The role of people, parks and precipitation on the frequency and timing of fires in a sub-Saharan savanna ecosystem
Julius R. Dewald A * , Jane Southworth B and Imelda K. Moise CA
B
C
Abstract
The Lupande Game Management Area (GMA) and the adjacent South Luangwa National Park (NP) in Zambia allow comparison of fire regimes in African savannas with different human densities.
To investigate humans’ effects on fire regimes within a sub-Saharan savanna ecosystem.
We delineated burned areas for the Lupande GMA and South Luangwa NP using 156 Landsat images from 1989 to 2017. We performed comparisons of fire regimes between the Lupande GMA and South Luangwa NP using various burned area variables and assessed their association with precipitation.
Overall, and compared with the South Luangwa NP, the Lupande GMA had a greater extent of burned area and a higher frequency of repeat burns. The Lupande GMA experienced fires earlier in the fire season, which are typically less damaging to woody vegetation. We observed a significant positive relationship between precipitation and burned area trends in South Luangwa NP but not in the Lupande GMA, suggesting that precipitation increases burned area in South Luangwa NP.
Results support the theory that human fire management mitigates climate’s effect, particularly rainfall, on interannual burned area variation.
This study shows that human-dominated fire regimes in savannas can alter the influence of precipitation.
Keywords: burnt area, fire management, Game Management Area, human land use, Lupande, rainfall, South Luangwa, Zambia.
Introduction
Fires play an integral role in determining the composition and structure of African savannas (Govender et al. 2006; Lehmann et al. 2009). Without disturbances such as fire, many savannas experiencing annual rainfall amounts over 650–700 mm are likely to develop into closed woodlands (Andersen et al. 2003; Bond et al. 2005; Sankaran et al. 2005; Govender et al. 2006; Staver et al. 2011). How fires affect the woody vegetation of savannas is contingent on many variables such as fire frequency, fire severity, fuel loads, seasonality and climate (Bond et al. 2005; Werf et al. 2008; Grégoire et al. 2013; Andela and van der Werf 2014). With the human population increasing in many African savannas, it is also important to note that humans are a critical component of fire regimes, with human activities producing most ignitions in African savanna fires (Archibald et al. 2009; Bowman et al. 2011). Little research has focused on teasing apart the human from natural effects on fire regimes in Africa in response to human population growth. Research that has been performed uses low-resolution (500 m) satellite data (Archibald et al. 2009). Understanding the relationship between fire regimes and human habitation in African savannas would benefit from more spatially focused, higher-resolution studies.
The two main factors impacting how fires in savanna ecosystems affect woody plants are frequency and fire severity, and these two factors are negatively correlated with each other (Higgins et al. 2000; Midgley et al. 2010; Smit et al. 2010; Giddey et al. 2022). Frequent fires typically reduce tree cover, whereas infrequent fires promote tree cover (Trollope and Tainton 1986; Bond et al. 2003; Hoffmann and Solbrig 2003; van Langevelde et al. 2003; Bond et al. 2005; Archibald et al. 2010; Staver et al. 2011; Daskin et al. 2016). Concerning fire severity, more severe fires can force resprouting at the base of small trees, reducing overall aboveground woody biomass compared with fire exclusion sites (Trapnell 1959; Hodgkinson 1998; Higgins et al. 2000; Govender et al. 2006). The present study uses the term fire severity following the definition proposed by Keeley (2009), which states that fire severity refers to the loss of or change in organic matter above ground and below ground as a result of a fire, although the precise metric varies with management needs. When tree canopy cover exceeds 40%, a feedback effect occurs, signalling a tipping point between two stable states of forest and fire-maintained grasslands (Bond et al. 2005; Archibald et al. 2009). To comprehensively understand the trajectory of a savanna’s vegetative composition necessitates an understanding of various factors, including but not limited to current fire severity and frequency.
The timing of fires in savanna ecosystems is a multifaceted process influenced by a range of interconnected factors, including climatic conditions, seasonal changes, winds, leaf drop and other variables that can vary both gradually and suddenly depending on the specific characteristics of the savanna in question. Traditionally, it has been suggested that late dry season fires tend to be more intense owing to drier conditions and larger grass fuel loads, whereas early dry season fires are considered less intense (Brockett et al. 2001; Bond et al. 2005; Govender et al. 2006; Smit et al. 2016). However, recent research, such as the work by Laris et al. (2017) has challenged the binary classification of fire seasons, demonstrating that fire temperature and burn completeness can be highest during the middle of the season, blurring the distinction between early and late fire periods. Furthermore, the factors contributing to tree die-back in relation to fire, whether fire severity, the vulnerability of trees at the time of later fires (e.g. low moisture content or releafing) or other variables, remain areas of ongoing investigation. This highlights the complexity and variability of fire season dynamics in savannas, and the need for a more nuanced and region-specific understanding of these processes.
Previous research found that the relationship between available moisture and fire activity is highly variable and ecosystem-dependent (Abatzoglou et al. 2018), with continent-scale fire–climate models showing rainfall variability explaining interannual variability in fire activity north of the equator but failing to explain any variability of burned areas in Africa’s southern hemisphere (Andela and van der Werf 2014; Zubkova et al. 2019). It is important to mention that on smaller scales, rainfall variability has less effect on fire activity in mesic savannas than on xeric savannas (Karp et al. 2023). However, moisture conditions before a fire season in the drier savannas of southern Africa can promote biomass productivity and increase flammable fuel loads in the following year (Archibald et al. 2009). To determine the degree to which climate influences fire regimes can be difficult as humans are also a significant influence on fire activity in Africa. As a result, it can be challenging to decouple the effects of these two drivers from each other (Bowman et al. 2011; Zubkova et al. 2019).
Certain human activities such as land-use modification, fire ignitions and fire suppression can dampen or magnify the effects of climate on fire (Archibald et al. 2009; Grégoire et al. 2013; Andela and van der Werf 2014; Laris et al. 2015; Abatzoglou et al. 2018). As human density increases in the savanna regions of Africa, fires are expected to decrease in burnt area and fire activity owing to fuel load becoming more fragmented (Archibald et al. 2009). The most important human factors influencing burnt area size are road density, grazing, the fraction of transformed land (rural/urban) and population density (Archibald et al. 2009). Previous research using the Moderate Resolution Imaging Spectroradiometer (MODIS) burned area data has shown a decline in fire activity in southern Africa’s savannas with increased human density or savanna-to-cropland conversion (Archibald et al. 2009; Grégoire et al. 2013). However, using the low-resolution MODIS burned area product (MCD64A1) to measure trends in burned area has limitations. The MODIS product fails to capture most burn scars less than 100 ha, resulting in underestimates in regions with small fires dominating the total burned area, such as in areas of human habitation (Liu et al. 2018; Boschetti et al. 2019; Roteta et al. 2019).
This study examines the fire regimes of two protected areas in Zambia: the uninhabited South Luangwa National Park (South Luangwa NP) and the Lupande Game Management Area (Lupande GMA). Additionally, it models how climatic variables may be influencing trends in burned areas within these regions. These sites present an ideal study location to investigate how different human densities and fire management strategies impact fire regimes in African savannas. South Luangwa NP, being uninhabited with no established fire management program, offers a contrasting scenario, while the Lupande GMA has a growing human population that follows local fire practices. Specifically, we anticipate that in the Lupande GMA, individual burned areas will decrease in size, and fires will occur earlier in the fire season (Laris et al. 2018). Despite these differences, these protected areas are adjacent, located in the Luangwa Valley, and contain similar ecological and climatic conditions as indicated by the General Management Plans for both study sites created by the Department of National Parks and Wildlife of Zambia (DNPW 2011a, 2011b). To ensure a valid comparison, the present study compares locations with similar characteristics between the two sites, including elevation, slope and distance from roads, to minimise confounding variables. The annual rainfall in the Luangwa Valley ranges from 700 mm in the south to 900 mm in the north (Astle 1999; Shrader et al. 2010). This transitional precipitation zone represents a shift from grass-dominated (<700 mm) savannas to tree-dominated (>900 mm) savannas, as determined by previous research in the region. Within this transitional zone, the influence of fire, soil moisture, precipitation and other environmental variables begins to shift towards disturbance-dependent tree-dominated savannas (Campo-Bescós et al. 2013). By utilising 29 years (1989–2018) of 30-m resolution Landsat satellite imagery and climate data, the present study aims to achieve two main objectives:
To investigate and decouple the impacts of climate variability and human population growth on historical changes in fire regimes within South Luangwa NP and Lupande GMA; and
To examine how the unique rainfall zone, around the cusp of 750–900 mm, impacts the relationship between fire regimes and different levels of human densities.
Data and methods
As stated, this study focuses on South Luangwa NP and the Lupande GMA, which are situated in the Luangwa Valley of Zambia (see Fig. 1). South Luangwa NP (9050 km2) does not allow permanent human settlement within its borders and is a protected area where biodiversity and ecosystem processes flourish. South Luangwa NP is renowned for its abundance and variety of wildlife and attracts approximately 10 000 tourists annually (Mvula 2001). The Lupande GMA (4725 km2) flanks the eastern edge of South Luangwa NP, functions as a buffer zone and encourages the sustainable use of wildlife through trophy hunting and photo tourism to generate money for local communities (Astle 1999; Watson et al. 2015). As of the 2010 Zambian census, the Lupande GMA has a total population of 45 000 (Nyirenda et al. 2010). Within the Lupande GMA, human settlement is concentrated mainly along major tributaries and roads (Watson et al. 2015). Previous research has demonstrated that the rural farmers in Lupande GMA use fires in the early dry season to encourage vegetation regeneration for pasture, create firebreaks, prevent destructive late dry season fires, and kill pests and weeds (Eriksen 2007; Hollingsworth et al. 2015). In the late dry season, people use fires to clear the bush, clear and fertilise arable fields, collect honey, facilitate hunting and produce new animal pastures (Eriksen 2007; Hollingsworth et al. 2015). However, the Lupande GMA experienced rapid growth in the human population within recent decades (69% between 2000 and 2010) (Kakumbi 2011). The effect this growth may have on the fire regimes of the Lupande GMA and the adjacent South Luangwa NP is unclear. However, previous studies in other regions would lead us to expect a decline in area burned with increasing agriculture from population growth (Archibald et al. 2010; Laris et al. 2018).
Landsat surface reflectance data with Worldwide Reference System (WRS)-2 coordinates (Path 170, Row 69) obtained between 1989 and 2017 were utilised in this research. In addition, selected images with less than 30% cloud cover were used, along with other satellite imagery sources (see Table 1). Landsat 7 was excluded from the analysis owing to scan line error. The sparsity of imagery between 2002 and 2013 due to this exclusion of any Landsat 7 data makes it challenging to compile complete fire season burned areas for these years. A total of 156 images were used, including 100 Landsat 5 Thematic Mapper (TM) images and 56 Landsat 8 Operational Land Imager (OLI) images. The quality assessment band (QA-band) provided with each Landsat image was used to delete pixels containing clouds and shadows from the data. Each pixel in the QA-band contains a decimal value representing surface, atmosphere and sensor conditions that can affect the overall usefulness of a given pixel. Pixels shown to contain cloud and cloud shadows were used to identify and remove these sources of error from the analysis. Additionally, areas of permanent water were removed using the Global Surface Water dataset from the European Commission and manual delineation (Pekel et al. 2016). Lastly, all possible hill-shaded areas falsely detected as burned areas were omitted from further analysis through the use of the hill-shade tool in ArcMap and elevation data from a 30-m digital elevation model (DEM) compiled from the Shuttle Radar Topographic Mission (SRTM) obtained from US Geological Survey (GS) Earth Explorer website in February 2019. To determine the maximum possible area affected by shade, the sun angle and azimuth used in calculating possible hill shade used the conditions found during the winter solstice of Zambia, which was 20 June at 10 a.m.
Four SPOT (Satellite Pour l’Observation de la Terre) satellite images (SPOT 5: 2.5 m pan-sharpened, SPOT 6 and 7: 1.5 m) that coincided with available Landsat images were acquired for determining the appropriate burned area index and optimal threshold. SPOT images were used as they were the highest-resolution imagery available for the area across this time period and had the greatest temporal resolution. The locations and dates of these SPOT images were stratified to see how year and location affect burned area delineation accuracy. The time of capture for the SPOT images was stratified across the fire season to ascertain how the time of year affects fire delineation (Fig. 2). The image taken in 2014 was taken during the end of the dry season in South Luangwa NP. The image of 2016 was located at the intersection of South Luangwa NP and the Lupande GMA taken during the beginning of the dry season. The image of 2018 was taken during the middle of the dry season and located in the Lupande GMA. The last SPOT image was taken on 12 July 2006, for determining a new threshold for Landsat 5 imagery owing to the differences in wavelength designations between Landsat 5 and 8.
(a) SPOT imagery extents for the years of 2018, 2016, 2014 and 2006 used for accuracy assessment with the Landsat imagery. The position and placement of the SPOT imagery extents are a result of combination of imagery availability and the aim to encompass representative areas within protected regions. The different years for each SPOT image were used to test threshold values for different points in the dry season and for the burn indices derived from the different Landsat satellites. (b) Display of the manually delineated areas within the 2006 SPOT image that were used for accuracy assessment analysis.
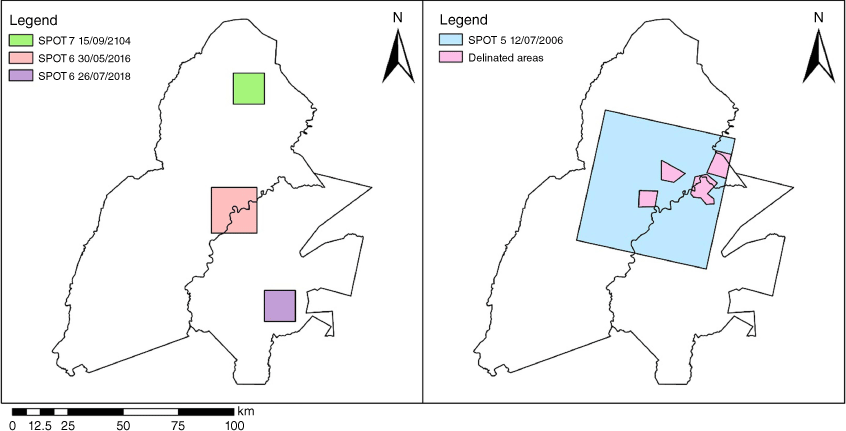
Burned area delineation using observed burned areas relied on the visual interpretation of the SPOT imagery, as there are no reference data for burned areas in the Luangwa valley. Despite the potential to introduce errors of commission or omission, visual interpretation is commonly used in remote sensing studies to identify burn scars (Bastarrika et al. 2011; Liu et al. 2018). The Landsat images that temporally corresponded with the SPOT images were converted into three indices: the Burned Area Index (BAI) (Chuvieco and Martín 1998), the Normalized Burned Ratio 2 (NBR) (García and Caselles 1991), and the Mid-Infrared Burned Index (MIRBI) (Trigg and Flasse 2001) (Table 2). Each of these indices has been used in remote sensing studies of burned area, providing accurate results (Chuvieco et al. 2002; Veraverbeke et al. 2010; Yang et al. 2013; Vargas-Sanabria and Campos-Vargas 2020; van Gerrevink and Veraverbeke 2021; Wall et al. 2021; Alcaras et al. 2022; Wu et al. 2022).
Spectral index | Equation | |
---|---|---|
Burned Area Index | ||
Mid-Infrared Burned Index | ||
Normalised Burned Ratio 2 |
NIR, near-infrared band of the Landsat imagery (0.77–0.90 µm Landsat 5) (0.845–0.885 µm Landsat 8);
Red, red band of the Landsat imagery (0.63–0.69 µm Landsat 5) (0.630–0.680 µm Landsat 8);
SSWIR, shorter short-wave infrared (1.55–1.75 µm Landsat 5) (1.56–1.66 µm Landsat 8);
LSWIR, longer short-wave infrared (2.09–2.35 µm Landsat 5) (2.10–2.30 µm Landsat 8).
Approximately one point per 2 km2 was randomly generated within each SPOT image. These points were used as accuracy assessment locations (whether the ground at a point appears burnt within the SPOT image). The threshold value of a tested index was then compared with these points, with the overall accuracy recorded using an error matrix. This accuracy assessment process was iteratively repeated across the three different burned area indices, varying the threshold with each iteration. BAI started at a threshold value of 10 and increased by a factor of 10 until the threshold value of 100. MIRBI started at the value of 1 and increased by a factor of 0.1 for 10 intervals. NBR started at 0.1 and increased by a factor of 0.03 for 10 intervals. The maximum value used for each index represents an approximate 95% quantile value for the 2016 image. Owing to the differences in burned areas between the 2006 SPOT image and the corresponding Landsat image, a manual delineation of sections with limited burned area disparities was used for threshold assessment (Fig. 2). A flow chart of the burned area delineation methods can be seen in Fig. 3. Briefly, Landsat images were converted to various indices to be compared with SPOT imagery to determine the optimal index and threshold. The final index and threshold were applied to the rest of the Landsat imagery to create a Landsat burned area product.
A flow chart detailing the methods used to delineate burned areas across the compiled Landsat 5 and Landsat 8 images.
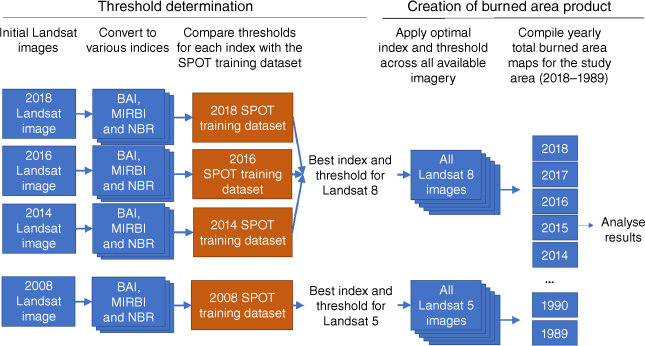
According to the error matrix (Supplementary Table S1), BAI had the highest overall accuracy (percentage correctly classified value) for the study area. Each year, BAI had the highest overall accuracy value of the three indices and across each image. The final threshold used for Landsat 8 imagery was 50, the average of the three thresholds of 2018, 2016 and 2014. Meanwhile, the threshold used for Landsat 5 imagery was 90.
To analyse changes in burn seasonality, the Landsat images were binned into early burn season (May–June), middle burn season (July–August) and late burn season (August–September) for each year within the study consistent with previous studies that stratify the burning season in this manner (Chidumayo 1995; Hollingsworth et al. 2015). Landsat burned area products that fell into one of these bins were consolidated into a single burned area image for that period. Burned areas in previous periods were removed from subsequent burn periods. For instance, an area burned in the early season would not qualify as being burned in the middle or late season.
Propensity score matching (PSM) was used to directly compare the Lupande GMA and South Luangwa NP, which allows the creation of a control group (South Luangwa NP) with similar location characteristics when compared with units from the treated group (Lupande GMA) based on their propensity score. By doing this, PSM attempts to reduce bias from confounding variables that could be affecting the outcomes among treatment and control units (Rosenbaum and Rubin 1983). The propensity score can be interpreted as an index of the characteristics of the unit of analysis weighted by their importance in predicting exposure to confounding variables (Rosenbaum and Rubin 1983; Blackman 2013). Using propensity score matching, selected areas of the Lupande GMA and South Luangwa NP that have similar conditions relating to elevation, slope and distance from roads were averaged on a 3 km grid matrix (Archibald et al. 2009; Estes et al. 2017). The propensity score for each grid cell was calculated and treated units (the Lupande GMA grid cells) were matched to the control units (South Luangwa NP grid cells) using a 1-to-1 nearest neighbour method (see Fig. 4). The matched areas were used to compare the fire regimes within the Lupande GMA and South Luangwa NP, while the unmatched areas were discarded from further analysis. Through the use of the landscape metric measurement software Fragstats, four variables were extracted from the delineated burned areas: (1) percentage of area burned, (2) burn seasonality, (3) burn frequency, and (4) the number of individual fires (McGarigal et al. 2012). Overall fire frequency can be quantified by the fire rotation period (FRP), which is the time needed to burn an area of the same extent (Pereira Júnior et al. 2014).
where N is the number of years in the study period, S is the area susceptible to burn in the study area, A is the annual area burned, and is the sum of A within the period N. The burned areas from each Landsat image were compiled into yearly comprehensive maps to analyse the heterogeneity of fires within both the Lupande GMA and South Luangwa NP. Burned areas that were detected in multiple images within a year were attributed to the earliest image. The number of individual burned areas for each year was counted using the Fragstats software. The propensity score-matched areas were used to compare burn scar heterogeneity between the two study locations (Fig. 4). As the propensity score-matched areas are equal in size, the number of burned areas would also indicate the density of burned areas for each study location. The number of Landsat images used to determine the total burned area for each year varies within the data set. These variations would likely influence a direct comparison of the number of burned areas across time. Therefore, a ratio was created of the number of burned areas in the Lupande GMA versus the number of burned areas of South Luangwa NP for each year and these values were compared across time. This ratio indicates the relationship and differences between the individual burned areas within the Lupande GMA when compared with South Luangwa NP. Lastly, the mean and standard deviation of individual burned area sizes (km2) were also calculated for the Lupande GMA and South Luangwa NP per year.
Following the methodology of Zubkova et al. (2019), the effect climate might have on burned area was calculated by using the Famine Early Warning Systems Network Land Data Assimilation System (FLDAS) data (Zubkova et al. 2019). This dataset contains a monthly series of land surface parameters relating to climate at a resolution of 0.1 × 0.1 degrees, simulated from the Noah 3.6.1 model in the Famine Early Warning Systems (FEWS) network. The FEWS network and FLDAS dataset use rainfall and other meteorological inputs (temperature, humidity, radiation and wind) to produce multi-model and multi-forcing estimates of hydro-climate conditions such as soil moisture, evapotranspiration and runoff (McNally et al. 2017). For the purposes of the present study, the variables used were precipitation rate (kg/m2·s), evapotranspiration (kg/m2·s) and soil moisture (0–10 cm underground) (m3/m3). Precipitation and evapotranspiration values were converted to millimetres per month. A fourth variable, effective rainfall, was calculated by taking the difference between precipitation and evapotranspiration. Only precipitation, effective rainfall and soil moisture were considered for analysis as previous African fire studies found them to be strong drivers of determining burned area (Daniau et al. 2013; Lehmann et al. 2014; McNally et al. 2017; Zubkova et al. 2019). Yearly values for each variable across the two study locations (South Luangwa NP and the Lupande GMA) were calculated by accumulating the monthly averages for each rainy season preceding each fire season. The variability of each variable for each study location can be seen in Fig. 5. For example, the climate variable values for the 2016 burn season used data from July 2015 through June 2016. Preparation of the multilinear models was conducted using R software and ArcGIS v. 10.8 (R CoreTeam 2020).
Variability in climatic conditions across time at the different study locations: (a) precipitation (mm); (b) effective rainfall (mm); and (c) soil moisture (m3/m3).
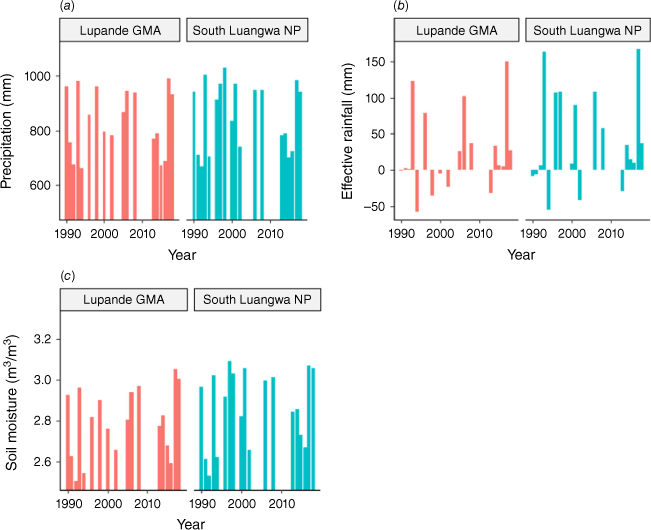
Concurrent and antecedent climate conditions were used within a multilinear model to estimate each variable’s relationship to burned area. This model also log-transformed burned areas, as previous studies have demonstrated that burned areas tend to be right-skewed.
This model was built for each variable in each land system (South Luangwa NP and Lupande GMA). β and β1 are the slopes of the concurrent (C) and antecedent (C1) climate conditions, respectively, and ε represents the error term of the equation. The ratio of the slopes from the predicted and observed burned areas’ linear interpolations were calculated to determine what climate variables can drive the degree of burned area in the two systems. To test for multicollinearity, the Variance Inflation Factor (VIF) for each predictor variable (C and C1) was calculated. This test concluded that all South Luangwa NP and Lupande GMA predictor variables demonstrated no multicollinearity across the various models. Finally, to test for temporal autocorrelation, the Durbin–Watson test was used, which provided no evidence of temporal autocorrelation across the predictor variables in the models (D–W statistic 2.03, P-value 0.986).
To further investigate the relationship of fire and precipitation within the study area, Collection 6 MODIS Global Burned Area Product (MCD64A1) data were obtained to construct a burned area time series for both the Lupande GMA and South Luangwa NP for each month between 2002 and 2018. Monthly average precipitation in millimetres was extracted for both systems across the same time scale using the Climate Hazards Center InfraRed Precipitation with Station (CHIRPS) dataset. The burned area and precipitation variables were de-seasonalised by subtracting the seasonal component from the time series for each system to ensure stationarity and that the less apparent trends were retained rather than the dominant trends associated with seasonal cycles. From there, cross-correlation analysis was conducted to test if the variability in precipitation could affect the burned area in the subsequent fire season.
Results
Burned area trends
Throughout the study’s time span, the analysis using the propensity score-matched areas revealed that 66.15% of the total area of the Lupande GMA burns every year, whereas 31.89% of the area within South Luangwa NP burns. Across the time span of the study, there was no statistically significant change in the total burned area for the Lupande GMA (P = 0.95), whereas South Luangwa NP had a slightly positive trend, although it was not statistically significant (P = 0.21) (Fig. 6). When observing the seasonal differences between the two systems, it is apparent that the Lupande GMA experiences a higher proportion of burning during the early (May-June) and middle dry season (July–August) (Supplementary Fig. S1). On average, 25% of the total burned area occurs during the early dry season, and 51% occurs during the middle dry season. Meanwhile, South Luangwa NP experienced burns later in the dry season, with 49% of the total burned area occurring during the late dry season (September–October) (Table 3). Additionally, Supplementary Fig. S1 visually highlights that fires in South Luangwa NP tend to be larger and less fragmented compared with those in the Lupande GMA.
Percentage of total area burned for each system and each year within the propensity score matched areas. Lines of best fit and 95% confidence intervals (grey areas) are included for each system.
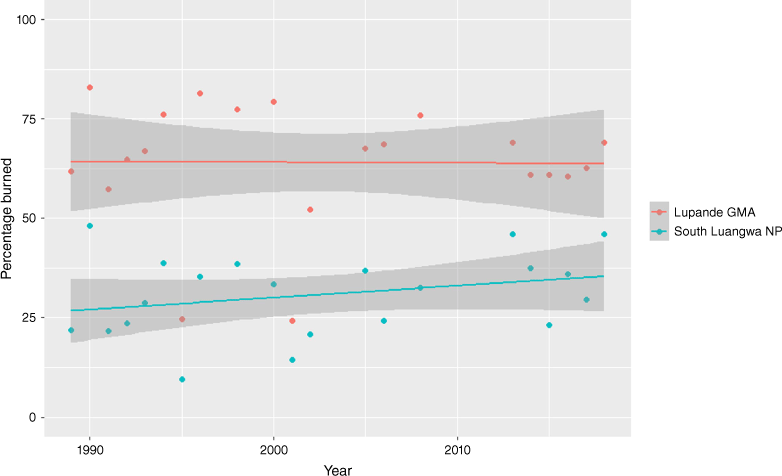
System | Average burned area (%) | s.d. | ||
---|---|---|---|---|
Total burned area | Lupande GMA | 65.16 | 12.89 | |
South Luangwa NP | 31.89 | 9.67 | ||
Early dry season (May–June) A | Lupande GMA | 25.23 | 20.39 | |
South Luangwa NP | 11.98 | 8.57 | ||
Middle dry season (July–August) A | Lupande GMA | 51.13 | 17.60 | |
South Luangwa NP | 38.95 | 21.04 | ||
Late dry season (September–October) A | Lupande GMA | 23.64 | 16.13 | |
South Luangwa NP | 49.08 | 21.36 |
Regarding the fire rotation period, the Lupande GMA experienced a consistent fire rotation interval of 1–2 years throughout the study’s time span. However, the fires in South Luangwa NP were less frequent, with a rotation interval of 3–4 years during the 1990s, then 2–3 years in the 2010s (Table 4). In reference to fire heterogeneity, the number of individual burns in the Lupande GMA always were greater than those found in South Luangwa NP. On average, the Lupande GMA had a 4.07 times more patches than South Luangwa NP (Supplementary Table S2). The linear model indicated no significant trend (R2 = 0.01, P value 0.27) in the ratio values over time (Fig. 7, Supplementary Table S3). Concerning the difference in the average patch size of individual burned areas (Supplementary Table S2) between Lupande GMA and South Luangwa NP, the individual Mann–Whitney U test found that they were statistically different for 20 out of the 23 years analysed for this study. Further, the overall average individual burned area across all the years was statistically significantly smaller (P ≤ 0.05) in the Lupande GMA (0.02 km2) than in South Luangwa NP (0.03 km2).
Site and time period | Fire rotation period (years) | |
---|---|---|
South Luangwa NP 1990s | 3.51 | |
South Luangwa NP 2010s | 2.75 | |
Lupande GMA 1990s | 1.55 | |
Lupande GMA 2010s | 1.56 |
These values are based on the burned area data and FRP equation.
Climate and burned area
The results of the multilinear models revealed significant positive correlations (P ≤ 0.05) between yearly burned area (km2) in South Luangwa NP and antecedent soil moisture and antecedent effective rainfall (Supplementary Table S4 and Fig. 8). No significant correlations were found between burned area in the Lupande GMA and any of the concurrent or antecedent climate variables (Supplementary Table S5). The models that performed best in both systems relied on soil moisture, explaining 14.01% of the observed burned area trend in South Luangwa NP and 13.53% in the Lupande GMA (Tables 5 and 6). Effective rainfall accounted for only 1.17% of the observed trend in burned area for South Luangwa NP and 4.64% for the Lupande GMA, whereas precipitation explained −7.59% for South Luangwa NP and −5.52% for the Lupande GMA. Furthermore, despite the lack of statistically significant correlations for the Lupande GMA and the other climate variables in South Luangwa NP, the models for both study areas reasonably reproduced the general shapes of the observed burned area trends (Fig. 8). This suggests that although climatic conditions may not mediate the trends in burned area, they still hold importance (Tables 5 and 6).
Observed versus predicted burned area in South Luangwa NP and the Lupande GMA. (BA obs, burned area estimated from the Landsat imagery; BA pred, burned area predicted by linear models; ER, effective rainfall; P, precipitation; SM, soil moisture). Dashed lines represent lines of best fit.
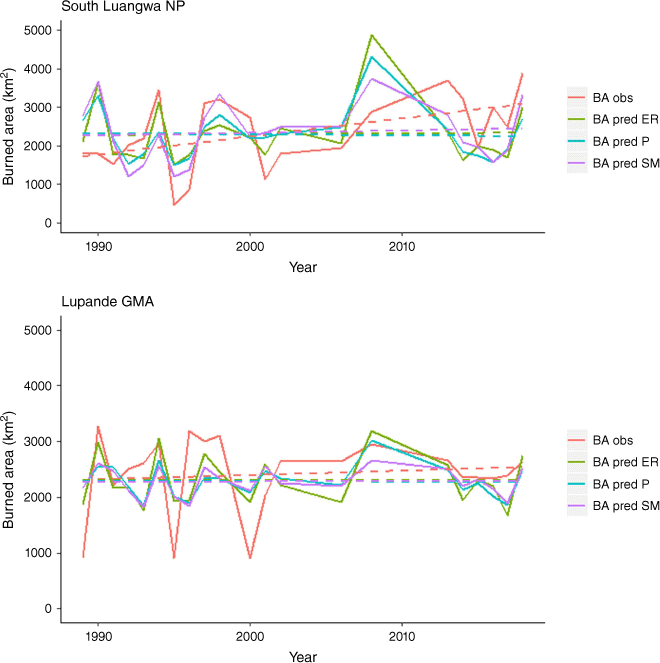
Climatic variable | South Luangwa NP (%) | Lupande GMA (%) | |
---|---|---|---|
Soil moisture (SM) | 14.01 | 13.53 | |
Effective rainfall (ER) | 1.17 | 4.64 | |
Precipitation (P) | −7.59 | −5.52 |
GMA Trends | South Luangwa NP Trends | |||||
---|---|---|---|---|---|---|
Model | Trend (% year−1) | MAE | Model | Trend (% year−1) | MAE | |
BA obs | 0.35 | – | BA obs | 1.96 | – | |
BA pred P | −0.03 | 10.73 | BA pred P | −0.11 | 20.40 | |
BA pred ER | 0.00 | 16.62 | BA pred ER | 0.09 | 31.03 | |
BA pred SM | 0.05 | 10.06 | BA pred SM | 0.27 | 25.29 |
MAE is the mean absolute error as a percentage of the average observed burned area.
MODIS de-seasoned climate analysis
Within South Luangwa NP, the 9- and 19-month lags showed a significant positive correlation between precipitation and burned area (see Fig. 9). The strong positive correlation at 9- and 19-month temporal lags reveals that South Luangwa NP, a largely unmanaged savanna ecosystem, showed a positive relationship of precipitation with burned area, meaning that increases in precipitation during the wet season led to increases in burned area during the dry season. It is worth mentioning that there is an unexplained strong negative correlation at a 19-month lag. In the Lupande GMA, the relationship of burned area with precipitation is reversed. Increased wet season rainfall in the Lupande GMA leads to a reduction in burned area during the dry season. Significant negative correlations between precipitation and burned area were found at 3- and 18-month lags (Fig. 9). The 18-month lag was more substantial than the 3-month lag, indicating that the antecedent precipitation conditions were more influential than concurrent conditions.
Cross-correlation function (CCF) graphs comparing de-seasoned data of burned area and precipitation. Cross-correlation is a measure of similarity of the two time series as a function of the displacement of one relative to the other, with −1 and 1 indicating maximum correlation and 0 indicating no correlation. The blue lines denote the values beyond which the correlations are (statistically) significantly different from zero. Strong positive correlations at 8- and 18-month delays can be seen in South Luangwa NP.
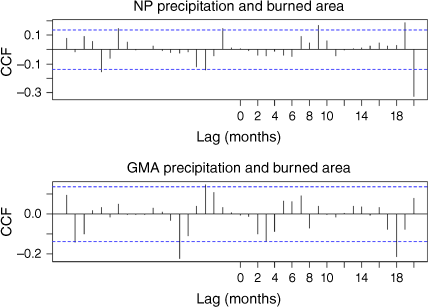
MODIS trend analysis
The cross-correlation analysis was repeated with the trends extracted from the raw time series data to investigate if the general trends in burned area and precipitation were correlated. There were significant positive correlations between precipitation levels within South Luangwa NP and trends of burned area when a 1-year lag was present (see Fig. 10), indicating that increases in rainfall during the wet season relate to increases in burned area in the subsequent year. However, no significant correlations were detected between the precipitation and burned area within the Lupande GMA, and any correlations that were measured were negative (Fig. 10). Thus, varying levels of rainfall within the Lupande GMA did not result in any changes in burned area.
Cross-correlation graphs comparing trends of burned area and precipitation. Cross-correlation is a measure of similarity of the two time series as a function of the displacement of one relative to the other, with −1 and 1 indicating maximum correlation and 0 indicating no correlation. The blue lines denote the values beyond which the correlations are (statistically) significantly different from zero. South Luangwa NP demonstrated a correlation between precipitation and burned area at multiple lags, unlike the Lupande GMA.
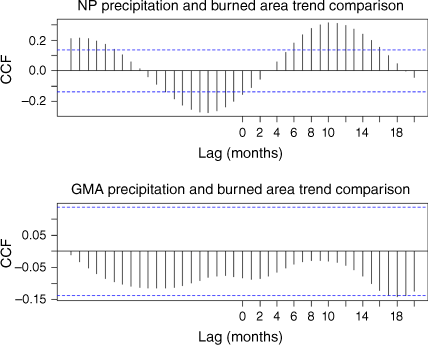
Discussion
The populated Lupande GMA and the adjacent unpopulated South Luangwa NP provide an opportunity to investigate the effects humans have on fire regimes within a sub-Saharan savanna ecosystem over various time and space scales. Overall, the Lupande GMA had a greater burned area per year, a higher frequency of fires and earlier fires when compared with South Luangwa NP. It is worth noting that although less intense fires in the Lupande GMA may indeed limit the immediate impact of fire on mature woody vegetation, the ecological implications extend beyond recruitment alone. Although many tree species in these fire-prone ecosystems have evolved adaptations to withstand frequent fires, such as vigorous resprouting and seedling production, concern arises from the potential for recurrent fires to ‘trap’ trees in the juvenile phase and contribute to dieback of mature trees over time (Higgins et al. 2000; Higgins et al. 2007; Bond 2008; Case and Staver 2017). So, although the current fire regime may not be reducing woody vegetation in the Lupande GMA, it can potentially limit the ability for new trees to become established and maintain current tree cover.
Climate variability, particularly changes in precipitation patterns, played a significant role in shaping the fire regimes of the two areas. According to our study using Landsat-derived burned area data, antecedent soil moisture in South Luangwa NP is a predictor of burned area (Zubkova et al. 2019). This result is likely due to soil moisture in previous wet seasons promoting herbaceous biomass productivity and hence more flammable fuels for subsequent years (Lehmann et al. 2008; Archibald et al. 2010). The influence of soil moisture over burned area would suggest that the fire regime of South Luangwa NP is, at least in part, dictated by fluctuations in the climatic conditions. This result is important when compared with previous research that investigated the drivers of large-scale vegetation change in southern African savannas. That research found that soil moisture in areas with similar amounts of annual rainfall to the Luangwa valley is less influential on changes in vegetation patterns than variables such as fire (Campo-Bescós et al. 2013). Our results provide more specific information on the relationship between fire regimes and soil moisture and demonstrate that soil moisture itself may be driving the increased influence of fire in these savannas. However, it is still important to consider, especially at finer scales, that this relationship between fire and soil moisture is potentially contingent on the degree of human influence on the fire regimes, as there was no significant relationship between soil moisture and burned area in the Lupande GMA. The results of the time series models using the MODIS burned area data further support the finding that the influence of climate on burned area differs between the two areas. The relationship between precipitation and burned area trends is positive and significant in South Luangwa NP. In contrast, the negative correlation between precipitation and burned area in the Lupande GMA suggests that a lack of precipitation increased burned area. Overall, these results support the theory that human fire management in African savannas mediates the effect of climate on interannual burned area variation (Archibald et al. 2010; Laris 2011).
The growth of the human population in the Lupande GMA did not result in any positive or negative trend in burned areas over time. However, the human-dominated landscape of the Lupande GMA demonstrated a higher fire frequency and larger burned area than the non-human-dominated South Luangwa NP. In contrast to Lupande GMA, there was a slight increase in burned area over time for South Luangwa NP. The lack of any observable trend in burned areas indicates that an increase in the number of people per square kilometre alone does not determine the threshold (Archibald et al. 2009; Archibald et al. 2010). Furthermore, burned area trends did not emerge despite extensive land cover conversions to agriculture in the past 30 years (Grégoire et al. 2013). This can likely be explained by the chitimene burned regimes that are used by communities in Miombo woodlands. Despite clearing land for agriculture, within chitimene practices, fires are still used as a fertilisation method in cleared plots (Fritz 2013). This practice explains the continued detection of burned areas in places that have undergone land cover change (Chidumayo 1987). The consistently large burned area in the Lupande GMA contradicts previous studies’ findings suggesting that burning was more extensive inside national parks of southern Africa than outside (Grégoire et al. 2013). This may be the result of the present study’s use of higher-resolution Landsat imagery, which allows the detection of smaller burned areas in the human-dominated area of the GMA.
Building on our findings concerning fire regimes and their underlying drivers, it is essential to place our research within the broader context of mitigating methane emissions from savanna wildfires, a globally recognised challenge linked to reducing methane, a potent greenhouse gas, with increasing concerns about wildfires as a significant contributor (Lipsett-Moore et al. 2018; Laris et al. 2021, 2023). Although earlier research had suggested the potential for substantial emission reductions through a global-scale strategy of shifting fire periods to the early dry season in African savannas (Lipsett-Moore et al. 2018), our findings challenge this assumption. In regions where human influence dominates fire regimes, exemplified by the Lupande GMA, fires are already prevalent during the early dry season, and this pattern persists irrespective of changes in human population density. This observation underscores that mere adjustments to the timing of fire regimes may prove inadequate for achieving substantial emissions reductions, given that many communities across sub-Saharan Africa are already initiating fires early in the dry season. Furthermore, as others have noted, the complex nature of wildfire emissions from fires raises doubts about whether altering the timing of burning would yield the desired outcomes (Laris et al. 2021, 2023).
Although this study did produce significant findings regarding the fire regimes, there were also several limitations inherent to the study. The largest limitation is the temporal gaps in Landsat imagery, which are due to the Landsat 7 scan-line correction failure, cloud cover and lack of available imagery. This results in multiple years with few or no available images to be used in determining total burned area. These constraints reduced the amount of imagery we could draw from for making yearly composites of burned area. Furthermore, the 16-day return period of the Landsat satellites results in yet another unavoidable omission in the dataset. Burn scars are also ephemeral, and certain recent burned areas may not have been detected as they may have decreased in intensity by the time of image acquisition. Previous research suggests that the remotely sensed signal of a burned area can return to pre‐burn levels in as little as 1–2 weeks (Trigg and Flasse 2000). These temporal gaps mean that we may have underestimated the impact of fire on tree cover in South Luangwa NP in particular, because fires that burned large areas in the national park may have occurred during the temporal gaps in the Landsat data.
Conclusion
As the climate becomes more variable and human pressure on African savannas increases, developing a better understanding of relationships and improving management practices in such sensitive and vital landscapes is imperative. Our studied showed that the different management practices of the South Luangwa NP and the Lupande GMA resulted in contrasting burned area patterns, the ramifications of which could have lasting implications for the ecology of the region over time. The recruitment of new trees in Lupande GMA will struggle as the frequent fires will inhibit growth of young trees. Additionally, woody vegetation in the Lupande GMA is felled for agriculture and local communities continue to harvest wood for home use (Watson et al. 2015). As for South Luangwa NP, woody vegetation may increase as a relatively low fire frequency will allow more growth. This study contributes to the growing body of literature illuminating the relationship between anthropogenic pressures, climatic conditions and fires, but additional remote sensing analysis of human fire behaviour is needed in other southern African localities to further validate the relationships observed. A key finding relates to how climatic conditions outweigh human influences in unpopulated areas such as the areas within South Luangwa NP. Such findings are crucial to contribute to the broader literature on savanna fire systems and highlight important factors for consideration in managing both protected areas.
Acknowledgements
We would like to acknowledge Dr Douglas O. Fuller for the helping with the inception and early stages of this research.
References
Abatzoglou JT, Williams AP, Boschetti L, Zubkova M, Kolden CA (2018) Global patterns of interannual climate–fire relationships. Global Change Biology 24, 5164-5175.
| Crossref | Google Scholar | PubMed |
Alcaras E, Costantino D, Guastaferro F, Parente C, Pepe M (2022) Normalized Burn Ratio Plus (NBR+): a new index for Sentinel-2 imagery. Remote Sensing 14, 1727.
| Crossref | Google Scholar |
Andela N, van der Werf GR (2014) Recent trends in African fires driven by cropland expansion and El Niño to La Niña transition. Nature Climate Change 4, 791-795.
| Crossref | Google Scholar |
Archibald S, Roy DP, Van Wilgen BW, Scholes RJ (2009) What limits fire? An examination of drivers of burnt area in southern Africa. Global Change Biology 15, 613-630.
| Crossref | Google Scholar |
Archibald S, Scholes RJ, Roy DP, Roberts G, Boschetti L (2010) Southern African fire regimes as revealed by remote sensing. International Journal of Wildland Fire 19, 861-878.
| Crossref | Google Scholar |
Bastarrika A, Chuvieco E, Martín MP (2011) Mapping burned areas from Landsat TM/ETM+ data with a two-phase algorithm: balancing omission and commission errors. Remote Sensing of Environment 115, 1003-1012.
| Crossref | Google Scholar |
Blackman A (2013) Evaluating forest conservation policies in developing countries using remote sensing data: an introduction and practical guide. Forest Policy and Economics 34, 1-16.
| Crossref | Google Scholar |
Bond WJ (2008) What limits trees in C4 grasslands and savannas? Annual Review of Ecology Evolution and Systematics 39, 641-659.
| Google Scholar |
Bond WJ, Midgley GF, Woodward FI (2003) What controls South African vegetation – climate or fire? South African Journal of Botany 69, 79-91.
| Crossref | Google Scholar |
Bond WJ, Woodward FI, Midgley GF (2005) The global distribution of ecosystems in a world without fire. New Phytologist 165, 525-538.
| Crossref | Google Scholar | PubMed |
Boschetti L, Roy DP, Giglio L, Huang H, Zubkova M, Humber ML (2019) Global validation of the collection 6 MODIS burned area product. Remote Sensing of Environment 235,.
| Crossref | Google Scholar | PubMed |
Bowman DMJS, Balch J, Artaxo P, Bond WJ, Cochrane MA, D’Antonio CM, DeFries R, Johnston FH, Keeley JE, Krawchuk MA, Kull CA, Mack M, Moritz MA, Pyne S, Roos CI, Scott AC, Sodhi NS, Swetnam TW, Whittaker R (2011) The human dimension of fire regimes on Earth. Journal of Biogeography 38, 2223-2236.
| Crossref | Google Scholar | PubMed |
Brockett BH, Biggs HC, van Wilgen BW (2001) A patch mosaic burning system for conservation areas in southern African savannas. International Journal of Wildland Fire 10, 169-183.
| Crossref | Google Scholar |
Campo-Bescós MA, Muñoz-Carpena R, Kaplan DA, Southworth J, Zhu L, Waylen PR (2013) Beyond precipitation: physiographic gradients dictate the relative importance of environmental drivers on savanna vegetation. PLoS One 8, e72348.
| Crossref | Google Scholar | PubMed |
Case MF, Staver AC (2017) Fire prevents woody encroachment only at higher-than-historical frequencies in a South African savanna. Journal of Applied Ecology 54, 955-962.
| Crossref | Google Scholar |
Chidumayo EN (1987) A shifting cultivation land use system under population pressure in Zambia. Agroforestry Systems 5, 15-25.
| Crossref | Google Scholar |
Chuvieco E, Martín MP, Palacios A (2002) Assessment of different spectral indices in the red–near-infrared spectral domain for burned land discrimination. International Journal of Remote Sensing 23, 5103-5110.
| Crossref | Google Scholar |
Daniau A-L, Sánchez Goñi MF, Martinez P, Urrego DH, Bout-Roumazeilles V, Desprat S, Marlon JR (2013) Orbital-scale climate forcing of grassland burning in southern Africa. Proceedings of the National Academy of Sciences 110, 5069-5073.
| Crossref | Google Scholar | PubMed |
Daskin JH, Stalmans M, Pringle RM (2016) Ecological legacies of civil war: 35-year increase in savanna tree cover following wholesale large-mammal declines. Journal of Ecology 104, 79-89.
| Crossref | Google Scholar |
Eriksen C (2007) Why do they burn the ‘bush’? Fire, rural livelihoods, and conservation in Zambia. Geographical Journal 173, 242-256.
| Crossref | Google Scholar |
Estes BL, Knapp EE, Skinner CN, Miller JD, Preisler HK (2017) Factors influencing fire severity under moderate burning conditions in the Klamath Mountains, northern California, USA. Ecosphere 8, e01794.
| Crossref | Google Scholar |
Fritz A (2013) Chitimene agriculture: Cultural and economic factors related to population increase contributing to forest degradation of Miombo woodlands in north and central Zambia. Agrarian Frontiers 1, 12-22.
| Google Scholar |
García MJL, Caselles V (1991) Mapping burns and natural reforestation using Thematic Mapper data. Geocarto International 6, 31-37.
| Crossref | Google Scholar |
Giddey BL, Baard JA, Kraaij T (2022) Fire severity and tree size affect post-fire survival of Afrotemperate forest trees. Fire Ecology 18, 5.
| Crossref | Google Scholar |
Govender N, Trollope WSW, Van Wilgen BW (2006) The effect of fire season, fire frequency, rainfall and management on fire intensity in savanna vegetation in South Africa. Journal of Applied Ecology 43, 748-758.
| Crossref | Google Scholar |
Grégoire JM, Eva HD, Belward AS, Palumbo I, Simonetti D, Brink A (2013) Effect of land-cover change on Africa’s burnt area. International Journal of Wildland Fire 22, 107.
| Crossref | Google Scholar |
Higgins SI, Bond WJ, February EC, Bronn A, Euston-Brown DI, Enslin B, Govender N, Rademan L, O’Regan S, Potgieter ALF, Scheiter S, Sowry R, Trollope L, Trollope WSW (2007) Effects of four decades of fire manipulation on woody vegetation structure in savanna. Ecology 88, 1119-1125.
| Crossref | Google Scholar | PubMed |
Higgins SI, Bond WJ, Trollope WSW (2000) Fire, resprouting and variability: a recipe for grass-tree coexistence in savanna. Journal of Ecology 88, 213-229.
| Crossref | Google Scholar |
Hodgkinson KC (1998) Sprouting success of shrubs after fire: height-dependent relationships for different strategies. Oecologia 115, 64-72.
| Crossref | Google Scholar | PubMed |
Hoffmann WA, Solbrig OT (2003) The role of topkill in the differential response of savanna woody species to fire. Forest Ecology and Management 180, 273-286.
| Crossref | Google Scholar |
Karp AT, Uno KT, Berke MA, Russell JM, Scholz CA, Marlon JR, Faith JT, Staver AC (2023) Non-linear rainfall effects on savanna fire activity across the African Humid Period. Quaternary Science Reviews 304, 107994.
| Crossref | Google Scholar |
Keeley JE (2009) Fire intensity, fire severity and burn severity: a brief review and suggested usage. International Journal of Wildland Fire 18, 116-126.
| Crossref | Google Scholar |
Laris P (2011) Humanizing savanna biogeography: linking human practices with ecological patterns in a frequently burned savanna of Southern Mali. Annals of the Association of American Geographers 101, 1067-1088.
| Crossref | Google Scholar |
Laris P, Caillault S, Dadashi S, Jo A (2015) The human ecology and geography of burning in an unstable savanna environment. Journal of Ethnobiology 35, 111-139.
| Crossref | Google Scholar |
Laris P, Koné M, Dadashi S, Dembele F (2017) The early/late fire dichotomy: time for a reassessment of Aubréville’s savanna fire experiments. Progress in Physical Geography: Earth and Environment 41, 68-94.
| Crossref | Google Scholar |
Laris P, Jo A, Wechsler SP (2018) Effects of landscape pattern and vegetation type on the fire regime of a mesic savanna in Mali. Journal of Environmental Management 227, 134-145.
| Crossref | Google Scholar | PubMed |
Laris P, Koné M, Dembélé F, Rodrigue CM, Yang L, Jacobs R, Laris Q (2021) Methane gas emissions from savanna fires: what analysis of local burning regimes in a working West African landscape tell us. Biogeosciences 18, 6229-6244.
| Crossref | Google Scholar |
Laris P, Koné M, Dembélé F, Rodrigue CM, Yang L, Jacobs R, Laris Q, Camara F (2023) The pyrogeography of methane emissions from seasonal mosaic burning regimes in a West African landscape. Fire 6, 52.
| Crossref | Google Scholar |
Lehmann CER, Prior LD, Williams RJ, Bowman DMJS (2008) Spatio-temporal trends in tree cover of a tropical mesic savanna are driven by landscape disturbance. Journal of Applied Ecology 45, 1304-1311.
| Crossref | Google Scholar |
Lehmann CER, Prior LD, Bowman DMJS (2009) Fire controls population structure in four dominant tree species in a tropical savanna. Oecologia 161, 505-515.
| Crossref | Google Scholar | PubMed |
Lehmann CER, Anderson TM, Sankaran M, Higgins SI, Archibald S, Hoffmann WA, Hanan NP, Williams RJ, Fensham RJ, Felfili J, Hutley LB, Ratnam J, San Jose J, Montes R, Franklin D, Russell-Smith J, Ryan CM, Durigan G, Hiernaux P, Haidar R, Bowman DMJS, Bond WJ (2014) Savanna vegetation–fire–climate relationships differ among continents. Science 343, 548-552.
| Crossref | Google Scholar | PubMed |
Lipsett-Moore GJ, Wolff NH, Game ET (2018) Emissions mitigation opportunities for savanna countries from early dry season fire management. Nature Communications 9, 2247.
| Crossref | Google Scholar | PubMed |
Liu JX, Heiskanen J, Maeda EE, Pellikka PKE (2018) Burned area detection based on Landsat time series in savannas of southern Burkina Faso. International Journal of Applied Earth Observation and Geoinformation 64, 210-220.
| Crossref | Google Scholar |
McNally A, Arsenault K, Kumar S, Shukla S, Peterson P, Wang S, Funk C, Peters-Lidard CD, Verdin JP (2017) A land data assimilation system for sub-Saharan Africa food and water security applications. Scientific Data 4, 170012.
| Crossref | Google Scholar | PubMed |
Midgley JJ, Lawes MJ, Chamaillé-Jammes S (2010) Savanna woody plant dynamics: the role of fire and herbivory, separately and synergistically. Australian Journal of Botany 58, 1-11.
| Crossref | Google Scholar |
Mvula CD (2001) Fair trade in tourism to protected areas – A micro case study of wildlife tourism to South Luangwa National Park, Zambia. International Journal of Tourism Research 3, 393-405.
| Crossref | Google Scholar |
Nyirenda V, Chansa WC, Myburgh WJ, Reilly BK (2010) Social capital and community responses to natural resource management in the Luangwa Valley, Zambia. Journal of Sustainable Development in Africa 12, 158-180.
| Google Scholar |
Pekel J-F, Cottam A, Gorelick N, Belward AS (2016) High-resolution mapping of global surface water and its long-term changes. Nature 540, 418-422.
| Crossref | Google Scholar | PubMed |
Pereira Júnior AC, Oliveira SL, Pereira JM, Turkman MA (2014) Modelling fire frequency in a cerrado savanna protected area. PLoS One 9, e102380.
| Crossref | Google Scholar | PubMed |
R CoreTeam (2020) R: A language and environment for statistical computing. Available at https://www.R-project.org/
Rosenbaum PR, Rubin DB (1983) The central role of the propensity score in observational studies for causal effects. Biometrika 70, 41-55.
| Crossref | Google Scholar |
Roteta E, Bastarrika A, Padilla M, Storm T, Chuvieco E (2019) Development of a Sentinel-2 burned area algorithm: generation of a small fire database for sub-Saharan Africa. Remote Sensing of Environment 222, 1-17.
| Crossref | Google Scholar |
Sankaran M, Hanan NP, Scholes RJ, Ratnam J, Augustine DJ, Cade BS, Gignoux J, Higgins SI, Le Roux X, Ludwig F, Ardo J, Banyikwa F, Bronn A, Bucini G, Caylor KK, Coughenour MB, Diouf A, Ekaya W, Feral CJ, February EC, Frost PG, Hiernaux P, Hrabar H, Metzger KL, Prins HH, Ringrose S, Sea W, Tews J, Worden J, Zambatis N (2005) Determinants of woody cover in African savannas. Nature 438, 846-849.
| Crossref | Google Scholar | PubMed |
Shrader AM, Pimm SL, van Aarde RJ (2010) Elephant survival, rainfall and the confounding effects of water provision and fences. Biodiversity and Conservation 19, 2235-2245.
| Crossref | Google Scholar |
Smit IPJ, Asner GP, Govender N, Vaughn NR, van Wilgen BW (2016) An examination of the potential efficacy of high-intensity fires for reversing woody encroachment in savannas. Journal of Applied Ecology 53, 1623-1633.
| Crossref | Google Scholar |
Smit IPJ, Asner GP, Govender N, Kennedy-Bowdoin T, Knapp DE, Jacobson J (2010) Effects of fire on woody vegetation structure in African savanna. Ecological Applications 20, 1865-1875.
| Crossref | Google Scholar | PubMed |
Staver AC, Archibald S, Levin S (2011) Tree cover in sub-Saharan Africa: rainfall and fire constrain forest and savanna as alternative stable states. Ecology 92, 1063-1072.
| Crossref | Google Scholar | PubMed |
Trapnell CG (1959) Ecological results of woodland and burning experiments in Northern Rhodisia. Journal of Ecology 47, 129-168.
| Crossref | Google Scholar |
Trigg S, Flasse S (2000) Characterizing the spectral-temporal response of burned savannah using in situ spectroradiometry and infrared thermometry. International Journal of Remote Sensing 21, 3161-3168.
| Crossref | Google Scholar |
Trigg S, Flasse S (2001) An evaluation of different bi-spectral spaces for discriminating burned shrub-savannah. International Journal of Remote Sensing 22, 2641-2647.
| Crossref | Google Scholar |
Trollope WSW, Tainton NM (1986) Effect of fire intensity on the grass and bush components of the Eastern Cape thornveld. Journal of the Grassland Society of Southern Africa 3, 37-42.
| Crossref | Google Scholar |
van Gerrevink MJ, Veraverbeke S (2021) Evaluating the hyperspectral sensitivity of the differenced normalized burn ratio for assessing fire severity. Remote Sensing 13, 4611.
| Crossref | Google Scholar |
van Langevelde F, Van De Vijver CADM, Kumar L, van de Koppel J, de Ridder N, van Andel J, Skidmore AK, Hearne JW, Stroosnijder L, Bond WJ, Prins HHT, Rietkerk M (2003) Effects of fire and herbivory on the stability of savanna ecosystems. Ecology 84, 337-350.
| Crossref | Google Scholar |
Vargas-Sanabria D, Campos-Vargas C (2020) Multitemporal comparison of burned areas in a tropical dry forest using the burned area index (BAI). Revista Forestal Mesoamerica Kurú 17(41), 29-36.
| Crossref | Google Scholar |
Veraverbeke S, Verstraeten WW, Lhermitte S, Goossens R (2010) Illumination effects on the differenced Normalized Burn Ratio’s optimality for assessing fire severity. International Journal of Applied Earth Observation and Geoinformation 12, 60-70.
| Crossref | Google Scholar |
Wall WA, Hohmann MG, Just MG, Hoffmann WA (2021) Characterizing past fire occurrence in longleaf pine ecosystems with the Mid-Infrared Burn Index and a Random Forest classifier. Forest Ecology and Management 500, 119635.
| Crossref | Google Scholar |
Watson FGR, Becker MS, Milanzi J, Nyirenda M (2015) Human encroachment into protected area networks in Zambia: implications for large carnivore conservation. Regional Environmental Change 15, 415-429.
| Crossref | Google Scholar |
Werf G, Randerson J, Giglio L, Gobron N, Dolman H (2008) Climate controls on the variability of fires in the tropics and subtropics. Global Biogeochemical Cycles 22, GB3028.
| Crossref | Google Scholar |
Wu B, Zheng H, Xu ZL, Wu ZW, Zhao YD (2022) Forest burned area detection using a novel spectral index based on multi-objective optimization. Forests 13, 1787.
| Crossref | Google Scholar |
Yang W, Zhang SW, Tang JM, Bu K, Yang JC, Chang LP (2013) A MODIS time series data based algorithm for mapping forest fire burned area. Chinese Geographical Science 23, 344-352.
| Crossref | Google Scholar |
Zubkova M, Boschetti L, Abatzoglou JT, Giglio L (2019) Changes in fire activity in Africa from 2002 to 2016 and their potential drivers. Geophysical Research Letters 46, 7643-7653.
| Crossref | Google Scholar | PubMed |