). The systemic bias towards higher radiance fires means that vegetation types with lower biomass likely have right-shifted FRP distributions.
Conclusion
In this study, we explored the feasibility of determining practical links between fire intensity – as quantified by the MODIS-derived metric of fire radiative power – with vegetation classes and fire history in Alaska. The results confirmed our expectation that relationships exist, though the impact of fire legacy was less robust than anticipated. Variations in recorded FRP were broadly consistent with distinguishing characteristics of aboveground biomass and fuel conditions between forest and shrub, tundra, and peatland vegetation groups. Fire history factors of the number and frequency of previous fires were of negligible influence on fire intensity over the abbreviated period of analysis. Bottom-up parameters such as geographic location and topography were the most important independent variables in the random forest model, likely because of the highly deterministic role of climate and topography on vegetation type across the state.
These general associations validate the premise that FRP data warrant further investigation into their role as signifiers of near-real-time fire dynamics and subsequent impacts. The extreme spatial and temporal variability of FRP means that no single value is meaningful; instead, it can only be interpreted in the context of ancillary information. Incorporating up-to-date maps of land cover and fuel biomass with site and weather conditions could allow researchers to further parameterize the expected range of fire intensities for a boreal ecosystem. The continuation of MODIS with the Suomi-NPP Visible Infrared Imaging Radiometer Suite (VIIRS) satellite could be critical for future studies because of the higher-resolution FRP product (375/750 m) and lack of the scan angle issues inherent to MODIS (Li et al. 2018). The expanded baseline of information, combined with field validation of post-fire effects, could allow us to better understand the potential implications of anomalously high or low FRP values in different environmental settings. Future research may benefit from those improvements as research focus shifts from investigating fire intensity as an outcome of precursor conditions to determining its role in shaping vegetation recovery.
Data availability
Extracted FRP values and associated fire perimeter and vegetation class information are available in Walker et al. (2025).
Acknowledgements
Any use of trade, product, or firm names is for descriptive purposes only and does not imply endorsement by the U.S. Government. The authors thank Sandra Haire (Haire Laboratory for Landscape Ecology) and Lisa Saperstein (US Fish and Wildlife Service) for constructive reviews, and Jennifer Barnes (National Park Service, Alaska Region) and Jennifer Hrobak (Bureau of Land Management, Alaska Fire Service) for their invaluable contributions to our understanding of fire behavior, fire effects, and fire science priorities in Alaska. We also thank the two anonymous reviewers whose insightful comments helped to improve this manuscript.
References
Alaska Climate Research Center (2022a) Temperature Normals. [Dataset]. Available at https://akclimate.org/data/air-temperature-normals/
Alaska Climate Research Center (2022b) Climate Change in Alaska. Available at https://akclimate.org/climate-change-in-alaska/ [accessed 5 May 2022]
Alexander HD, Mack MC (2016) A canopy shift in interior Alaskan boreal forests: consequences for above- and belowground carbon and nitrogen pools during post-fire succession. Ecosystems 19, 98-114.
| Crossref | Google Scholar |
Alexander HD, Mack MC, Goetz S, Beck PSA, Belshe ET (2012) Implications of increased deciduous cover on stand structure and aboveground carbon pools of Alaskan boreal forests. Ecosphere 3(5), 45.
| Crossref | Google Scholar |
Ascoli D, Hacket-Pain A, LaMontagne JM, Cardil A, Conedera M, Maringer J, Motta R, Pearse IS, Vacchiano G (2020) Climate teleconnections synchronize Picea glauca masting and fire disturbance: evidence for a fire-related form of environmental prediction. Journal of Ecology 108(3), 1186-1198.
| Crossref | Google Scholar |
Baltzer JL, Day NJ, Walker XJ, Greene D, Mack MC, Alexander HD, Arseneault D, Barnes J, Bergeron Y, Boucher Y, Bourgeau-Chavez L, Brown CD, Carrière S, Howard BK, Gauthier S, Parisien MA, Reid KA, Rogers BM, Roland C, Sirois L, Stehn S, Thompson DK, Turetsky MR, Veraverbeke S, Whitman E, Yang J, Johnstone JF (2021) Increasing fire and the decline of fire adapted black spruce in the boreal forest. Proceedings of the National Academy of Sciences of the United States of America 118, e2024872118.
| Crossref | Google Scholar | PubMed |
Barrett K, Kasischke ES (2013) Controls on variations in MODIS fire radiative power in Alaskan boreal forests: implications for fire severity conditions. Remote Sensing of Environment 130, 171-181.
| Crossref | Google Scholar |
Barrett K, Kasischke ES, McGuire AD, Turetsky MR, Kane ES (2010) Modeling fire severity in black spruce stands in the Alaskan boreal forest using spectral and non-spectral geospatial data. Remote Sensing of Environment 114(7), 1494-1503.
| Crossref | Google Scholar |
Barrett K, Mcguire AD, Hoy EE, Kasischke ES (2011) Potential shifts in dominant forest cover in interior Alaska driven by variations in fire severity. Ecological Applications 21(7), 2380-2396.
| Crossref | Google Scholar | PubMed |
Barrett K, Loboda T, McGuire AD, Genet H, Hoy E, Kasischke E (2016) Static and dynamic controls on fire activity at moderate spatial and temporal scales in the Alaskan boreal forest. Ecosphere 7(11), e01572.
| Crossref | Google Scholar |
Baughman C (2024) Corrected fire perimeters of Alaska’s National Wildlife Refuges. [U.S. Geological Survey data release]. 10.5066/P143UCFQ
Beck PSA, Goetz SJ, Mack MC, Alexander HD, Jin Y, Randerson JT, Loranty MM (2011) The impacts and implications of an intensifying fire regime on Alaskan boreal forest composition and albedo. Global Change Biology 17(9), 2853-2866.
| Crossref | Google Scholar |
Biecek P (2018) DALEX: Explainers for complex predictive models in R. Journal of Machine Learning Research 19(84), 1-5.
| Google Scholar |
Boschetti L, Roy DP (2009) Strategies for the fusion of satellite fire radiative power with burned area data for fire radiative energy derivation. Journal of Geophysical Research Atmospheres 114(20), D20302.
| Crossref | Google Scholar |
Breiman L (2001) Random forests. Machine Learning 45, 5-32.
| Crossref | Google Scholar |
Bret-Harte MS, Mack MC, Shaver GR, Huebner DC, Johnston M, Mojica CA, Pizano C, Reiskind JA (2013) The response of Arctic vegetation and soils following an unusually severe tundra fire. Philosophical transactions of the Royal Society of London. Series B, Biological Sciences 368(1624), 20120490.
| Crossref | Google Scholar | PubMed |
Buma B, Hayes K, Weiss S, Lucash M (2022) Short‑interval fires increasing in the Alaskan boreal forest as fire self‑regulation decays across forest types. Scientific Reports 12, 4901.
| Crossref | Google Scholar |
Bureau of Land Management Alaska Interagency Coordination Center (2021) Alaska Fire History Perimeter Polygons, 1940-2021. [GIS Dataset]. Available at https://fire.ak.blm.gov/predsvcs/maps.php
Cattau ME, Wessman C, Mahood A, Balch JK (2020) Anthropogenic and lightning-started fires are becoming larger and more frequent over a longer season length in the U.S.A. Global Ecology and Biogeography 29(4), 668-681.
| Crossref | Google Scholar |
Chapin FS, III, McGuire AD, Randerson J, Pielke R, Sr., Baldocchi D, Hobbie SE, Roulet N, Eugster W, Kasischke E, Rastetter EB, Zimov SA, Running SW (2000) Arctic and boreal ecosystems of western North America as components of the climate system. Global Change Biology 6, 211-223.
| Crossref | Google Scholar | PubMed |
Chen Y, Hu FS, Lara MJ (2021) Divergent shrub-cover responses driven by climate, wildfire, and permafrost interactions in Arctic tundra ecosystems. Global Change Biology 27, 652-663.
| Crossref | Google Scholar | PubMed |
Connot JA (2014) ‘LANDFIRE 2001 and 2008 refresh: geographic area report: Alaska.’ (U.S. Geological Survey: Reston, VA) Available at https://pubs.usgs.gov/publication/70103363
Coulston JW, Blinn CE, Thomas VA, Wynne RH (2016) Approximating prediction uncertainty for random forest regression models. Photogrammetric Engineering and Remote Sensing 82(3), 189-197.
| Crossref | Google Scholar |
Cronan J, Jandt R (2008) How succession affects fire behavior in boreal black spruce forest of interior Alaska. BLM Alaska Technical Report 59. (U.S. Department of the Interior, Bureau of Land Management: Anchorage, Alaska) Available at https://www.blm.gov/sites/default/files/documents/files/Library_Alaska_TechnicalReport59.pdf
Cumming SG (2001) Forest type and wildfire in the Alberta boreal mixedwood: what do fires burn? Ecological Applications 11(1), 97-110.
| Crossref | Google Scholar |
Cutler DR, Edwards TC, Jr, Beard KH, Cutler A, Hess KT, Gibson J, Lawler JJ (2007) Random forests for classification in ecology. Ecology 88(11), 2783-2792.
| Crossref | Google Scholar | PubMed |
Dash CB, Fraterrigo JM, Hu FS (2016) Land cover influences boreal-forest fire responses to climate change: geospatial analysis of historical records from Alaska. Landscape Ecology 31, 1781-1793.
| Crossref | Google Scholar |
DeVelice RL (2012) Accuracy of the LANDFIRE Alaska Existing Vegetation map over the Chugach National Forest. Available at https://landfire.gov/sites/default/files/documents/LANDFIRE_ak_110evt_accuracy_summary_013012.pdf
Dinno A (2017) dunn.test: Dunn’s Test of Multiple Comparisons Using Rank Sums. Available at https://CRAN.R-project.org/package=dunn.test
Dissing D, Verbyla DL (2003) Spatial patterns of lightning strikes in interior Alaska and their relations to elevation and vegetation. Canadian Journal of Forest Research 33(5), 770-782.
| Crossref | Google Scholar |
Duffy PA, Epting J, Graham JM, Rupp TS, McGuire AD (2007) Analysis of Alaskan burn severity patterns using remotely sensed data. International Journal of Wildland Fire 16(3), 277-284.
| Crossref | Google Scholar |
Dunn OJ (1964) Multiple comparisons using rank sums. Technometrics 6(3), 241-252.
| Crossref | Google Scholar |
Dyrness CT, Norum RA (1983) The effects of experimental fires on black spruce forest floors in interior Alaska. Canadian Journal of Forest Research 13, 879-893.
| Crossref | Google Scholar |
Eckmann TC, Roberts DA, Still CJ (2008) Using multiple endmember spectral mixture analysis to retrieve subpixel fire properties from MODIS. Remote Sensing of Environment 112(10), 3773-3783.
| Crossref | Google Scholar |
Falgout JT, Gordon J (2021) ‘USGS Advanced Research Computing, USGS Yeti Supercomputer.’ (U.S. Geological Survey) 10.5066/F7D798MJ
Freeborn PH, Wooster MJ, Roberts G (2011) Addressing the spatiotemporal sampling design of MODIS to provide estimates of the fire radiative energy emitted from Africa. Remote Sensing of Environment 115(2), 475-489.
| Crossref | Google Scholar |
Freeborn PH, Wooster MJ, Roy DP, Cochrane MA (2014) Quantification of MODIS fire radiative power (FRP) measurement uncertainty for use in satellite-based active fire characterization and biomass burning estimation. Geophysical Research Letters 41(6), 1988-1994.
| Crossref | Google Scholar |
French NHF, Goovaerts P, Kasischke ES (2004) Uncertainty in estimating carbon emissions from boreal forest fires. Journal of Geophysical Research 109, D14S08.
| Crossref | Google Scholar |
French NHF, Jenkins LK, Loboda TV, Flannigan M, Jandt R, Bourgeau-Chavez LL, Whitley M (2015) Fire in arctic tundra of Alaska: past fire activity, future fire potential, and significance for land management and ecology. International Journal of Wildland Fire 24, 1045-1061.
| Crossref | Google Scholar |
Frost GV, Loehman RA, Saperstein LB, Macander MJ, Nelson PR, Paradis DP, Natali SM (2020) Multi-decadal patterns of vegetation succession after tundra fire on the Yukon-Kuskokwim Delta, Alaska. Environmental Research Letters 15(2), 025003.
| Crossref | Google Scholar |
Gauthier S, Bernier P, Kuuluvainen T, Shvidenko AZ, Schepaschenko DG (2015) Boreal forest health and global change. Science 349(6250), 819-822.
| Crossref | Google Scholar | PubMed |
Gibson CM, Turetsky MR, Cottenie K, Kane ES, Houle G, Kasischke ES (2016) Variation in plant community composition and vegetation carbon pools a decade following a severe fire season in interior Alaska. Journal of Vegetation Science 27(6), 1187-1197.
| Crossref | Google Scholar |
Giglio L (2007) Characterization of the tropical diurnal fire cycle using VIRS and MODIS observations. Remote Sensing of Environment 108(4), 407-421.
| Crossref | Google Scholar |
Giglio L, Descloitres J, Justice CO, Kaufman YJ (2003) An enhanced contextual fire detection algorithm for MODIS. Remote Sensing of Environment 87(2–3), 273-282.
| Crossref | Google Scholar |
Giglio L, Csiszar I, Justice CO (2006) Global distribution and seasonality of active fires as observed with the Terra and Aqua Moderate Resolution Imaging Spectroradiometer (MODIS) sensors. Journal of Geophysical Research 111(2), G02016.
| Crossref | Google Scholar |
Giglio L, Schroeder W, Justice CO (2016) The collection 6 MODIS active fire detection algorithm and fire products. Remote Sensing of Environment 178, 31-41.
| Crossref | Google Scholar | PubMed |
Gorelick N, Hancher M, Dixon M, Ilyushchenko S, Thau D, Moore R (2017) Google Earth Engine: planetary-scale geospatial analysis for everyone. Remote Sensing of Environment 202, 18-27.
| Crossref | Google Scholar |
Gower ST, Krankina O, Olson RJ, Apps M, Linder S, Wang C (2001) Net primary production and carbon allocation patterns of boreal forest ecosystems. Ecological Applications 11(5), 1395-1411.
| Crossref | Google Scholar |
Gustine DD, Brinkman TJ, Lindgren MA, Schmidt JI, Rupp TS, Adams LG (2014) Climate-driven effects of fire on winter habitat for caribou in the Alaskan-Yukon Arctic. PLoS One 9(7), e100588.
| Crossref | Google Scholar | PubMed |
Hayes K, Buma B (2021) Effects of short‐interval disturbances continue to accumulate, overwhelming variability in local resilience. Ecosphere 12, e03379.
| Crossref | Google Scholar |
Higuera PE, Chipman ML, Barnes JL, Urban MA, Hu FS (2011) Variability of tundra fire regimes in Arctic Alaska millennial‐scale patterns and ecological implications. Ecological Applications 21(8), 3211-3226.
| Crossref | Google Scholar |
Hollingsworth TN, Breen AL, Hewitt RE, Mack MC (2021) Does fire always accelerate shrub expansion in Arctic tundra? Examining a novel grass-dominated successional trajectory on the Seward Peninsula. Arctic, Antarctic, and Alpine Research 53(1), 93-109.
| Crossref | Google Scholar |
Holm S (1979) A simple sequentially rejective multiple test. Scandinavian Journal of Statistics 6(2), 65-70.
| Google Scholar |
Holsinger LM, Parks SA, Saperstein LB, Loehman RA, Whitman E, Barnes J, Parisien MA (2022) Improved fire severity mapping in the North American boreal forest using a hybrid composite method. Remote Sensing in Ecology and Conservation 8(2), 222-235.
| Crossref | Google Scholar |
Ichoku C, Giglio L, Wooster MJ, Remer LA (2008) Global characterization of biomass-burning patterns using satellite measurements of fire radiative energy. Remote Sensing of Environment 112(6), 2950-2962.
| Crossref | Google Scholar |
Johnstone JF (2006) Response of boreal plant communities to variations in previous fire-free interval. International Journal of Wildland Fire 15(4), 497-508.
| Crossref | Google Scholar |
Johnstone JF, Chapin III FS, Foote J, Kemmett S, Price K, Viereck L (2004) Decadal observations of tree regeneration following fire in boreal forests. Canadian Journal of Forest Research 34, 267-273.
| Crossref | Google Scholar |
Johnstone JF, Hollingsworth TN, Chapin FSI, Mack MC (2010) Changes in fire regime break the legacy lock on successional trajectories in Alaskan boreal forest. Global Change Biology 16(4), 1281-1295.
| Crossref | Google Scholar |
Johnstone JF, Celis G, Chapin FS, Hollingsworth TN, Jean M, Mack MC (2020) Factors shaping alternate successional trajectories in burned black spruce forests of Alaska. Ecosphere 11(5), e03129.
| Crossref | Google Scholar |
Jones B, Kolden C, Jandt R, Abatzoglou J, Urban F, Arp C (2009) Fire behavior, weather, and burn severity of the 2007 Anaktuvuk River tundra fire, North Slope, Alaska. Arctic, Antarctic, and Alpine Research 41(3), 309-316.
| Crossref | Google Scholar |
Jones MW, Abatzoglou JT, Veraverbeke S, Andela N, Lasslop G, Forkel M, Smith AJP, Burton C, Betts RA, van der Werf GR, Sitch S, Canadell JG, Santín C, Kolden C, Doerr SH, Le Quéré C (2022) Global and regional trends and drivers of fire under climate change. Reviews of Geophysics 60(3), e2020RG000726.
| Crossref | Google Scholar |
Jorgensen AG, Alfaro-Sánchez R, Cumming SG, White AL, Degré-Timmons GÉ, Day N, Turetsky M, Johnstone JF, Walker XJ, Baltzer JL (2023) The influence of postfire recovery and environmental conditions on boreal vegetation. Ecosphere 14(7), e4605.
| Crossref | Google Scholar |
Kasischke ES, French NHF (1997) Constraints on using AVHRR composite index imagery to study patterns of vegetation cover in boreal forests. International Journal of Remote Sensing 18(11), 2403-2426.
| Crossref | Google Scholar |
Kasischke ES, Hoy EE (2012) Controls on carbon consumption during Alaskan wildland fires. Global Change Biology 18, 685-699.
| Crossref | Google Scholar |
Kasischke ES, Turetsky MR (2006) Recent changes in the fire regime across the North American boreal region — Spatial and temporal patterns of burning across Canada and Alaska. Geophysical Research Letters 33(9), L09703.
| Crossref | Google Scholar |
Kasischke ES, Williams D, Barry D (2002) Analysis of the patterns of large fires in the boreal forest region of Alaska. International Journal of Wildland Fire 11(2), 131-144.
| Crossref | Google Scholar |
Kasischke ES, Turetsky MR, Ottmar RD, French NHF, Hoy EE, Kane ER (2008) Evaluation of the composite burn index for assessing fire severity in Alaskan black spruce forests. International Journal of Wildland Fire 17, 515-526.
| Crossref | Google Scholar |
Kasischke ES, Verbyla DL, Rupp TS, McGuire AD, Murphy KA, Jandt R, Barnes JL, Hoy EE, Duffy PA, Calef M, Turetsky MR (2010) Alaska’s changing fire regime — implications for the vulnerability of its boreal forests. Canadian Journal of Forest Research 40(7), 1313-1324.
| Crossref | Google Scholar |
Kaufman YJ, Remer LA, Ottmar RD, Ward DE, Li R-R, Kleidman R, Fraser RS, Flynn L, McDougal D, Shelton G (1996) Relationship between remotely sensed fire intensity and rate of emission of smoke: SCAR-C experiment. In ‘Global Biomass Burning’. (Ed. J Levine) pp. 685–696. (MIT Press: Cambridge, MA, USA)
Kaufman YJ, Justice CO, Flynn LP, Kendall JD, Prins EM, Giglio L, Ward DE, Menzel WP, Setzer AW (1998) Potential global fire monitoring from EOS-MODIS. Journal of Geophysical Research: Atmospheres 103(D24), 32215-32238.
| Crossref | Google Scholar |
Keeley JE (2009) Fire intensity, fire severity and burn severity: a brief review and suggested usage. International Journal of Wildland Fire 18, 116-126.
| Crossref | Google Scholar |
Kruskal WH, Wallis WA (1952) Use of ranks in one-criterion variance analysis. Journal of the American Statistical Association 47(260), 583-621.
| Crossref | Google Scholar |
Kuhn M, Wickham H (2020) Tidymodels: a collection of packages for modeling and machine learning using tidyverse principles. Available at https://www.tidymodels.org
Kumar SS, Roy DP, Boschetti L, Kremens R (2011) Exploiting the power law distribution properties of satellite fire radiative power retrievals: a method to estimate fire radiative energy and biomass burned from sparse satellite observations. Journal of Geophysical Research Atmospheres 116(19), D19303.
| Crossref | Google Scholar |
Kurkowski TA, Mann DH, Rupp TS, Verbyla DL (2008) Relative importance of different secondary successional pathways in an Alaskan boreal forest. Canadian Journal of Forest Research 38(7), 1911-1923.
| Crossref | Google Scholar |
Lantz TC, Gergel SE, Henry GHR (2010) Response of green alder (Alnus viridis subsp. fruticosa) patch dynamics and plant community composition to fire and regional temperature in north‐western Canada. Journal of Biogeography 37(8), 1597-1610.
| Crossref | Google Scholar |
Lantz TC, Marsh P, Kokelj SV (2013) Recent shrub proliferation in the Mackenzie Delta uplands and microclimatic implications. Ecosystems 16, 47-59.
| Crossref | Google Scholar |
Lentile LB, Holden ZA, Smith AMS, Falkowski MJ, Hudak AT, Morgan P, Lewis SA, Gessler PE, Benson NC (2006) Remote sensing techniques to assess active fire characteristics and post-fire effects. International Journal of Wildland Fire 15(3), 319-345.
| Crossref | Google Scholar |
Li F, Zhang X, Kondragunta S, Csiszar I (2018) Comparison of fire radiative power estimates from VIIRS and MODIS observations. Journal of Geophysical Research: Atmospheres 123(9), 4545-4563.
| Crossref | Google Scholar |
Macander MJ, Nelson PR, Nawrocki TW, Frost GV, Orndahl KM, Palm EC, Wells AF, Goetz SJ (2022) Time-series maps reveal widespread change in plant functional type cover across Arctic and boreal Alaska and Yukon. Environmental Research Letters 17(5), 054042.
| Crossref | Google Scholar |
Mack MC, Treseder KK, Manies KL, Harden JW, Schuur EAG, Vogel JG, Randerson JT, Chapin FS (2008) Recovery of aboveground plant biomass and productivity after fire in mesic and dry black spruce forests of interior Alaska. Ecosystems 11(2), 209-225.
| Crossref | Google Scholar |
Markon C, Gray S, Berman M, Eerkes-Medrano L, Hennessy T, Huntington H, Littell J, McCammon M, Thoman R, Trainor S (2018) Alaska. In ‘Impacts, Risks, and Adaptation in the United States: Fourth National Climate Assessment. Vol. II’. (Eds DR Reidmiller, CW Avery, DR Easterling, KE Kunkel, KLM Lewis, TK Maycock, BC Stewart) pp. 1185–1241. (U.S. Global Change Research Program: Washington, DC, USA)
Masrur A, Petrov AN, DeGroote J (2018) Circumpolar spatio-temporal patterns and contributing climatic factors of wildfire activity in the Arctic tundra from 2001–2015. Environmental Research Letters 13, 014019.
| Crossref | Google Scholar |
Masrur A, Taylor A, Harris L, Barnes J, Petrov A (2022) Topography, climate and fire history regulate wildfire activity in the Alaskan tundra. Journal of Geophysical Research: Biogeosciences 127, e2021JG006608.
| Crossref | Google Scholar |
Michalek JL, French NHF, Kasischke ES, Johnson RD, Colwell JE (2000) Using Landsat TM data to estimate carbon release from burned biomass in an Alaskan spruce forest complex. International Journal of Remote Sensing 21(2), 323-338.
| Crossref | Google Scholar |
Murphy KA, Reynolds JH, Koltun JM (2008) Evaluating the ability of the differenced Normalized Burn Ratio (dNBR) to predict ecologically significant burn severity in Alaskan boreal forests. International Journal of Wildland Fire 17(4), 490-499.
| Crossref | Google Scholar |
National Interagency Fire Center (2022) Wildland Fire Interagency Geospatial Services (WFIGS) Interagency Fire Perimeters to Date. [GIS Dataset]. Available at https://data-nifc.opendata.arcgis.com/datasets/nifc::wfigs-interagency-fire-perimeters/about
Nelson JL, Zavaleta ES, Chapin FS (2008) Boreal fire effects on subsistence resources in Alaska and adjacent Canada. Ecosystems 11(1), 156-171.
| Crossref | Google Scholar |
Palm EC, Suitor MJ, Joly K, Herriges JD, Kelly AP, Hervieux D, Russell KLM, Bentzen TW, Larter NC, Hebblewhite M (2022) Increasing fire frequency and severity will increase habitat loss for a boreal forest indicator species. Ecological Applications 32(3), 2549.
| Crossref | Google Scholar | PubMed |
Picotte JJ, Dockter D, Long J, Tolk B, Davidson A, Peterson B (2019) LANDFIRE Remap prototype mapping effort: developing a new framework for mapping vegetation classification, change, and structure. Fire 2, 35.
| Crossref | Google Scholar |
Racine CH, Johnson LA, Viereck LA (1987) Patterns of vegetation recovery after tundra fires in northwestern Alaska, U.S.A. Arctic and Alpine Research 19(4), 461-469.
| Crossref | Google Scholar |
Rantanen M, Karpechko AY, Lipponen A, Nordling K, Hyvärinen O, Ruosteenoja K, Vihma T, Laaksonen A (2022) The Arctic has warmed nearly four times faster than the globe since 1979. Communications Earth & Environment 3(1), 168.
| Crossref | Google Scholar |
R Core Team (2021) ‘R: A language and environment for statistical computing.’ (R Foundation for Statistical Computing: Vienna, Austria) Available at https://www.R-project.org/
Rignot E, Williams C, Viereck L (1994) Radar estimates of aboveground biomass in boreal forests of interior Alaska. IEEE Transactions on Geoscience and Remote Sensing 32(5), 1117-1124.
| Crossref | Google Scholar |
Rocha AV, Loranty MM, Higuera PE, Rastetter EB, Goetz SJ, Shaver GR (2020) The footprint of Alaskan tundra fires during the past half-century: implications for surface properties and radiative forcing. Environmental Research Letters 7, 044039.
| Crossref | Google Scholar |
Roland CA, Schmidt JH, Winder SG, Stehn SE, Nicklen EF (2019) Regional variation in interior Alaskan boreal forests is driven by fire disturbance, topography, and climate. Ecological Monographs 89(3), e01369.
| Crossref | Google Scholar |
Rollins MG (2009) LANDFIRE: a nationally consistent vegetation, wildland fire, and fuel assessment. International Journal of Wildland Fire 18, 235-249.
| Crossref | Google Scholar |
Ruefenacht B, Finco MV, Nelson MD, Czaplewski R, Helmer EH, Blackard JA, Holden GR, Lister AJ, Salajanu D, Weyermann D, Winterberger K (2008) Conterminous U.S. and Alaska forest type mapping using Forest Inventory and Analysis data. Photogrammetric Engineering and Remote Sensing 74(11), 1379-1388.
| Crossref | Google Scholar |
Rupp TS, Olson M, Adams LG, Dale BW, Joly K, Henkelman J, Collins WB, Starfield AM (2006) Simulating the influences of various fire regimes on caribou winter habitat. Ecological Applications 16(5), 1730-1743.
| Crossref | Google Scholar | PubMed |
Safanelli JL, Poppiel RR, Ruiz LFC, Bonfatti BR, Mello FAO, Rizzo R, Demattê JAM (2020) Terrain analysis in Google Earth Engine: a method adapted for high-performance global-scale analysis. ISPRS International Journal of Geo-Information 9, 400.
| Crossref | Google Scholar |
Schindler DW, Lee PG (2010) Comprehensive conservation planning to protect biodiversity and ecosystem services in Canadian boreal regions under a warming climate and increasing exploitation. Biological Conservation 143(7), 1571-1586.
| Crossref | Google Scholar |
Screen JA, Simmonds I (2010) The central role of diminishing sea ice in recent Arctic temperature amplification. Nature 464(7293), 1334-1337.
| Crossref | Google Scholar | PubMed |
Sirin A, Medvedeva M (2022) Remote sensing mapping of peat-fire-burnt areas: iIdentification among other wildfires. Remote Sensing 14(1), 194.
| Crossref | Google Scholar |
Smith AMS, Wooster MJ (2005) Remote classification of head and backfire types from MODIS fire radiative power and smoke plume observations. International Journal of Wildland Fire 14(3), 249-254.
| Crossref | Google Scholar |
Sperling S, Wooster MJ, Malamud BD (2020) Influence of satellite sensor pixel size and overpass time on undercounting of cerrado/savannah landscape-scale fire radiative power (FRP): an assessment using the MODIS airborne simulator. Fire 3(2), 11.
| Crossref | Google Scholar |
Turetsky MR, Kane ES, Harden JW, Ottmar RD, Manies KL, Hoy E, Kasischke ES (2011) Recent acceleration of biomass burning and carbon losses in Alaskan forests and peatlands. Nature Geoscience 4(1), 27-31.
| Crossref | Google Scholar |
U.S. Geological Survey (2020) 3D Elevation Program 10-meter Resolution Digital Elevation Model. Available at https://code.earthengine.com
Van Cleve K, Dyrness CT, Viereck LA, Fox J, Chapin FS, Oechel W (1983) Taiga ecosystems in interior Alaska. BioScience 33(1), 39-44.
| Crossref | Google Scholar |
Vanderlinden LA (1996) Applying stand replacement prescribed fire in Alaska. In ‘The use of fire in forest restoration: A general session at the annual meeting of the Society for Ecological Restoration’, 1995, Seattle, WA. (Eds CC Hardy, SF Arno) pp. 78–80. (U.S. Department of Agriculture, Forest Service, Intermountain Research Station: Seattle, WA)
Veraverbeke S, Rogers BM, Goulden ML, Jandt RR, Miller CE, Wiggins EB, Randerson JT (2017) Lightning as a major driver of recent large fire years in North American boreal forests. Nature Climate Change 7(7), 529-534.
| Crossref | Google Scholar |
Veraverbeke S, Dennison P, Gitas I, Hulley G, Kalashnikova O, Katagis T, Kuai L, Meng R, Roberts D, Stavros N (2018) Hyperspectral remote sensing of fire: state-of-the-art and future perspectives. Remote Sensing of Environment 216(May), 105-121.
| Crossref | Google Scholar |
Viereck LA (1973) Wildfire in the taiga of Alaska. Quaternary Research 3(3), 465-495.
| Crossref | Google Scholar |
Viereck LA, Dyrness CT, Van Cleve K, Foote MJ (1983) Vegetation, soils, and forest productivity in selected forest types in interior Alaska. Canadian Journal of Forest Research 13, 703-720.
| Crossref | Google Scholar |
Walker DA (2000) Hierarchical subdivision of Arctic tundra based on vegetation response to climate, parent material and topography. Global Change Biology 6(Suppl. 1), 19-34.
| Crossref | Google Scholar | PubMed |
Walker J, Loehman R, Smith B, Soulard C (2025) MODIS Fire Radiative Power data compiled from active fires in Alaska, 2003-2022. [U.S. Geological Survey data release] 10.5066/P1DRAPCK
Wendler G, Shulski M (2009) A century of climate change for Fairbanks, Alaska. Arctic 62(3), 295-300.
| Crossref | Google Scholar |
Wolfe RE, Roy DP, Vermote E (1998) MODIS land data storage, gridding, and compositing methodology: Level 2 Grid. IEEE Transactions on Geoscience and Remote Sensing 36(4), 1324-1338.
| Crossref | Google Scholar |
Wooster MJ (2002) Small-scale experimental testing of fire radiative energy for quantifying mass combusted in natural vegetation fires. Geophysical Research Letters 29(21), 2027.
| Crossref | Google Scholar |
Wooster MJ, Zhang YH (2004) Boreal forest fires burn less intensely in Russia than in North America. Geophysical Research Letters 31(20), L20505.
| Crossref | Google Scholar |
Wooster MJ, Roberts G, Perry GLW, Kaufman YJ (2005) Retrieval of biomass combustion rates and totals from fire radiative power observations: FRP derivation and calibration relationships between biomass consumption and fire radiative energy release. Journal of Geophysical Research Atmospheres 110(24), D24311.
| Crossref | Google Scholar |
Wright MN, Ziegler A (2017) ranger: A fast implementation of random forests for high dimensional data in C++ and R. Journal of Statistical Software 77(1), 1-17.
| Crossref | Google Scholar |
York A, Bhatt US, Gargulinski E, Grabinski Z, Jain P, Soja A, Thoman RL, Ziel R (2020) Wildland Fire in High Northern Latitudes. NOAA Arctic Report Card 2020 1–11. 10.25923/2gef-3964
Zhou Z, Liu L, Jiang L, Feng W, Samsonov SV (2019) Using long-term SAR backscatter data to monitor post-fire vegetation recovery in tundra environment. Remote Sensing 11(19), 2230.
| Crossref | Google Scholar |