A comparison of smoke modelling tools used to mitigate air quality impacts from prescribed burning
Megan M. Johnson

A Department of Civil, Construction, and Environmental Engineering, North Carolina State University, Raleigh, NC 27606, USA.
International Journal of Wildland Fire 32(7) 1162-1173 https://doi.org/10.1071/WF22172
Submitted: 24 July 2022 Accepted: 28 April 2023 Published: 18 May 2023
© 2023 The Author(s) (or their employer(s)). Published by CSIRO Publishing on behalf of IAWF. This is an open access article distributed under the Creative Commons Attribution-NonCommercial-NoDerivatives 4.0 International License (CC BY-NC-ND)
Abstract
Background: Prescribed fire is a land management tool used extensively across the United States. Owing to health and safety risks, smoke emitted by burns requires appropriate management. Smoke modelling tools are often used to mitigate air pollution impacts. However, direct comparisons of tools’ predictions are lacking.
Aims: We compared three tools commonly used to plan prescribed burning projects: the Simple Smoke Screening Tool, VSmoke and HYSPLIT.
Methods: We used each tool to model smoke dispersion from prescribed burns conducted by the North Carolina Division of Parks and Recreation over a year. We assessed similarity among the tools’ predicted smoke fields, areas of concern and potential population impacts.
Key results: The total smoke area predicted by the tools differs by thousands of square kilometres and, as such, spatial agreement was low. When translated into numbers of residents potentially exposed to smoke, tool estimates can vary by an order of magnitude.
Conclusions: Our analysis of an operational burning program suggests that the differences among the tools are significant and inconsistent.
Implications: While our analysis shows that improved and more consistent smoke modelling tools could better support land management, clear guidelines on how to apply their predictions are also necessary to obtain these benefits.
Keywords: air pollution, dispersion modelling, HYSPLIT, particulate matter, prescribed burn, prescribed fire, Simple Smoke Screening Tool, smoke, VSmoke.
Introduction
Prescribed fire is a land management tool extensively used on public and private wildland across the US. Prescribed burns can serve several purposes, including wildfire risk reduction, wildlife habitat maintenance and support of fire-dependent species (Haines et al. 2001; Mitchell et al. 2014; James et al. 2020). In the US, prescribed fire use has steadily increased over the last decade and recently exceeded 4 million hectares treated annually (Melvin 2020). It is applied most frequently in the southeastern region of the country, where fire is an essential component of ecosystems (Frost 1998; Fowler and Konopik 2007; Lafon 2010). However, smoke from wildland fire (wildfire and prescribed fire) is one of the largest sources of fine particulate matter (PM2.5) emissions in the US (US EPA 2021) and is associated with negative health outcomes, including respiratory morbidities and premature mortality (Reid et al. 2016; Cascio 2018). Owing to health and safety risks (Wilkins et al. 2018; Huang et al. 2019; Afrin and Garcia‐Menendez 2020), smoke emitted by burns requires appropriate management (NWCG 2020). Smoke impacts and management are perceived by many southern land managers as a barrier to using and increasing prescribed fire (Melvin 2012; Kobizar et al. 2015).
Smoke management requirements in the US differ by location but often rely on similar methods (NWCG 2020). For example, the under the 1999 North Carolina Prescribed Burning Act (North Carolina General Statutes 1999), certified burners are not liable for civil action resulting from smoke from their burn if they abide by the state’s Smoke Management Program. This program allows two methods to determine acceptable smoke emissions: the Ventilation Index System (VIS) and atmospheric dispersion modelling (NCFS 2020). The VIS is a simple method that uses the product of the mixing height and transport wind speed to calculate a ventilation rate. The rates correspond to six categories that indicate whether conditions are appropriate for burning. Alternatively, managers can use atmospheric dispersion modelling to show that smoke impacts from a burn project will be appropriate and not affect smoke-sensitive targets. Smoke dispersion models are often used by land managers to meet the burning requirements of different locations and mitigate air pollution impacts.
Multiple smoke modelling tools have been developed over time for specific applications or conditions. The Simple Smoke Screening Tool is based on a graphical smoke screening system for prescribed burning in southern US fuels (Mobley et al. 1976). Planned Burn (PB)-Piedmont was designed to model night-time ground-level smoke transport through valley terrain typical of the Piedmont region of the southeastern US (Achtemeier 2005). Daysmoke is an empirical-statistical smoke dispersion model adapted from a model developed to simulate ash deposition from sugar cane fires (Achtemeier 1998; Achtemeier et al. 2011). Tools like NFSpuff (Harrison 1995) and Tiered Smoke/Air Resource System (TSARS) Plus (Hardy et al. 2001) were developed primarily for the western US or complex terrain. VSmoke is a steady-state Gaussian plume model used to estimate peak downwind smoke concentrations and visibility impacts (Lavdas 1996). Other tools, such as the Hybrid Single-Particle Lagrangian Integrated Trajectory (HYSPLIT) (Stein et al. 2015) and CALPUFF models (Scire et al. 2000) were not specifically developed to simulate smoke dispersion but have been adapted to enable this. The BlueSky framework, which is available as a web application (BlueSky Playground), brings together independent models of fuels, consumption and emissions, along with meteorological forecasts, to use with VSmoke or HYSPLIT as dispersion models (Larkin et al. 2009).
Beyond dispersion models, Eulerian grid models, such as the Community Multiscale Air Quality Modelling System (CMAQ) (Byun and Schere 2006) or Weather Research and Forecasting model coupled with Chemistry (WRF-Chem) (Grell et al. 2005, 2011), have been used to simulate the transport and chemical evolution of prescribed fire smoke. The modelling systems include more complex representations of chemical and physical atmospheric processes, but often require specialised training and significant computing resources. Thus, grid models are not typically used by land managers when conducting individual prescribed projects, but are more commonly used for comprehensive air quality simulations in atmospheric research, air quality forecasting, or regional-scale planning. For example, the HiRes2 forecasting system relies on the CMAQ model to forecast the impacts of various emissions sources over the state of Georgia, including all prescribed fires (Odman et al. 2018). Further coupling gridded atmospheric models to fire-activity models is an active area of research (Mandel et al. 2011, 2014).
Smoke modelling tools vary in complexity and ease of use. A comprehensive review of methods used to model smoke, covering the wide range of complexity – box models, plume models, grid models, full-physics smoke models and coupled modelling frameworks – was compiled by Goodrick et al. (2013). However, direct comparisons of available tools as used by land managers are lacking. A qualitative comparison of simplified smoke tools by the US Forest Service focused on appropriate applications and end users (Breyfogle and Ferguson 1996), but the assessment, conducted over 25 years ago, includes tools inadequate outside the western US and several no longer in use. Further, it did not compare model outputs for specified burns. More recently, larger-scale efforts such as the Fire and Smoke Model Evaluation Experiment (FASMEE) (Prichard et al. 2019) have compared model predictions with observations of pollutant concentrations. In FASMEE, Liu et al. (2019) analysed several smoke forecasting systems and two computationally fast local smoke dispersion tools, Daysmoke and (PB)-Piedmont. However, the models considered in the study were not used to simulate consistent fire scenarios and model outputs were not systematically compared.
In this study, we compared three smoke modelling tools commonly used at present to plan prescribed burning projects. Based on fire records from the North Carolina Division of Parks and Recreation (NC State Parks), we used each tool to model smoke dispersion from all prescribed burns conducted by NC State Parks’ land management program over a complete year. We then compared predicted smoke dispersion among the tools and with smoke fields from a state-of-the-science air quality modelling system. We assessed the similarity of the tools’ outputs and how their results translate into potential population impacts. The tools considered represent a range of scientific complexity, yet all remain freely accessible to fire practitioners, who frequently use them to inform burning decisions in land management operations. Further, by using historical data from an active burning program, the analysis provides additional insights into potential smoke impacts in areas that experience frequent burning activity.
Methods
Smoke modelling tools
We analysed three smoke modelling tools that are widely used and readily accessible to land managers in the US. The tools considered can be accessed from multiple host institutions and are among those compiled by the Southern Fire Exchange fire science communication program (Southern Fire Exchange 2021).
The Simple Smoke Screening Tool (SSST) is a web-based screening tool that uses a graphical method to delineate smoke impact zones (Mobley et al. 1976; Wade and Lunsford 1989; Wade and Mobley 2007). This tool is intended to identify smoke-sensitive areas, rather than estimate concentrations. It estimates smoke impact distance based on fuels, ignition and the Lavdas Dispersion Index (Lavdas 1986). User inputs include the burn location, size, fuel type, ignition method and wind direction. The tool output produces a graphical display of two zones: a smaller ‘critical smoke-sensitive area’ defining the most probable smoke impact area, and a larger area defining ‘less severe’ smoke impacts. The tool was developed by the Southern High Resolution Modelling Consortium and is currently hosted online by the Florida Forest Service.
VSmoke is a steady-state Gaussian plume dispersion model that can be used to analyse the impacts from single prescribed fires (Lavdas 1996). VSmoke-Web is a web-based version of the program (FCAMMS 2021). VSmoke estimates PM2.5 concentrations along a plume trajectory based on the approach of Turner (1970), adapted to predict near-ground smoke from prescribed fires. Users provide information about fire location, size, duration, fuel type and load, and ignition method, as well as atmospheric mixing height, transport wind and stability class. The tool provides suggested default values for additional inputs (e.g. fuel load, consumption, PM2.5 emission factor and rate and plume rise fraction), which users can change if better estimates are available. The web-based tool outputs peak hourly PM2.5 concentration contours, formatted to reflect hourly US Environmental Protection Authority (EPA) Air Quality Index (AQI) values.
HYSPLIT is an air parcel trajectory and dispersion model developed by the National Oceanic and Atmospheric Administration (NOAA) used for many applications, including dispersion of PM2.5 from prescribed burns (Stein et al. 2015). It is used in NOAA’s operational Smoke Forecasting System (Rolph et al. 2009). HYSPLIT relies on a hybrid modelling method in which a moving reference frame (i.e. a Lagrangian approach) is used to calculate air parcel trajectories and a fixed reference frame (i.e. a Eulerian approach) is used to calculate pollutant concentrations. The model can be run as a web-based tool using the NOAA Air Resources Laboratory’s Real-time Environmental Applications and Display sYstem (READY) (Rolph et al. 2017), with some constraints, or be used as a standalone program. The basic inputs required by the READY tool are fire location, size and start time. Based on this information, the tool uses the emissions processing components of the BlueSky Framework (O’Neill et al. 2008), including the Fuel Characteristic Classification System (FCCS) module, CONSUME fuel consumption model and compiled fire emission factors to estimate PM2.5 emissions, heat release and plume rise. Time-varying, three-dimensional gridded meteorology fields are used to drive the simulations. HYSPLIT-compatible forecast and archived meteorological data from multiple models and operational forecasts can be accessed within the online tool or downloaded to run the standalone software. Model outputs are given as sub-hourly to daily average fire-related PM2.5 concentration contours, corresponding to hourly AQI values.
Although these tools differ in their complexity, they are all recommended for smoke management planning by fire science and practitioner entities in the southeastern US (e.g. the Southern Fire Exchange wildland fire science communication program (Southern Fire Exchange 2021)). States or other authorities may determine acceptable tools for burn authorisation purposes and specify conditions for their use (e.g. scale, topography, proximity to urban areas). The approved tools differ between authorities and some may not require dispersion modelling at all. For example, VSmoke and HYSPLIT are both considered acceptable tools in the prescribed fire permitting process by the state of North Carolina, although VSmoke may only be used for ‘low complexity burns’ (NCFS 2020). However, the US Forest Service Region 8 has suggested using VSmoke during early burn planning to investigate potential impacts under various weather conditions and using HYSPLIT the day before a burn to aid in making go/no-go decisions (USFS 2017). We compared the smoke modelling tools’ predictions with a simulation conducted with a state-of-the-science chemical transport model, CMAQ (Appel et al. 2017). The model configuration is detailed in the Supplementary information. Table 1 outlines required inputs and outputs of each of the tools considered. Further details about the tools’ set-up are included in Supplementary Table S1. All tools are available online as web-based applications: SSST is hosted by the Florida Forest Service, VSmoke-Web is hosted by the Georgia Forestry Commission and HYSPLIT is hosted on the NOAA’s READY system. VSmoke and HYSPLIT are also available as downloadable software and accessible through the US Forest Service’s updated BlueSky Playground web-based system. Because ease of use was an important study consideration, we focused on comparing web-based versions of the tools. However, we used the PC version of HYSPLIT in our main tool comparison as it allows users to define emissions data and therefore enables simulations that are more consistent with those produced with the other smoke tools considered.
Simple Smoke Screening Tool | VSmoke-Web | HYSPLIT | |
---|---|---|---|
Inputs | |||
Meteorology | Wind at point | Wind, mixing height and stability at point | Time-varying 3D gridded fields |
Fire characteristics | Coordinates | Coordinates | Coordinates |
Area burned | Aea burned | Area burned | |
Fuel type | Fuel type | Duration | |
Ignition method | Ignition method | ||
Duration of burn | |||
Fuel moisture | |||
Outputs | Two areas of concern for smoke impacts | Maximum hourly PM2.5 concentration contours (μg/m3) | Hourly average PM2.5 concentration contours (μg/m3) |
Meteorology
We ran the smoke modelling tools with 3-km resolution High-Resolution Rapid Refresh (HRRR v1) meteorological data, archived and available through NOAA Air Resources Laboratory’s READY platform. HRRR is a real-time weather model developed by NOAA that is cloud-resolving and convection allowing, and assimilates radar data every 15 min (NOAA Earth Systems Research Laboratory 2015). These are the highest-resolution data available through the READY platform. Among the tools considered, only HYSPLIT uses three-dimensional gridded meteorology. Point meteorology information for SSST and VSmoke-Web (e.g. transport wind and mixing height) was extracted from the three-dimensional HRRR meteorology fields at the grid cell nearest to each burn location. Additionally, weather inputs used for SSST and VSmoke-Web, which do not rely on time-varying meteorology, corresponded to burn start times. The CMAQ simulation considered was driven by 4-km resolution meteorological fields centred on the state of North Carolina generated with the WRF model (Skamarock et al. 2008).
Prescribed burn information
To compare smoke tool predictions, we used NC State Parks prescribed burning records for 2016. Records include burn coordinates, date of the burn and the total area burned. In 2016, 60 burns were conducted on NC State Parks land. However, owing to gaps in archived HRRR meteorology, we were able to model between 44 and 56 of these burns depending on the tool considered. Prescribed burns were conducted on diverse landscapes extending from the North Carolina Coastal Plain to the Appalachian Mountains, with burn areas ranging from less than 8 hectares to over 80. The locations and sizes of the prescribed burns are shown in Fig. 1.
Locations and burn areas of prescribed burns conducted on North Carolina State Parks’ land in 2016. Population at the block group level and locations of EPA air quality monitors are also shown on the map. Area burned can be converted to hectares by dividing acres by 2.47.
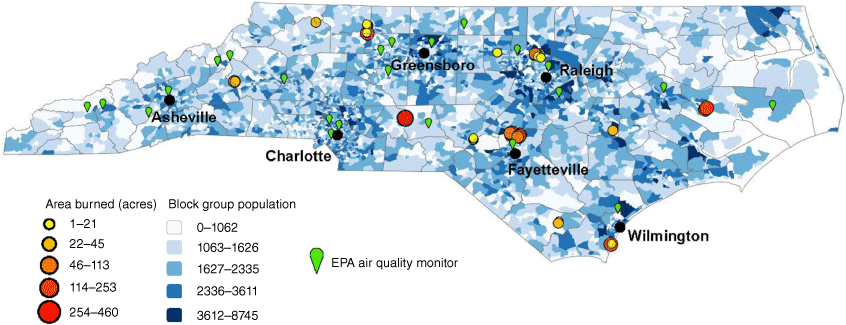
To address differences in inputs among the tools and the data available from burning records, several assumptions were considered to model smoke impacts and increase consistency among the simulations. HYSPLIT sets fuels burned based on the location of the fire, using the BlueSky framework; however, users of VSmoke-Web and SSST must provide fuel information (e.g. type, load and moisture level). We used the Landfire Data Distribution Site Viewer with the Anderson 13 Fire Behaviour Fuel Models (Anderson 1982) to determine the fuel type and loading at each burn location. Burn duration is not included in NC State Parks’ burn records, but is required by VSmoke-Web. HYSPLIT requires a simulation duration so, for both tools, we assumed a duration of 8 h. Similarly, start time is not captured in NC State Parks fire records. We assumed all burns began at 10:00 am Eastern Time, following Huang et al. (2019). Further details about the assumed smoke tool settings and inputs are included in Supplementary Table S1.
Emissions profiles
The web versions of VSmoke and HYSPLIT can determine PM2.5 emissions factors and time-varying emissions profiles based on the fuel characteristics defined. We used default emissions factors determined by VSmoke-Web. The web version of HYSPLIT uses emissions profiles generated with the BlueSky framework’s emissions models; however, the emissions profiles differ significantly from those predicted by the latest release of the BlueSky Playground (version 3; https://info.airfire.org/playground). Notably, emissions predicted by the web version of HYSPLIT peak shortly after burns begin whereas those predicted by BlueSky Playground steadily increase for several hours after ignition (Supplementary Fig. S1). For comparison with SSST and VSmoke-Web, we chose to generate temporal emissions profiles using BlueSky Playground v3 and use these as inputs to the PC version of HYSPLIT. To evaluate the impacts of these differences in emission profiles on predicted smoke dispersion, we further compared the outputs of HYSPLIT driven by BlueSky Playground emissions profiles (HYSPLIT-PC-BlueSky) with those of the HYSPLIT web-based system (HYSPLIT-Web).
Comparison criteria and metrics
The outputs of the smoke modelling tools considered vary significantly. To examine these, we defined comparable ‘areas of concern’ based on the tool output descriptions and format. SSST outputs two downwind areas. The smallest, closest to the ignition point, identifies ‘critical smoke-sensitive areas’, or the most probable smoke impact area. A larger area represents ‘an area of less severe smoke impact’. We designated SSST’s larger smoke impacts area as the area of concern to compare with other smoke tool predictions. Both VSmoke-Web and HYSPLIT provide outputs in the form of PM2.5 concentration contours that are colour-coded to correspond with the AQI scale. HYSPLIT can provide predictions at sub-hourly intervals for the duration of the burn, whereas VSmoke-Web outputs a single set of contours representing the ‘peak hourly concentrations of PM2.5’ for each burn. We designated the areas predicted as AQI moderate level of concern, corresponding to hourly PM2.5 concentrations ≥39 µg/m3, as areas to compare across smoke tools. Although there is not an hourly AQI scale, this concentration is based on Wildfire Smoke, A Guide for Public Health Officials, which divides the 24-h AQI concentration breakpoints by 0.4 for a 1-h ratio (Lipsett et al. 2008). For HYSPLIT output, we combined hourly predictions of moderate AQI areas into a single field, representing the entire area predicted to experience this level of smoke for the duration of each burn. To compare the CMAQ modelling results with smoke tool outputs, we considered the CMAQ grid cells with hourly PM2.5 concentrations ≥39 µg/m3.
To compare smoke dispersion predictions across tools, we calculated the overlap fraction between the tools’ areas of concern for each burn. The overlap fraction is based on the tools’ average area of concern and was used to examine the degree of similarity across the predictions. Eqn 1 describes the overlap fraction calculation for two tools:
To estimate population within areas of concern due to prescribed fire smoke, we used 2010 US Census data at the block-group level. Where areas of concern overlapped a census block group, we calculated the fraction of the block group area covered by the predicted area of concern and considered the same fraction of the block group’s population as being at moderate risk of smoke exposure from the burn. Fig. 2 illustrates these calculations. The comparison metrics were first assessed for outputs from SSST, VSmoke-Web and HYSPLIT-PC-BlueSky. Then, the metrics were estimated for HYSPLIT-PC-BlueSky outputs and HYSPLIT-Web to examine the impact of differing emissions profiles.
Smoke tool overlap metrics were calculated using the intersecting area of concern (purple) of predictions from at least two tools, here shown in red and blue. Population impacts were estimated by calculating the fraction of block group area covered by areas of concern and considering the same fraction of the block group population as potentially exposed to moderate smoke levels.
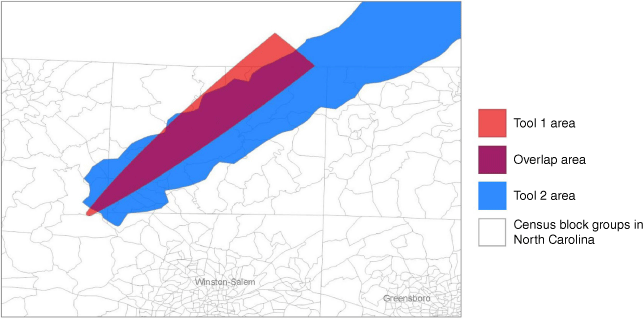
Results
Smoke tool predictions
In 2016, the North Carolina Division of Parks burned more than 1940 hectares on state park land. Here, 46–56 prescribed burns were simulated with each of the smoke tools considered. These occurred across the state and throughout the year, but the largest total area was burned in Carver’s Creek State Park, near Fayetteville, NC, and most burns were conducted in the month of March. Across all parks, the mean and median burn areas were 34 and 20 hectares, respectively, and approximately 25% of burns were over 40 hectares. The largest burn was conducted at Morrow Mountain State Park (80 km east of Charlotte) and was 186 hectares.
To compare the outputs from the tools, we examined the total areal coverage of the areas of concern modelled by each and estimated the population that would fall within these. Table 2 summarises tool outputs for prescribed burns in which an area of concern due to smoke was predicted. VSmoke-Web and SSST met the comparison criteria (i.e. an area of concern as defined in the Methods was predicted) for all 56 burns modelled. HYSPLIT-Web met the comparison criteria for 43 of the 44 burns for which complete HRRR archived meteorological fields were available, whereas HYSPLIT-PC-BlueSky met the criteria for 18 of these burns. The largest area of concern summed across all burns, approximately 20 000 km2 (n = 43), was predicted by HYSPLIT-Web using the default emissions profiles. This is an order of magnitude larger than the total area of concern modelled by other tools – approximately 6 times larger than that predicted by VSmoke-Web (3250 km2, n = 56) and 11 times larger than that predicted by the SSST (1790 km2, n = 56). When modelled with BlueSky Playground emissions profiles, the total area of concern predicted by HYSPLIT is reduced significantly (1125 km2, n = 18), as only 33% of the burns met the comparison criteria. Although the total area of concern predicted by VSmoke-Web exceeds those predicted by SSST and HYSPLIT-PC-BlueSky, this is largely due to a relatively small number of burns for which VSmoke-Web predicts particularly large areas of concern. The average area of concern per area burned is smallest when using VSmoke-Web, but this tool has the largest variability. For most burns, VSmoke-Web predicts the smallest area of concern due to moderate smoke.
Simple Smoke Screening Tool | VSmoke-Web | HYSPLIT-PC-BlueSky | HYSPLIT-Web | |
---|---|---|---|---|
No. of burns meeting criteria | 56 | 56 | 18 | 43 |
Total area of concern (km2) | 1793 | 3254 | 1125 | 20 426 |
Average area of concern per burn (km2) | 32 | 58 | 62 | 475 |
Median area of concern per burn (km2) | 21 | 3 | 15 | 196 |
Total population potentially impacted | 150 079 | 186 858 | 132 228 | 1 143 539 |
Average population potentially impacted per burn | 2680 | 3337 | 7346 | 26 594 |
Median population potentially impacted per burn | 1201 | 187 | 454 | 6934 |
Estimates are based on prescribed burns for which an area of concern was predicted.
Differences in estimates of population potentially exposed to moderate smoke pollution reflect the tools’ area of concern predictions. Across all burns, HYSPLIT-Web predicts over 1 million individuals within areas of concern due to smoke from the prescribed fires considered. The estimates derived from VSmoke-Web, SSST and HYSPLIT-PC-BlueSky range from 132 000 to 187 000 individuals within predicted areas of concern. For all tools, the average number of people in areas of concern due to smoke is greater than 2500 per burn. However, impact estimates vary substantially across prescribed fires, as some burns affect areas with greater population density. As in the comparison of area of concern predictions, differences in population affected by smoke between the tools are not consistent across the prescribed burns considered.
A small number of burns were associated with significantly larger areas of concern, most notably in the VSmoke-Web predictions. Among the five largest predicted areas of concern for each tool (Supplementary Table S2), most were for prescribed burns that occurred in February or March, burned over 81 hectares and were often conducted at Carvers Creek State Park near Fayetteville, NC. The burns resulting in the largest predicted areas of concern were not necessarily the same as those for which the largest impacted population was estimated (Supplementary Table S3). Depending on the smoke tool, up to four of the five largest population impacts estimates were for burns at Carvers Creek State Park. Only VSmoke-Web and HYSPLIT-Web predicted large areas of concern and high population impacts for the largest prescribed burn conducted on NC State Parks land during the year examined (a 186 hectare burn at Morrow Mountain Park).
Differences among the smoke tools are further reflected in the overlap fractions between the areas of concern predicted. Fig. 3 summarises the overlap fraction among SSST, VSmoke-Web and HYSPLIT-PC-BlueSky predictions. Across the prescribed burns modelled, the average area of concern overlap fraction (Eqn 1) was highest between SSST and VSmoke-Web and equal to 28%. The overlap fraction between either of these tools and HYSPLIT-PC-BlueSky was much smaller, 7 or 14%, on average. When considering the overlap in areas of concern predicted by all three tools, the average overlap fraction was only 5%. Supplementary Fig. S2 summarises the overlap fractions among predictions from HYSPLIT-PC-BlueSky and HYSPLIT-Web. The differences in emissions profiles between these simulations lead to minimal average overlap between the HYSPLIT outputs.
Total areas of concern due to smoke pollution predicted by HYSPLIT-PC-BlueSky, VSmoke-Web and SSST for prescribed burns meeting the comparison criteria with the three tools (n = 18). The average overlap fractions between the tools are indicated as a percentage in the overlapping sections of the diagram. The centre section indicates the average overlap fraction between predictions from the three tools.
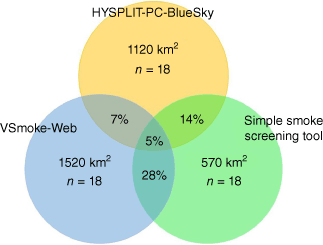
The overlap in estimates of population potentially impacted by smoke reflects the discrepancies in tool outputs. Fig. 4 summarises the average overlap fraction in population potentially affected based on SSST, VSmoke-Web and HYSPLIT-PC-BlueSky predictions of area of concern. Although the total area of concern predicted for the prescribed burns meeting the comparison criteria was smallest with HYSPLIT-PC-BlueSky, this area corresponds to the largest population across the tools. Only 9% or 15% of these individuals would have also been identified as at risk of moderate smoke pollution based on VSmoke-Web and SSST predictions, respectively, and a small fraction (6%) is within the areas of concern predicted by all the tools. Agreement between VSmoke-Web and SSST is larger, with a 28% overlap fraction in the populations potentially affected by smoke. When examining comparable predictions modelled by HYSPLIT-PC-BlueSky and HYSPLIT-Web, the differences in emissions profiles between the simulations resulted in population estimates varying by nearly 200 000 (Supplementary Fig. S2).
Total population within areas of concern due to smoke pollution predicted by HYSPLIT-PC-BlueSky, VSmoke-Web and SSST for prescribed burns meeting the comparison criteria with the three tools (n = 18). The average population overlap fractions between the tools are indicated as a percentage in the overlapping sections of the diagram. The centre section indicates the average population overlap fraction between predictions from the three tools.
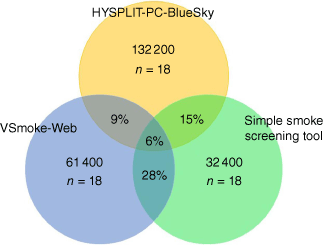
Examples of area of concern predictions modelled by HYSPLIT-PC-BlueSky, VSmoke-Web and SSST are shown in Fig. 5 for several burns at Carver’s Creek State Park. Although all of these occurred within the same state park, the differences among the tool outputs are substantial. Further, the predictions show no consistency in the discrepancies among the tools. For this set of burns, specific smoke tools can predict the largest or smallest area of concern, depending on the burn. These burns also provide insights into the implications of tool selection on land management. If burning decisions followed guidelines based on population potentially affected by smoke, the choice of tool could significantly impact fire planning. Management plans that include alerting neighbouring residents to potential smoke pollution prior to burns could entail targeting substantially different populations if different smoke tools were used to identify the individuals that should be reached.
Areas of concern due to smoke pollution predicted by HYSPLIT-PC-BlueSky, VSmoke-Web and SSST for four prescribed burns conducted at Carvers Creek Park near Fayetteville, NC, in 2016. The date of burn is listed in the top right of each panel. Census block group boundaries are outlined in grey. Population within areas of concern are listed for each panel. Predicted areas of concern typically overlapped near the ignition point but vary widely downwind. Panels (a) and (b) show how time-varying meteorology in HYSPLIT can affect predicted areas of concern. Panels (c) and (d) show that VSmoke-Web and SSST predictions can vary widely depending on the burn, and neither tool consistently results in a larger area of concern.
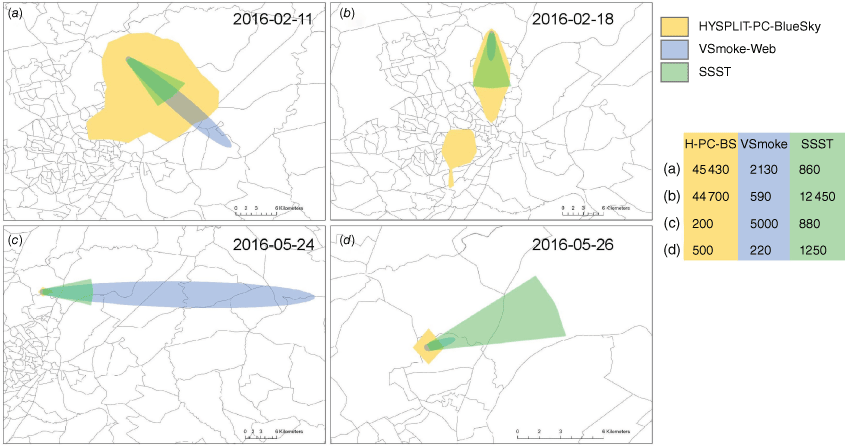
In our CMAQ simulation of PM2.5 associated with the prescribed fires, only five of the burns led to concentrations meeting the criteria used to compare areas of concern predicted by the tools (hourly PM2.5 ≥ 39 µg/m3). The CMAQ simulation resulted in greater dispersion of fire emissions and thus smaller areas of elevated PM2.5 concentrations. Comparatively lower concentrations predicted by CMAQ are likely associated with the Eulerian grid structure and coarser resolution of the model, which estimates uniform concentrations within each 4 × 4 km cell. This resulted in minimal comparable spatial smoke fields and population impacts. Decreasing the hourly PM2.5 concentration threshold considered to reflect the most recent AQI guidance for moderate concern, 30 µg/m3 (US EPA 2018), would increase the comparable areas of concern predicted among the tools. In the case of HYSPLIT-PC-BlueSky, the number of prescribed burns leading to areas of moderate concern due to smoke would increase by 33% if this lower hourly concentration level were considered.
Sensitivity of smoke predictions
As the smoke tools differ greatly in complexity and their inputs, it is difficult to isolate singular causes for the significant differences in results. Although we aimed to use the tools as consistently as possible for comparison, we additionally performed sensitivity analyses of available options to further investigate the drivers of differences between the modelling results. Using the burns highlighted in Fig. 5, we examined the differences in the predicted area of concern that arise from the available options for fuels, emission rates, ignition method, plume rise, duration and wind direction. Many of these options are not modifiable across all tools considered, in particular HYSPLIT.
Whereas the BlueSky Framework determines fuel type using the FCCS and the coordinates of the burn, the available options are more limited for VSmoke-Web and SSST. Each of these tools has four fuel type options, and VSmoke-Web has three fuel loading options for each fuel type. Supplementary Figs S3 and S4 show how fuel choices influence the areas of concern predicted by SSST and VSmoke-Web, respectively, and Supplementary Fig. S5 shows the influence of fuel loading in VSmoke-Web. In SSST, grass and litter fuels lead to similarly sized SSST areas of concern, while shrub and slash fuels lead to substantially greater predicted areas (4–30 times larger). In VSmoke-Web, the particulate emission rate (g/s) and the heat release rate (MW) vary with the fuel type, load and moisture scenario. Emissions can span an order of magnitude across fuel types and increase from grass, to litter, to shrub and up to slash. Predicted areas of concern do not vary linearly in response to fuels. Moderate and heavy fuel loads in VSmoke-Web release twice and three times the emissions of light loads, respectively. Fuel moisture selections determine the level of fuel consumption, which ranges from 90 to 25% as fuel moisture is varied from very dry to wet. Increasing available moisture levels in VSmoke-Web changes the emission rate by 30%. Supplementary Fig. S6 shows predicted areas of concern when varying the fuel moisture.
Two of the smoke tools have options for different ignition methods, but predicted areas of concern change in opposite ways depending on the tool. We assumed the ignition method for every burn was backing/spot, as this is the most commonly practiced method. When changed to head/aerial ignition, the VSmoke-Web area of concern is 40% that predicted using backing/spot ignition. With head or aerial ignition, VSmoke-Web assumes fires burn more intensely and plume rise is greater, changing the default plume rise fraction from −0.5 to 0.75 (where a negative value indicates smoke curtaining). However, when using head/aerial ignition in SSST, the predicted area of concern is over three times larger than that estimated with backing or spot ignition. The discrepancy reflects the difference in the tools’ treatment of the balance between fire intensity and plume rise beyond the mixing layer height derived from the ignition method used. Supplementary Fig. S7 shows the impact of ignition method on both SSST and VSmoke-Web predictions. There is no option for ignition method within HYSPLIT or the BlueSky Playground. Plume rise in HYSPLIT is calculated internally by the model based on heat release, wind velocity and friction velocity (Stein et al. 2015).
Among the tools considered, only VSmoke-Web has the option to modify burn duration. Decreasing the burn duration increases the area of concern, as shown in Supplementary Fig. S8. The emission rate in VSmoke-Web is proportional to the duration of the burn (e.g. reducing the duration from 8 to 4 h doubles the emission rate). Although only HYSPLIT uses time-varying meteorology, SSST is primarily intended to examine how wind may influence smoke impacts from a prescribed burn. As such, key inputs in SSST are wind direction and an indication of its potential variability (in degrees). As expected, increasing the wind direction range increases the area of concern radially (Supplementary Fig. S9). This allowance may improve planning capacity for a burn, as it highlights a range of areas that could be affected under variable wind conditions. VSmoke-Web does not include an option for variable wind direction.
The basic user inputs and operational learning curves of SSST and VSmoke-Web, coupled with their instantaneous results, make the use of these tools very practical for fire practitioners. However, as shown in this sensitivity analysis, many input options and assumptions represent significant simplifications that can lead to predictions lacking spatial and temporal nuance that may be important to plan smoke management. Although a major advantage of HYSPLIT is the time-varying meteorology, the tool set-up and interface(s) may be non-intuitive and require training. Although differing emissions assumptions across the versions of HYSPLIT can lead to very different results, the user can ultimately make adjustments using the PC version. Still, additional tools are typically needed to generate these inputs. Further, using HYSPLIT for planning purposes may be significantly limited by the availability of gridded forecast meteorology.
Discussion
Implications for smoke management
Prescribed fire plays an important role in supporting ecosystem services and reducing wildfire risk, but smoke from burns must be managed to protect human health and mitigate other detrimental effects. Traditionally, ventilation rates have been used to evaluate environmental conditions for smoke management. However, ventilation rates are highly simplified indicators of smoke dispersion that cannot be used to predict likely impacted downwind populations. Smoke dispersion tools can better aid prescribed burn planning but differences in complexity and user inputs greatly affect their smoke impact predictions. Understanding these differences, and how the choice of tool can influence burn-related decisions, are critical to improving smoke modelling for land management.
Here, we compared smoke tools varying in scientific complexity but commonly used for smoke management while planning prescribed burns. Our analysis of a year of operational burning on North Carolina State Parks land suggests that the differences among the tools are significant and inconsistent. The total smoke area predicted by the tools differs by thousands of square kilometres and, as such, average spatial agreement was low. When translated into numbers of residents potentially exposed to smoke over the course of a year, tool estimates vary by an order of magnitude. To our knowledge, no other work has systematically compared smoke modelling tool outputs and associated potential population impacts across a set of prescribed burns.
In our analysis of smoke modelling tools, we aimed to conduct burn simulations as consistently as possible across tools using the available options within them. However, differences among the tools’ inputs and predictions impede fully compatible simulations. Several assumptions included in our treatment of meteorology, including burn duration and start time, can influence smoke dispersion simulations (Garcia-Menendez et al. 2013; Miller et al. 2019). Differences in the tools’ treatment of prescribed fire emissions are unavoidable and contribute to discrepancies (Yang et al. 2011; Larkin et al. 2012, 2014). Although we focus on areas of concern due to moderate smoke pollution predicted by the tools, comparisons of predictions at different pollution levels may differ from our results.
The extent to which smoke dispersion predictions affects the capacity of land managers to conduct prescribed burns in practice remains uncertain and difficult to assess. Although ventilation rate estimates, such as VIS rates, are unequivocally grouped into burning categories instructing land managers to burn, not burn, or burn with specific considerations, clear thresholds or guidance are lacking for dispersion modelling. For example, the North Carolina Forest Service’s Guidelines for Managing Smoke from Forestry Burning Operations states that a ‘[m]odel run must show that the 24-h National Ambient Air Quality Standard (NAAQS) for PM2.5, primary and secondary, will not be violated as a result of particulate matter emitted from the burn’ (NCFS 2020). This condition does not specify a spatial scale. It would also be difficult for most prescribed burns to cause an exceedance of the 24-h NAAQS for PM2.5 value (35 µg/m3) downwind given that they typically only span several hours within a day, even though peak concentrations may far exceed the NAAQS value. Reliance on 24-h concentrations can conceal higher diurnal smoke levels (Altshuler et al. 2020). Further, the SSST and VSmoke-Web tools are not designed to predict 24-h PM2.5 concentrations and converting their outputs into ambient daily-average concentrations may not be possible.
The smoke modelling tools approved or recommended by different state or local authorities for prescribed burn permitting and planning vary. For example, the North Carolina Forest Service and Region 8 of the US Forest Service only accept dispersion modelling results from VSmoke, VSmoke-Web and the PC version of HYSPLIT. The Region 8 Air Resources Team recommends against using HYSPLIT-Web owing to concerns of overprediction of downwind concentrations (USFS 2017), an issue our comparison of tool outputs may also suggest. Specific training may further be required to use the tools to support prescribed burning projects. As we show here, predictions from smoke modelling tools can differ significantly and, depending on how land managers apply them, these discrepancies may lead to cases where using one tool allows for burning while employing another restricts it. The degree to which choice of smoke dispersion tool affects prescribed burning decision-making and influences the operations of prescribed fire programs is an important research need that must be addressed with a clearer understanding of smoke management in prescribed fire planning processes (Altshuler et al. 2020). Although our analysis of a prescribed fire program shows that improved and more consistent smoke dispersion tools could better support land management, clear guidelines on how to apply their predictions are also necessary to obtain these benefits.
The smoke simulations conducted here underscore several additional research needs. Our analysis covered prescribed burns in North Carolina State Parks and did not focus on the smoke modelling requirements of a specific authority. Additional comparisons of smoke dispersion predictions in other regions or landscapes, and produced with other tools approved by state and regional agencies, would show if the model discrepancies observed here are representative of prescribed fire planning across the US. Importantly, the lack of model evaluations against smoke measurements makes it infeasible to assess and compare the accuracy of tool predictions. Evaluating smoke dispersion tools is complicated by the short-lived and sporadic nature of prescribed fire projects, the scarcity of permanent air quality monitoring stations near most burns and limitations in satellite-based observations of air pollution. Fewer than 50% of the burns we modelled were detected using infrared sensors on MODIS satellites and smoke was visible (or discernible from clouds) in satellite imagery for fewer than 25% of burns. Temporary monitors and low-cost sensors placed near prescribed fires or in areas with frequent burning may provide valuable data to analyse smoke tool performance (Ottmar et al. 2017; Liu et al. 2019; Prichard et al. 2019; Huang et al. 2021). Additionally, important opportunities remain to advance smoke modelling tools for prescribed fire. Tools frequently used today are based on models developed decades ago, and are constrained by the absence of chemical process representations and overly simplified meteorology. Although Eulerian grid models are frequently updated to include new research findings, they continue to be ill-suited for day-to-day burning operations and typically predict atmospheric composition at spatial scales beyond those relevant to most prescribed fire projects. Addressing these research needs, while considering the value of smoke predictions for burn-related decisions, can improve support for prescribed fire programs.
Data availability
The data used for this study are available at https://fgarciam.wordpress.ncsu.edu/data.
Acknowledgements
We thank Jon Blanchard and the North Carolina State Parks for sharing operational burn data and information. We thank Kirk Baker, Shannon Koplitz and research staff at the US EPA for assisting with the CMAQ simulation and sharing meteorological fields and fire emission profiles. We thank Fiona Tennyson for assistance with computational modelling and analysis. We gratefully acknowledge the NOAA Air Resources Laboratory (ARL) for the provision of the HYSPLIT transport and dispersion model and READY website (https://www.ready.noaa.gov) used in this publication.
References
Achtemeier GL (1998) Predicting dispersion and deposition of ash from burning cane. Sugar Cane 1, 17-22.
| Google Scholar |
Achtemeier GL (2005) Planned Burn – Piedmont. A local operational numerical meteorological model for tracking smoke on the ground at night: model development and sensitivity tests. International Journal of Wildland Fire 14, 85-98.
| Crossref | Google Scholar |
Achtemeier GL, Goodrick SA, Liu Y, Garcia-Menendez F, Hu Y, Odman MT (2011) Modeling smoke plume-rise and dispersion from southern United States prescribed burns with daysmoke. Atmosphere 2, 358-388.
| Crossref | Google Scholar |
Afrin S, Garcia‐Menendez F (2020) The Influence of Prescribed Fire on Fine Particulate Matter Pollution in the Southeastern United States. Geophysical Research Letters 47, e2020GL088988.
| Crossref | Google Scholar |
Altshuler SL, Zhang Q, Kleinman MT, Garcia-Menendez F, Moore CT, Hough ML, Stevenson ED, Chow JC, Jaffe DA, Watson JG (2020) Wildfire and prescribed burning impacts on air quality in the United States. Journal of the Air & Waste Management Association 70, 961-970.
| Crossref | Google Scholar |
Anderson HE (1982) Aids to Determining Fuel Models For Estimating Fire Behavior. General Technical Report INT-122. (USDA Forest Service, Intermountain Forest and Range Experiment Station: Ogden, UT) 10.2737/INT-GTR-122
Appel KW, Napelenok SL, Foley KM, Pye HOT, Hogrefe C, Luecken DJ, Bash JO, Roselle SJ, Pleim JE, Foroutan H, Hutzell WT, Pouliot GA, Sarwar G, Fahey KM, Gantt B, Gilliam RC, Heath NK, Kang D, Mathur R, Schwede DB, Spero TL, Wong DC, Young JO (2017) Description and evaluation of the Community Multiscale Air Quality (CMAQ) modeling system version 5.1. Geoscientific Model Development 10, 1703-1732.
| Crossref | Google Scholar |
Breyfogle S, Ferguson SA (1996) User assessment of smoke-dispersion models for wildland biomass burning. General Technical Report PNW-GTR-379. (USDA Forest Service, Pacific Northwest Research Station: Portland, OR) 10.2737/PNW-GTR-379
Byun D, Schere KL (2006) Review of the Governing Equations, Computational Algorithms, and Other Components of the Models-3 Community Multiscale Air Quality (CMAQ) Modeling System. Applied Mechanics Reviews 59, 51-77.
| Crossref | Google Scholar |
Cascio WE (2018) Wildland fire smoke and human health. Science of the Total Environment 624, 586-595.
| Crossref | Google Scholar |
Fire Consortia for Advanced Modeling of Meteorology and Smoke (2021) VSmoke-Web. Available at https://weather.gfc.state.ga.us/GoogleVsmoke/vsmoke-Good2.html
Fowler C, Konopik E (2007) The history of fire in the southern United States. Human Ecology Review 14, 165-176.
| Google Scholar |
Frost CC (1998) Presettlement fire frequency regimes of the United States: a first approximation. In ‘Fire in ecosystem management: shifting the paradigm from suppression to prescription. Tall Timbers Fire Ecology Conference Proceedings, No. 20,’ 7-10 May 1996, Boise, ID. (Eds TL Pruden and LA Brennan) pp. 70–81. (Tall Timbers Research Station: Tallahassee, FL)
Garcia-Menendez F, Hu Y, Odman MT (2013) Simulating smoke transport from wildland fires with a regional-scale air quality model: Sensitivity to uncertain wind fields. Journal of Geophysical Research: Atmospheres 118, 6493-6504.
| Crossref | Google Scholar |
Goodrick SL, Achtemeier GL, Larkin NK, Liu Y, Strand TM (2013) Modelling smoke transport from wildland fires: A review. International Journal of Wildland Fire 22, 83-94.
| Crossref | Google Scholar |
Grell GA, Peckham SE, Schmitz R, McKeen SA, Frost G, Skamarock WC, Eder B (2005) Fully coupled ‘online’ chemistry within the WRF model. Atmospheric Environment 39, 6957-6975.
| Crossref | Google Scholar |
Grell G, Freitas SR, Stuefer M, Fast J (2011) Inclusion of biomass burning in WRF-Chem: Impact of wildfires on weather forecasts. Atmospheric Chemistry and Physics 11, 5289-5303.
| Crossref | Google Scholar |
Haines TK, Busby RL, Cleaves DA (2001) Prescribed Burning in the South: Trends, Purpose, and Barriers. Southern Journal of Applied Forestry 25, 149-153.
| Crossref | Google Scholar |
Huang R, Hu Y, Russell AG, Mulholland JA, Odman MT (2019) The impacts of prescribed fire on PM2.5 air quality and human health: Application to asthma-related emergency room visits in Georgia, USA. International Journal of Environmental Research and Public Health 16, 2312.
| Crossref | Google Scholar |
Huang R, Lal R, Qin M, Hu Y, Russell AG, Odman MT, Afrin S, Garcia-Menendez F, O’Neill SM (2021) Application and evaluation of a low-cost PM sensor and data fusion with CMAQ simulations to quantify the impacts of prescribed burning on air quality in Southwestern Georgia, USA. Journal of the Air & Waste Management Association 71, 815-829.
| Crossref | Google Scholar |
Kobziar LN, Godwin D, Taylor L, Watts AC (2015) Perspectives on Trends, Effectiveness, and Impediments to Prescribed Burning in the Southern US. Forests 6(3), 561-580.
| Google Scholar |
Lafon CW (2010) Fire in the American South: Vegetation Impacts, History, and Climatic Relations. Geography Compass 4, 919-944.
| Crossref | Google Scholar |
Larkin NK, O’Neill SM, Solomon R, Raffuse S, Strand T, Sullivan DC, Krull C, Rorig M, Peterson J, Ferguson SA (2009) The BlueSky smoke modeling framework. International Journal of Wildland Fire 18, 906-920.
| Crossref | Google Scholar |
Larkin NK, Strand TM, Drury SA, Raffuse SM, Solomon RC, O’Neill SM, Wheeler N, Huang S, Rorig M, Hafner HR (2012) Phase 1 of the Smoke and Emissions Model Intercomparison Project (SEMIP): Creation of SEMIP and Evaluation of Current Models. JFSP Research Project Reports. 42. http://digitalcommons.unl.edu/jfspresearch/42
Larkin NK, Raffuse SM, Strand TM (2014) Wildland fire emissions, carbon, and climate: US emissions inventories. Forest Ecology and Management 317, 61-69.
| Crossref | Google Scholar |
Lavdas LG (1996) Program VSMOKE—Users Manual. General Technical Report SRS-6. (US Department of Agriculture, Forest Service, Southern Research Station: Asheville, NC) 10.2737/SRS-GTR-6
Lipsett M, Materna B, Stone SL, Therriault S, Blaisdell R, Cook J (2008) Wildfire Smoke: A Guide for Public Health Officials. Available at https://www.amadorgov.org/home/showpublisheddocument/352/635219509683530000
Liu Y, Kochanski A, Baker KR, Mell W, Linn R, Paugam R, Mandel J, Fournier A, Jenkins MA, Goodrick S, Achtemeier G, Zhao F, Ottmar R, French NHF, Larkin N, Brown T, Hudak A, Dickinson M, Potter B, Clements C, Urbanski S, Prichard S, Watts A, McNamara D (2019) Fire behaviour and smoke modelling: Model improvement and measurement needs for next-generation smoke research and forecasting systems. International Journal of Wildland Fire 28, 570-588.
| Crossref | Google Scholar |
Mandel J, Beezley JD, Kochanski AK (2011) Coupled atmosphere–wildland fire modeling with WRF 3.3 and SFIRE 2011. Geoscientific Model Development 4, 591-610.
| Crossref | Google Scholar |
Mandel J, Amram S, Beezley JD, Kelman G, Kochanski AK, Kondratenko VY, Lynn BH, Regev B, Vejmelka M (2014) Recent advances and applications of WRF-SFIRE. Natural Hazards and Earth System Sciences 14, 2829-2845.
| Crossref | Google Scholar |
Melvin MA (2012) National prescribed fire use survey report. Coalition of Prescribed Fire Councils. Technical Report 01-12. Available at https://prescribedfire.net
Melvin MA (2020) National Prescribed Fire Use Survey Report. Technical Bulletin 04-20. Coalition of Prescribed Fire Councils, Inc. and National Association of State Foresters. Available at https://www.prescribedfire.net/pdf/2020-Prescribed-Fire-Use-Report.pdf
Miller C, O’Neill S, Rorig M, Alvarado E (2019) Air-quality challenges of prescribed fire in the complex terrain and wildland urban interface surrounding Bend, Oregon. Atmosphere 10, 515.
| Crossref | Google Scholar |
Mitchell RJ, Liu Y, O’Brien JJ, Elliott KJ, Starr G, Miniat CF, Hiers JK (2014) Future climate and fire interactions in the southeastern region of the United States. Forest Ecology and Management 327, 316-326.
| Crossref | Google Scholar |
NOAA Earth Systems Research Laboratory (2015) High-Resolution Rapid Refresh (HRRR). Available at https://rapidrefresh.noaa.gov/hrrr/
North Carolina General Statutes (1999) ‘North Carolina Prescribed Burning Act.’ Ch. 106, Article 80. Available at https://www.ncleg.net/EnactedLegislation/Statutes/HTML/ByArticle/Chapter_106/Article_80.html
Odman MT, Huang R, Pophale AA, Sakhpara RD, Hu Y, Russell AG, Chang ME (2018) Forecasting the impacts of prescribed fires for dynamic air quality management. Atmosphere 9, 220.
| Crossref | Google Scholar |
O’Neill SM, Larkin N, (Sim) K, Hoadley J, Mills G, Vaughan JK, Draxler RR, Rolph G, Ruminski M, Ferguson SA (2008) Chapter 22 Regional Real-Time Smoke Prediction Systems. Developments in Environmental Science 8, 499-534.
| Crossref | Google Scholar |
Ottmar R, Brown TJ, French NHF, Larkin NK (2017) Fire and Smoke Model Evaluation Experiment (FASMEE) study plan. Joint Fire Sciences Program Project 15-S-01-01, Available at https://www.firescience.gov/projects/15-S-01-01/project/15-S-01-01_FASMEE_StudyPlan_Final_07-11-17.pdf
Prichard S, Larkin NS, Ottmar R, French NHF, Baker K, Brown T, Clements C, Dickinson M, Hudak A, Kochanski A, Linn R, Liu Y, Potter B, Mell W, Tanzer D, Urbanski S, Watts A (2019) The Fire and Smoke Model Evaluation Experiment – A plan for integrated, large fire-atmosphere field campaigns. Atmosphere 10, 66.
| Crossref | Google Scholar |
Reid CE, Brauer M, Johnston FH, Jerrett M, Balmes JR, Elliott CT (2016) Critical review of health impacts of wildfire smoke exposure. Environmental Health Perspectives 124, 1334-1343.
| Crossref | Google Scholar |
Rolph G, Stein A, Stunder B (2017) Real-time Environmental Applications and Display sYstem: READY. Environmental Modelling & Software 95, 210-228.
| Crossref | Google Scholar |
Rolph GD, Draxler RR, Stein AF, Taylor A, Ruminski MG, Kondragunta S, Zeng J, Huang H-C, Manikin G, McQueen JT, Davidson PM (2009) Description and Verification of the NOAA Smoke Forecasting System: The 2007 Fire Season. Weather and Forecasting 24, 361-378.
| Crossref | Google Scholar |
Scire JS, Strimaitis DG, Yamartino RJ (2000) ‘A User’s Guide for the CALPUFF Dispersion Model.’ (Earth Tech, Inc.: Concord, MA) 10.1016/0196-9781(85)90178-0
Skamarock WC, Klemp JB, Dudhia J, Gill DO, Barker DM, Duda MG, Huang X-Y, Wang W, Powers JG (2008) A Description of the Advanced Research WRF Version 3. National Center for Atmospheric Research, Mesoscale and Microscale Meteorology Division Technical Note NCAR/TN–475+STR. (University Corporation for Atmospheric Research: Boulder, CO) 10.5065/D68S4MVH
Southern Fire Exchange (2021) Models. Available at https://southernfireexchange.org/models-tools-apps/
Stein AF, Draxler RR, Rolph GD, Stunder BJB, Cohen MD, Ngan F (2015) NOAA’s HYSPLIT Atmospheric Transport and Dispersion Modeling System. Bulletin of the American Meteorological Society 96, 2059-2077.
| Crossref | Google Scholar |
USFS (2017) Using Smoke Modeling Tools for Prescribed Fire Planning and Implementation: A Quick Set of Instructions. p. 23. (USDA Forest Service, Region 8 Air Resource Team). Available at https://webcam.srs.fs.usda.gov/tools/vsmoke/FEPS%20VSMOKE%20and%20HYSPLIT%20Instructions.pdf
Wilkins JL, Pouliot G, Foley K, Appel W, Pierce T (2018) The impact of US wildland fires on ozone and particulate matter: A comparison of measurements and CMAQ model predictions from 2008 to 2012. International Journal of Wildland Fire 27, 684-698.
| Crossref | Google Scholar |
Yang ES, Christopher SA, Kondragunta S, Zhang X (2011) Use of hourly Geostationary Operational Environmental Satellite (GOES) fire emissions in a Community Multiscale Air Quality (CMAQ) model for improving surface particulate matter predictions. Journal of Geophysical Research: Atmospheres 116, D04303.
| Crossref | Google Scholar |