Revisiting tropical pasture intake: what has changed in 50 years?
E. Charmley

A CSIRO Agriculture and Food, Private Mail Bag PO, Aitkenvale, Townsville, Qld 4814, Australia.
B CSIRO Agriculture and Food, Centre for Environment and Life Sciences, Private Bag 5, Wembley, WA 6913, Australia.
C CSIRO Agriculture and Food, 306 Carmody Road, St Lucia, Qld 4067, Australia.
Abstract
The measurement and prediction of pasture intake in extensive grazing systems, typical of northern Australia, remain elusive after 50 years of research. The aim of this paper is to review research conducted over the past 50 years, highlight advances in understanding, discuss remaining challenges and consider future developments with digital technologies. While the fundamental components of voluntary intake are well understood, their measurement is difficult, particularly in extensive grazing systems, which has limited the development of predictive models that adequately address the interplay of factors influencing intake from the bite to the landscape scale. Ongoing research by the authors is used as an example to highlight the potential application of digital technologies to overcome limitations in measurement and prediction. Digital technologies offer the opportunity for monitoring factors that control voluntary pasture intake at scale and under commercial conditions. However, our ability to ground-truth novel indices of intake remains limited without ongoing development of physical methods. This will limit the accuracy and precision of predictive models incorporating digital technologies that can be applied to the extensive grazing conditions of northern Australia. The advent of precision livestock management for extensive cattle production is essential if the industry is to remain viable in the future where production is transparent, ethically sound and environmentally defensible as well as profitable.
Keywords: Beef cattle, grazing behaviour, information technology, intake, modelling, pasture, review, tropical.
Introduction
The critical importance of feed intake to animal performance and productivity has been known for centuries. Poppi (1996) summarised the state of research in the 1990s and concluded that prediction equations would continue to improve as larger datasets were amassed, statistical methods improve, and rapid methods of characterisation would evolve. Yet despite decades of research, it is still challenging to measure or predict the intake of pastures by grazing animals. Methods such as use of oesophageally cannulated animals to estimate diet quality, coupled with indigestible markers or total faecal collection are laborious and time consuming, while before and after grazing measurement to estimate disappearance constrain the grazing environment (Penning 2004). In this review, the advances in our understanding of the control of pasture intake are discussed with particular reference to the extensive grazing systems of northern Australia. While our knowledge has increased, the ability to directly measure or predict pasture intake remains elusive. The advent of digital technologies may offer new opportunities to observe in detail the sward, the animal, and its behaviour for research and under commercial conditions, and use these observations to improve intake measurement and prediction.
Foraging theory
Foraging theory can be simply described as the drive for an individual to meet its nutrient requirements to survive and reproduce. This involves balancing the need to maximise the supply and balance of nutrients, while expending the least amount of energy, with the need to avoid becoming nutrients for animals higher up the food chain (Owen-Smith et al. 2010). While this is a rather simplistic description, it serves as a good starting place in understanding how this theory has relevance for domestic cattle in extensive grazing conditions. In the domestic context, humans have become the dominant predator, but we aim to improve on nature by minimising other predators, enhancing the feed supply, and improving the fitness of the species in a particular environment.
Tropical pasture intake is primarily influenced by two factors, the rate of disappearance of material from the reticulorumen (hereafter termed the rumen), which can be characterised as potential intake (PISC 2007), and the presentation of nutrients in the grazing environment that influences the actual intake (Coleman 2005). Under intensive cattle production, where feed availability is controlled by management, dry-matter (DM) intakes can exceed 3% of liveweight (LW), with energy-dense feed producing gains of over 2.5 kg/day in growing cattle and feed efficiency in terms of the ratio of DM intake to unit LW gain of less than 6:1 (NASEM 2016). However, for the grazing ruminant, the animal exploits a more natural fibre-based diet, which, without the intermediary step of enteric digestion, would not play a role in the human food chain. Therefore, we accept lower and more variable performance and efficiencies. Nevertheless, within the constraints of the system, understanding the controlling factors of intake and how to manipulate these for optimum performance is still important.
Why is pasture intake important?
Feed is the single largest variable cost in ruminant production systems (Webster 2013; Greenwood and Bell 2014). The efficient conversion of feed into animal product therefore underpins the economics and productivity of ruminant livestock systems. Yet, for grazing cattle, that constitute about 95% of the Australian beef herd, feed efficiency cannot be calculated as we remain unable to measure or accurately predict pasture intake, especially in the extensive grazing lands of northern Australia. The advent of genomics and marker-assisted selection has revolutionised cattle improvement (Hayes et al. 2013). It can be argued that the advances in ruminant nutrition have not kept up with the advances in genetic improvement. The heyday of ruminant nutrition in the 1970s and 1980s saw major advances in the understanding of ruminant nutrition on pastures and the factors that control pasture intake and efficiency. But this level of endeavour was not sustained. The recent development of residual feed intake (RFI), the difference between observed and expected feed intake to attain a given LW gain has led to renewed research investigating the underlying factors controlling intake and growth of ruminants (Herd and Arthur 2009; Kenny et al. 2018). While RFI does not tell us much about the control of pasture intake, research into the mechanisms contributing to RFI have highlighted differences in maintenance requirements, digestibility and efficiency of utilisation of energy for gain among individuals and among breeds (Cantalapiedra-Hijar et al. 2018). Selection for improved growth rate or feed efficiency in livestock has contributed to the development of more efficient genetics (Hammond 2006). The following question remains: does selection under confinement feeding reflect efficiency under grazing conditions? Constraints to intake such as potential intake rate, chemical and physical properties of forage, the ability of animals to select higher-quality material through selective gazing, and environmental factors such as climate, topography and access to water, create a much more complex model of intake than do confinement feeding conditions (Weston 2002). Herd et al. (2002) suggested that there was a positive genetic correlation between feedlot and pasture RFI. However, there is also evidence that RFI measured under confinement feeding does not correlate well with RFI measured on pasture (Lawrence et al. 2013; Oliveira et al. 2018).
It can be argued that the feeding standards developed in temperate climates 40 plus years ago are not applicable to the tropical cattle or today. The ability to select for ‘grazing-efficiency’ traits must be included in breeding programs of the future.
The management of pastures to ensure optimum quantity and quality of the feedbase is constrained when the rate and composition of biomass removal by cattle is unknown. Today pasture is considered to be more than a feedbase. It also protects the soil from erosion (Ash et al. 1995; O’Reagain and Scanlan 2013), contributes to carbon storage in soils (Boval and Dixon 2012) and provides habitat for a plethora of animals and plants that have intrinsic value (Kutt et al. 2012). The relationship of pasture biomass with these factors (soil erosion, carbon storage and biodiversity) has not changed over time, but societal attitudes, and hence the importance placed on these factors, have. More than ever, our ability to control grazing to maintain a healthy pasture ecosystem is critical to both the economic and environmental drivers of the industry (Chilcott et al. 2020; Bell and Sangster 2023).
The contribution of grazing ruminants in northern Australia to increases in atmospheric methane, and hence their contribution to global warming, is significant and linked directly to DM intake (Charmley et al. 2016). Poor diet quality limits DM intake, reduces LW gain and weaning rate and results in high methane per unit of product (methane intensity; Harrison et al. 2015). Nevertehless, the contribution of soils and trees to carbon storage in eucalypt woodlands of the north counteract livestock emissions (Bray et al. 2014).
Major advances in productivity in intensive crop and livestock systems have arisen through precision management of the individual plant or animal throughout the production cycle. Efficient use of nutrients (crops) or feed (animals) is central to optimising the production system. In contrast, for extensive grazing systems, only a few indices (e.g. weight, weaning rate/weight, deaths) are measured at the herd level across seasons. The challenge of precision livestock management under the extensive production systems of northern Australia is daunting but essential if the industry is to remain viable in a world where social licence to operate and access to markets insist that we manage our cattle with precision and sustainability. Central to this is the measurement or accurate prediction of pasture intake and feed efficiency. The adoption of new technologies in intensive agriculture have led to improvements in efficiency (e.g. Berckmans 2014). The opportunity exists for the pastoral industry of northern Australia to also benefit from these advances.
Factors influencing intake
Pasture quality
Tropical forages are noted for their poor nutritive value relative to temperate forages. In part this is attributed to rapid maturation after summer rains, leading to increases in the fibre fraction, and particularly lignin, and concomitant reductions in N content (Minson 1981a, 1981b; Poppi and McLennan 2010). Course fibrous feed compromises the ability of cellulolytic bacteria to degrade structural carbohydrates, leading to reduced digestibility (Minson 1981b) and low rate of passage from the rumen (Forbes 2003). The rate of passage is directly related to intake and this is controlled primarily by the neutral detergent fibre (NDF) content of the diet (Mertens 1987). The close association between the NDF, apparent digestibility and DM intake (DMI) is well understood for mixed diets (NASEM 2016). For pasture-based diets, the plant species (C3 versus C4, grass versus legume) can have modifying effects on rate of passage and the relationship between NDF, digestibility and intake (Krizsan et al. 2010).
The importance of digestibility in the control of tropical pasture intake has been established for decades. For example, Topps (1969) presented data showing the characteristic increase in organic-matter (OM) intake as OM digestibility increased in steers of varying bodyweight (BW) grazing veld grasses. However, even in that early study, the author noted that ‘It would seem that the relationship between intake and digestibility for subtropical grasses is not so close as that proposed by Blaxter et al. (1961) for temperate roughages’ (Topps 1969, p. 253). Nevertheless, numerous papers since then have demonstrated that digestibility of tropical forages is central to regulating intake. Within experiments, numerous equations have described this relationship (Hodgson et al. 1977; Coleman et al. 2014). Hodgson et al. (1977) summarised several early papers that suggested that the intake response to increasing digestibility was less above an OM digestibility of 60% (predominantly temperate forages), than it was below 60% OM digestibility (predominantly tropical forages). The authors suggested separate relationships of tropical and temperate forages, as follows:
where OMI is OM intake in g/kg BW and OMD is OM digestibility as a percentage. This suggestion that the relationship between digestibility of tropical or temperate forages and intake differs has been explored further (Freer 1981; Minson 1981a). Minson (1981a) concluded that at any given digestibility, the intake of tropical forages may be as much as 20% higher than that of temperate forages. However, the source of the data used was not cited and was represented by a graph with just seven points (reproduced here as Fig. 1a). It could equally be argued that a single line representing both tropical and temperate forages was just as applicable. Indeed, when data also presented in Minson (1981a) and representing different tropical and temperate grass species are depicted graphically (Fig. 1b) a single relationship is apparent. We therefore question the notion that tropical forages have a higher intake than do temperate forages at the same digestibility. This is important because the higher intake for tropical grasses has been incorporated into the PISC (2007) equation for the determination of intake of tropical forages (PISC 2007). This model has been widely used as the basis for estimating intake of tropical pastures by researchers and extension specialists (McLennan 2014). Minson (1981a) postulated sound morphological reasons why higher intake of a tropical (C4) grass compared with a temperate (C3) grass of similar digestibility might be expected. He pointed out that at 60% digestibility, a tropical grass is still vegetative while a temperate grass is reproductive. Differences between C3- and C4-dominated pastures in spatial distribution, forage structure, feed-component selectivity, rumen breakdown and rate of passage are all factors that potentially confound relationships between digestibility and intake between tropical and temperate pastures.
The relationship from Minson (1981a) supporting the view that (a) intake of tropical forages is 20% higher than that of temperate forages of similar digestibility and (b) the relationship derived from table 8.1 in the same paper. Red squares denote tropical forages, blue circles temperate forages.
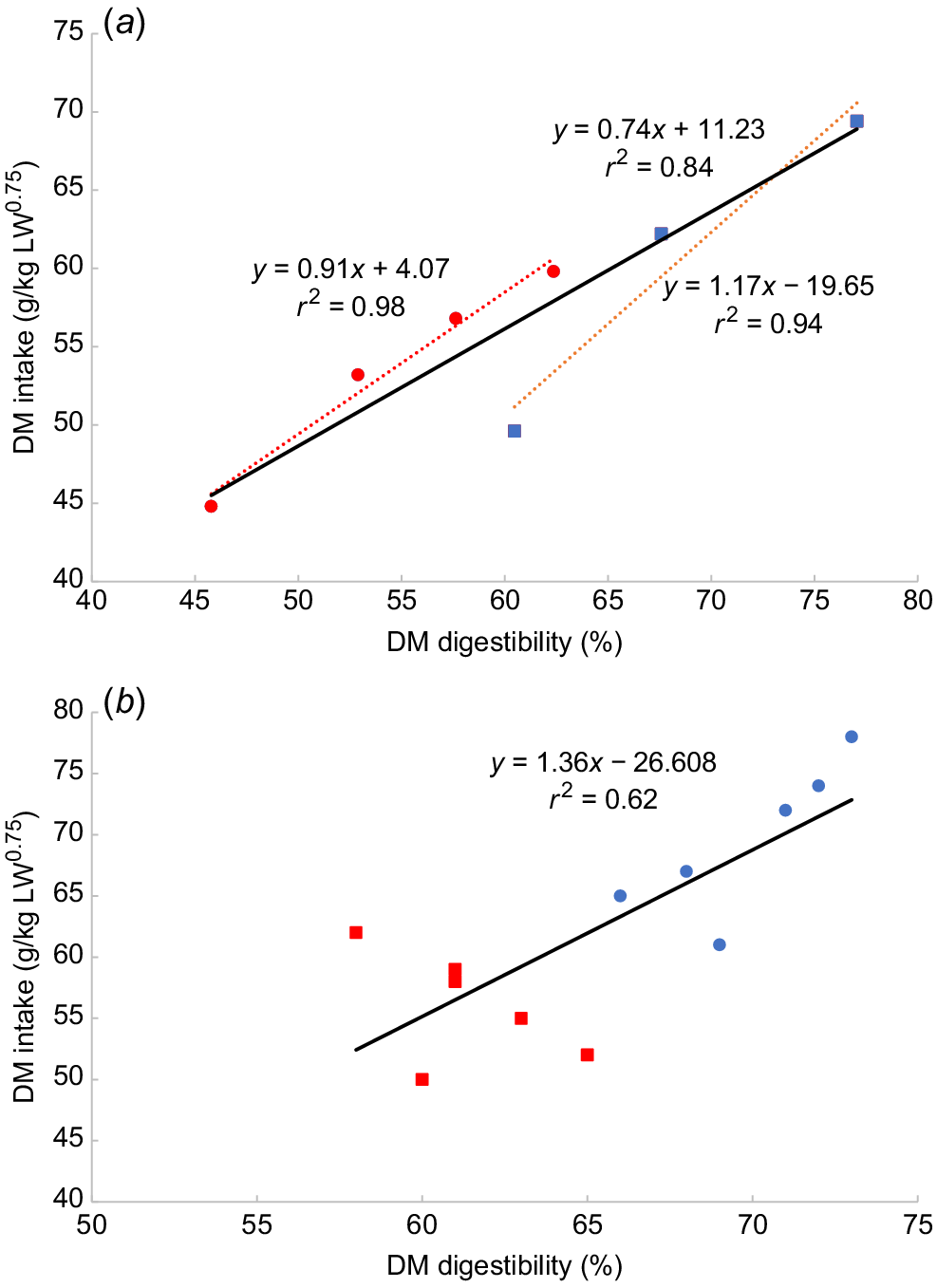
At equal NDF, intake of temperate legumes is approximately 20% higher than that of grasses (Freer and Jones 1984). Thornton and Minson (1973) compared eight grasses and six legumes and confirmed that intake of legumes was 28% higher than that of grasses in sheep, due to shorter retention time in the rumen. There are limited data on the relative intake of tropical grasses and legumes. Typically, when legume content of tropical grass-based diets is increased, there is both a legume effect and a diet-quality effect, which cannot be separated (Stifkens et al. 2022). However, Archimède et al. (2011) conducted a meta-analysis of data, comparing 28 tropical C4 grasses with 12 tropical legumes. Across all diets, legumes were lower in NDF, higher in crude protein (CP), but not higher in intake (Table 1). However, OMD was much lower for legumes (48%) than grasses (62%), which biased the data. Consequently, intake relative to OMD was 35% higher for tropical legumes than for C4 grasses. One explanation for this is that lignification reduced fibre digestibility of legumes but did not have as marked an effect on intake. Indeed, the disparity between tropical legumes and C4 grasses in OMI relative to NDF was much less (20%) than in OMI relative to OMD (Archimède et al. 2011).
Item | Tropical grasses (n = 28) | Tropical legumes (n = 12) | |||||
---|---|---|---|---|---|---|---|
Mean ± s.d. | Minimum | Maximum | Mean ± s.d. | Minimum | Maximum | ||
NDF (% DM) | 65 ± 9.8 | 41 | 75 | 57 ± 9.9 | 44 | 69 | |
CP (% DM) | 11 ± 6.3 | 2.4 | 25 | 16 ± 5.7 | 9.3 | 24 | |
OMI (% LW) | 1.77 ± 7.0 | 0.74 | 3.10 | 1.86 ± 4.6 | 0.73 | 2.52 | |
OM digestibility (%) | 62 ± 0.57 | 51 | 71 | 48 ± 0.53 | 42 | 57 |
Research by Poppi et al. (1981) demonstrated that intake of leaf was higher than that of stem in C4 grasses, even though both were of similar NDF content and digestibility. A similar observation was made for the tropical legume Lablab purpureus by Hendricksen et al. (1981). Both authors attributed this to the faster comminution of leaf than stem particles during rumination, allowing for earlier escape from the rumen via the reticulo-omasal orifice.
Low nutritive value of tropical pastures, especially in the dry season, can be offset to some degree by selective grazing for higher-quality components of the pasture (Topps 1968; Thomas et al. 2010). Fig. 2 shows results over a 12-month grazing trial at Lansdown Research Station in northern Queensland (19°39′S, 146°50′E; E Charmley, P McSweeney, P Greenwood, S Denman, G Bishop-Hurley, unpubl. data). Samples of the standing herbage, harvested at 50 mm above ground level were analysed for CP by using near-infrared (NIR) spectroscopy (Coates and Dixon 2011) and compared with CP measured by NIR in faecal samples. Dietary CP content was consistently higher than pasture CP (Fig. 2a), especially when pasture CP content was low (Fig. 2b), indicating that selectivity is influenced by change in diet quality.
Effect of season (a) on pasture (blue circles) and diet (red squares) crude protein concentration measured using NIR and (b) on diet crude protein compared with pasture crude protein (blue circles), measured at varying times over 12 months in 2021. Data from Charmley et al. (unpubl. data).
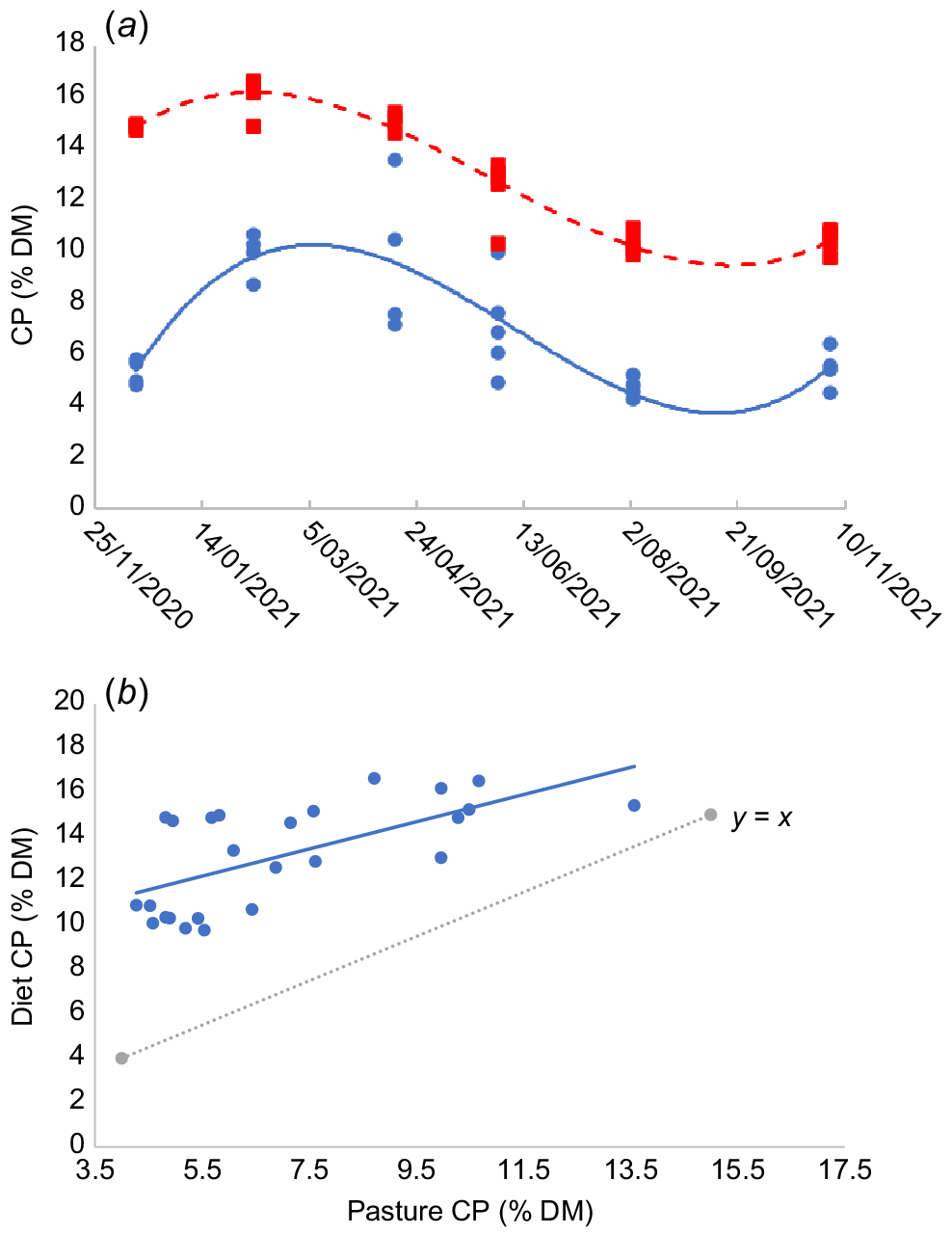
Nitrogen is essential to provide the building blocks for microbial growth. According to PISC (2007), optimum microbial protein synthesis of 130 g/kg digestible OM (DOM) can be achieved when N supply is above 30 g/kg DOM. Below this concentration, N availability in the diet limits the growth of rumen microbes, slows rate of DM degradability, rate of passage from the rumen, and hence intake (Lazzarini et al. 2009). Supplementation of with N up to approximately 1.3% DM (Batista et al. 2017) increases microbial protein synthesis, which can be accompanied by increased rate of passage and intake (Hunter and Siebert 1985; McLennan et al. 2017). However, Panjaitan et al. (2010) demonstrated that the relationship between forage CP content and efficiency of microbial protein synthesis is not simple, with different responses being observed between tropical grasses of similar NDF. This was possibly related to the interactions among varying values of N, lignin and digestibility among species. In low-N diets, if N content per se elicits an additional influence on intake over and above digestibility, then a curvilinear or broken-stick response on intake to N would be expected. In some datasets, this is apparent (e.g. Hunter and Siebert 1987) but in others it is not. Fig. 3 summarises data from six trials with tropical grasses. It shows the change in OM intake of tropical grasses in relation to increased N content. Over a three-fold range in N content, which covers the expected point at which N limitations on microbial protein synthesis would cease, there was no evidence of curvilinearity or a break point in slopes. For practical purposes, it appears that the digestibility effect on intake is so large that it effectively masks the nuances around supply of N for microbial protein synthesis to promote intake.
The relationship between N content of the diet and OM intake (OMI) for a range of tropical forages. The arrow indicates approximate N content range at which the N effect on intake would be nullified (Siebert and Hunter 1977; Poppi and McLennan 1995; Panjaitan et al. 2010; Kennedy and Charmley 2012; Batista et al. 2017; McLennan et al. 2017).
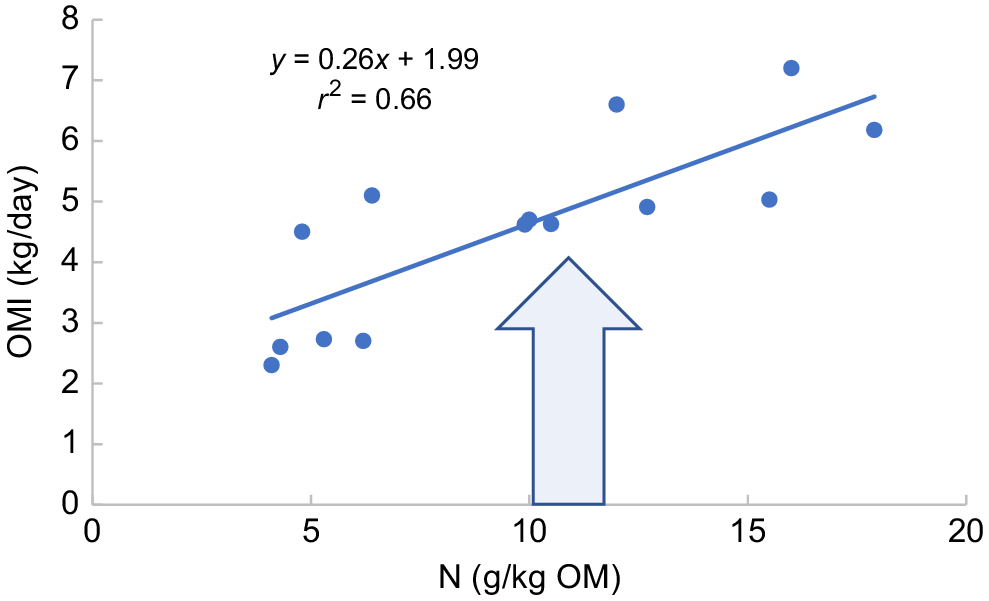
Pasture availability (relative intake)
The adequate supply of pasture and the conditions under which that supply is presented have major implications for voluntary intake under extensive grazing systems of northern Australia. Despite a large amount of research into the ecological and grazing aspects of foraging theory, there is little information on how these influence pasture intake. This section will consider the factors that influence intake, and how they may affect the ability to measure or estimate intake under such conditions.
The relationship between the grazing animal and the sward is complex and defined across spatial and temporal scales (Varva and Ganskopp 1998; Roguet et al. 1998). Nutritional optimisation is but one of several factors that influence intake. Other factors include environmental (paddock size, distance to water, shelter, predators; Hunt et al. 2007), animal (breed, genetics, social hierarchy within the group, cognitive ability; Roguet et al. 1998) and sward (Benvenutti et al. 2009) factors. Roguet et al. (1998) identified four spatiotemporal scales of grazing behaviour, namely, bite, feeding site, feeding station and habitat. Pasture intake is influenced by variables at each scale.
Cumulative intake over a period such as a day is the product of the number and mass of individual bites. Extensive research has demonstrated that bite mass is strongly influenced by diet quality as defined by digestibility, fibre content or leaf:stem ratio. Early research with the tropical grass Setaria sphacelata by Chacon and Stobbs (1976) elegantly demonstrated that cattle graze in horizons, with the uppermost horizon grazed first, comprising mostly leaf. As grazing continues and the upper horizon becomes depleted, cattle graze proportionally more of lower horizons that have a higher proportion of stem and less leaf. Bite mass declines as biomass is progressively removed from the sward, forcing cattle to graze in lower horizons with more stem and less leaf. Cattle initially compensate by increasing bite rate and grazing time. However, ultimately bite rate and grazing time decrease and pasture intake declines. It is not a reduction in nutritive value per se that reduces intake but the avoidance of stem in the bite and the effect of this on bite mass.
At this micro-scale, the potential effect of these morphological differences in grasses on voluntary intake is difficult to assess. Da Silva et al. (2013) used individual grazing paddocks to overcome this issue (Table 2). Grazing pressure in swards of Bothriochloa brizantha was manipulated to maintain the grass at four sward heights (100, 200, 300, and 400 mm) over almost a year. As noted nearly 40 years previously (Chacon and Stobbs 1976), bite mass was highest and bite rate lowest at the highest sward height. However, this study also demonstrated that sward height also influenced pasture intake, LW gain and diet quality (Table 2). An inevitable consequence of the design was the large differences in stocking rates. Thus, there was clear relationship among stocking rate, grazing behaviour at the bite scale, intake and LW gain. In agreement with Chacon and Stobbs (1976), diet quality as determined from CP and OMD was less important than was sward height.
Item | Sward height (mm) | ||||
---|---|---|---|---|---|
100 | 200 | 300 | 400 | ||
Stocking rate (AU/plot) | 4.1 | 3.1 | 2.1 | 1.1 | |
Grazing days (n/plot) | 178 | 116 | 82 | 51 | |
Bite mass (g/bite) | 0.5 | 0.8 | 1.2 | 1.5 | |
Bite rate (bites/minute) | 46 | 30 | 24 | 18 | |
Crude protein (% DM) | 14 | 13 | 12 | 11 | |
In vitro OM digestibility (%) | 67 | 66 | 63 | 62 | |
DMI (% LW) | 1.3 | 1.8 | 1.8 | 2.0 | |
LW gain (kg/day) | 0.19 | 0.51 | 0.75 | 0.93 |
A similar, but short-term experiment was conducted by Benvenutti et al. (2016) with sward heights of 330, 440 and 610 mm. For the three grazing-height treatments, there was a clear break point when residual sward height was approximately 100 mm, at which digestibility, intake and rumination time all declined. Thus, cattle can compensate for increased stemminess and reduced sward height, up to a point, but eventually intake will be affected.
The preceding experiments (Chacon and Stobbs 1976; Da Silva et al. 2013; Benvenutti et al. 2016) all demonstrate a relationship between sward biomass/height and bite mass/rate that appears to be related to the leaf:stem ratio in the grazing horizon. Consequently, pasture intake is reduced through the presence of stemmy material in the grazing horizon slowing the prehension of feed, which may or may not be accompanied by a concomitant reduction in digestibility, potentially further contributing to reduced intake.
A feeding station is defined as the sward surface accessible to the animal without moving its forefeet (Roguet et al. 1998). Depending on the nature of the sward and climatic and edaphic variables, they may be close together or further apart, but groups of stations comprise a feeding site, which in a grazing context is often defined as a patch or uniform area differentiated from surrounding areas due to its nature and appearance (MacArthur and Pianka 1966). From a grazing efficiency perspective, the biomass and quality within a station are important. Abundant leafy grass will result in larger bite mass, than does either lower biomass (Da Silva et al. 2013) or high biomass of stemmy material (Gregorini et al. 2011). Gregorini et al. (2011) examined in detail the relationship between grazing behaviour at the station and site level, by using short-term observations of rumen-evacuated cattle offered swards of low, medium and high sward height/biomass. They noted that as sward height was reduced, intake per feeding station declined, steps per minute and eating distance increased, the area grazed increased, bite rate increased, but bites per feeding station declined. In simple terms, cattle spent more time looking for grass, as the availability per feeding station was reduced. Cattle were optimising their energy budget by visiting more feeding stations but taking fewer and shallower bites at each station. Clearly, these observations are relevant in northern grazing systems. This view is supported by Stobbs (1970), who reported that time spent grazing tropical grasses was 550 versus 360 min per day in more homogenous temperate pastures. Hunt et al. (2007) suggested that animals seek out grazing stations low in biomass but high in quality. The proximity of stations within a patch, and the proximity and size of patches will have important implications on the cumulative DM intake, with many smaller bites reducing intake rate compared with fewer larger bites (Da Silva et al. 2013).
In northern Australian grazing conditions, cattle may have to walk considerable distances between patches and water points and have marked preference for certain patches within a landscape. McGavin et al. (2018) used GPS to relate daily distances walked to paddock size. At a GPS fix rate of 10 s, daily distance travelled increased rapidly as paddock size increased to approximately 50 ha, but thereafter paddock size had little influence on distance travelled, which reached a plateau at approximately 15 km/day. Hunt et al. (2007) demonstrated how cattle utilise patches within large paddocks (9–57 km2) by using GPS tracking. Within the landscape, cattle spent more time close to waterpoints and other attractants in the landscape. Within these large areas, cattle will establish home ranges (Hunt et al. 2007). These show some elasticity with paddock size (Hunt et al. 2007), topography (Bailey et al. 2006), and familiarity (Tomkins and O’Reagain 2007). Hunt et al. (2007) found that home ranges vary, occupying a higher proportion of small paddocks than larger paddocks, consistent with there being a maximum distance travelled limiting the area an animal can access in a day (McGavin et al. 2018). Patches within home ranges become over-grazed due to their proximity to attractants in the landscape. The preference to spend more time close to attractants in the landscape leads to biomass contours, with low biomass close to attractants and increasing biomass further away from attractants. This piosphere effect has been associated with larger paddocks (Manthey and Peper 2010; Frank et al. 2012) and contributes to poor overall pasture utilisation of ~10% standing biomass (Hunt et al. 2007). Coleman (2005), citing Raeburn (1986), demonstrated the concept of relative intake in relation to the maximum intake expected for that class of animal on that quality of pasture. Relative intake was attributed to biomass availability and stocking rate (Fritz and Garine-Wichatitsky 1996). Intake increases rapidly to approximately 90% of maximum when pasture biomass is approximately 2–3 t DM/ha. Under extensive, tropical conditions, it is the patch or home-range biomass that is critical, rather than the whole paddock, and at this scale there is a lack of evidence to support a threshold biomass at which relative intake approaches potential intake.
The highly seasonal nature of diet nutritive value in northern Australia causes fluctuations in the plane of nutrition that results in compensatory gain. Cattle transitioning from a low plane of nutrition to a higher one, such as after the transition from the dry to the wet season (break of season), typically exhibit higher than expected rates of LW gain (e.g. Tomkins et al. 2006; Silva et al. 2022). This compensatory growth has been attributed to increased lean tissue (protein plus associated water) deposition (Berge 1991; Mota et al. 2020), increased proportional digestive tract weight as well as a lower proportional maintenance requirement (Berge 1991). Thus, for a given level of DM intake, cattle will exhibit increased LW gain. In addition, cattle transitioning from a restricted plane of nutrition frequently exhibit increased appetite, with increases in DM intake being considered to average 10–20% (Berge 1991). The changes in feed conversion and intake associated with compensatory growth therefore influence the relationships between intake and both diet nutritive value and LW gain. Estimations of DMI rely on one or both of these variables depending on the estimation method chosen. Thus, in the wet season, DMI would be routinely underestimated to a varying extent and compensatory gain should be accounted for when attempting to predict or estimate wet-season pasture DM intake. However, this is not the case and represents a deficiency in the accurate prediction of DMI.
Lower maintenance requirements for Brahmans have been confirmed with the view that they result from a lower fasting heat production (Vercoe 1970; Chizzotti et al. 2007; Cadenas-Soberanis et al. 2021). Given that there are differences in metabolism between taurine and indicine cattle, presumably as an adaption to their original environments, it follows that there may be differences in voluntary intake. Research suggests that if there are differences, they are small and inconsistent. Hunter and Siebert (1985, 1987) compared voluntary intake of Herefords or Angus steers with Brahman steers. Over 12 treatment comparisons, they observed a 5% higher intake in Brahmans than the British breeds, but this was attributed to one result with young cattle, and when these data were removed, there was no difference. Azevêdo et al. (2010), with feedlot diets, found the opposite response when gain was above 1.4 kg/day, with Bos indicus × Bos taurus cross-cattle having a higher intake than did B. indicus cattle. For slower-growing cattle, given less energy-dense diets, both breed types exhibited the same voluntary intake. It can be concluded that although there are metabolic differences between the two subspecies, voluntary intake of forage-based diets is essentially the same.
Methods of pasture intake measurement
Several reviews (Coleman 2005; Decruyenaere et al. 2009; Smith et al. 2021) and the book Pasture Intake Handbook (Penning 2004) cover the various methods of pasture-intake measurement and developments over the years. The classical method of sampling oesophageal extrusa to determine diet digestibility coupled with total collection of faeces has evolved into less invasive methods (Penning 2004). The dosing or release of in-rumen capsules of inert markers such as chromic oxide or even chain alkanes allows for estimation of faecal output, which, when combined with faecal NIR estimates of diet digestibility, represents a less invasive option to the total collection method (Dove and Mayes 1991). Administration of markers is problematic as attaining a steady-state concentration in the rumen and, hence, faeces is influenced by dosing frequency or variable release rates from in-rumen capsules. While inert markers present in the diet have been studied, such as acid-insoluble ash or lignin, the question of how inert they really are remains (Sales 2012). The alternative approach of measuring the disappearance of DM from a grazed sward can also be used (Greenwood et al. 2014). Methods to measure before- and after-grazing biomass, the comparison of biomass in and outside exclusion cages and measurement of sward height have all been used with varying degrees of success. Given the shortcomings of physical methods and the cost, labour and invasiveness of these methods, prediction equations remain the best option for on-farm application.
Review of empirical equations
The equation for prediction of pasture intake published in PISC (2007) is the most widely used in Australia. Although developed for temperate regions, factors can be included to account for potential differences between C3 (temperate) and C4 (tropical) grasses. The DMI predictions from this (PISC 2007) and other equations (Hodgson et al. 1977; Siebert and Hunter 1977; Minson and McDonald 1987; Azevêdo et al. 2010; Coleman et al. 2014; Fernandes et al. 2022; Table 3) were tested against papers with observed measures of intake from tropical forages. Five papers (Kloppenburg et al. 1995; Boval et al. 2007; Krueger et al. 2008; Madruga et al. 2017; Velazco et al. 2017) with observed measures of intake using 14 comparisons that included LW gain data were used to evaluate the equations requiring LW gain. Two additional papers (Ash and McIvor 1998; Andrade et al. 2016) with observed measures of intake contributing 17 additional comparisons were used to evaluate equations that required data only on diet quality.
Source | Equation inputs required | |
---|---|---|
Equations based on animal and diet characteristics | ||
PISC (2007) | LW, birth weight, age, digestibility, legume content, C3:C4 | |
Coleman et al. (2014) | LW, OMD | |
Equations based on LW and LW change | ||
Minson and McDonald (1987) | LW, LWG | |
Azevêdo et al. (2010)A | LW, LWG | |
Fernandes et al. (2022)B | LW, LWG | |
Equations based on digestibility or N content | ||
Hodgson et al. (1977) | OMD | |
Siebert and Hunter (1977) | N |
AEquation for Nellore cattle (Bos indicus).
BModel 1.
None of the equations was able to provide precise and accurate predictions of observed intakes (Table 4). The PISC (2007) model showed very poor correlation with observed data, confirming the conclusions made by McLennan (2014) and McLennan et al. (2020). A lack of data on the age and genetics of the animals involved in the published literature may have contributed to poor performance, as these are key inputs required for the model. Further, the PISC model relies on the Allden and McDWhittaker (1970) relationship between herbage availability and rate of intake developed from temperate-pasture grazing studies. This relationship may be quite different for tropical pastures due to high spatial heterogeneity and other sward characteristics mentioned earlier. The Minson and McDonald (1987) equation based on LW and LW gain showed the best agreement, with observed values having the lowest root mean square prediction error (RMSE). The Azevêdo et al. (2010) and Fernandes et al. (2022) equations, also based on LW and LWG and derived from large Brazilian datasets, both performed reasonably well. A major limitation of these methods is the length of time required to record an accurate measure of LW gain, particularly if the diet characteristics are changing, and accurate LW is susceptible to errors arising from compensatory gain. In extensive grazing conditions where diet quality is poor, the energy requirement for activity (walking, grazing, etc.) can be up to 40% of the total energy requirements (McGavin et al. 2018) and affects equations relying on LWG (e.g. Minson and McDonald 1987). Given these methods are easily applied in the field and appear to work quite well, the use of digital technologies to (1) improve LW measurement through development of rolling average LW gain from in-paddock autonomous weighting systems and (2) measure activity from on animal GPS sensors, should further improve their utility for research and commercial application.
Source | Predicted/observed | Slope | r2 | Root mean square error (kg) | |
---|---|---|---|---|---|
Equations based on animal and diet characteristics | |||||
PISC (2007) | 1.26 | 0.26 | 0.10 | 2.12 | |
PISCrA | 0.96 | 0.34 | 0.18 | 1.18 | |
Coleman et al. (2014) | 0.96 | 0.53 | 0.37 | 1.20 | |
Equations based on LW and LW change | |||||
Minson and McDonald (1987) | 1.00 | 0.61 | 0.57 | 0.94 | |
Azevêdo et al. (2010)B | 0.94 | 0.73 | 0.56 | 1.22 | |
Fernandes et al. (2022)C | 0.82 | 0.44 | 0.49 | 1.82 | |
Equations based on digestibility or N content | |||||
Siebert and Hunter (1977) | 0.82 | 1.14 | 0.23 | 3.65 | |
Hodgson et al. (1977) | 1.43 | 1.57 | 0.39 | 2.49 |
ARevised equation without C4 factor.
BEquation for Nellore cattle (Bos indicus).
CModel 1.
Under controlled feeding conditions, response in intake to changes in digestibility or CP has been well characterised and is clearly related to the appropriate balance of nutrients in the rumen, such as, for example, the ME:N ratio (Panjaitan et al. 2010). However, under grazing conditions, availability of pasture supply, the heterogeneity in space (patch grazing), quality (stem, N content, legume versus grasses) and time (change in biomass and quality) effectively reduce the actual intake relative to potential intake (Allden and McDWhittaker 1970; Coleman 2005). Thus, it is not surprising that the relationships derived between intake and N content (Siebert and Hunter 1977) or digestibility (Hodgson et al. 1977) failed to predict intake under grazing conditions. It was expected that equations, including characteristics of both the diet and the animal, would have out-performed other equations. Although the Coleman et al. (2014) equation based on LW and DM digestibility underestimated intake, it responded well to the range in observed intake, having an RMSE of 1.20 kg.
The poor performance of the PISC (2007) model for predicting intake of tropical forages by tropically adapted cattle was addressed by McLennan (2014) and McLennan et al. (2020). They observed that the model overpredicted DMI, especially at higher intakes, i.e. the slope of the relationship was significantly different from 1. These authors revised the equations defining the utilisation of energy by cattle for growth and released a spreadsheet intake calculator (QuikIntake), which utilised the PISC (2007) methodology to predict DMI. The relationships between predicted intakes using QuikIntake and those observed in a number of pen-feeding studies (Mclennan et al. 2020) are shown in Fig. 4. Relative to the PISC (2007) model, changes were made to the maintenance requirements, the efficiency of use of energy for maintenance and gain, the energy value of gain, and the energy density of the diet. With these changes, the QuikIntake model better predicted intake of pen-fed cattle and was defined by the following equation:
where DMI is DM intake in kg/day, r2 is the correlation coefficient and SSE is the sum of squared errors. Dove et al. (2010) reviewed the difficulties of accurately estimating intake of low-quality tropical pastures, highlighting the importance of herbage supply and legume content in the prediction of actual intake. They concluded that prediction of intake from pasture and animal characteristics was so fraught with difficulties that a better option was to predict intake from known growth rate relying on accurate prediction of diet ME (from faecal NIR) and efficiencies of energy utilisation, noting that the latter have been called into question by McLennan et al. (2020).
Relationships between dry-matter intake using QuikIntake and those observed for cattle fed forage diets (after McLennan et al. 2020). Eqn 1 PISC equation; Eqn 2 is Eqn 1 adjusted for changes in the maintenance requirements, energetic efficiency for maintenance, and the energy value of gain; Eqn 3 is Eqn 2 adjusted for changes in the energy value of the diet; Eqn 4 is Eqn 3 adjusted for changes in the energetic efficiency for gain; and Eqn 5 is Y = X.
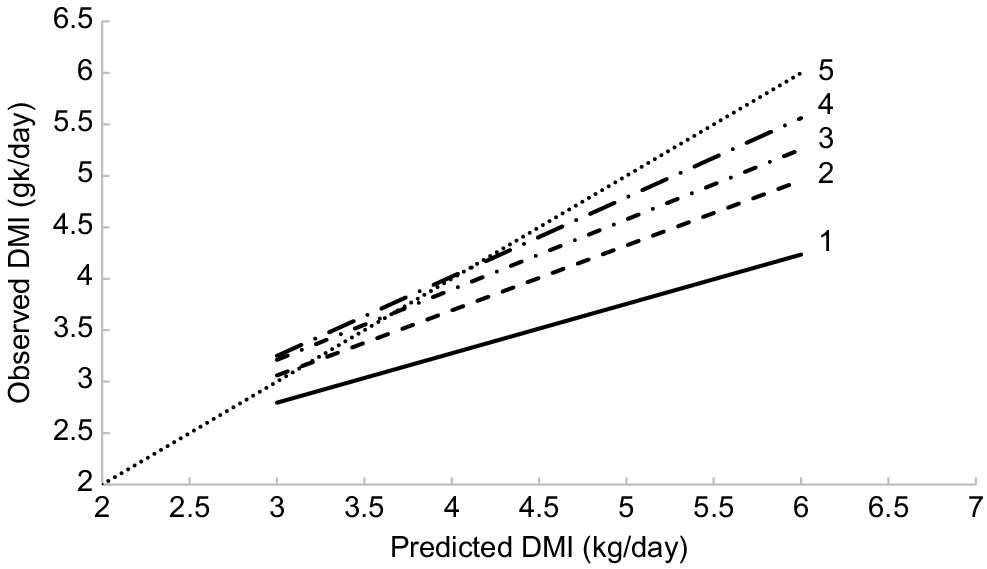
We adopted a different approach in attempting to improve the predictive ability of PISC (2007) for DMI, by looking at the relationships between diet characteristics and intake. It was noted that incorporating the ‘C4 factor’ of 0.16 used in PISC (2007) markedly increased the DMI. The use of this factor in PISC (2007) was attributed to data published by Minson (1981a). In this review, we concluded that the Minson (1981a) data relating intake to digestibility could be represented by a single line as opposed to two separate lines for temperate and tropical diets. Thus, this factor was removed from a revised PISC equation (PISCr) used in our analysis and this resulted in improvements in accuracy but not precision (Table 4).
Can technology provide solutions?
Digital technologies are advancing rapidly and their application in assisting our understanding of livestock behaviour is increasing (Smith et al. 2021). How these technologies can assist researchers in understanding voluntary intake on pastures is yet to be fully realised, but the promise of monitoring animal behaviour under varied grazing situations to integrate our existing knowledge of factors influencing intake is exciting. Remote sensing of pasture characteristics is now a commercial reality for Australian producers and the recent integration with on-animal tracking capability (https://www.mla.com.au/extension-training-and-tools/tools-calculators/australian-feedbase-monitor/) demonstrates that the grazing industry is finally entering the digital age. These commercial developments will inevitably improve pasture management and animal production in the near term and will drive the demand for research to improve the quantitative estimation of voluntary intake for producers. Smith et al. (2021) published a comprehensive review of the methods and sensors now available to monitor grazing behaviour and, by extension, estimate voluntary intake.
Here, we focus on selected data that have relevance to understanding voluntary intake in extensive grazing systems in northern Australia. The temporal, spatial and nutritional heterogeneity of tropical pastures present challenges in developing methods that can measure intake under such diverse conditions. Historically, these challenges were addressed by specifically focussing on the components of grazing behaviour and the relationship to intake. While this resulted in fine-scale understanding of these various components, such as diet quality, bite characteristics, selective grazing and patch grazing, it has been impossible to integrate reductionist science into a systems framework as complex as grazing. While models exist that attempt to achieve this (e.g. Illius and Gordon 1991; PISC 2007; Coleman et al. 2014; NASEM 2016) only PISC (2007) can be applied to tropical grazing situations. However, the model is a poor predictor of observed measures of tropical pasture intake due to a lack of integrated observational data. Digital technologies may offer the solution by providing integrated data collection of pasture, grazing and performance characteristics that collectively influence voluntary intake.
Digital technologies can distinguish cattle behaviours including, grazing, walking, ruminating, bites, and resting. These can be coded further into relationships, including grazing per unit distance walked, bites per minute, duration and frequency of bite events (Smith et al. 2016). These relationships match with observations of grazing behaviour such as instantaneous intake rate (bite rate), feeding stations (bite events), site and patch grazing (grazing per unit distance walked). It is not currently practicable to use a digital approach of such detail under commercial conditions. A database of digital signatures that have been classified into behaviours using visual identification from video or direct in-field observations is a required prerequisite. The use of machine learning and common measurement platforms are essential to expediting this challenge. However, this is not a trivial task. Development of an intake prediction method from grazing behaviour is ongoing but has already taken over 5 years (Greenwood et al. 2017; Hu et al., in press). Nevertheless, improvements in technology, energy storage and generation, miniaturisation and communications will accelerate adoption of these technologies.
The needs of any commercial system differ according to the requirements of the technology. For example, if ranking individuals within a herd for feed efficiency on pasture is the goal, then estimation of past intake is sufficient. However if pasture budgeting is the goal, then some means to predict future intake are required.
The components of a method to estimate past intake for growing animals over a defined time could include the following:
Satellite or drone-based imagery of pasture biomass and heterogeneity
Estimates of diet quality by using faecal NIR
Measurement of LW and LWG by using remote in-paddock weighing
Estimate of activity and behaviours by using GPS and accelerometry data from on animal devices
An app to integrate information on pasture biomass and quality, animal weight change and animal behaviour to predict intake from metabolisable energy (ME) expenditure (MJ/day) divided by ME of the diet (MJ/kg DM) to estimate DM intake (kg/day).
The components of such a model are already commercially available, or will be within a few years. A predictive model would require all of the above plus a pasture-growth model driven by weather predictions, with accompanying measures of uncertainty that would allow for stocking-rate scenarios. Extrapolating such models from growing cattle to include breeding cattle adds another layer of complexity and uncertainty.
A recent study (Charmley et al., unpubl. data) demonstrated several applications of digital technologies that might work in practice. In the first scenario, the Greenwood et al. (2017) equation is applied to our measures of grazing time. They have developed a method to estimate intake by using grazing time according to the following equation:
where DMI is dry-matter intake in kg/day, GT is grazing time in hours, r2 is the correlation coefficient and RSD the residual standard deviation. Using preliminary data from one Brahman heifer collected in late October–early November 2021 in the Lansdown trial (Charmley et al., unpubl. data), the Greenwood et al. (2017) equation estimated DM intake to be 6.2 kg/day.
The second scenario employs digital technologies to estimate energy requirements of the animal for activity, maintenance, and gain (and potentially reproduction). From a knowledge of the distance travelled (km/day) and grazing and ruminating time (h/day), energy expenditure for activity can be calculated. Knowledge of animal LW, sex, breed, condition and age can be used to estimate maintenance (excluding activity), and LW gain can determine energy for growth. These data are from the same animal and dates as those used to estimate intake from grazing time. The heifer weighed 319 kg, was gaining 0.0 kg/day and was consuming a diet with a DMD of 56%, being equivalent to 7.9 MJ/kg DM. By using PISC (2007), the combined energetic expenditure for activity, maintenance and gain can be calculated (47.2 MJ/day). From the faecal NIR estimate of ME (7.9 MJ/day), the predicted DMI was 6.0 kg/day (1.9% LW), close to that estimated from grazing time (6.2 kg/day; Greenwood et al. 2017). The above scenarios show the theory of the approach, but also demonstrate the proportional importance of energy requirements for activity in cattle grazing tropical pastures. In the energetics example, activity accounted for 31% of total energy requirement. Estimated intake using the equation of Minson and McDonald (1987), which relies solely on LW and LW change, was 5.8 kg/day and does not account for variation in activity. As activity varies with paddock size, and pasture characteristics, the ability to measure activity should improve an energetics basis for predicting intake in extensive grazing conditions.
Digital technologies can also assist in dealing with understanding home ranges and patch grazing in very large paddocks. By using the same study (Charmley et al., unpubl. data), detailed observations of cattle behaviour were combined with detailed characterisation of the sward. GPS collars were placed on Brahman heifers grazing 16 ha paddocks at the Lansdown Research Station, northern Queensland. Data analysis of this trial is ongoing; so, only preliminary data from one group will be used to illustrate the utility of this approach. Fig. 5 shows the spatial characteristics of grazing behaviours and Fig. 6 shows pasture biomass. While visualisation is useful, examining the data behind the response surfaces allows for determination of metrics that can be used to augment intake prediction.
Activity map for one heifer from 29 September to 1 October 2021, showing positions while grazing (green), walking (red), ruminating (yellow), and resting (blue). Data from Charmley et al. (unpubl. data).
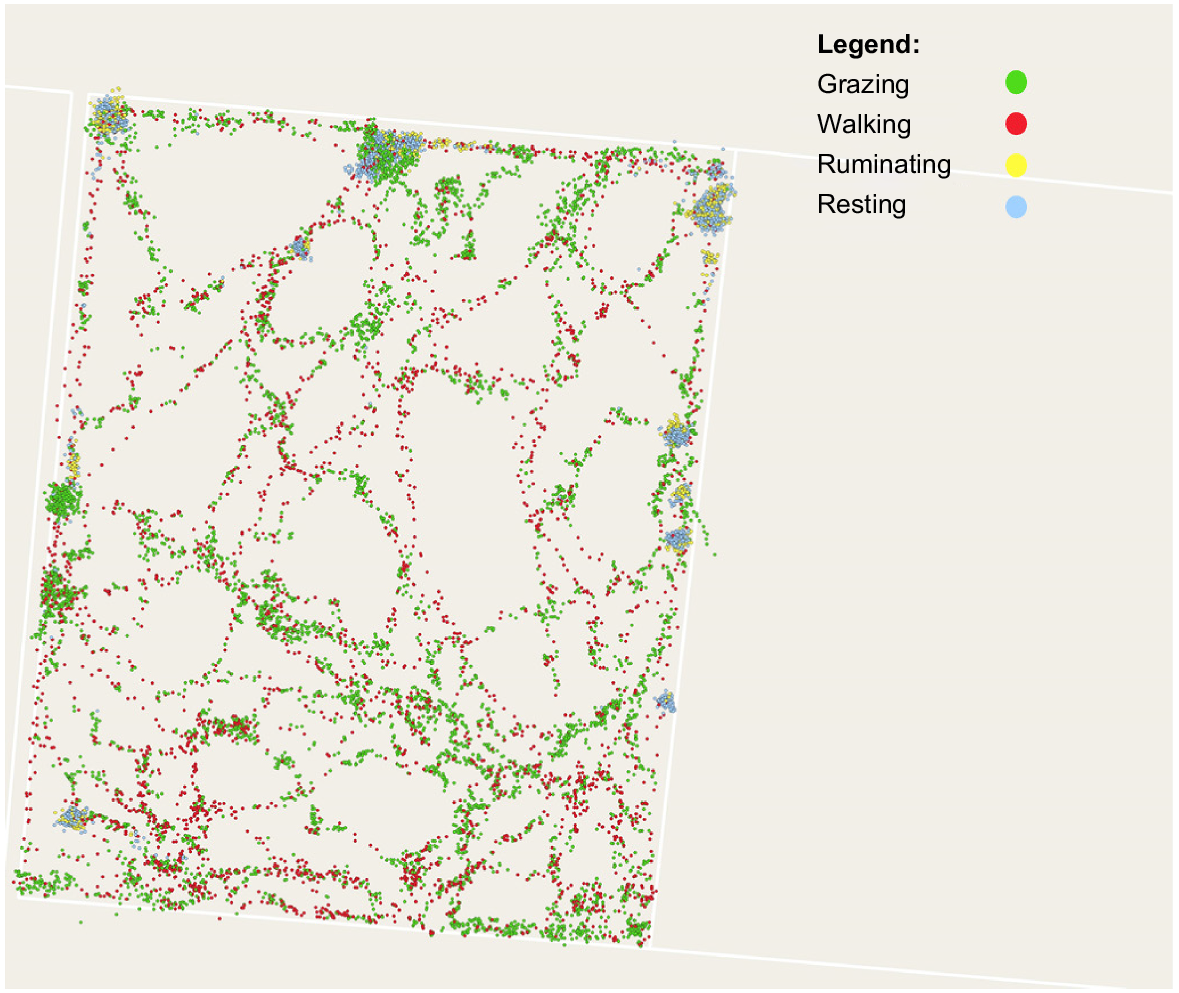
Spatial distribution of biomass (t DM/ha) measured on 6 November 2021 in the same paddock as Fig. 4. Data from Charmley et al. (unpubl. data).
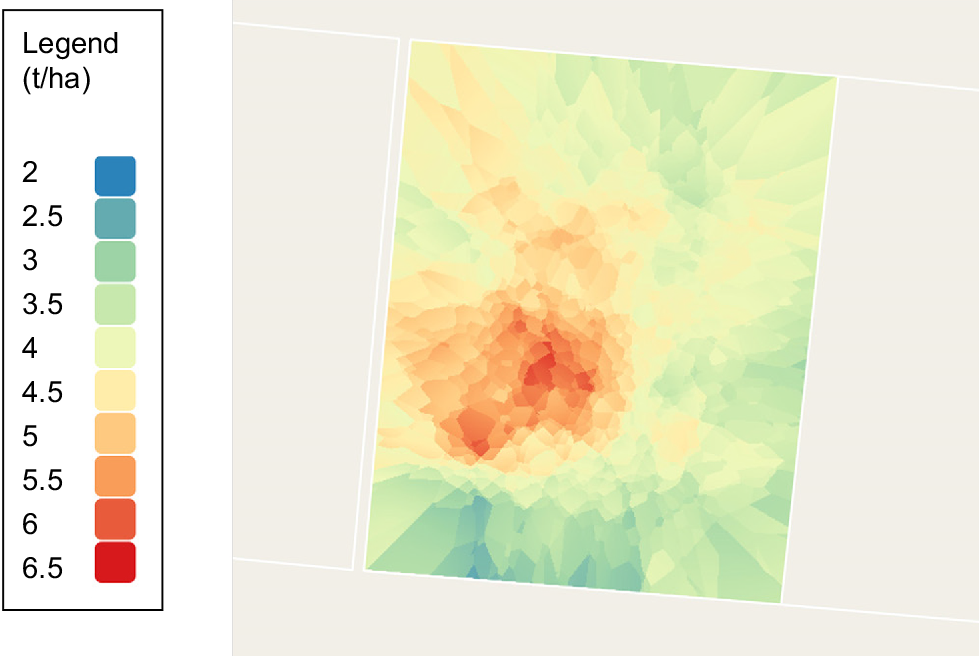
For example, biomass DM data were categorised in 1 t/ha assemblages that can be envisaged as analogous to grazing patches. Preference indices were developed for the frequency rates with which cattle visited each biomass assemblage. As biomass increased, preference declined, suggesting that cattle avoided areas of high biomass, as noted by Hunt et al. (2007). These technologies allow for automated measurement of the interaction between patch biomass and grazing preference within the paddock. By applying knowledge of the influence of biomass on relative intake (Coleman 2005), pasture availability at the patch scale can be incorporated into a simple model that adjusts potential intake derived from diet ME (calculated from faecal NIR) to actual DMI. Currently, such a method relies on collar-mounted GPS systems, but ear tag-sized versions appropriate for commercial use are becoming available (e.g. https://cerestag.com).
Digital technologies offer a range of measurements at a resolution and frequency previously not possible. The ability to monitor in near real-time animal status (LW from walk over weighing, diet composition from faecal NIR), animal behaviour (grazing activities from tag-mounted behaviour monitoring) and pasture characteristics (biomass from satellite imaging) at scale and across varied environments should provide enough data to greatly improve predictions of voluntary intake under extensive grazing conditions. Improving the precision and accuracy of voluntary intake will allow the selection of appropriate cattle for the environment, sustainably match the productivity of the sward with the requirements of the animal, and more accurately meet market specifications. Digital technologies offer the prospect of introducing precision livestock management to the extensive grazing industry.
While these preliminary observations are encouraging, challenges remain. From a research perspective, a direct physical measurement of DMI by grazing animals is still needed. Faecal NIR has been validated as a method to estimate ME of the diet, but ongoing research is required to update the method to expand the database to a wider range of forages and forage mixtures. Animal house data with cattle and sheep are required to calibrate spectra with in vivo digestibility data. Accurate measures of ME can then be combined with marker-based methods of estimating faecal excretion of DM and nutritional components of interest. The alkane method has been extensively researched and offers one method (Dove and Mayes 1991). Greenwood et al. (2014) developed a ‘pasture intake research platform’ and used DM disappearance in small plots with single animals as an alternative approach. A coordinated research approach to address these requirements is needed to standardise a methodology that can be used to measure intake across a wide range of grazing scenarios.
Machine-learning techniques are revolutionising the interpretation of behavioural signals that correlate with grazing behaviours such as grazing, ruminating, walking and resting. While it is hoped that universal algorithms could be applied to all grazing conditions, our preliminary data suggest that this may not be the case. Further research is required to test the robustness of models both within and between intensive and extensive grazing environments.
Conclusions
The zenith of research activity into understanding the control of voluntary intake from tropical forages was 40 years ago. Those experiments established the fundamentals of pasture intake and have since been refined. However, the scarcity of data relevant to northern grazing conditions remains an impediment to improving our understanding of intake of these extensive, heterogenous pastures. Extrapolation of data from temperate environments cannot account for the heterogeneity found under more extensive situations and also fails to account for differences in plant morphology and chemistry.
Some of the previously held views regarding intake of ‘tropical’ diets should be re-examined, but direct measurement of tropical pasture intake is possibly more difficult today than it was in the past due to animal welfare and health and safety constraints. The advent of digital technologies represents an opportunity to significantly improve the measurement of voluntary DMI in northern Australia, but significant challenges remain. Concerted and coordinated research effort is needed to realise this opportunity.
Data availability
The data used to generate some results in this paper will be shared upon reasonable request to the corresponding author.
References
Allden WG, McDWhittaker IA (1970) The determinants of herbage intake by grazing sheep: the interrelationship of factors influencing herbage intake and availability. Australian Journal of Agricultural Research 21, 755-766.
| Crossref | Google Scholar |
Andrade EA, Almeida EX, Raupp GT, Miguel MF, de Liz DM, Carvalho PCF, Bayer C, Ribeiro-Filho HMN (2016) Herbage intake, methane emissions and animal performance of steers grazing dwarf elephant grass v. dwarf elephant grass and peanut pastures. Animal 10, 1684-1688.
| Crossref | Google Scholar |
Archimède H, Eugène M, Marie Magdeleine C, Boval M, Martin C, Morgavi DP, Lecomte P, Doreau M (2011) Comparison of methane production between C3 and C4 grasses and legumes. Animal Feed Science and Technology 166–167, 59-64.
| Crossref | Google Scholar |
Ash AJ, McIvor JG (1998) Forage quality and feed intake responses of cattle to improved pastures, tree killing and stocking rate in open eucalypt woodlands of north-eastern Australia. The Journal of Agricultural Science 131, 211-219.
| Crossref | Google Scholar |
Ash AJ, McIvor JG, Corfield JP, Winter WH (1995) How land condition alters plant–animal relationships in Australia’s tropical rangelands. Agriculture, Ecosystems & Environment 56, 77-92.
| Crossref | Google Scholar |
Azevêdo JAG, Valadares Filho SdC, Pina DdS, Chizzotti ML, Valadares RFD (2010) A meta-analysis of dry matter intake in Nellore and Zebu-crosses cattle. Revista Brasileira de Zootecnia 39, 1801-1809.
| Crossref | Google Scholar |
Bailey DW, VanWagoner HC, Weinmeister R (2006) Individual animal selection has the potential to improve uniformity of grazing on foothill rangeland. Rangeland Ecology & Management 59, 351-358.
| Crossref | Google Scholar |
Batista ED, Detmann E, Gomes DI, Rufino LMA, Paulino MF, Valadares Filho SC, Franco MO, Sampaio CB, Reis WLS (2017) Effect of protein supplementation in the rumen, abomasum, or both on intake, digestibility, and nitrogen utilisation in cattle fed high-quality tropical forage. Animal Production Science 57, 1993-2000.
| Crossref | Google Scholar |
Bell A, Sangster N (2023) Research, development and adoption for the north Australian beef cattle breeding industry: an analysis of needs and gaps. Animal Production Science 63, 1-40.
| Crossref | Google Scholar |
Benvenutti MA, Gordon IJ, Poppi DP, Crowther R, Spinks W, Moreno FC (2009) The horizontal barrier effect of stems on the foraging behaviour of cattle grazing five tropical grasses. Livestock Science 126, 229-238.
| Crossref | Google Scholar |
Benvenutti MA, Pavetti DR, Poppi DP, Gordon IJ, Cangiano CA (2016) Defoliation patterns and their implications for the management of vegetative tropical pastures to control intake and diet quality by cattle. Grass and Forage Science 71, 424-436.
| Crossref | Google Scholar |
Berckmans D (2014) Precision livestock farming technologies for welfare management in intensive livestock systems. Revue Scientifique et Technique – Office International des Epizooties 33, 189-196.
| Crossref | Google Scholar |
Berge P (1991) Long-term effects of feeding during calfhood on subsequent performance in beef cattle (a review). Livestock Production Science 28, 179-201.
| Crossref | Google Scholar |
Blaxter KL, Wainman FW, Wilson RS (1961) The regulation of food intake by sheep. Animal Production 3, 51-61.
| Crossref | Google Scholar |
Boval M, Dixon RM (2012) The importance of grasslands for animal production and other functions: a review on management and methodological progress in the tropics. Animal 6, 748-762.
| Crossref | Google Scholar |
Boval M, Fanchone A, Archimède H, Gibb MJ (2007) Effect of structure of a tropical pasture on ingestive behaviour, digestibility of diet and daily intake by grazing cattle. Grass and Forage Science 62, 44-54.
| Crossref | Google Scholar |
Bray S, Doran-Browne N, O’Reagain P (2014) Northern Australian pasture and beef systems. 1. Net carbon position. Animal Production Science 54, 1988-1994.
| Crossref | Google Scholar |
Cadenas-Soberanis A, Jiménez-Ocampo R, Arceo-Castillo JI, López-Zapata CP, Aguilar-Pérez CF, Ku-Vera JC (2021) Net energy requirement for maintenance of crossbred beef heifers (Bos taurus × Bos indicus) as measured with the washed-rumen technique and indirect calorimetry. Livestock Science 251, 104612.
| Crossref | Google Scholar |
Cantalapiedra-Hijar G, Abo-Ismail M, Carstens GE, Guan LL, Hegarty R, Kenny DA, McGee M, Plastow G, Relling A, Ortigues-Marty I (2018) Review: Biological determinants of between-animal variation in feed efficiency of growing beef cattle. Animal 12(S2), s321-s335.
| Crossref | Google Scholar |
Chacon E, Stobbs TH (1976) Influence of progressive defoliation of a grass sward on the eating behaviour of cattle. Australian Journal of Agricultural Research 27, 709-727.
| Crossref | Google Scholar |
Charmley E, Williams SRO, Moate PJ, Hegarty RS, Herd RM, Oddy VH, Reyenga P, Staunton KM, Anderson A, Hannah MC (2016) A universal equation to predict methane production of forage-fed cattle in Australia. Animal Production Science 56, 169-180.
| Crossref | Google Scholar |
Chilcott C, Ash A, Lehnert S, Stokes C, Charmley E, Collins K, Pavey C, Macintosh A, Simpson A, Berglas R, White E, Amity M (2020) Northern Australia beef situation analysis. A report to the Cooperative Research Centre for Developing Northern Australia. CRC for Developing Northern Australia, Townsville, Qld, Australia.
Chizzotti ML, Valadares Filho SC, Tedeschi LO, Chizzotti FHM, Carstens GE (2007) Energy and protein requirements for growth and maintenance of F1 Nellore × Red Angus bulls, steers, and heifers. Journal of Animal Science 85, 1971-1981.
| Crossref | Google Scholar |
Coates DB, Dixon RM (2011) Developing robust faecal near infrared spectroscopy calibrations to predict diet dry matter digestibility in cattle consuming tropical forages. Journal of Near Infrared Spectroscopy 19, 507-519.
| Crossref | Google Scholar |
Coleman SW (2005) Predicting forage intake by grazing ruminants. Paper presented at ‘Florida ruminant symposium’ In pp. 72-90.
| Google Scholar |
Coleman SW, Gunter SA, Sprinkle JE, Neel JPS (2014) Beef Species Symposium: Difficulties associated with predicting forage intake by grazing beef cows. Journal of Animal Science 92, 2775-2784.
| Crossref | Google Scholar |
Da Silva SC, Gimenes FMA, Sarmento DOL, Sbrissia AF, Oliveira DE, Hernadez-Garay A, Pires AV (2013) Grazing behaviour, herbage intake and animal performance of beef cattle heifers on marandu palisade grass subjected to intensities of continuous stocking management. The Journal of Agricultural Science 151, 727-739.
| Crossref | Google Scholar |
Decruyenaere V, Buldgen A, Stilmant D (2009) Factors affecting intake by grazing ruminants and related quantification methods: a review. Biotechnology Agronomy Society Environment 13, 559-573.
| Google Scholar |
Dove H, Mayes RW (1991) The use of plant wax alkanes as marker substances in studies of the nutrition of herbivores: a review. Australian Journal of Agricultural Research 42, 913-952.
| Crossref | Google Scholar |
Fernandes GA, de Oliveira AS, de Araujo CV, Couto VRM, de Moraes KAK, de Moraes EHBK (2022) Prediction of pasture intake by beef cattle in tropical conditions. Tropical Animal Health and Production 54, 13.
| Crossref | Google Scholar |
Forbes JM (2003) The multifactorial nature of food intake control. Journal of Animal Science 81, E139-E144.
| Google Scholar |
Frank ASK, Dickman CR, Wardle GM (2012) Habitat use and behaviour of cattle in a heterogeneous desert environment in central Australia. The Rangeland Journal 34, 319-328.
| Crossref | Google Scholar |
Freer M, Jones DB (1984) Feeding value of subterranean clover, lucerne, phalaris and Wimmera ryegrass for lambs. Australian Journal of Experimental Agriculture and Animal Husbandry 24, 156-164.
| Crossref | Google Scholar |
Fritz H, Garine-Wichatitsky M (1996) Foraging in a social antelope: effects of group size on foraging choices and resource perception in impala. Journal of Animal Ecology 65, 736-742.
| Crossref | Google Scholar |
Greenwood PL, Bell AW (2014) Consequences of nutrition during gestation, and the challenge to better understand and enhance livestock productivity and efficiency in pastoral ecosystems. Animal Production Science 54, 1109-1118.
| Crossref | Google Scholar |
Greenwood PL, Valencia P, Overs L, Paull DR, Purvis IW (2014) New ways of measuring intake, efficiency and behaviour of grazing livestock. Animal Production Science 54, 1796-1804.
| Crossref | Google Scholar |
Greenwood PL, Paull DR, McNally J, Kalinowski T, Ebert D, Little B, Smith DV, Rahman A, Valencia P, Ingham AB, Bishop-Hurley GJ (2017) Use of sensor-determined behaviours to develop algorithms for pasture intake by individual grazing cattle. Crop & Pasture Science 68, 1091-1099.
| Crossref | Google Scholar |
Gregorini P, Gunter SA, Bowman MT, Caldwell JD, Masino CA, Coblentz WK, Beck PA (2011) Effect of herbage depletion on short-term foraging dynamics and diet quality of steers grazing wheat pastures. Journal of Animal Science 89, 3824-3830.
| Crossref | Google Scholar |
Hammond K (2006) Breeding strategies for the development of the Australian beef industry: an overview. Australian Journal of Experimental Agriculture 46, 183-198.
| Crossref | Google Scholar |
Harrison MT, McSweeney C, Tomkins NW, Eckard RJ (2015) Improving greenhouse gas emissions intensities of subtropical and tropical beef farming systems using Leucaena leucocephala. Agricultural Systems 136, 138-146.
| Crossref | Google Scholar |
Hayes BJ, Lewin HA, Goddard ME (2013) The future of livestock breeding: genomic selection for efficiency, reduced emissions intensity, and adaptation. Trends in Genetics 29, 206-214.
| Crossref | Google Scholar |
Hendricksen RE, Poppi DP, Minson DJ (1981) The voluntary intake, digestibility and retention time by cattle and sheep of stem and leaf fractions of a tropical legume (Lablab purpureus). Australian Journal of Agricultural Research 32, 389-398.
| Crossref | Google Scholar |
Herd RM, Arthur PF (2009) Physiological basis for residual feed intake. Journal of Animal Science 87, E64-E71.
| Crossref | Google Scholar |
Herd RM, Hegarty RS, Dicker RW, Archer JA, Arthur PF (2002) Selection for residual feed intake improves feed conversion ratio on pasture. Animal Production in Australia 24, 85-88.
| Google Scholar |
Hodgson J, Capriles JMR, Fenlon JS (1977) The influence of sward characteristics on the herbage intake of grazing calves. The Journal of Agricultural Science 89, 743-750.
| Crossref | Google Scholar |
Hu S, Arablouei R, Bishop-Hurley G, Reverter A, Ingham A Predicting bite rate of grazing cattle from accelerometry data via semi-supervised regression. Social Science Research Network in press.
| Crossref | Google Scholar |
Hunt LP, Petty S, Cowley R, Fisher A, Ash AJ, MacDonald N (2007) Factors affecting the management of cattle grazing distribution in northern Australia: preliminary observations on the effect of paddock size and water points. The Rangeland Journal 29, 169-179.
| Crossref | Google Scholar |
Hunter RA, Siebert BD (1985) Utilization of low-quality roughage by Bos taurus and Bos Indicus cattle 2. The effect of rumen-degradable nitrogen and sulphur on voluntary food intake and rumen characteristics. British Journal of Nutrition 53, 649-656.
| Crossref | Google Scholar |
Hunter RA, Siebert BD (1987) The effect of supplements of rumen-degradable protein and formaldehyde-treated casein on the intake of low-nitrogen roughages by Bos taurus and Bos indicus steers at different stages of maturity. Australian Journal of Agricultural Research 38, 209-218.
| Crossref | Google Scholar |
Illius AW, Gordon IJ (1991) Prediction of intake and digestion in ruminants by a model of rumen kinetics integrating animal size and plant characteristics. The Journal of Agricultural Science 116, 145-157.
| Crossref | Google Scholar |
Kennedy PM, Charmley E (2012) Methane yields from Brahman cattle fed tropical grasses and legumes. Animal Production Science 52, 225-239.
| Crossref | Google Scholar |
Kenny DA, Fitzsimons C, Waters SM, McGee M (2018) Invited review: Improving feed efficiency of beef cattle – the current state of the art and future challenges. Animal 12, 1815-1826.
| Crossref | Google Scholar |
Kloppenburg PB, Kiesling HE, Kirksey RE, Donart GB (1995) Farage quality, intake, and digestibility of year-long pastures for steers. Journal of Range Management 48, 542-548.
| Crossref | Google Scholar |
Krizsan SJ, Ahvenjärvi S, Huhtanen P (2010) A meta-analysis of passage rate estimated by rumen evacuation with cattle and evaluation of passage rate prediction models. Journal of Dairy Science 93, 5890-5901.
| Crossref | Google Scholar |
Krueger NA, Adesogan AT, Staples CR, Krueger WK, Kim SC, Littell RC, Sollenberger LE (2008) Effect of method of applying fibrolytic enzymes or ammonia to Bermudagrass hay on feed intake, digestion, and growth of beef steers. Journal of Animal Science 86, 882-889.
| Crossref | Google Scholar |
Kutt AS, Vanderduys EP, O’Reagain P (2012) Spatial and temporal effects of grazing management and rainfall on the vertebrate fauna of a tropical savanna. The Rangeland Journal 34, 173-182.
| Crossref | Google Scholar |
Lawrence P, Kenny DA, Earley B, McGee M (2013) Intake of conserved and grazed grass and performance traits in beef suckler cows differing in phenotypic residual feed intake. Livestock Science 152, 154-166.
| Crossref | Google Scholar |
Lazzarini I, Detmann E, Sampaio CB, Paulino MF, Valadares Filho SC, Souza MA, Oliveira FA (2009) Transit and degradation dynamics of neutral detergent fiber in cattle fed low-quality tropical forage and nitrogenous compounds. Arquivo Brasileiro de Medicina Veterinária e Zootecnia 61, 635-647.
| Crossref | Google Scholar |
MacArthur RH, Pianka ER (1966) On optimal use of a patchy environment. The American Naturalist 100, 603-609.
| Crossref | Google Scholar |
Madruga A, Mainau E, González LA, Rodríguez-Prado M, Ruíz de la Torre JL, Manteca X, Ferret A (2017) Effect of forage source included in total mixed ration on intake, sorting and feeding behavior of growing heifers fed high-concentrate diets. Journal of Animal Science 95, 3322-3330.
| Crossref | Google Scholar |
Manthey M, Peper J (2010) Estimation of grazing intensity along grazing gradients – the bias of nonlinearity. Journal of Arid Environments 74, 1351-1354.
| Crossref | Google Scholar |
McGavin SL, Bishop-Hurley GJ, Charmley E, Greenwood PL, Callaghan MJ (2018) Effect of GPS sample interval and paddock size on estimates of distance travelled by grazing cattle in rangeland, Australia. The Rangeland Journal 40, 55-64.
| Crossref | Google Scholar |
McLennan SR, Bolam MJ, Kidd JF, Chandra KA, Poppi DP (2017) Responses to various protein and energy supplements by steers fed low-quality tropical hay. 1. Comparison of response surfaces for young steers. Animal Production Science 57, 473-488.
| Crossref | Google Scholar |
McLennan S, McLean I, Paton C (2020) Re-defining the animal unit equivalence (AE) for grazing ruminants and its application for determining forage intake, with particular relevance to the northern Australian grazing industries. Project B.GBP.0036 Final Report. Meat & Livestock Australia, Sydney, NSW, Australia.
Mertens DR (1987) Predicting intake and digestibility using mathematical models of ruminal function. Journal of Animal Science 64, 1548-1558.
| Crossref | Google Scholar |
Minson DJ, McDonald CK (1987) Estimating forge intake from the growth of beef cattle. Tropical Grasslands 21, 116-122.
| Google Scholar |
Mota VAC, Fernandes RM, Prados LF, Alves Neto JA, Berti GF, Resende FD, Siqueira GR (2020) Relationship between gain rate during the growing phase and forage allowance in the finishing phase in Nellore cattle. Tropical Animal Health and Production 52, 1881-1891.
| Crossref | Google Scholar |
NASEM (2016) ‘Nutrient requirements of beef cattle.’ 8th revised edn. (National Academies Press: Washington, DC, USA) doi:10.17226/19014
Oliveira LF, Ruggieri AC, Branco RH, Cota OL, Canesin RC, Costa HJU, Mercadante MEZ (2018) Feed efficiency and enteric methane production of Nellore cattle in the feedlot and on pasture. Animal Production Science 58, 886-893.
| Crossref | Google Scholar |
Owen-Smith N, Fryxell JM, Merrill EH (2010) Foraging theory upscaled: the behavioural ecology of herbivore movement. Philosophical Transactions of the Royal Society B: Biological Sciences 365, 2267-2278.
| Crossref | Google Scholar |
O’Reagain PJ, Scanlan JC (2013) Sustainable management for rangelands in a variable climate: evidence and insights from northern Australia. Animal 7, 68-78.
| Crossref | Google Scholar |
Panjaitan T, Quigley SP, McLennan SR, Swain T, Poppi DP (2010) Intake, retention time in the rumen and microbial protein production of Bos indicus steers consuming grasses varying in crude protein content. Animal Production Science 50, 444-448.
| Crossref | Google Scholar |
Poppi DP (1996) Predictions of food intake in ruminants from analyses of food composition. Australian Journal of Agricultural Research 47, 489-504.
| Crossref | Google Scholar |
Poppi DP, McLennan SR (1995) Protein and energy utilization by ruminants at pasture. Journal of Animal Science 73, 278-290.
| Crossref | Google Scholar |
Poppi DP, McLennan SR (2010) Nutritional research to meet future challenges. Animal Production Science 50, 329-338.
| Crossref | Google Scholar |
Poppi DP, Minson DJ, Ternouth JH (1981) Studies of cattle and sheep eating leaf and stem fractions of grasses. 2. Factors controlling the retention of feed in the reticulo-rumen. Australian Journal of Agricultural Research 32, 109-121.
| Crossref | Google Scholar |
Roguet C, Dumont B, Prache S (1998) Selection and use of feeding sites and feeding stations by herbivores: a review. Annales de Zootechnie 47, 225-244.
| Crossref | Google Scholar |
Sales J (2012) A review on the use of indigestible dietary markers to determine total tract apparent digestibility of nutrients in horses. Animal Feed Science and Technology 174, 119-130.
| Crossref | Google Scholar |
Siebert BD, Hunter RA (1977) Prediction of herbage intake and liveweight gain of cattle grazing tropical pastures from the composition of the diet. Agricultural Systems 2, 199-208.
| Crossref | Google Scholar |
Silva TACC, Quigley SP, Kidd LJ, Anderson ST, McLennan SR, Schatz TJ, McCosker KD, Poppi DP (2022) Growth and reproductive performance responses to post-weaning supplementation of early and normally-weaned Brahman crossbred heifers raised in tropical rangelands. PLoS ONE 17, e0263786.
| Crossref | Google Scholar |
Smith D, Rahman A, Bishop-Hurley GJ, Hills J, Shahriar S, Henry D, Rawnsley R (2016) Behavior classification of cows fitted with motion collars: decomposing multi-class classification into a set of binary problems. Computers and Electronics in Agriculture 131, 40-50.
| Crossref | Google Scholar |
Smith WB, Galyean ML, Kallenbach RL, Greenwood PL, Scholljegerdes EJ (2021) Understanding intake on pastures: how, why, and a way forward. Journal of Animal Science 99(6), skab062.
| Crossref | Google Scholar |
Stifkens A, Matthews EM, McSweeney CS, Charmley E (2022) Increasing the proportion of Leucaena leucocephala in hay-fed beef steers reduces methane yield. Animal Production Science 62, 622-632.
| Crossref | Google Scholar |
Stobbs TH (1970) Automatic measurement of grazing time by dairy cows on tropical grasses and legume pastures. Tropical Grasslands 4, 237-244.
| Google Scholar |
Thomas DT, Milton JTB, Revell CK, Ewing MA, Dynes RA, Murray K, Lindsay DR (2010) Preference of sheep among annual legumes is more closely related to plant nutritive characteristics as plants mature. Animal Production Science 50, 114-123.
| Crossref | Google Scholar |
Thornton RF, Minson DJ (1973) The relationship between apparent retention time in the rumen, voluntary intake, and apparent digestibility of legume and grass diets in sheep. Australian Journal of Agricultural Research 24, 889-898.
| Crossref | Google Scholar |
Tomkins N, O’Reagain P (2007) Global positioning systems indicate landscape preferences of cattle in the subtropical savannas. The Rangeland Journal 29, 217-222.
| Crossref | Google Scholar |
Tomkins NW, Harper GS, Bruce HL, Hunter RA (2006) Effect of different post-weaning growth paths on long-term weight gain, carcass characteristics and eating quality of beef cattle. Australian Journal of Experimental Agriculture 46, 1571-1578.
| Crossref | Google Scholar |
Topps JH (1969) Nutritional problems of livestock production from semi-arid grasslands in the tropics. Grass and Forage Science 24, 250-257.
| Crossref | Google Scholar |
Varva M, Ganskopp D (1998) Grazing behaviour in ungulates: current concepts and future challenges. Annals of the Arid Zone 37, 319-335.
| Google Scholar |
Velazco JI, Herd RM, Cottle DJ, Hegarty RS (2017) Daily methane emissions and emission intensity of grazing beef cattle genetically divergent for residual feed intake. Animal Production Science 57, 627-635.
| Crossref | Google Scholar |
Vercoe JE (1970) The fasting metabolism of Brahman, Africander and Hereford × Shorthorn cattle. British Journal of Nutrition 24, 599-606.
| Crossref | Google Scholar |
Webster J (2013) Audits of animals in agriculture. In ‘Animal husbandry regained: the place of farm animals in sustainable agriculture’. (Eds J Webster, J Porritt) pp. 41–67. (Routledge) doi:10.4324/9780203094228-13