Improving the accuracy of predictions for cow survival by multivariate evaluation model
M. Khansefid


A Agriculture Victoria Research, AgriBio, Centre for AgriBioscience, 5 Ring Road, Bundoora, Vic. 3083, Australia.
B School of Applied Systems Biology, La Trobe University, Bundoora, Vic. 3083, Australia.
C Corresponding author. Email: majid.khansefid@agriculture.vic.gov.au
Animal Production Science - https://doi.org/10.1071/AN21128
Submitted: 4 March 2021 Accepted: 12 May 2021 Published online: 7 July 2021
Journal compilation © CSIRO 2021 Open Access CC BY-NC-ND
Abstract
Context: Cow survival measures the ability of cows to survive from the current to subsequent lactation. In addition to economic gain, genetic selection for survival could improve animal welfare by increasing the adaptability and resilience of the cows to both environmental and health challenges. However, survival is a complex trait because it results from a diverse range of reasons for culling of cows from the herd. Consequently, the accuracy of genetic predictions of direct survival are often low.
Aims: Our aim was to increase the accuracy of predictions of survival in Holstein and Jersey sires by including important predictor traits in multi-trait evaluation models.
Methods: Phenotypic and genetic correlations between survival trait deviations (TDs) and 35 routinely measured traits (including milk yield, fertility and type traits) were estimated using bivariate sire models. Survival TDs for 538 394 Holstein and 63 839 Jersey cows were used in our study; these cows or their close relatives also had milk, fertility and type traits records between 2002 and 2019. These genetic parameters were required to assess the potential usefulness of predictor traits for the prediction of survival.
Key results: Survival was genetically correlated with milk, fat and protein yields, overall type, composite mammary system and fertility TDs in both Holstein and Jersey. Further, most of the type traits related to feet and legs, and rump, were also correlated with survival TDs in Jersey. For sires, the accuracy of predictions for survival increased by 0.05 for Holsteins (from 0.54 to 0.59) and for Jerseys (from 0.48 to 0.53) through the use of multivariate models compared with univariate models.
Conclusions: Survival was genetically associated with traits affecting voluntary and involuntary culling and when included in multi-trait genetic evaluation models, they moderately improved the accuracy of genetic prediction of survival.
Implications: Predictor traits can be used to increase the accuracy of predictions of survival through the use of multi-trait models. The inclusion of breed-specific predictor traits should be considered, especially for Jerseys in genetic evaluations of survival.
Additional keywords: longevity, functional traits, conformation traits, milk traits, dairy cattle.
Introduction
Cow survival reflects the ability of a cow to avoid being removed from the herd because of voluntary culling (poor milk production) or involuntary culling (infertility, illnesses, injuries and death; Weigel et al. 2003). Survival is the second-most important trait after protein yield in the Australian balanced performance index, mainly because it has a large direct contribution in reducing replacement costs (Byrne et al. 2016). High-producing cows that last longer in dairy herds are likely to produce a higher proportion of milk yield as mature cows (Pritchard et al. 2013). Moreover, selecting for survival is associated with improved fertility and animal welfare through increased productive life and improved resistance to infectious diseases, such as mastitis, in addition to reducing environmental emissions (Garnsworthy 2004; Pritchard et al. 2013; Barkema et al. 2015; Pryce and Bell 2017).
Culling decisions made by farmers are often based on the profitability of cows in the herd. The costs and benefits of culling cows from a herd depend on several factors, such as age, days in milk and pregnancy status, the production system (seasonal pasture-based or intensive), the price of milk and female replacements and farmers’ desire to expand their herds (Weigel et al. 2003; Giordano et al. 2011). Hence, genetic evaluation of productive life is challenging, because cow survival is highly affected by management decisions.
The heritability (h2) of survival measured as the reappearance of a cow in the next lactation is low. Haile-Mariam and Pryce (2015) reported that the h2 of survival from first to second lactation in Australian Holsteins ranged between 0.03 and 0.07 in ~20 years (1993–2012). The h2 for other longevity traits, such as days of productive life, has been reported to be low (~0.05) as well (Pritchard et al. 2013). However, the estimated h2 from survival models (0.10–0.18) were often marginally higher than those from linear models (0.03–0.11) because the data from existing cows in the herd can be handled more appropriately in survival analytical models (Forabosco et al. 2009; Sasaki 2013). Although the h2 for survival is generally low, this trait is still quite important in breeding programs due to its large economic value compared with other traits (Byrne et al. 2016).
Given the low h2 and, subsequently, expectation of low to modest accuracies of estimated breeding values (EBVs) for survival, traits correlated with survival can be used for indirect selection, or as predictor traits in a multi-trait genetic evaluation model (Forabosco et al. 2009). In fact, one of the main incentives to select for improved conformation and fertility is to reduce the chance of involuntary culling of cows, which allows the farmers to increase the selection pressure for milk production traits (Weigel et al. 2003). However, the correlation between survival and other traits, such as culling reasons, may vary over time, or across lactations, which could limit the usefulness of exploiting predictor traits in improving the accuracy of EBVs for survival (Haile-Mariam and Pryce 2015; Workie et al. 2021).
The aims of our research were to (1) estimate the phenotypic and genetic correlations between survival and milk production traits (milk, fat and protein yields), 25 linear and five composite conformation traits, body condition score (BCS) and fertility (calving interval) trait deviations (TDs) and then (2) test the usefulness of the most promising predictor traits that are genetically correlated with survival, so as to increase the accuracy of EBVs for survival in Holstein and Jersey breeds in multi-trait models. This is important, particularly for the Jersey breed because most of the predictors of survival in Australia are developed using Holstein data.
Materials and methods
Data
The data used in the present study was provided by DataGene Pty Ltd (Melbourne, Australia). In Australia, the dominant dairy farming system is a pasture-based seasonal production system with a low level of supplementation (Haile-Mariam and Pryce 2019; Ooi et al. 2021). The data consisted of survival TDs for 538 394 pure Holstein and 63 839 pure Jersey cows. The survival TD is a measure of the number of lactations that cows remained in the herd adjusted for all known fixed effects, as described by Haile-Mariam et al. (2015). In addition to survival TDs, we had access to milk production records and type-trait scores (25 linear and 5 composite traits as described in DataGene (2021b)), BCS and fertility TDs of these cows and their close relatives that produced between 2002 and 2019. The pedigree-based breed composition was used to retain only phenotypes of purebred cows in the analyses. The pedigree file was also trimmed by removing the individuals that were not connected to the purebred cows present in our study.
Univariate models
The univariate sire models were run separately for Holstein and Jersey breeds in ASRemL (Gilmour et al. 2015) and were as follows:

where, y is the vector of survival TDs (the phenotypes were previously adjusted for fixed effects including herd–year– season, month of calving, parity and age at calving); b, u ~N(0, Aσ2u) and e ~N(0, Iσ2e) are the vectors of fixed effects (i.e. average), EBVs/2 (i.e. predicted transmitting ability) and random residual effects, in which A and I are the relationship and the identity matrices and σ2u and σ2e are sire and residual variances respectively; X and Z are design matrices relating survival TDs to the corresponding fixed and random effects respectively.
Bivariate models
We ran bivariate sire models in ASRemL to estimate the phenotypic and genetic correlations between survival TDs and the other traits. The model used was as follows:
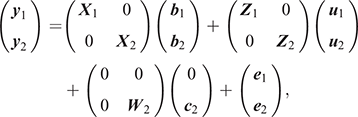
where, y1, b1, u1 and e1 are the vectors of observations, fixed effects, EBVs/2 and random residual effects for Trait 1 (i.e. survival) respectively and y2 is the vector of phenotypic records for Trait 2 (i.e. each of the 35 potential predictor traits were selected one at a time). For the fixed effects, b2 contains the effects of average as well as, for milk traits: herd–year–season, parity and age at calving; for type traits and BCS: herd–year–classifier, days in milk and age at classification date; for fertility TDs: the phenotypes were adjusted by DataGene for the fixed effects similar to survival TDs. For the random terms, u2 and e2 are the vectors EBV/2 and random residual effects for Trait 2 respectively; c2 ~N(0, Iσ2c2) is the vector of random cow permanent environment effects fitted just for the milk traits (repeatable traits), in which σ2c2 is the permeant environment variance. X, Z and W are design matrices relating phenotypes to the corresponding effects for Trait 1 or Trait 2, according to their subscript.
The sire and residual effects are assumed to be ) and
) respectively.
Including important predictor traits in multivariate models
Using the estimated genetic correlations between survival and other traits in the bivariate models and the main reasons for voluntary and involuntary culling (Weigel et al. 2003; Workie et al. 2021), we fitted four traits (survival TD, milk yield, composite overall type and fertility TD) in the multivariate sire model as follows:
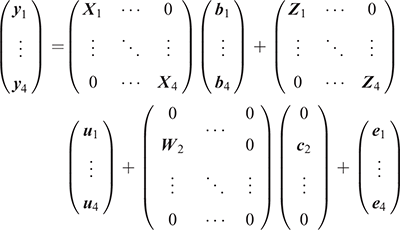
where, the model terms for Traits 1–4 are similar to the previously described models, and just for milk yield (i.e. Trait 2), the cow permanent environment effects are estimated in the model. The sire and residual effects for the four traits in the multivariate model are assumed to be and
respectively.
Accuracy of predictions for survival
The accuracy of genetic predictions for survival was calculated as
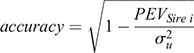
where, PEVSire i is the prediction error variance for Sire i and σ2u is the estimated sire genetic variance in the model. As the number of daughters per sire had a large variation, we also reported the accuracy of predictions in five groups (sires with <10, 10–49, 50–99, 100–499 and ≥500 daughters with survival TDs).
Results
Correlation between survival and other traits in bivariate models
The estimated phenotypic and genetic correlations between survival and other traits in bivariate models for Holsteins and Jerseys are shown in Tables 1 and 2 respectively. The number of cows with records/scores/TDs, h2 (and repeatability for milk traits) and summary statistics for different traits are shown in the tables as well.
The phenotypic correlations between survival TDs and different traits were generally low to moderate except for milk traits, overall type and fertility TDs. Survival in Holsteins was genetically highly correlated with milk (0.21 ± 0.02), fat (0.45 ± 0.02) and protein (0.50 ± 0.02) yields, composite overall type (0.25 ± 0.03), composite mammary system (0.27 ± 0.03), composite rump (0.14 ± 0.04) and fertility (i.e. calving interval) TDs (–0.44 ± 0.02).
In Jerseys, most of the traits were genetically correlated with survival TDs, such as milk (0.60 ± 0.04), fat (0.73 ± 0.04) and protein (0.72 ± 0.04) yields, composite overall type (0.50 ± 0.07), composite mammary system (0.50 ± 0.07) and fertility TDs (–0.41 ± 0.07).
Correlation among different traits in multivariate models
The phenotypic and genetic correlations among the four traits in multivariate models (i.e. survival TD, milk yield, overall type and fertility TD) for Holsteins and Jerseys are shown in Tables 3 and 4 respectively. According to the estimated h2, which are reported in the tables, the h2 for survival in multivariate compared with univariate models slightly increased in Jerseys (from 0.18 ± 0.02 to 0.20 ± 0.02), but did not change in Holstein (0.16 ± 0.01). Generally, the estimated h2 and correlations between the traits and survival in multivariate models were close to those from bivariate models. Nevertheless, exploring the genetic correlations among the traits in our multivariate models could be useful to understand the potential associations among the traits that were correlated with survival, and help remove redundant traits.
Accuracy of predictions in different models
In Fig. 1, the average, and distribution of the accuracy of predictions for survival in sires are shown using the univariate, bivariate (survival plus either milk yield, composite overall type or fertility TDs) and multivariate models. The accuracy of predictions improved in multivariate compared with univariate models in Holstein (from 0.54 to 0.59) and Jersey (from 0.48 to 0.53). However, there was no obvious benefit in using multiple predictor traits in multivariate models compared with any of the bivariate models.
The number of daughters with survival TDs affects the accuracy of predictions in sires. Therefore, in Fig. 1, the accuracy of predictions in Holsteins (on average, 22.8 daughters) was higher than in Jerseys (on average, 12.5 daughters). In Fig. 2, the distribution of the accuracy of predictions of survival are shown for Holstein and Jersey sires with varying numbers of daughters. As expected, the accuracy of prediction increased with the number of daughters in both breeds. However, the improvement in accuracy from multivariate compared with univariate models was more obvious when the number of daughters with survival TDs per sire was lower (i.e. <100).
Discussion
In dairy herds, the return on investment raising heifer replacements starts when lactation commences and is wasted if a calf never becomes productive or cannot survive as a lactating cow before reaching her full lactation potential (Chamberlain 2012). In fact, recovering of costs does not occur until at least 1.5 lactations have occurred and that just covers the costs of breeding and rearing replacement heifers (van der Heide et al. 2020).
The success in producing replacement heifers depends on prenatal survival (affected by early to late embryonic loss, abortion and stillbirth), as well as calf or juvenile survival (which is the loss between birth and first calving and mostly because of genetic defects, diseases, accidents or failure to conceive; Wathes et al. 2008). Calf survival is highly influenced by environmental factors and has been reported to have a low h2 (0.01–0.04; Hansen et al. 2003; Fuerst-Waltl and Sørensen 2010; Pritchard et al. 2013). Data to study calf mortality are scarce, especially in Australian dairy farms, as many female replacements are officially identified for the first time only when they commence milk recording. However, calf survival has been reported to be genetically correlated with cow survival (0.31 ± 0.14), potentially because cows lasting longer in dairy herds are likely to have daughters that survive and become replacement heifers (Pritchard et al. 2013). Compared with calf survival, cow survival has been reported to have a higher h2 (0.03–0.18) and the data to study cow survival can be easily obtained from milk-recording organisations (Forabosco et al. 2009; Pritchard et al. 2013; Sasaki 2013). Consequently, selecting for increased productive life, or functional longevity of cows, is a practical approach, which could also have some benefits in improving calf survival.
The estimated h2 for cow survival in our study (0.16–0.20) was comparatively high relative to previous estimates using linear models (Forabosco et al. 2009). The high estimate of h2 for cow survival could be because survival TDs were repeated records and they were not adjusted for traits such as milk and conformation traits, which are moderately to highly heritable and strongly associated with culling decisions. However, we combined survival records across lactations to calculate survival TDs without considering that culling reasons may vary throughout the cows’ productive life. To overcome this problem, instead of using survival TDs in the prediction model, raw survival records can be adjusted for the traits that largely influence survival (i.e. milk yield and functional traits) to produce residual survival, which is easier to be incorporated in the selection index as well (Byrne et al. 2016).
Survival can be considered as the same, or different traits, over lactations. Workie et al. (2021) showed that low production and poor fertility were the major reasons for culling in the first lactation, while mastitis was the main reason for culling of old cows in Australia. Further, these authors reported that the probability of culling cows due to infertility showed an increasing trend over the years, whereas culling for low production has reduced between 1995 and 2016. Accordingly, it is expected that early survival (survival to second lactation) is likely to be highly correlated with milk yield, some type traits and fertility, and late survival (survival to later lactations) may therefore be more correlated with mastitis resistance. Therefore, the correlations between survival and other traits could be different across lactations. This can be challenging to address properly in the process of adjusting survival for production and functional traits to calculate residual survival. A practical solution for this problem could be defining two survival traits, such as early and late survival.
To consider potential differences in survival across lactations and years, Haile-Mariam and Pryce (2015) assessed only the survival of cows from the first to second lactation and estimated the h2 and correlations between survival and milk and conformation traits across years by using linear random regression. As the total number of completed lactations cannot be measured until culling, early survival might be preferable instead of actual survival in breeding programs because it can be measured sooner. However, using information on early survival could be more associated with voluntarily culling, such as culling on milk production and type traits in some herds. Whereas, late survival could be associated with the ability of cows to avoid involuntary culling (Workie et al. 2021). In our study, we assumed that survival was the same trait over lactations and years because our aim was to find general predictor traits that can be used to improve the accuracy of predictions for the number of lactations that the cows could last in the herd.
In general, the genetic correlations between survival and functional traits in our study were in line with the expectations and the previous reports (Zavadilová et al. 2011; Sasaki 2013; Haile-Mariam and Pryce 2015). The strong favourable correlation between milk traits and survival could result from culling decisions, which were made according to the productivity of cows, which is likely to be especially important in the first parity. Moreover, farmers tend to be less strict in culling the high-producing cows that have poor conformation or fertility. The genetic correlation between survival and milk traits in Jerseys was stronger than in Holsteins. This could be associated with a higher probability removing Jersey cow from the herd due to low milk yield. This contrasts with higher involuntary culling rate in high-producing Holstein cows (Haile-Mariam et al. 2003; Sasaki 2013; Workie et al. 2021). The favourable genetic correlations between survival and mammary system traits could be due to the association between these traits and milk production as well as positive correlation with mastitis resistance (Zavadilová et al. 2011).
In addition to composite mammary system, the other composite conformation traits were favourably correlated with survival, except dairy strength (composed of stature, muzzle width, chest width, body depth, angularity, loin strength and bone quality). The unfavourable correlation of dairy strength with survival could be driven by the association of type traits such as angularity, body depth, bone quality with fertility (Haile-Mariam and Pryce 2015). This means that if fertility is included as a predictor of survival, dairy strength may not add further information, and so, may not help increase accuracy of prediction of survival.
In the traits related to feet and legs composite, rear legs – rear view had the strongest correlation with survival in both Holstein and Jersey, which could be due its linear association with locomotion problems as cows with hocked-in leg are susceptible to become lame (Boettcher et al. 1998; Zavadilová et al. 2011). The correlation between rump traits and survival could be related to the association between these traits and calving difficulty and, consequently, longer calving interval (i.e. poor fertility). In line with the expected association between rump traits and involuntary culling, Haile-Mariam and Pryce (2015) reported that the genetic correlation between pin set and survival remained similar (0.28–0.35 across different years) after adjusting the survival phenotypes for milk yield. In Jerseys, the moderately high correlation between body size (stature and body length) and survival could be because breeders are interested in increasing the body size of Jersey cows. Note, the correlations of survival with body size associated traits in Holsteins were close to zero.
The genetic correlations among the traits in our multi-trait models showed that milk yield and overall type in Jersey and fertility in Holstein had the strongest correlation with survival. In Fig. 1, the average accuracy for survival was the highest in Jerseys when milk yield was present in the prediction model. Further, the genetic correlation between milk yield and overall type was strong in Jerseys (0.61 ± 0.06), which could be the reason for the positive correlation we observed between most of the conformation traits and survival in Jersey. The antagonistic genetic correlations between survival and fertility (measured as calving interval) as well as milk yield and fertility were stronger in Holstein than in Jersey. Consequently, the high-producing Holstein cows may be more prone to impaired fertility, which could increase the likelihood of involuntary culling in Holstein. The previous studies also found unfavourable genetic correlation between milk yield and calving interval (0.18–0.58) in Australian Holstein (Haile-Mariam et al. 2003; Haile-Mariam and Pryce 2015). Moreover, fertility performance is directly associated with cow survival, as poor fertility is one of the major causes of culling, particularly in seasonal calving systems. Hence, selection for cows that survive longer in a herd could result in some improvements in herd fertility as well.
The accuracy of predictions for survival by adding predictor traits improved in our study. However, there was no extra benefit in including multiple predictor traits in the model. Probably because the predictor traits in the multi-variate models were highly correlated, or because we disregarded variation in culling reasons across lactations (Workie et al. 2021). Due to the large variation in annual genetic gain for different traits, the correlation between survival and other traits tends to change over years, which could also explain the limited improvement in accuracy in multi-trait compared with univariate models (Haile-Mariam and Pryce 2015). However, we found that for the sires with a limited number of daughters (i.e. young bulls), the predictor traits were quite useful in improving the accuracy of predictions for survival. Conceivably, random regression models could be useful in genetic evaluations for survival because they allow variances and covariances to vary over time (Forabosco et al. 2009). Moreover, the survival data for cows that are still in the herd (i.e. censored data) could be suitably handled in random regression models (Haile-Mariam and Pryce 2015). Hence, using predictor traits in random regression models could be investigated in future studies for the potential increase in accuracy and reduction of bias of predictions for cow survival.
Currently, genetic evaluation for survival in Australian dairy cattle is based on direct culling data (survival from current lactation to the next lactation) and use of predictor traits such as overall type, pin set, udder depth and likeability (workability) trait for both Holstein and Jersey breeds (DataGene 2021a). The results presented here suggest that some of the predictor traits are more valuable for Jersey than for Holstein. Furthermore, genetic prediction for survival is currently a two-step process in Australia, where EBVs based on indirect predictor traits are added to survival calculated from direct culling data (DataGene 2021a). The potential to use multi-trait models to calculate EBVs for survival by adding predictor traits with direct culling data needs to be examined to find out whether the accuracy of predicting survival, particularly for bulls with no or small number of daughters, can be improved.
Conclusions
Survival is a complex trait that is affected by the factors associated with voluntary and involuntary culling. Therefore, finding traits genetically correlated with survival could improve our understanding of genetic components of culling reasons. We found that fertility performance, milk yield and most of the conformation traits were favourably correlated with survival. This could indicate that (1) survival could be potentially improved by indirect selection for conformation traits and (2) selection for increased herd life could simultaneously improve milk production and fertility. Using milk yield, overall type and fertility as predictor traits in multivariate evaluation models, we could marginally improve the accuracy of predictions for survival in both Holstein and Jersey sires. As expected, the improvements were higher for sires with fewer daughters, with little effect for sires with large number of daughters. We found that some of the predictor traits could be more valuable for Jerseys than Holstein. Therefore, we recommend that traits such as body size (stature and body length) to be considered for genetic evaluation models of survival in the Jersey breed. We expect that the accuracy of predictions for survival could be further improved by using a random regression multi-trait model, which could take the variation in correlation between traits over years into account.
Conflicts of interest
The authors declare no conflicts of interest.
Acknowledgements
The authors acknowledge DataGene for providing the data for our research. We also appreciate financial support from DairyBio, a joint venture of Dairy Australia, The Gardiner Foundation, and Agriculture Victoria (Melbourne, Australia).
References
Barkema HW, von Keyserlingk MAG, Kastelic JP, Lam TJGM, Luby C, Roy JP, LeBlanc SJ, Keefe GP, Kelton DF (2015) Invited review: changes in the dairy industry affecting dairy cattle health and welfare. Journal of Dairy Science 98, 7426–7445.| Invited review: changes in the dairy industry affecting dairy cattle health and welfare.Crossref | GoogleScholarGoogle Scholar | 26342982PubMed |
Boettcher PJ, Dekkers JCM, Warnick LD, Wells SJ (1998) Genetic analysis of clinical lameness in dairy cattle. Journal of Dairy Science 81, 1148–1156.
| Genetic analysis of clinical lameness in dairy cattle.Crossref | GoogleScholarGoogle Scholar | 9594404PubMed |
Byrne TJ, Santos BFS, Am PR, Martin-Collado D, Pryce JE, Axford M (2016) New breeding objectives and selection indices for the Australian dairy industry. Journal of Dairy Science 99, 8146–8167.
| New breeding objectives and selection indices for the Australian dairy industry.Crossref | GoogleScholarGoogle Scholar | 27522425PubMed |
Chamberlain T (2012) Understanding the economics of dairy farming Part 1: income, costs and profit. Livestock (London) 17, 30–33.
| Understanding the economics of dairy farming Part 1: income, costs and profit.Crossref | GoogleScholarGoogle Scholar |
DataGene (2021a) ‘Survival and Australian breeding indices: Technote 4.’ Available at https://datagene.com.au/sites/default/files/Upload%20Files/Technote%204%20Survival%20and%20Australian%20Breeding%20Indices.pdf [Verified 15 February 2021]
DataGene (2021b) ‘Type ABVs explained: Genetics Backgrounder # 2.’ Available at https://datagene.com.au/sites/default/files/Upload%20Files/02%20Type%20ABVs%20explained%20Mar%202020_0.pdf [Verified 15 March 2021]
Forabosco F, Jakobsen JH, Fikse WF (2009) International genetic evaluation for direct longevity in dairy bulls. Journal of Dairy Science 92, 2338–2347.
| International genetic evaluation for direct longevity in dairy bulls.Crossref | GoogleScholarGoogle Scholar | 19389992PubMed |
Fuerst-Waltl B, Sørensen MK (2010) Genetic analysis of calf and heifer losses in Danish Holstein. Journal of Dairy Science 93, 5436–5442.
| Genetic analysis of calf and heifer losses in Danish Holstein.Crossref | GoogleScholarGoogle Scholar | 20965359PubMed |
Garnsworthy PC (2004) The environmental impact of fertility in dairy cows: a modelling approach to predict methane and ammonia emissions. Animal Feed Science and Technology 112, 211–223.
| The environmental impact of fertility in dairy cows: a modelling approach to predict methane and ammonia emissions.Crossref | GoogleScholarGoogle Scholar |
Gilmour AR, Gogel BJ, Cullis BR, Welham S, Thompson R (2015) ‘ASReml user guide release 4.1 structural specification.’ (VSN International Ltd: Hemel Hempstead, UK) Available at www.vsni.co.uk
Giordano JO, Fricke PM, Wiltbank MC, Cabrera VE (2011) An economic decision-making support system for selection of reproductive management programs on dairy farms. Journal of Dairy Science 94, 6216–6232.
| An economic decision-making support system for selection of reproductive management programs on dairy farms.Crossref | GoogleScholarGoogle Scholar | 22118110PubMed |
Haile-Mariam M, Pryce JE (2015) Variances and correlations of milk production, fertility, longevity, and type traits over time in Australian Holstein cattle. Journal of Dairy Science 98, 7364–7379.
| Variances and correlations of milk production, fertility, longevity, and type traits over time in Australian Holstein cattle.Crossref | GoogleScholarGoogle Scholar | 26254520PubMed |
Haile-Mariam M, Pryce JE (2019) Genetic evaluation of gestation length and its use in managing calving patterns. Journal of Dairy Science 102, 476–487.
| Genetic evaluation of gestation length and its use in managing calving patterns.Crossref | GoogleScholarGoogle Scholar | 30343913PubMed |
Haile-Mariam M, Bowman PJ, Goddard ME (2003) Genetic and environmental relationship among calving interval, survival, persistency of milk yield and somatic cell count in dairy cattle. Livestock Production Science 80, 189–200.
| Genetic and environmental relationship among calving interval, survival, persistency of milk yield and somatic cell count in dairy cattle.Crossref | GoogleScholarGoogle Scholar |
Haile-Mariam M, Pryce J, Schrooten C, Hayes B (2015) Including overseas performance information in genomic evaluations of Australian dairy cattle. Journal of Dairy Science 98, 3443–3459.
| Including overseas performance information in genomic evaluations of Australian dairy cattle.Crossref | GoogleScholarGoogle Scholar | 25771052PubMed |
Hansen M, Madsen P, Jensen J, Pedersen J, Christensen LG (2003) Genetic parameters of postnatal mortality in Danish Holstein calves. Journal of Dairy Science 86, 1807–1817.
| Genetic parameters of postnatal mortality in Danish Holstein calves.Crossref | GoogleScholarGoogle Scholar | 12778591PubMed |
Ooi E, Stevenson MA, Beggs DS, Mansell PD, Pryce JE, Murray A, Pyman MF (2021) Herd manager attitudes and intentions regarding the selection of high-fertility EBV sires in Australia. Journal of Dairy Science 104, 4375–4389.
| Herd manager attitudes and intentions regarding the selection of high-fertility EBV sires in Australia.Crossref | GoogleScholarGoogle Scholar | 33485678PubMed |
Pritchard T, Coffey M, Mrode R, Wall E (2013) Understanding the genetics of survival in dairy cows. Journal of Dairy Science 96, 3296–3309.
| Understanding the genetics of survival in dairy cows.Crossref | GoogleScholarGoogle Scholar | 23477814PubMed |
Pryce JE, Bell MJ (2017) The impact of genetic selection on greenhouse-gas emissions in Australian dairy cattle. Animal Production Science 57, 1451–1456.
| The impact of genetic selection on greenhouse-gas emissions in Australian dairy cattle.Crossref | GoogleScholarGoogle Scholar |
Sasaki O (2013) Estimation of genetic parameters for longevity traits in dairy cattle: a review with focus on the characteristics of analytical models. Animal Science Journal 84, 449–460.
| Estimation of genetic parameters for longevity traits in dairy cattle: a review with focus on the characteristics of analytical models.Crossref | GoogleScholarGoogle Scholar | 23607602PubMed |
van der Heide EMM, Veerkamp RF, van Pelt ML, Kamphuis C, Ducro BJ (2020) Predicting survival in dairy cattle by combining genomic breeding values and phenotypic information. Journal of Dairy Science 103, 556–571.
| Predicting survival in dairy cattle by combining genomic breeding values and phenotypic information.Crossref | GoogleScholarGoogle Scholar | 31704017PubMed |
Wathes DC, Brickell JS, Bourne NE, Swali A, Cheng Z (2008) Factors influencing heifer survival and fertility on commercial dairy farms. animal 2, 1135–1143.
| Factors influencing heifer survival and fertility on commercial dairy farms.Crossref | GoogleScholarGoogle Scholar | 22443725PubMed |
Weigel KA, Palmer RW, Caraviello DZ (2003) Investigation of factors affecting voluntary and involuntary culling in expanding dairy herds in Wisconsin using survival Analysis. Journal of Dairy Science 86, 1482–1486.
| Investigation of factors affecting voluntary and involuntary culling in expanding dairy herds in Wisconsin using survival Analysis.Crossref | GoogleScholarGoogle Scholar | 12741574PubMed |
Workie ZW, Gibson JP, van der Werf JHJ (2021) Analysis of culling reasons and age at culling in Australian dairy cattle. Animal Production Science 61, 680–689.
| Analysis of culling reasons and age at culling in Australian dairy cattle.Crossref | GoogleScholarGoogle Scholar |
Zavadilová L, Němcová E, Štípková M (2011) Effect of type traits on functional longevity of Czech Holstein cows estimated from a Cox proportional hazards model. Journal of Dairy Science 94, 4090–4099.
| Effect of type traits on functional longevity of Czech Holstein cows estimated from a Cox proportional hazards model.Crossref | GoogleScholarGoogle Scholar | 21787944PubMed |