Nationally consistent mapping of wildland fuel types across Australia using satellite-derived vegetation structural data
Rakesh C. Joshi A * , Miguel G. Cruz
A
B
Abstract
Knowledge of the distribution of wildland fuels across the landscape is necessary for the appropriate application of models used to support a broad range of fire management activities.
To develop an automated and nationally consistent method that generates up-to-date spatial fuel type information across Australia.
Data from various space-borne broad-band optical, LiDAR and radar sensors were combined with land use data to generate structural descriptions of vegetation that were then converted into fuel types.
An Australian fuel type spatial layer was generated using the Bushfire Fuel Classification fuel typology. Evaluation against field measurements revealed accuracies of 89 and 71% for native forest and non-forest fuel types, respectively. This product provides a higher level of spatial and structural detail than previously obtained by other national-level fuel classification approaches in Australia.
The developed fuel type layer is made available and can be readily used in research applications. The data also have use in supporting jurisdictional-level fuel mapping for a range of fire management applications, such as fire behaviour prediction, fire danger forecasting and risk assessment.
Keywords: Bushfire fuels, Bushfire Fuel Classification, Eucalypt forest, Fuel arrangement, Fuel types, Remote sensing, Vegetation structure, Wildfire fuel map.
Introduction
As the management of wildland fires increases in complexity owing to factors such as global warming (Bowman et al. 2013; Sharples et al. 2016), changes in fire regimes (Bradstock 2010) and human encroachment into wildfire-prone areas (Syphard et al. 2012; Gill et al. 2013; Moritz et al. 2014), there is a need to improve how land management and fire control agencies quantify wildfire potential and impact to human values and ecological resources. Knowledge of the distribution of wildland fuels across the landscape is necessary for the appropriate use of models for such applications as fire danger and fire risk assessment, fire behaviour prediction, prescribed burn planning and smoke forecasting.
Wildland fuels form complex three-dimensional assemblages of combustible cellulosic materials (Keane 2015). Fuel descriptions are often based on the intended applications and can be related to vegetation structure (e.g. forest, shrubland, grassland), dominant plant taxa (e.g. in Australia, eucalypt forest, spinifex woodland, pine forest), fuel strata (e.g. surface, canopy), and chemical (e.g. heat content) and physical properties (e.g. mass, density) (Hollis et al. 2015). Fuels are often classified or categorised into fuel types as a way to overcome the complexity of describing physical fuel characteristics in a consistent manner (Pyne 1984; Keane et al. 2001), with a fuel type encompassing fuel complexes of similar structure and potential fire behaviour. Fuel type classification systems are often linked with fire danger and behaviour prediction systems, such as the fuel types associated with the Canadian Fire Behavior Prediction (FBP) system (Forestry Canada Fire Danger Group 1992), the US National Fire Danger Rating system (Deeming et al. 1974; Cohen and Deeming 1985; Jolly et al. 2024) and Fire Behaviour Prediction System (Albini 1976; Scott and Burgan 2005; Andrews et al. 2008), and the Australian Fire Danger Rating System, AFDRS (Hollis et al. 2024b; Kenny et al. 2024). Other systems have been proposed throughout the world that rely on the above-mentioned fire behaviour modelling systems, namely in New Zealand (Clifford et al. 2010), Spain (ICONA 1990), Portugal (Fernandes et al. 2009) and Greece (Dimitrakopoulos 2002).
In Australia, multiple fuel classification systems are currently used operationally. Each state and territory typically uses one, or more, state-specific fuel classification systems that links vegetation floristics and structure with fire behaviour model inputs (Tolhurst 2005; Watson 2011) to support their jurisdictional fire management activities. At a national level, three main approaches have been proposed: (i) the AS3959 standard for construction of buildings in fire-prone areas (Standards Australia 2018); (ii) the Bushfire Fuel Classification, BFC (Hollis et al. 2015; Cruz et al. 2018); and (iii) the AFDRS fuel type classification (Matthews et al. 2019; Kenny et al. 2024). Currently, an Australian fire control agency will possibly deal with at least three distinct fuel classification systems on its day-to-day business.
Independent of the geographical location, a fuel type will have an associated set of fire spread and behaviour models (e.g. Forestry Canada Fire Danger Group 1992; Cruz et al. 2015b; Hollis et al. 2024a), or a distinct set of input fuel values for such models, such as in the fuel model concept used in systems based on the Rothermel (1972) surface fire spread model (e.g. Finney 2004, 2006; Monedero et al. 2019). When estimating fire behaviour, inaccuracies in fuel type mapping can lead to applying the wrong fire behaviour and/or the wrong fuel models, resulting in possible large uncertainties in forecast fire behaviour, danger and risk.
Early efforts mapping fuel types at local (e.g. forest estate), regional or national levels combined existing ecological and vegetation maps (sometimes enhanced with aerial photography interpretation) with heuristic rules (Kessell 1976; Chandler et al. 1983; Phelps and Beverly 2022; Kenny et al. 2024). The availability of satellite remote sensing has provided a cost-effective process to derive fuel type maps at variable scales (Wilson et al. 1994; Keane et al. 2001; Chuvieco et al. 2003). The use of passive sensors (e.g. multispectral and hyperspectral optical sensors) has received the most attention (Chuvieco et al. 2020; Abdollahi and Yebra 2023). Typically, this approach entails determining vegetation classes from spectral analysis with a fuel type/model being assigned to each class through a ruleset or machine learning approach (e.g. Chuvieco et al. 2020; Stefanidou et al. 2022). Rollins (2009) and Aragoneses et al. (2023) are good examples of the extension of these methods to map fuel types at large spatial extents in, respectively, the USA and Europe. The temporal and spatial resolutions of available passive sensors also allow for the capture of changes in vegetation due to recent wildfires and land use practices.
The use of active sensors, such as Light Detection and Ranging (LiDAR) and radar, provides information on the three-dimensional structure of vegetation. In combination with spectral sensors (Marselis et al. 2016; Scarth et al. 2019; Potapov et al. 2021; Lang et al. 2023; Mihajlovski et al. 2023; Ticehurst and Newnham 2024), these active sensors overcome some of the shortcomings of methods that rely solely on spectral imagery to detect vegetation class–fuel type associations (e.g. Erdody and Moskal 2010; Abdollahi and Yebra 2023).
We aim to develop an automated and nationally consistent method that identifies and classifies Australian wildland fuel types relevant to a range of fire management applications, such as fire behaviour prediction, fire danger forecasting and risk assessment. The approach primarily uses up-to-date, space-borne remote sensing data, supplemented with existing spatial vegetation information when necessary. We apply the data through a set of rules to derive wildland fuel type layers using the BFC fuel typology. An evaluation of the modelling approach and the resulting fuel type layers against independent ground truth data is conducted to understand limitations of the data and processes used and the implications of these limitations, and to identify areas of improvement.
Methods
An overview of the source data layers, variables and methods used to derive Australian fuel type layers is presented in Fig. 1. Data from various space-borne sensors, like broad-band optical, LiDAR and radar sensors, were combined to generate structural descriptions of vegetation that could then be converted into fuel types. For several specific fuel types that are characterised by features not captured through remote sensing, we relied on existing information layers (e.g. land use, and vegetation and plantation types) to identify their spatial extent. Rule-based algorithms were developed to classify vegetation into the selected fuel typology.
Overview of the approach taken to map fuel types across Australia. The approach incorporates a diverse range of remotely sensed data sources as well as existing spatial layers. Specific variables are extracted from these sources that describe underlying structural attributes (such as height and cover) and specific vegetation/fuel types (such as cropping). These are processed into generic fuel structural types, which are then converted into fuel type layers according to specific fuel typologies – in our case, the Bushfire Fuel Classification. (BoM, Bureau of Meteorology.)
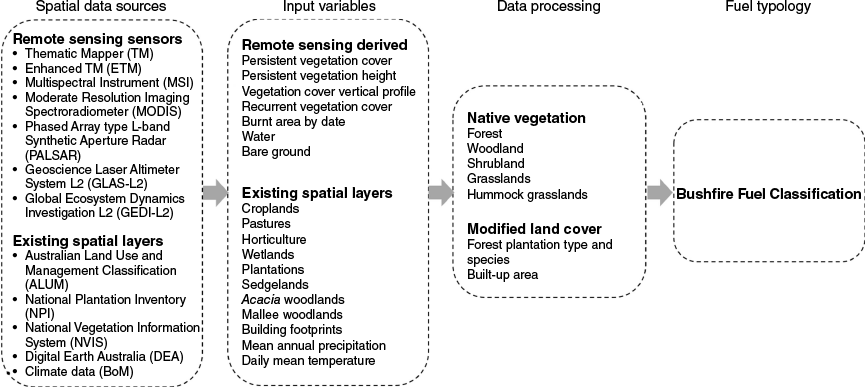
The Bushfire Fuel Classification typology
The focus of this work is to generate layers of fundamental fuel structural attributes and then to convert these into fuel type layers using an existing fuel typology. Although any structurally based typology could be used, we used the BFC (Hollis et al. 2015; Cruz et al. 2018). This typology is a hierarchical classification of wildland fire fuels developed in close consultation with stakeholders to meet the requirements of Australian fire management applications (Hollis et al. 2015). At its basis, the classification integrates life form (e.g. trees, shrubs, grass), vegetation height and foliage cover characteristics, as per Specht (1970) and Carnahan (1990), to derive a structural representation of fuel types. The hierarchical approach comprises three distinct tiers that allow for descriptions of fuel types for a range of applications and data accuracies. Top-tier fuel types give a broad overview of the overarching fuel type (e.g. open forest, woodland, shrubland) but do not have enough detail for fire behaviour modelling in a number of fuel types. The mid-tier fuel types are nested within the top-tier group and provide information on understorey fuels, allowing the most appropriate fire behaviour model to be used in each fuel type. The bottom-tier fuel types were envisioned to provide detailed static fuel information; yet, as fuel is temporally dynamic, this bottom tier can be replaced by models of fuel structure dynamics (e.g. fuel load accumulation with time since the most recent fire (Watson 2011)).
Input variables
The approach to derive the fuel type layer relied as much as possible on the use of current remote sensing data. For selected fuel types for which remote sensing products did not offer enough discriminatory power, we relied on existing spatial layers relating to land use and vegetation type. Table 1 lists the input variables required for the rulesets used to generate the BFC fuel type layers. More detailed descriptions of the remote sensing-derived variables are given below. Variables in Table 1 that were obtained from existing spatial sources and used directly without further modification (noted in the Table 1 as ‘Used as per source’) are not further described here. Any input data not already in the Australian Albers GDA94 projection were reprojected and resampled with a 90 m grid resolution. The nearest neighbour resampling method was used when the original input resolution was higher than 90 m, and bilinear interpolation was used for lower resolutions.
Variable | Notation | Resolution (m) | Data currency | Reference | Satellite sensors | Description (including units) | Purpose | |
---|---|---|---|---|---|---|---|---|
Cropland | Ac | 250 | 2016 | ABARES (2021) | NA | Cropping [SECV8, 3.3] land use (a value of 1 denotes cropping). Used as per source | Identify fuel type | |
Horticulture | Ah | 250 | 2016 | ABARES (2021) | NA | Intensive horticulture [SECV8, 5.1] and irrigated perennial horticulture [SECV8, 4.4] land uses (a value of 1 denotes horticulture). Used as per source | Identify fuel type | |
Bare ground | Cb | 500 | 2001–2022 | Guerschman and Hill (2018) | MODIS | Proportion of ground free from live or dead vegetation cover (e.g. sand, bare soil) (%) | Identify fuel type | |
Woody foliage cover | Cp | 250 | 2001–2022 | Donohue and Renzullo (2025) | MODIS | Woody foliage cover (%) | Representation of woody foliage cover | |
Woody foliage cover 0–5 m | Cp1 | 30 | 2001–2022 | Scarth et al. (2019) and Donohue and Renzullo (2025) | TM, ETM, PALSAR, GLAS-L2/MSI, MODIS | Woody foliage cover (%) for vegetation between 0 and 5 m height | Representation of canopy cover of vegetation below 5 m | |
Woody foliage cover over 5 m | Cp2 | 30 | 2001–2022 | Scarth et al. (2019) and Donohue and Renzullo (2025) | TM, ETM, PALSAR, GLAS-L2/MSI, MODIS | Woody foliage cover (%) for vegetation above 5 m height | Representation of canopy cover of vegetation above 5 m | |
Woody foliage cover over 10 m | Cp3 | 30 | 2001–2022 | Scarth et al. (2019) and Donohue and Renzullo (2025) | TM, ETM, PALSAR, GLAS-L2/MSI, MODIS | Woody foliage cover (%) for vegetation over 10 m height | Representation of canopy cover of vegetation above 10 m | |
Maximum grass foliage cover | Crx | 250 | 2001–2022 | Donohue and Renzullo (2025) | MODIS | Maximum grass foliage cover within an average year (fraction) | Representation of peak grass cover | |
Grass to woody ratio | TG/S | 250 | 2001–2022 | Donohue and Renzullo (2025) | MODIS | Maximum grass foliage cover divided by woody foliage | Identify dominant understorey | |
Building density | D | 30 | 2022 | AHDAP (2022) | Multiple proprietary, super-fine resolution sensors | Building density (ha/ha) | Identify Built-up and WUI fuel types | |
Elevation | E | 30 | 2000 | Farr et al. (2007) | SIR-C | Height of land surface above sea level (m). Used as per source | Identify bare ground fuel type | |
Canopy height | H | 10–30 | 2009 and 2020 | Lang et al. (2023) | Sentinel-2, GEDI-L2, TM, ETM, PALSAR, GLAS-L2/MSI | Top of canopy height (m) | Representation of canopy height | |
Scarth et al. (2019) | ||||||||
Hummock grassland | HG | 250 | 2001–2022 | See main text | MODIS | Distribution of hummock (Spinifex) grassland (a value of 1 denotes hummock grassland) | Identify fuel type | |
Pasture | Ap | 250 | 2016 | ABARES (2021) | NA | Grazing modified pasture [SECV8, 3.2] and grazing irrigated modified pasture [SECV8, 4.2] land uses (a value of 1 denotes pasture). Used as per source | Identify fuel type | |
Plantation broadleaf | Pb | 100 | 2021 | ABARES (2022) | NA | NPI, FOR_TYPE hardwood plantation [FOR_CODE 91] (a value of 1 denotes a broadleaf plantation). Used as per source | Identify fuel type | |
Plantation conifer | Pc | 100 | 2021 | ABARES (2022) | NA | NPI, FOR_TYPE softwood plantation [FOR_CODE 92] (a value of 1 denotes a conifer plantation). Used as per source | Identify fuel type | |
Plantation other | Po | 100 | 2021 | ABARES (2022) | NA | NPI, FOR_TYPE mixed-species plantation [FOR_CODE 93] (a value of 1 denotes other plantation types). Used as per source | Identify fuel type | |
Precipitation | R | 5000 | 1991–2020 | Jones et al. (2009) | NA | Average of total annual precipitation (mm/year). Used as per source | Identify high and low precipitation regions for allocating fuel types to fire models | |
Sedgeland | S | 100 | 2016 | DCCEEW (2020) | NA | Sedgeland vegetation type [NVIS-MVG 21] (a value of 1 denotes sedgeland). Used as per source | Identify fuel type | |
Air temperature | Ta | 5000 | 1991–2020 | Jones et al. (2009) | NA | Average of annual mean temperature (°C). Used as per source | Identify warm and cool regions for allocating fuel types to fire models | |
Wetlands | W | 250 | 2016 | ABARES (2021) | NA | Marsh/wetland land use [SECV8, 6.5] (a value of 1 denotes wetland). Used as per source | Identify fuel type | |
Water cover frequency | Z | 250 | 2001–2022 | Donohue et al. (2022) | MODIS | Average proportion of the year a location is covered in water (%) | Identify fuel type |
Descriptions include data resolution, currency, reference and the purpose of each variable within the workflow. The Satellite sensors field indicates whether a variable has been derived directly from satellite imagery and, if so derived, from which sensor the imagery was acquired (see Table 2 for sensors description). NA denotes not applicable in the table.
Remote sensing
Remote sensing data from multiple space-borne sensors were used to characterise foliage cover and height characteristics, including the vertical arrangement of cover, and its partitioning by vegetation type: woody plants (trees and shrubs) and grasses. Remote sensing was also used to characterise specific arid-region grassland types, such as spinifex (Triodia sp.), as well as water bodies, bare ground and building footprints. The approach integrated data from numerous satellite-based sensors (Table 2).
Sensor type | Satellite platform | Sensor | Spatial resolution (m) | Data currency | Used by and purpose | |
---|---|---|---|---|---|---|
Optical | Landsat-5 onwards | Thematic Mapper (TM), Enhanced TM (ETM) | 30 | 1987–2010 | Used by Scarth et al. (2019) for land use land cover classification | |
Sentinel-2 | Multispectral Instrument (MSI) | 20 | 2019–2020 | Used by Lang et al. (2023) to extrapolate GEDI vegetation height | ||
Terra, Earth Observing System | Moderate Resolution Imaging Spectroradiometer (MODIS) | 250–500 | 2001–2022 | Used to identify woody and grass cover | ||
Radar | Advanced Land Observing Satellite (ALOS) | Phased Array type L-band Synthetic Aperture Radar (PALSAR) | 25 | 2010 | Used by Scarth et al. (2019) for land use land cover classification | |
Shuttle Radar Topographic Mission (SRTM)-Space shuttle Endeavour | Synthetic Aperture Radars (SIR-C) | 30 | 2000 | Used to identify high-elevation regions | ||
Light Detection and Ranging (LiDAR) | Ice, Cloud, and land Elevation Satellite-2 (ICESat-2) | Geoscience Laser Altimeter System L2 (GLAS-L2) | LiDAR scan | 2003–2009 | Used by Scarth et al. (2019) to estimate vertical foliage profiles and height metrics | |
International Space Station (ISS) | Global Ecosystem Dynamics Investigation L2 (GEDI-L2) | LiDAR scan | 2019–2020 | Used by Lang et al. (2023) to estimate vegetation height |
Canopy height information was obtained from Lang et al. (2023), who produced a worldwide canopy-top height layer by combining the sparse height data from the GEDI (Global Ecosystem Dynamics Investigation) space-borne LiDAR sensor with optical data from Sentinel-2’s Multi Spectral Instrument (Tables 1 and 2) through a deep learning model. This layer reports height up to the year 2020. Given the substantial impact of the 2019–2020 south-eastern Australia wildfires, where 24% (6.5 million ha) of the forest ecosystems in NSW and Victoria were impacted (Davey and Sarre 2020), we applied the total canopy height product derived by Scarth et al. (2019) to those 2019–2020 burnt areas. These burned areas were identified using the 500 m resolution MODIS burnt area product (MOD64A1 Collection 6.1; Giglio et al. 2021). The Scarth et al. (2019) height product combined space-borne radar and LiDAR data (from ALOS-PALSAR and ICESat-GLAS) and Landsat-derived Foliage Projective Cover (FPC) to derive vegetation height. Hence, canopy height (H, m) was derived as:
Here, HL and HS are the canopy height data from Lang and Scarth, respectively, and BDOY is the burn date as identified in the MODIS burnt area product (which identifies the day of year of any detected burns). The BDOY values in Eqn 1 denote 1 July 2019 and 31 June 2020.
Data describing total foliage cover – the percentage of ground covered vertically by green (live) foliage – were derived from the MODIS Normalised Difference Vegetation Index (MOD13Q1 Collection 6.1) product (Justice et al. 1998). The MODIS data were converted to total green foliage cover using the method presented in Donohue and Renzullo (2025), which also splits total cover into cover from woody vegetation and grasses. These cover data had a spatial resolution of 250 m and a 16-day time-step. Woody foliage cover (Cp, %) was calculated as the 2001–2022 average of the woody cover data. An important note is that the woody cover data do not discriminate between overstorey and understorey vegetation (such as saplings and shrubs) but represents the combined cover observed by the satellite sensors.
The dataset of Scarth et al. (2019) also describes the foliage cover in four fixed-height layers: 0–5 m (CL1), 5–10 m (CL2), 10–30 m (CL3) and above 30 m (CL4). We used the relative foliage abundances in these height layers to reapportion Cp (woody foliage cover as defined above) into three different height classes: below 5 m (Cp1), above 5 m (Cp2) and above 10 m (Cp3):
where
The Cpi quantities are used to estimate how much of the total woody cover comes from the canopy and how much comes from the understorey layer or layers for different height intervals.
The MODIS-based grass foliage cover, estimated using the method of Donohue and Renzullo (2025), was used to calculate maximum grass cover (Crx, %) as the average intra-annual maximum grass cover over the 2001–2022 period.
Hummock (spinifex) grasslands are a widespread grass type across semi-arid and arid Australia and are characterised by low plant cover. A map of the distribution of these grasslands was generated by combining brown (senesced) cover, soil texture, geology and C4 grass proportion (the ratio of cover from C4 grasses to the cover of all grasses). The mapping process is described in Appendix 1.
Water cover frequency (Z, %) is the average proportion of a year that a location is detected as being covered in water. The average was calculated for the 2001–2022 period. Z was derived using the Alpha-NDVI (Normalized Difference Vegetation Index) algorithm of Donohue et al. (2022) and is derived from the 250 m MODIS NDVI product (MOD13Q1 Collection 6.0).
Building density (D, ha/ha) describes the abundance of buildings per unit ground area, and is used to identify the Built-up and Wildland–urban interface (WUI) fuel types. Building density was derived from the 2020 building footprint polygon layer from National Housing Data Exchange Australia (AHDAP 2022), which integrates data from Microsoft Building Footprints, Bing Maps and OpenStreetMap footprints – all ultimately derived from super-fine resolution satellite imagery. A full description of the derivation of building density is given in Appendix 2.
Bare ground (Cb, %) is the percentage of ground not covered with live or dead vegetation (that is, exposed soil, rock, etc.). This variable was generated using the method of Guerschman and Hill (2018) applied to the 500 m MODIS NDVI product (MCD43A4 Collection 6.0). Cb was calculated as the long-term (2001–2020) average bare ground value.
Existing land use, vegetation and plantation data
Several available data layers were used to identify fuel types and features that could not be discriminated through available remote sensing data. The extent of industrial forest plantations was obtained from the National Plantations Inventory (NPI) (ABARES 2022) dataset. We used land use information from Australian Land Use and Management Classification System (ALUM) version 8 (ABARES 2021) to describe the extent of wetlands and of production agriculture (namely croplands, pasture and horticulture). Information from the National Vegetation Information System (NVIS) version 6 (DCCEEW 2020) major vegetation groups was used to describe the extent of sedgelands. Data currency for plantations is 2021, for land use is 2016 and for native vegetation (NVIS) is 2020.
Fuel type classification rules
Several rulesets were developed to derive the BFC fuel typologies from the underlying vegetation structural information. The rules are applied sequentially. For the top-tier BFC classes (Table 3), the ruleset aims to first classify native vegetation using data from vegetation height, and woody and grass cover. Classification of non-burnable (e.g. water, built-up, wetlands) and agricultural (e.g. horticulture and croplands) areas, which were mostly derived from existing land use databases, was conducted last. For open vegetation systems, such as woodlands and open shrublands, where the drivers of fire propagation are not related to the overstorey component, there is an interest to apply the BFC mid-tier classification that defines the type of understorey fuel. For these open systems, the understorey can be shrub, grass, spinifex or sparse (Sullivan et al. 2012). The BFC mid-tier ruleset is shown in Table 4 and is applied only to the pixels previously classified as woodland, low woodland or open shrubland.
BFC fuel type | Ruleset | |
---|---|---|
Sparse grassland | 0 < Crx ≤ 10 | |
Open grassland | 10 < Crx ≤ 30 | |
Grassland | Crx > 30 OR | |
Ap = 1 | ||
Hummock grassland | HG = 1 | |
Open shrubland | 0 < H ≤ 2 AND | |
5 < Cp ≤ 30 | ||
Shrubland | 0 < H ≤ 2 AND | |
Cp > 30 | ||
Tall shrubland | 2 < H ≤ 5 AND | |
Cp > 30 | ||
Low woodland | (5 < H ≤ 10 AND 5 < Cp2 ≤ 30) OR | |
(5 < H ≤ 10 AND 10 < Cp ≤ 15) | ||
Woodland | (10 < H ≤ 20 AND 5 < Cp2 ≤ 30) OR | |
(20 < H ≤ 30 AND 5 < Cp3 ≤ 30) | ||
Tall woodland | H > 30 AND | |
5 < Cp3 ≤ 30 | ||
Low open forest | 5 < H ≤ 10 AND | |
30 <Cp2 ≤ 75 | ||
Open forest | (10 < H ≤ 20 AND 30 < Cp2 ≤ 75) OR | |
(20 < H ≤ 30 AND 30 < Cp3 ≤ 75) | ||
Tall open forest | H > 30 AND 30 > Cp3 ≤ 75 | |
Closed forest | (5 < H ≤ 20 AND Cp2 > 75) OR | |
(20 < H ≤ 30 AND Cp3 > 75) OR | ||
(5 < H ≤ 30 AND Cp > 95) | ||
Tall, closed forest | (H < 30 AND Cp3 > 75) OR | |
(H < 30 AND Cp > 95) | ||
Wetlands | W = 1 AND | |
20 < Z ≤ 65 | ||
Horticulture | Ah = 1 | |
Croplands | Ac = 1 | |
Sedgeland | S = 1 AND | |
R > 600 | ||
Bare ground | Cb > 25 AND | |
E ≤ 1000 | ||
Other plantation | Po = 1 | |
Conifer plantation | Pc = 1 | |
Broad leaf plantation | Pb = 1 | |
Built-up | D > 0.125 | |
WUI 3 | 0.025 < D ≤ 0.125 | |
WUI 2 | 0.0025 < D ≤ 0.025 | |
WUI 1 | 0 < D ≤ 0.0025 | |
Permanent water | Z > 65 |
Rulesets are applied sequentially from the top such that any rule overrides the previous one. See Table 1 for variable definitions.
BFC fuel type | Ruleset | |
---|---|---|
Open shrubland with grassy understorey | Crx > 30 AND | |
0 < H ≤ 2 AND | ||
5 < Cp ≤ 30 | ||
Open shrubland with spinifex understorey | HG = 1 AND | |
0 < H ≤ 2 AND | ||
5 < Cp ≤ 30 | ||
Open shrubland with sparse understorey | Not in any of the above Open shrubland categories AND | |
0 < H ≤ 2 AND | ||
5 < Cp ≤ 30 | ||
Low woodland with grassy understorey | (Crx + Cp1) > 25 AND | |
(Crx/Cp1) > TG/S AND | ||
(5 < H ≤ 10 AND 5 < Cp2 ≤ 30) OR | ||
(5 < H ≤ 10 AND 10 < Cp ≤ 15)) | ||
Low woodland with shrubby understorey | (Crx + Cp1) > 25 AND | |
(Crx/Cp1) ≤ TG/S AND | ||
((5 < H ≤ 10 AND 5 < Cp2 ≤ 30) OR | ||
(5 < H ≤ 10 AND 10 < Cp ≤ 15)) | ||
Low woodland with spinifex understorey | HG = 1 AND | |
((5 < H ≤ 10 AND 5 < Cp2 ≤ 30) OR | ||
(5 < H ≤ 10 AND 10 < Cp ≤ 15)) | ||
Low woodland with sparse understorey | Not in any of the above Low woodland categories AND | |
((5 < H ≤ 10 AND 5 < Cp2 ≤ 30) OR | ||
(5 < H ≤ 10 AND 10 < Cp ≤ 15)) | ||
Woodland with grassy understorey | (Crx + Cp1) > 25 AND | |
(Crx/Cp1) > TG/S AND | ||
((10 < H ≤ 20 AND (5 < Cp2 ≤ 30) OR | ||
(20 < H ≤ 30 AND 5 < Cp3 ≤ 30)) | ||
Woodland with shrubby understorey | (Crx + Cp1) > 25 AND | |
(Crx/Cp1) ≤ TG/S AND | ||
((10 < H ≤ 20 AND 5 < Cp2 ≤ 30) OR | ||
(20 < H ≤ 30 AND 5 < Cp3 ≤ 30)) | ||
Woodland with spinifex understorey | HG = 1 AND | |
((10 < H ≤ 20 AND 5 < Cp2 ≤ 30) OR | ||
(20 < H ≤ 30 AND 5 < Cp3 ≤ 30)) | ||
Woodland with sparse understorey | Not in any of the above Woodland categories AND | |
((10 < H ≤ 20 AND 5 < Cp2 ≤ 30) OR | ||
(20 < H ≤ 30 AND 5 < Cp3 ≤ 30)) | ||
Tall woodland with grassy understorey | H > 30 AND | |
5 < Cp3 ≤ 30 |
Rulesets are applied sequentially from the top such that any rule overrides the previous one. See Table 1 for variable definitions.
TG/S is dynamic and varies with precipitation (TG/S = 3 if R ≤ 500 and 2 otherwise).
Validation of fuel type classification
Evaluation of the generated BFC fuel type layer was undertaken using existing field data obtained from several sources (Table 5 and Fig. 2). Field data for forests were largely obtained from published studies that reported vegetation type, plot location and, in some cases, forest structural variables such as overstorey cover and height. Overall, a total of eight studies containing forest information were selected for the evaluation, comprising 469 field plot locations. Not all these studies explicitly reported canopy height and cover, which are required to define the BFC type (as per Table 3). Some studies only provided environmental or ecological forest type descriptors (such as dry forest or wet sclerophyll forest). For such cases the height and/or cover of the forest had to be inferred from the given descriptions. Given the subjectivity introduced by inferring structure, we further separated the forest evaluation data into those reporting structure (n = 331) and those where structure was inferred (n = 138).
Source | Number of observations | Vegetation type as described in study | |
---|---|---|---|
Forest environment with reported forest structure | |||
Burrows et al. (2023) | 70 | Tall open wet forest | |
Cawson et al. (2018) | 98 | Wet forest | |
Hollis (unpublished)A | 125 | Open forest | |
Furlaud et al. (2021a) | 38 | Tall wet forest | |
Forest environment with inferred forest structure | |||
McCaw (2011) | 67 | Open forest | |
Furlaud et al. (2021b) | 17 | Tall wet forest | |
Slijepcevic et al. (2018) | 7 | Forest (wet, moist, dry) | |
Sparrow et al. (2021) | 47 | Forest – rainforest, wet, open | |
Non-forest environment | |||
Sparrow et al. (2021) | 282 | Woodland, shrubland, grasslands |
For non-forest sites, we used information from the Terrestrial Ecosystem Research Network (TERN) Ecosystem Surveillance AusPlots network (Sparrow et al. 2021). TERN AusPlots is a national network for native vegetation monitoring plots. We used the structural and floristic description of the vegetation, plus photographic records to classify each plot according to the most suitable BFC fuel type. Only plots with cover and height data were selected for evaluation, for a total of 282 locations.
Assessment of the BFC fuel type classification scheme was performed through the calculation of overall accuracy when comparing ground-observations with the algorithm-classified fuel types. Analysis of contingency tables was also used to understand patterns in classification error. Given the lack of detail in some of the forest data, for the evaluation process ‘Tall forest’ incorporates ‘Tall open forest’ and ‘Tall closed forest’, and ‘Open forest’ contains ‘Open forest’ and ‘Low open forest’.
Results
Native vegetation structural properties
The first step of the process of classifying vegetation into structurally defined fuel types was to spatially estimate the underlying vegetation structural attributes. The derived woody cover profile defines a three-dimensional vegetation distribution (Fig. 3a), which, when combined with grass cover (Fig. 3b) and woody vegetation canopy height (Fig. 3c), allows the definition of the dominant fuel type and understorey fuels (Fig. 3d).
Variation of vegetation structural characteristics along a 55-km long transect (black line) across the Australia Capital Territory (boundary in yellow). (a) Woody vegetation cover vertical profile along the transect, grouped in three height segments: <5, 5–10 and >10 m; (b, c) grass cover and canopy height, respectively, along the transect and (d) major vegetation formations and urban areas around the ACT. The western end of the transect has coordinates 35°29.483′S and 148°43.088′E and with the eastern end being located at 35°29.444′S and 149°15.510′E.
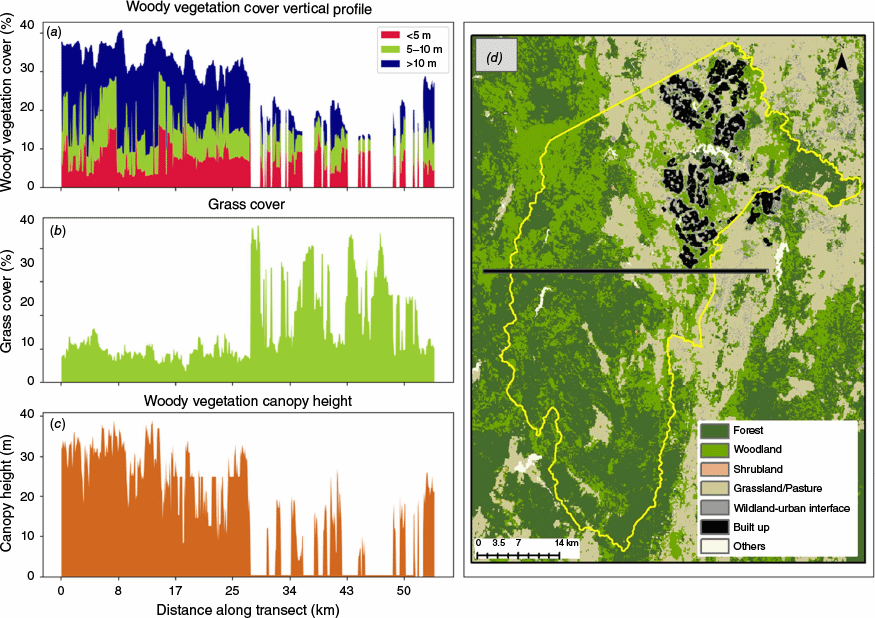
Fig. 3d illustrates how the approach captures the variability in fuel type across a 55 km long transect in south-eastern Australia covering a gradient of productivity and land use, with open forests (characterised by high woody cover, tall vegetation and relatively low grass cover) in the west of the transect transitioning to more open woodlands and pastures in the east (relatively high grass cover and discontinuous woody vegetation patches).
For open fuel types, such as woodland and open shrublands, the analysis aimed to produce mid-tier fuel types that identify the understorey fuel responsible for fire propagation: shrubby, grassy or hummock grass. Fig. 4c–e illustrates the process and the data layers used in identifying the woodland mid-tier understorey type along a 487 km transect in southern Western Australia (Fig. 4a, b). The analysis identified areas with overstorey cover varying between 5 and 30% and height above 5 m as woodland/low woodland (as per Table 3 ruleset). Within these areas, the cover of understorey vegetation (e.g. shrub (0–5 m height) as defined by Specht and Specht (1999), grass or spinifex) are weighted (as per Table 4 ruleset) to define the relevant understorey vegetation (Fig. 4).
Variation in the understorey of woodland fuel types along a 490-km long transect (black line in b) in Western Australia. (a) shows the distribution of Woodland and Low woodland BFC top‐tier fuel types across Australia, with the rectangle delineating the region represented in panel (b). (b) illustrates Woodland and Low woodland BFC mid‐tier fuel types in southern Western Australia, corresponding to the area highlighted in panel (a). (c, d) depict the overstorey and understorey cover profiles across the transect, respectively. (e) displaye the grass/shrub cover ratio along the transect, and (f) shows the derived mid-tier woodland fuel types across transect (legends are shown below plot (a)). The western end of the transect has coordinates 31°14.095′S and 119°18.586′E with the eastern end being located at 31°2.115′S and 124°21.160′E.
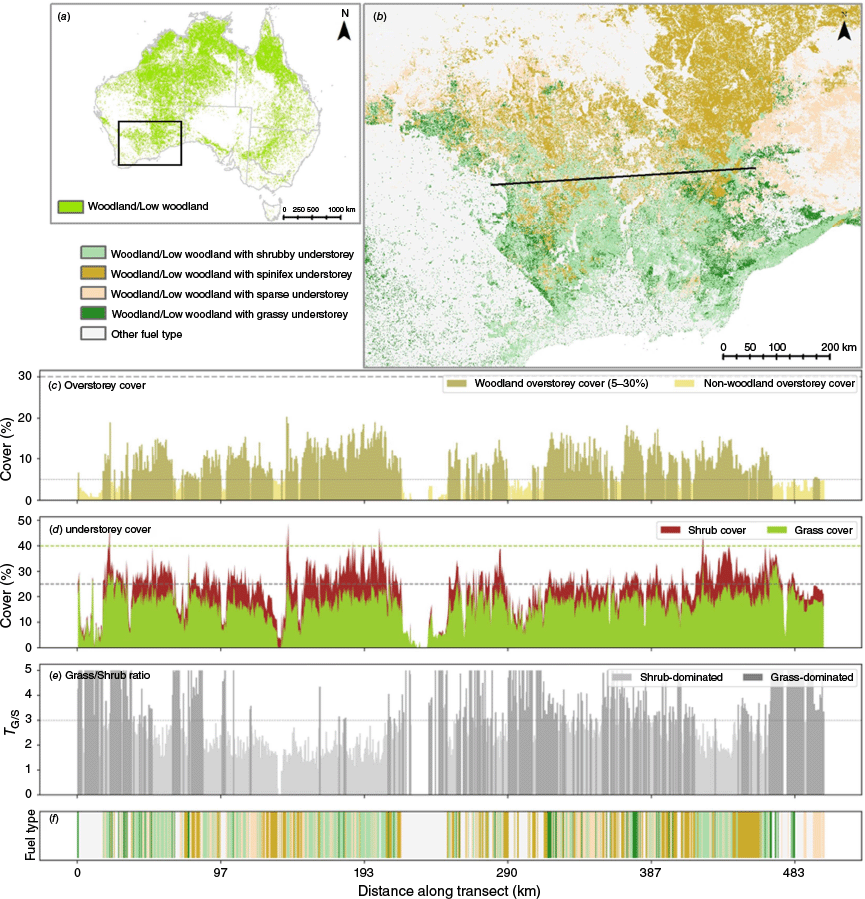
A simplified BFC framework
In implementing the BFC framework of Cruz et al. (2018), several modifications of the original framework became necessary, resulting in the simplified BFC framework shown in Fig. 5. Simplifications of the framework were made by aggregating a number of similar fuel type classes into one class. This was done partially because the remote sensing methods used could not provide the precision needed to differentiate between the structural characteristics of some adjacent classes. For example, we could not identify heights of shrubs lower than 2 m, so low shrublands and shrublands were fused into a single fuel type – Shrublands. It was noticed that there was likely too much detail and an inherent redundancy in the original fuel type classification, meaning – for practical purposes – some vegetation differences did not warrant separation into distinct fuel types. For the top-tier classification, we now use four aggregate fuel types, namely Closed forest, Low woodland, Open shrubland and Shrubland; each previously comprised two fuel types (Fig. 5). The fuel type map is shown in Fig. 6.
The Bushfire Fuel Classification top-tier fuel types as used in this study. Four fuel types were aggregated in comparison with the original Cruz et al. (2018a) top-tier fuel type matrix.
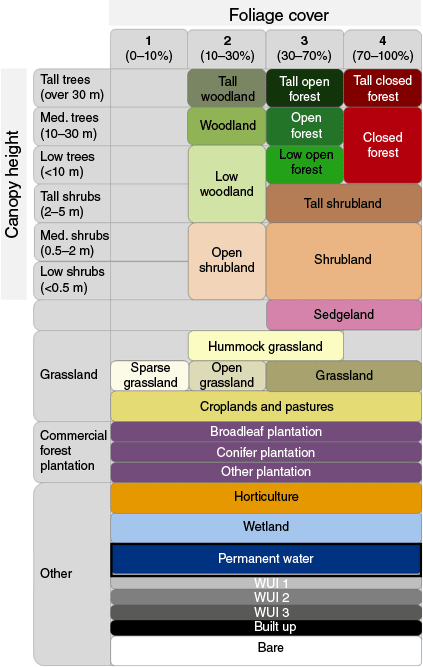
Australia-wide fuel types generated using the Bushfire Fuel Classification fuel typology. This shows the simplified top-tier BFC fuel types.
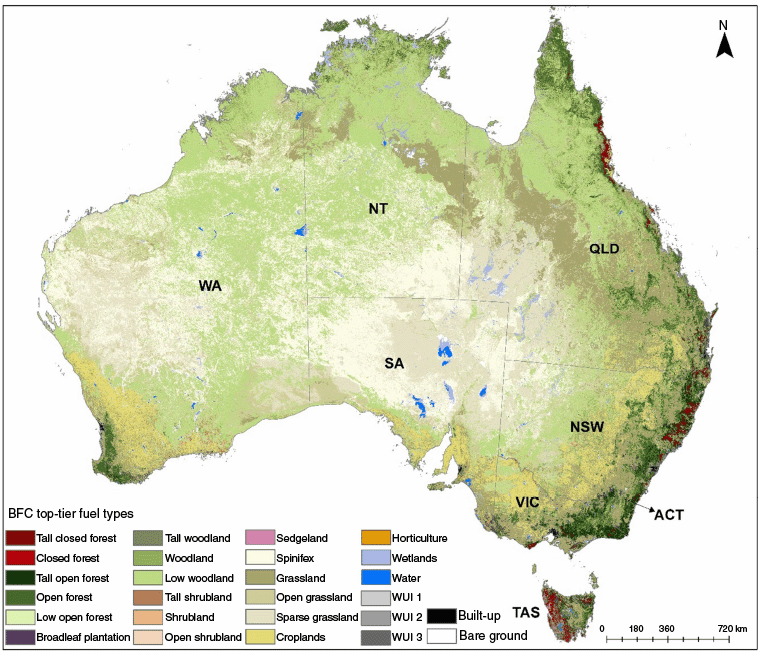
Table 6 summarises the relative areas of BFC (top-tier) fuel types for each Australian state and territory. The result shows that the distribution of fuel types varies substantially between the states. The relatively small jurisdictions of Victoria, Tasmania and the ACT have the highest proportion of forests. In contrast, the large jurisdictions of Western Australia, Northern Territory, South Australia and Queensland have the largest proportion of arid fuel types, such as hummock grasslands and open/sparse grasslands. The areal distributions of the mid-tier BFC types are shown in Table 7.
ACT | NSW | NT | Qld | SA | Tas | Vic | WA | ||
---|---|---|---|---|---|---|---|---|---|
Forests | |||||||||
Tall closed forest | NA | 1.3 | NA | 0.4 | NA | 7.0 | 0.8 | 0.0 | |
Closed forest | NA | 0.1 | 0.0 | 0.2 | 0.0 | 7.5 | 0.1 | 0.0 | |
Tall open forest | 3.9 | 4.0 | 0.0 | 0.3 | 0.0 | 8.2 | 9.4 | 0.0 | |
Open forest | 32.7 | 9.6 | 2.3 | 7.7 | 0.3 | 29.9 | 12.2 | 1.1 | |
Low open forest | 1.3 | 0.3 | 0.2 | 0.3 | 0.2 | 0.8 | 0.5 | 0.2 | |
Broadleaf plantation | NA | 0.1 | 0.0 | 0.0 | 0.0 | 2.9 | 0.7 | 0.1 | |
Conifer plantation | 3.1 | 0.4 | 0.0 | 0.1 | 0.1 | 1.1 | 0.9 | 0.0 | |
Other plantation | NA | NA | NA | NA | NA | 0.0 | 0.0 | 0.0 | |
Woodlands | |||||||||
Woodland | 25.6 | 6.5 | 4.5 | 8.8 | 0.3 | 4.8 | 6.8 | 1.5 | |
Low woodland | 4.0 | 21.0 | 48.4 | 25.2 | 11.4 | 0.3 | 7.9 | 39.5 | |
Shrublands | |||||||||
Tall shrubland | 0.3 | 0.4 | 0.4 | 0.4 | 0.1 | 0.1 | 0.4 | 0.5 | |
Shrubland | 0.1 | 0.2 | 0.4 | 0.3 | 0.1 | 0.0 | 0.0 | 0.3 | |
Open shrubland | 0.1 | 2.9 | 1.9 | 2.5 | 0.9 | 0.0 | 1.4 | 2.0 | |
Open Grasslands | |||||||||
Hummock grassland | NA | 6.8 | 25.3 | 4.3 | 36.0 | NA | 0.1 | 26.9 | |
Grassland | 18.4 | 19.8 | 8.2 | 25.2 | 4.9 | 23.1 | 31.4 | 3.7 | |
Open grassland | 0.0 | 2.8 | 3.0 | 4.0 | 5.0 | 0.0 | 1.1 | 7.0 | |
Sparse grassland | 0.0 | 8.8 | 1.9 | 14.5 | 31.3 | 0.0 | 0.3 | 11.4 | |
Sedgeland | 0.0 | 0.0 | 0.1 | 0.0 | 7.3 | 0.1 | 0.0 | ||
Agricultural – burnable | |||||||||
Croplands | 0.0 | 12.2 | 0.0 | 1.9 | 5.1 | 0.9 | 20.0 | 4.4 | |
Horticulture | NA | 0.1 | 0.0 | 0.0 | 0.1 | 0.0 | 0.5 | 0.0 | |
Other – non-burnable areas | |||||||||
Wetlands | 0.2 | 1.0 | 2.7 | 2.4 | 1.6 | 1.3 | 0.7 | 0.4 | |
Water | 0.1 | 0.1 | 0.1 | 0.1 | 0.9 | 1.5 | 0.4 | 0.3 | |
Wildland–urban interface | 3.9 | 0.9 | 0.0 | 0.3 | 0.2 | 1.6 | 2.4 | 0.1 | |
Built-up | 6.0 | 0.2 | 0.0 | 0.1 | 0.1 | 0.2 | 0.8 | 0.0 | |
Bare ground | 0.5 | 0.7 | 0.6 | 0.6 | 1.3 | 0.9 | 0.8 | 0.6 | |
Jurisdiction area (1000 km2) | |||||||||
2.4 | 800.6 | 1346.3 | 1729.9 | 983.1 | 68.2 | 227.2 | 2525.1 |
Bold numbers indicate the three highest percentage areas by state or territory. A value of 0.0 indicates the fuel type is present but occupies less than 0.05% of the jurisdiction area. Absence of a value indicates absence of fuel type in the jurisdiction. ACT, Australian Capital Territory; NSW, New South Wales; NT, Northern Territory; Qld, Queensland; SA, South Australia; Tas, Tasmania, Vic, Victoria; WA, Western Australia.
ACT | NSW | NT | Qld | SA | Tas | Vic | WA | ||
---|---|---|---|---|---|---|---|---|---|
Woodlands | |||||||||
Tall woodland with shrubby understorey | 4.1 | 0.2 | 0.0 | 0.0 | 0.0 | 0.7 | 1.0 | 0.0 | |
Woodland with shrubby understorey | 16.8 | 3.3 | 0.4 | 1.6 | 0.1 | 3.1 | 3.3 | 0.3 | |
Woodland with spinifex understorey | NA | 0.0 | 0.0 | 0.0 | 0.0 | NA | 0.0 | 0.1 | |
Woodland with grassy understorey | 4.2 | 1.7 | 3.8 | 6.3 | 0.2 | 0.7 | 2.5 | 1.0 | |
Woodland with sparse understorey | 0.6 | 1.3 | 0.3 | 1.0 | 0.0 | 0.4 | 0.1 | 0.2 | |
Low woodland with shrubby understorey | 2.8 | 4.4 | 1.4 | 2.0 | 1.4 | 0.2 | 4.9 | 3.4 | |
Low woodland with spinifex understorey | NA | 4.6 | 25.2 | 2.0 | 5.4 | NA | 0.5 | 24.4 | |
Low woodland with grassy understorey | 1.1 | 2.4 | 19.2 | 14.5 | 2.2 | 0.1 | 2.0 | 6.1 | |
Low woodland with sparse understorey | 0.1 | 9.5 | 2.7 | 6.7 | 2.5 | 0.0 | 0.6 | 5.7 | |
Shrublands | |||||||||
Open shrubland with spinifex understorey | NA | 0.3 | 0.5 | 0.1 | 0.1 | NA | 0.0 | 0.5 | |
Open shrubland with grassy understorey | 0.0 | 0.1 | 0.0 | 0.0 | 0.0 | 0.0 | 0.0 | 0.0 | |
Open shrubland with sparse understorey | 0.1 | 2.5 | 1.4 | 2.4 | 0.8 | 0.0 | 1.3 | 1.5 |
A value of 0.0 indicates the fuel type is present but occupies less than 0.05% of the jurisdiction area. NA denotes absence of that specific fuel type in the jurisdiction. ACT, Australian Capital Territory; NSW, New South Wales; NT, Northern Territory; Qld, Queensland; SA, South Australia; Tas, Tasmania, Vic, Victoria; WA, Western Australia.
The top-tier fuel typologies allow fire behaviour models to be assigned for all fuel types except the open woody vegetation systems, such as woodlands and open shrublands. In these open woody systems, the mid-tier BFC types provides the extra information required to allocate fuel behaviour models. However, although the current BFC fuel type mapping can be used to determine which fire spread model should be used at a given location (Appendix 3), it does not provide the fuel parameter values also required by the models. Further modelling is required to generate these parameters, which is commonly done using the fuel types as a framework.
Evaluation of fuel type classification
The forest field data were separated into sites that explicitly reported structure and those for which structure had to be inferred. For the former (n = 331), the BFC classification produced an overall accuracy of 89% (293 correctly classified cases out of 331), with study-specific accuracies varying between 81 and 95% (Fig. 7a). For these sites, misclassification of fuel types was more commonly associated with errors in estimating vegetation height (e.g. a tall open forest being classified as an open forest owingto underprediction of forest height) rather than cover (e.g. an open forest being classified as a woodland). Only 7 out of the 331 forest validation sites were misclassified as non-forest, and all of these were labelled as Woodland (which is only one cover class away from a forest type; see Fig. 5).
Contingency table for fuel type classification accuracy for forest data. (a) Studies with known forest cover and height. (b) Studies with unknown forest structure but with broad vegetation description given. The percentage number above each contingency table reports overall classification accuracy.
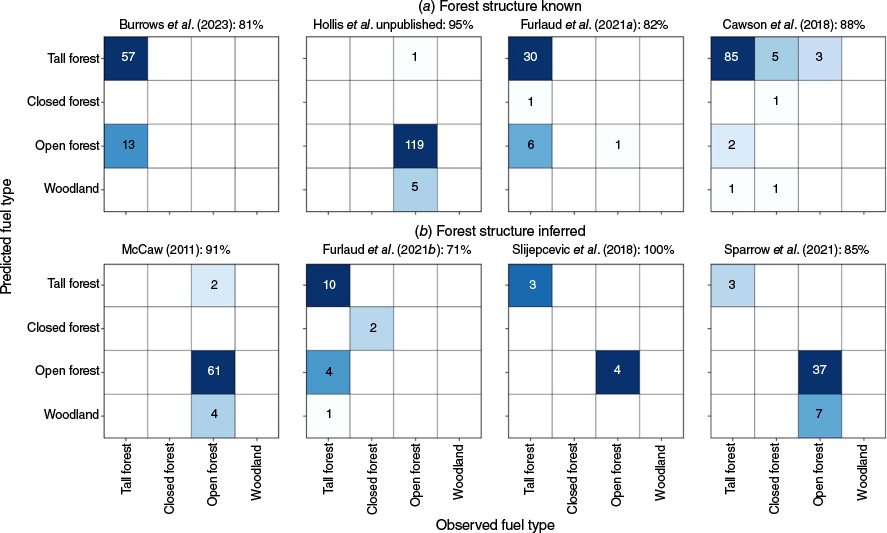
The overall accuracy for the sites where forest structure was assumed (n = 138) was 87% (120 cases classified correctly out of 138), ranging from 71 and 100% for individual studies (Fig. 7b). Specifically, the accuracy was 76% for tall forest (n = 21), 100% for closed forest (n = 2) and 89% for open forest (n = 115). This 89% accuracy for open forests contrasts with 94% accuracy when the evaluation is against data with measured forest structure rather than inferred, highlighting the uncertainty introduced when making assumptions about forest structure. It is noted that the Tall forest and Open forest categories included two BFC fuel types each (see description of evaluation methods), which may artificially increase the accuracy of the classification.
The fuel type classification algorithm classified correctly 71% of the TERN non-forest plots (n = 282). Most misclassifications were in the arid systems with open shrublands, shrublands and woodlands being mapped as grasslands and hummock grasslands (Fig. 8). Importantly, the errors tended to be largely within one fuel type class, e.g. underestimation of height leads to a woodland being predicted as open shrublands, or overestimation of cover leads to woodland being predicted as forest. Approximately 7% of the woodland observations were mapped as forest. The system showed good discrimination between hummock grasslands and grasslands. A combined evaluation metrics of forest and non-forest sites is shown in Fig. S1.
Discussion
The value of a remote-sensing approach to mapping fuel types
In Australian fire management reality, a practitioner aiming to estimate fire risk or behaviour potential for a given situation or location can be confronted with at least three distinct fuel classification schemes, with conflicting fuel types and fire behaviour potentials. The existence and adoption of nationally consistent and periodically updated fuel type data are needed for correspondingly consistent national-scale fire danger forecasting and local fire behaviour prediction and risk assessment. Accuracy in fuel type data is also critical, as errors due to misclassification of fuel type lead to the wrong fire spread model being applied, resulting in errors several times larger than errors associated with incorrect fire spread model fuel inputs.
The approach used herein to develop up-to-date, national fuel layers brings together distinct space-borne satellite sensor types (multispectral, LiDAR, radar) with pre-existing geospatial data. Our approach aimed to minimise the use of pre-existing information (usually pre-mapped, ecologically based vegetation types); such data were only used where specific floristics and growth forms could not be determined from remote sensed products (such as the Sedgeland fuel type), or for specific land uses (such as Horticulture).
The remote sensing basis of the fuel type layers ensures consistency across Australia, something that is not present in current vegetation and fuel type maps (e.g. Matthews et al. 2019), which contain drastic changes in fuel types across jurisdictional borders. Our modular approach also enables continuous updates that account for changes in fuel types due to events such as land clearing, tree and shrub encroachment into grasslands and the occurrence of high intensity, stand-replacing fires. For such continuous updates to be possible, all inputs need to be available at an annual time step. This is already true for woody and grass vegetation cover (e.g. Donohue and Renzullo 2025), burn area (e.g. Giglio et al. 2021) and burn severity (e.g. Gibson et al. 2020) mapping. The absence of annual height data is a current limitation.
The study results also highlights the value of combining multiple types of remotely sensed data to better describe fuel structure, especially the differentiation between grass, shrub and hummock (spinifex) understories in open woody systems. This is essential information for the accurate use of fire behaviour models (Cruz et al. 2015a). Such integration of diverse and new sensor technologies is an active area of research in the vegetation mapping community. Numerous recent studies have combined optical imagery, radar imagery and/or LiDAR data to generate improved fuel type maps, commonly with some machine learning component. For example, space-borne LiDAR data, such as from GEDI, have recently been utilised in mapping vegetation vertical profiles (Wang et al. 2024). Similarly, several studies have integrated vegetation phenological signatures from optical and radar satellite imagery with GEDI-based height information to estimate structural and floristic attributes (Schlund et al. 2023; Burns et al. 2024). Mihajlovski et al. (2023) demonstrated that combining airborne LiDAR with optical and SAR (Synthetic Aperture Radar) data significantly improves fuel classification in Mediterranean forests. At a regional scale, Chrysafis et al. (2023) employed fused Sentinel-1, Sentinel-2 and DEM (Digital Elevation Model) derivatives to classify fuel types, highlighting the value of SAR and topographic information. Earlier work by García et al. (2011) integrated multispectral imagery with airborne LiDAR using (Support Vector Machine) SVM to map fuel types with enhanced accuracy. Similarly, Domingo et al. (2020) showed that fusing Sentinel-2 and (Airborne Laser Scanner) ALS data improved post-fire fuel type classification in Mediterranean regions, emphasising the importance of structural and spectral information. Most of these models, however, are fuel type-specific or have been regionally trained, and so are difficult to incorporate into an Australian fuel type model where diverse fuel types exist and training data are sparse. The value of the method presented in our work is that it is generic within the Australian context (not restricted to any particular fuel type), requires no training or calibration, and represents one, nationally consistent method.
We intentionally mapped the primary vegetation structural attributes (from which fuel type classes can be derived), rather than mapping fuel types directly. Additionally, we also used a physically based ruleset for combining structural attributes. This approach, only made possible with remote sensing data, has four main advantages. The first is that any structurally based fuel type typology can be populated, as we have done here with the BFC layer. To populate structural-floristic typologies – such as the AFDRS – requires the addition of floristic data layers (which are far more challenging to derive using satellite imagery). Second, nuanced variations in classifications can be easily implemented for specific purposes – for example, the cover threshold that divides woodlands and open forests can be changed to better fit local needs and expectations. Third, fuel type maps can be updated to incorporate changes in structure caused by land use change (e.g. forest or woodland clearing for pasture or cropland) and disturbance–regrowth cycles. Fourth and finally, this approach, being based on physical rulesets, provides a methodological transparency useful for decoding model error sources. This contrasts with currently popular approaches that rely on various forms of machine learning, which mask links between variables. Such models are yet to routinely deliver more accurate results than statistically simpler and logical rule-based models (e.g. López-De-Castro et al. 2022; Alipour et al. 2023; Sismanis et al. 2023). The accuracy of such machine learning approaches is inherently limited by the quality and quantity of training data, and model applicability is generally limited to the spatial and temporal extents of the training data (Hengl et al. 2018; Nikparvar and Thill 2021).
The BFC fuel type layer
The BFC framework provides a fine level of spatial and structural detail, as demonstrated by the variation evident in fuel types along the transects in Figs 3 and 4. The approach of using remotely sensed structural data to populate the BFC layer means we inherently capture the landscape-scale variation in fuels caused by soils, topography, drainage, disturbances and land management. The ability to identify the understorey fuels responsible for fire propagation in woodland and shrubland systems ensures appropriate fire behaviour models are applied to these vegetation types. For example, fire spread predictions for a woodland with a shrub understorey will rely on the generic fire spread model of Anderson et al. (2015) for shrubland fuels, whereas for a woodland with a spinifex understorey, the most appropriate model is that developed by Burrows et al. (2018). Although no fuel characteristics are associated with the fuel types in the current description of the BFC, there is a link between BFC fuel types and associated fire behaviour models (see Appendix 3). This association determines which fuel characteristics need to be estimated to apply the relevant fire behaviour models.
Another strength of the BFC framework is that it is based on the widely used vegetation classification scheme of Specht (1970). This maximises the relevance of the fuel type layers – as well as the underlying structural layers – to other applications. One example is linking fire behaviour modelling with biodiversity and ecological modelling (Loudermilk et al. 2022). Another example relates to land surface parameterisations within Earth system models (ESMs). These generally represent vegetation using Euro-centric categories, such as deciduous forest and needle-leaf forest (e.g. Clark et al. 2011; Haverd et al. 2018). Being physically based, the BFC fuel types (or at least their underlying structural data) can provide the basis for improving vegetation parameterisations of the various ESMs that are used to predict the effects of climate change in Australia. This would provide greater consistency between fire and climate modelling systems, improving interoperability, and would arguably improve predictions of the multiple feedbacks between vegetation, fire and climate. One further strength of the BFC typology is that it is equivalent to the AS3959 fuel classification currently used by Australian fire agencies and private industry to guide the construction of buildings in bushfire-prone areas.
Herein, we applied a slightly revised version of the original BFC top-tier fuel types (see Fig. 5, Cruz et al. 2018). This was done for two main reasons: (i) the inability of current remote sensing products to discern differences in low woody vegetation heights; and (ii) because it was felt that there was some redundancy in a number of fuel types. In this revision (see Fig. 5), low closed forest and closed forest were combined into Closed forest, whereas low woodland and tall open shrublands formed Low woodland. Tall shrubland and tall closed shrublands were merged into Tall shrubland and an Other plantation class was added. Shrublands and low shrublands were integrated into Shrublands and the Sparse shrublands class was removed. Hummock grassland became a single class, and Grassland and Closed grassland were merged. We advocate that the new suggested fuel type definitions be adopted in future work.
Model error and future improvements
The remotely sensed height and foliage cover data used herein have known limitations. It is acknowledged that the GEDI product (Lang et al. 2023) is unable to provide estimates of woody vegetation height below 2 m, and there are substantial uncertainties in the estimation of height between 2 and 5 m. This limited the classification of shrub fuel types and low woodlands, and is one of the reasons why we pooled the Tall open shrubland and Low woodland into a single fuel type – Low woodland. Research into canopy height mapping is advancing quickly owing to the increasing availability of LiDAR data and machine learning techniques, and so this limitation is expected to ease in the near future.
There are three known limitations in the MODIS-based foliage cover data used herein. Firstly, the foliage cover of pure grasslands is systematically underestimated (Donohue and Renzullo 2025). Although this does not affect the ability to differentiate between woody and grass vegetation types, it means that some Grassland areas are likely to be incorrectly mapped as Open grasslands (Fig. 5) owing to this bias. Secondly, the nature of cover errors in sparsely vegetated systems means that even small absolute errors equate to large relative errors. This makes it difficult to accurately differentiate between grassy and woody fuel types when total woody foliage cover varies between 5 and 10%. Thirdly, estimates of woody foliage cover do not differentiate between canopy and understorey foliage. For this, we used the modelled vegetation height-cover profiles of Scarth et al. (2019), meaning that the accuracy of our BFC classification (especially the mid-tier classes) is partly determined by the accuracy of that height-cover product.
The evaluation results obtained for the BFC fuel typology against independent data, namely ~89% for native forest fuel types and 71% for other fuel types, are encouraging, especially when compared with other published results for the accuracies of large-scale fuel type maps (e.g. Rollins et al. 2004; Krasnow et al. 2009; Keane et al. 2013). It is important to note that the evaluation data focus on native vegetation, in particular forests and woodlands where the system is working best. We note that the lower and sparser the vegetation, the higher the uncertainty in the remote sensing data. The evaluation data do not adequately cover all fuel types, and so it is unclear how well the fuel type identification ruleset captures some of the fuel types, such as low shrubs. It is expected that the fuel types derived from NVIS and ALUM layers will have a higher level of uncertainty than that obtained for native vegetation fuel types mapped from remote sensing structural data.
A known limitation of our fuel type layers is that they comprise source data with varying spatial resolutions, ranging from 30 m up to 250 m for vegetation and 500 m for bare ground. Coarse-resolution satellite data are no less accurate per se than fine-resolution data for vegetation and fuel type mapping over homogeneously vegetated areas (in fact, fine-resolution imagery can become less accurate if the resolution is below the minimum mapping scales embedded in the conceptualisation of vegetation and fuel type classes). It is when the boundary locations between fuel types are required with high precision, such as within WUI areas, that the multi-scale nature of our products becomes limiting. It is also important when assessing fire risk for or planning fire operations around sensitive landscape features, such as fire-sensitive vegetation patches. Hence, a key enhancement of the method presented here is to generate foliage cover (and other cover-related variables) at 30 m resolution using a combination of Landsat and Sentinel-2 imagery.
Aside from addressing the above-mentioned limitations, arguably the next advance needed in Australian fuel type mapping relates to understorey structure. Here, we only mapped woodland and open shrubland understorey and only using four basic types – shrub, grass, hummock and ‘sparse’ (this, however, is a novel advance in itself). Ideally, understorey mapping would extend to forests and would be mapped in terms of the number of layers present, and their height and cover. These attributes can be mapped from airborne LiDAR (e.g. García et al. 2011 and Marino et al. 2016) – but, being from an airborne source, these data are only available over limited areas, especially in the Australian context. They can also be mapped from satellite LiDAR – but only at points across the continent. The greatest potential lies in a model that combines these two sources with other satellite imagery and environmental layers into a biophysical modelling framework (such as the ecohydrological equilibrium framework of Specht (1972)).
Comparison with Australia’s contemporary fuel type mapping
The fuel typology currently used operationally by the states and territories in Australia for fire danger rating is the AFDRS typology. This was initially developed from a combination of NVIS (for native vegetation) and land use (for non-native vegetation) spatial datasets for much of Australia with more detailed state and territory data used for specific fuel types, such as forests (Matthews et al. 2019). Hence, this is a structural-floristic typology. To date, floristics information (vegetation species and associations) cannot be routinely delineated from satellite imagery nationally, and so (for now), the AFDRS system is dependent on traditional state-level vegetation type mapping. This has a cascade of implications. One is that the quality of the fuel type mapping is largely determined by that of the underlying vegetation mapping – which is highly variable in its accuracy, consistency and currency at the national scale, as well as at the local scale over the more remote regions of Australia. As traditional mapping programs are expensive, neither the vegetation type maps themselves, nor the techniques used to derive them, are updated frequently. Similarly, until space-based detection of floristics becomes mainstream, advances in satellite-based vegetation mapping are difficult to incorporate into the AFDRS system. This means, among other things, that the advantages of the potentially high latency of satellite products cannot be realised within the system. If the fundamental fuel structural attributes could be perfectly quantified, there would be no need for fuel typologies to incorporate floristic information (as floristics serve only as proxies for the multiple effects of underlying structural attributes associated with specific vegetation and fuel assemblages). Ideally, Australia’s operational fuel typology needs to be flexible enough to accommodate potential future improvements in satellite-based (and airborne-based) vegetation structural mapping, which will potentially require a transition away from a floristic-based typology.
Concluding remarks
The methods presented here represent a new approach to fuel type mapping in Australia. In developing these methods, we prioritised the use of satellite imagery and focused on mapping the primary vegetation structural attributes from which fuel types are derived. Using the BFC fuel typology, we mapped fuels with accuracies of 89% for forest fuel types and 71% for non-forest types. The methods are simple, transparent and flexible; they represent a standardised approach across jurisdictions (and so generate nationally consistent products), and they have the potential to be automated to generate current-condition fuels information.
There are several key areas where these methods can be improved. Introducing annually updated vegetation height data and combining them with the existing sub-seasonal foliage cover data will enable fuel types to be updated annually. This is important in landscapes that experience rapid and significant changes in vegetation structure. Another improvement would be deriving all input variables at a fine resolution (30 m), which would require the development of new, finer-resolution foliage cover and burn history information than are currently available. Expanding understorey mapping into all shrublands and forests would enhance the representation of sub-canopy fuel characteristics across more landscapes. Finally, although we have validated the BFC fuel types at local scales (i.e. grid-cell-specific), we have only done this at a fairly small number of locations. Expanding the validation to cover more regions across the continent would provide a more comprehensive picture of our method’s accuracy.
Data availability
The BFC top-tier and mid-tier fuel type layers are available as geotiffs. These can be accessed via http://hdl.handle.net/102.100.100/701771.
Declaration of funding
This research was conducted as part of CSIRO’s National Bushfire Intelligence Capability project, which is funded by the Australian Climate Service and is supported by Australia’s National Emergency Management Agency.
Acknowledgements
We thank Jen Hollis (Department of Biodiversity, Conservation and Attractions, WA), Neil Burrows, Jane Cawson (University of Melbourne) and James Furlaud (CSIRO) for sharing their forest plot-level data, used in the evaluation of the BFC fuel type geospatial layer. We also thank Kimberley Opie and Chandrama Sarker for their contribution to the evaluation process. The authors acknowledge the valuable comments provided by Tim McVicar (CSIRO), Matt Plucinski (CSIRO) and Meaghan Jenkins (NSW RFS) on an earlier draft of this paper.
References
ABARES (2021) ‘Catchment Scale Land Use of Australia.’ (Australian Bureau of Agricultural and Resource Economics and Sciences) Available at https://www.agriculture.gov.au/abares/aclump [verified 15 October 2024]
ABARES (2022) ‘Australian Bureau of Agricultural and Resource Economics and Sciences, Australia’s Plantations.’ CC BY 4.0. (Australian Bureau of Agricultural and Resource Economics and Sciences: Canberra) Available at https://www.agriculture.gov.au/abares/forestsaustralia/forest-data-maps-and-tools/spatial-data/australias-plantations [verified 15 October 2024]
Abdollahi A, Yebra M (2023) Forest fuel type classification: review of remote sensing techniques, constraints and future trends. Journal of Environmental Management 342, 118315.
| Crossref | Google Scholar | PubMed |
ABS (2023) ‘Australian Bureau of Statistics. Average Floor Area of New Residential Dwellings.’ (ABS: Canberra, ACT) Available at https://www.abs.gov.au/articles/average-floor-area-new-residential-dwellings [verified 15-October-2024]
AHDAP (2022) ‘Australian Housing Data Analytics Platform.’ (A collaborative platform supported by the City Futures Research Centre, UNSW Sydney and the NCRIS-enabled Australian Research Data Commons (ARDC): CC BY 4.0) Available at https://housing-data-exchange.ahdap.org/dataset/building-footprints [verified 15 October 2024]
Alipour M, La Puma I, Picotte J, Shamsaei K, Rowell E, Watts A, Kosovic B, Ebrahimian H, Taciroglu E (2023) A multimodal data fusion and deep learning framework for large-scale wildfire surface fuel mapping. Fire 6(2), 36.
| Crossref | Google Scholar |
Anderson WR, Cruz MG, Fernandes PM, McCaw L, Vega JA, Bradstock RA, Fogarty L, Gould J, McCarthy G, Marsden-Smedley JB, Matthews S, Mattingley G, Pearce HG, van Wilgen BW (2015) A generic, empirical-based model for predicting rate of fire spread in shrublands. International Journal of Wildland Fire 24(4), 443-460.
| Crossref | Google Scholar |
Andrews PL, Bevins CD, Seli RC (2008) BehavePlus fire modeling system, version 4.0: User’s Guide. Report number RMRS-GTR-106WWW. (USDA Forest Service, Rocky Mountain Research Station: Ogden, UT, USA) Available at http://www.fs.fed.us/rm/pubs/rmrs_gtr106.pdf
Aragoneses E, García M, Salis M, Ribeiro LM, Chuvieco E (2023) Classification and mapping of European fuels using a hierarchical, multipurpose fuel classification system. Earth System Science Data 15(3), 1287-1315.
| Crossref | Google Scholar |
Bowman DMJS, Murphy BP, Boer MM, Bradstock RA, Cary GJ, Cochrane MA, Fensham RJ, Krawchuk MA, Price OF, Williams RJ (2013) Forest fire management, climate change, and the risk of catastrophic carbon losses. Frontiers in Ecology and the Environment 11(2), 66-67.
| Crossref | Google Scholar |
Bradstock RA (2010) A biogeographic model of fire regimes in Australia: current and future implications. Global Ecology and Biogeography 19(2), 145-158.
| Crossref | Google Scholar |
Burns P, Hakkenberg CR, Goetz SJ (2024) Multi-resolution gridded maps of vegetation structure from GEDI. Scientific Data 11(1), 881.
| Crossref | Google Scholar | PubMed |
Burrows N, Gill M, Sharples J (2018) Development and validation of a model for predicting fire behaviour in spinifex grasslands of arid Australia. International Journal of Wildland Fire 27(4), 271-279.
| Crossref | Google Scholar |
Burrows N, Wills A, Densmore V (2023) Fuel weight and understorey hazard dynamics in mature karri (Eucalyptus diversicolor) forests in southwest Western Australia. Australian Forestry 86(2), 68-81.
| Crossref | Google Scholar |
Cawson JG, Duff TJ, Swan MH, Penman TD (2018) Wildfire in wet sclerophyll forests: the interplay between disturbances and fuel dynamics. Ecosphere 9(5), e02211.
| Crossref | Google Scholar |
Cheney NP, Gould JS, Catchpole WR (1998) Prediction of fire spread in grasslands. International Journal of Wildland Fire 8(1), 1-13.
| Crossref | Google Scholar |
Cheney NP, Gould JS, McCaw WL, Anderson WR (2012) Predicting fire behaviour in dry eucalypt forest in southern Australia. Forest Ecology and Management 280, 120-131.
| Crossref | Google Scholar |
Chrysafis I, Damianidis C, Giannakopoulos V, Mitsopoulos I, Dokas IM, Mallinis G (2023) Vegetation fuel mapping at regional scale using Sentinel-1, Sentinel-2, and DEM derivatives—the case of the region of East Macedonia and Thrace, Greece. Remote Sensing 15(4), 1015.
| Crossref | Google Scholar |
Chuvieco E, Aguado I, Salas J, García M, Yebra M, Oliva P (2020) Satellite remote sensing contributions to wildland fire science and management. Current Forestry Reports 6(2), 81-96.
| Crossref | Google Scholar |
Clark DB, Mercado LM, Sitch S, Jones CD, Gedney N, Best MJ, Pryor M, Rooney GG, Essery RLH, Blyth E, Boucher O, Harding RJ, Huntingford C, Cox PM (2011) The Joint UK Land Environment Simulator (JULES), model description – Part 2: Carbon fluxes and vegetation dynamics. Geoscientific Model Development 4(3), 701-722.
| Crossref | Google Scholar |
Cruz MG, Alexander ME, Fernandes PAM (2008) Development of a model system to predict wildfire behaviour in pine plantations. Australian Forestry 71(2), 113-121.
| Crossref | Google Scholar |
Cruz MG, McCaw WL, Anderson WR, Gould JS (2013) Fire behaviour modelling in semi-arid mallee-heath shrublands of southern Australia. Environmental Modelling & Software 40, 21-34.
| Crossref | Google Scholar |
Cruz MG, Gould JS, Alexander ME, Sullivan AL, McCaw WL, Matthews S (2015b) Empirical-based models for predicting head-fire rate of spread in Australian fuel types. Australian Forestry 78, 118-158.
| Crossref | Google Scholar |
Cruz M, Gould J, Hollis J, McCaw W (2018) A hierarchical classification of wildland fire fuels for Australian vegetation types. Fire 1(1), 13.
| Crossref | Google Scholar |
Cruz MG, Cheney NP, Gould JS, McCaw WL, Kilinc M, Sullivan AL (2022) An empirical-based model for predicting the forward spread rate of wildfires in eucalypt forests. International Journal of Wildland Fire 31(1), 81-95.
| Crossref | Google Scholar |
Davey SM, Sarre A (2020) Editorial: the 2019/20 Black Summer bushfires. Australian Forestry 83(2), 47-51.
| Crossref | Google Scholar |
DCCEEW (2020) ‘Australia – present major vegetation groups – NVIS version 6.0 (Albers 100 m analysis product).’ (Department of Climate Change, Energy, the Environment and Water) Available at https://www.dcceew.gov.au/environment/land/native-vegetation/national-vegetation-information-system/data-products [verified 15 October 2024]
Dimitrakopoulos AP (2002) Mediterranean fuel models and potential fire behaviour in Greece. International Journal of Wildland Fire 11(2), 127-130.
| Crossref | Google Scholar |
Domingo D, de la Riva J, Lamelas MT, García-Martín A, Ibarra P, Echeverría M, Hoffrén R (2020) Fuel type classification using airborne laser scanning and Sentinel 2 data in Mediterranean forest affected by wildfires. Remote Sensing 12(21), 3660.
| Crossref | Google Scholar |
Donohue R (2021) ‘Testing the accuracy of Gramina, a model for monitoring Australia’s pasture and grassland biomass.’ CSIRO internal report. (CSIRO: Canberra, ACT, Australia) 10.4225/08/5a3953b0a4d5c
Donohue RJ, Renzullo LJ (2025) An assessment of the accuracy of satellite-derived woody and grass foliage cover estimates for Australia. Australian Journal of Botany 73(2), BT24060.
| Crossref | Google Scholar |
Donohue RJ, Mokany K, McVicar TR, O’Grady AP (2022) Identifying management-driven dynamics in vegetation cover: applying the Compere framework to Cooper Creek, Australia. Ecosphere 13(3), e4006.
| Crossref | Google Scholar |
Erdody TL, Moskal LM (2010) Fusion of LiDAR and imagery for estimating forest canopy fuels. Remote Sensing of Environment 114(4), 725-737.
| Crossref | Google Scholar |
Farr TG, Rosen PA, Caro E, Crippen R, Duren R, Hensley S, Kobrick M, Paller M, Rodriguez E, Roth L, Seal D, Shaffer S, Shimada J, Umland J, Werner M, Oskin M, Burbank D, Alsdorf D (2007) The shuttle radar topography mission. Reviews of Geophysics 45(2), RG2004.
| Crossref | Google Scholar |
Finney MA (2006) An overview of FlamMap fire modeling capabilities. In ‘Proceedings of Fuels Management-How to Measure Success: Conference Proceedings’, 28–30 March 2006, Portland, OR. Proceedings RMRS-P-41. Vol. 41. (Comps PL Andrews, BW Butler) pp. 213–220. (US Department of Agriculture, Forest Service, Rocky Mountain Research Station: Fort Collins, CO)
Furlaud JM, Prior LD, Williamson GJ, Bowman DMJS (2021a) Bioclimatic drivers of fire severity across the Australian geographical range of giant Eucalyptus forests. Journal of Ecology 109(6), 2514-2536.
| Crossref | Google Scholar |
Furlaud JM, Prior LD, Williamson GJ, Bowman DMJS (2021b) Fire risk and severity decline with stand development in Tasmanian giant Eucalyptus forest. Forest Ecology and Management 502, 119724.
| Crossref | Google Scholar |
García M, Riaño D, Chuvieco E, Salas J, Danson F M (2011) Multispectral and LiDAR data fusion for fuel type mapping using Support Vector Machine and decision rules. Remote Sensing of Environment 115(6), 1369-1379.
| Crossref | Google Scholar |
Gibson R, Danaher T, Hehir W, Collins L (2020) A remote sensing approach to mapping fire severity in south-eastern Australia using sentinel 2 and random forest. Remote Sensing of Environment 240, 111702.
| Crossref | Google Scholar |
Giglio L, Justice C, Boschetti L, Roy D (2021) ‘MODIS/terra+aqua burned area monthly L3 global 500m SIN grid V061.’ (NASA EOSDIS Land Processes DAAC: Washington, DC, USA). Available at https://lpdaac.usgs.gov/products/mcd64a1v006/
Gill AM, Stephens SL, Cary GJ (2013) The worldwide ‘wildfire’ problem. Ecological Applications 23(2), 438-454.
| Crossref | Google Scholar | PubMed |
Guerschman JP, Hill MJ (2018) Calibration and validation of the Australian fractional cover product for MODIS collection 6. Remote Sensing Letters 9(7), 696-705.
| Crossref | Google Scholar |
Haverd V, Smith B, Nieradzik L, Briggs PR, Woodgate W, Trudinger CM, Canadell JG, Cuntz M (2018) A new version of the CABLE land surface model (subversion revision r4601) incorporating land use and land cover change, woody vegetation demography, and a novel optimisation-based approach to plant coordination of photosynthesis. Geoscientific Model Development 11(7), 2995-3026.
| Crossref | Google Scholar |
Hengl T, Walsh MG, Sanderman J, Wheeler I, Harrison SP, Prentice IC (2018) Global mapping of potential natural vegetation: an assessment of machine learning algorithms for estimating land potential. PeerJ 6, e5457.
| Crossref | Google Scholar | PubMed |
Hollis JJ, Gould JS, Cruz MG, McCaw WL (2015) Framework for an Australian fuel classification to support bushfire management. Australian Forestry 78(1), 1-17.
| Crossref | Google Scholar |
Hollis JJ, Matthews S, Anderson WR, Cruz MG, Fox-Hughes P, Grootemaat S, Kenny BJ, Sauvage S (2024a) A framework for defining fire danger to support fire management operations in Australia. International Journal of Wildland Fire 33(3), WF23141.
| Crossref | Google Scholar |
Hollis JJ, Matthews S, Fox-Hughes P, Grootemaat S, Heemstra S, Kenny BJ, Sauvage S (2024b) Introduction to the Australian Fire Danger Rating System. International Journal of Wildland Fire 33(3), WF23140.
| Crossref | Google Scholar |
Jolly WM, Freeborn PH, Bradshaw LS, Wallace J, Brittain S (2024) Modernizing the US National Fire Danger Rating System (version 4): simplified fuel models and improved live and dead fuel moisture calculations. Environmental Modelling & Software 181, 106181.
| Crossref | Google Scholar |
Jones DA, Wang W, Fawcett R (2009) High-quality spatial climate data-sets for Australia. Australian Meteorological and Oceanographic Journal 58(4), 233-248.
| Crossref | Google Scholar |
Justice CO, Vermote E, Townshend JRG, Defries R, Roy DP, Hall DK, Salomonson VV, Privette JL, Riggs G, Strahler A, Lucht W, Myneni RB, Knyazikhin Y, Running SW, Nemani RR, Zhengming W, Huete AR, Leeuwen Wv, Wolfe RE, Giglio L, Muller J, Lewis P, Barnsley MJ (1998) The Moderate Resolution Imaging Spectroradiometer (MODIS): land remote sensing for global change research. IEEE Transactions on Geoscience and Remote Sensing 36(4), 1228-1249.
| Crossref | Google Scholar |
Keane RE, Burgan R, van Wagtendonk J (2001) Mapping wildland fuels for fire management across multiple scales: integrating remote sensing, GIS, and biophysical modeling. International Journal of Wildland Fire 10(4), 301-319.
| Crossref | Google Scholar |
Keane RE, Herynk JM, Toney C, Urbanski SP, Lutes DC, Ottmar RD (2013) Evaluating the performance and mapping of three fuel classification systems using forest inventory and analysis surface fuel measurements. Forest Ecology and Management 305, 248-263.
| Crossref | Google Scholar |
Kenny BJ, Matthews S, Sauvage S, Grootemaat S, Hollis JJ, Fox-Hughes P (2024) Australian Fire Danger Rating System: implementing fire behaviour calculations to forecast fire danger in a research prototype. International Journal of Wildland Fire 33(4), WF23142.
| Crossref | Google Scholar |
Krasnow K, Schoennagel T, Veblen TT (2009) Forest fuel mapping and evaluation of LANDFIRE fuel maps in Boulder County, Colorado, USA. Forest Ecology and Management 257(7), 1603-1612.
| Crossref | Google Scholar |
Lang N, Jetz W, Schindler K, Wegner JD (2023) A high-resolution canopy height model of the Earth. Nature Ecology & Evolution 7(11), 1778-1789.
| Crossref | Google Scholar | PubMed |
López-De-Castro M, Herráez D, Asensio M, Pagnini G (2022) A high-resolution fuel type mapping procedure based on satellite imagery and neural networks: updating fuel maps for wildfire simulators. Remote Sensing Applications: Society and Environment 27, 100810.
| Crossref | Google Scholar |
Loudermilk EL, O’Brien JJ, Goodrick SL, Linn RR, Skowronski NS, Hiers JK (2022) Vegetation’s influence on fire behavior goes beyond just being fuel. Fire Ecology 18(1), 9.
| Crossref | Google Scholar |
Malone B, Searle R (2022) Soil and Landscape Grid National Soil Attribute Maps - Sand (3″ resolution) - Release 2. 2.0. Terrestrial Ecosystem Research Network [Dataset]. 10.25919/rjmy-pa10.
Marino E, Ranz P, Tomé JL, Noriega MÁ, Esteban J, Madrigal J (2016) Generation of high-resolution fuel model maps from discrete airborne laser scanner and Landsat-8 OLI: a low-cost and highly updated methodology for large areas. Remote Sensing of Environment 187, 267-280.
| Crossref | Google Scholar |
Marsden-Smedley JB, Catchpole WR (1995) Fire modelling in Tasmanian buttongrass moorlands I. Fuel characteristics. International Journal of Wildland Fire 5(4), 203-214.
| Crossref | Google Scholar |
Marselis SM, Yebra M, Jovanovic T, van Dijk AIJM (2016) Deriving comprehensive forest structure information from mobile laser scanning observations using automated point cloud classification. Environmental Modelling & Software 82, 142-151.
| Crossref | Google Scholar |
McCaw WL (2011) Characteristics of jarrah (Eucalyptus marginata) forest at FORESTCHECK monitoring sites in south-west Western Australia: stand structure, litter, woody debris, soil and foliar nutrients. Australian Forestry 74(4), 254-265.
| Crossref | Google Scholar |
Mihajlovski B, Fernandes PM, Pereira JMC, Guerra-Hernández J (2023) Comparing forest understory fuel classification in Portugal using discrete airborne laser scanning data and satellite multi-source remote sensing data. Fire 6(9), 327.
| Crossref | Google Scholar |
Monedero S, Ramirez J, Cardil A (2019) Predicting fire spread and behaviour on the fireline. Wildfire analyst pocket: a mobile app for wildland fire prediction. Ecological Modelling 392, 103-107.
| Crossref | Google Scholar |
Moritz MA, Batllori E, Bradstock RA, Gill AM, Handmer J, Hessburg PF, Leonard J, McCaffrey S, Odion DC, Schoennagel T, Syphard AD (2014) Learning to coexist with wildfire. Nature 515(7525), 58-66.
| Crossref | Google Scholar | PubMed |
Nikparvar B, Thill J-C (2021) Machine learning of spatial data. ISPRS International Journal of Geo-Information 10(9), 600.
| Crossref | Google Scholar |
Phelps N, Beverly JL (2022) Classification of forest fuels in selected fire-prone ecosystems of Alberta, Canada—implications for crown fire behaviour prediction and fuel management. Annals of Forest Science 79(1), 40.
| Crossref | Google Scholar |
Plucinski MP, Sullivan AL, Rucinski CJ, Prakash M (2017) Improving the reliability and utility of operational bushfire behaviour predictions in Australian vegetation. Environmental Modelling & Software 91, 1-12.
| Crossref | Google Scholar |
Potapov P, Li X, Hernandez-Serna A, Tyukavina A, Hansen MC, Kommareddy A, Pickens A, Turubanova S, Tang H, Silva CE, Armston J, Dubayah R, Blair JB, Hofton M (2021) Mapping global forest canopy height through integration of GEDI and Landsat data. Remote Sensing of Environment 253, 112165.
| Crossref | Google Scholar |
Raymond OL, Retter AJ (2014) Surface Geology of Australia 1:1,000,000 Scale, 2010 Edition. (Atlas of Living Australia) Available at https://spatial.ala.org.au/layers, https://researchdata.edu.au/surface-geology-australia-2010-edition [verified 22 October 2024]
Rollins MG (2009) LANDFIRE: a nationally consistent vegetation, wildland fire, and fuel assessment. International Journal of Wildland Fire 18(3), 235-249.
| Crossref | Google Scholar |
Rollins MG, Keane RE, Parsons RA (2004) Mapping fuels and fire regimes using remote sensing, ecosystem simulation, and gradient modeling. Ecological Applications 14(1), 75-95.
| Crossref | Google Scholar |
Scarth P, Armston J, Lucas R, Bunting P (2019) A structural classification of Australian vegetation using ICESat/GLAS, ALOS PALSAR, and Landsat sensor data. Remote Sensing 11(2), 147.
| Crossref | Google Scholar |
Schlund M, Wenzel A, Camarretta N, Stiegler C, Erasmi S (2023) Vegetation canopy height estimation in dynamic tropical landscapes with TanDEM-X supported by GEDI data. Methods in Ecology and Evolution 14(7), 1639-1656.
| Crossref | Google Scholar |
Scott JH, Burgan RE (2005) Standard fire behavior fuel models: A comprehensive set for use with Rothermel’s surface fire spread model. Report number RMRS-GTR-153 (USDA Forest Service, Rocky Mountain Forest and Range Experiment Station: Fort Collins, CO, USA) Available at https://www.fs.fed.us/rm/pubs/rmrs_gtr153.pdf
Sharples JJ, Cary GJ, Fox-Hughes P, Mooney S, Evans JP, Fletcher M-S, Fromm M, Grierson PF, McRae R, Baker P (2016) Natural hazards in Australia: extreme bushfire. Climatic Change 139, 85-99.
| Crossref | Google Scholar |
Sismanis M, Stefanidou A, Stavrakoudis D, Gitas IZ (2023) Wildland fuel type mapping in Attica using Sentinel-2 time-series. In ‘Proceedings of 2023 8th International Conference on Smart and Sustainable Technologies (SpliTech)’, 20–23 June 2023. pp. 1–5. (IEEE) 10.23919/SpliTech58164.2023.10193645
Slijepcevic A, Anderson WR, Matthews S, Anderson DH (2018) An analysis of the effect of aspect and vegetation type on fine fuel moisture content in eucalypt forest. International Journal of Wildland Fire 27(3), 190-202.
| Crossref | Google Scholar |
Sparrow B, Tokmakoff A, Leitch E, Guerin G, O’Neill S, Macdonald C, Lowe A, Flitton R, Saleeba T, Coish C (2021) ‘TERN Ecosystem Surveillance Monitoring Program: Photopoint Panoramic Images’, Version 1.0 edn. (Terrestrial Ecosystem Research Network) Available at https://portal.tern.org.au/metadata/TERN/fe390ffb-8f30-44e2-872d-93fa96ec8f79
Specht R (1972) Water use by perennial evergreen plant communities in Australia and Papua New Guinea. Australian Journal of Botany 20(3), 273-299.
| Crossref | Google Scholar |
Stefanidou A, Gitas IZ, Katagis T (2022) A national fuel type mapping method improvement using sentinel-2 satellite data. Geocarto International 37(4), 1022-1042.
| Crossref | Google Scholar |
Sullivan A, McCaw L, Cruz M, Matthews S, Ellis PF (2012) Fuel, fire weather and fire behaviour in Australian Ecosystems. In ‘Flammable Australia: Fire Regimes, Biodiversity and Ecosystems in a Changing World’. (Eds RA Bradstock, AM Gill, RJ Williams) pp. 51–78. (CSIRO Publishing: Melbourne, Vic, Australia)
Syphard AD, Keeley JE, Massada AB, Brennan TJ, Radeloff VC (2012) Housing arrangement and location determine the likelihood of housing loss due to wildfire. PLoS One 7(3), e33954.
| Crossref | Google Scholar | PubMed |
Ticehurst C, Newnham G (2024) Producing annual Australia-wide vegetation height images from GEDI and Landsat data. International Journal of Remote Sensing 45(18), 6445-6469.
| Crossref | Google Scholar |
Tolhurst K (2005) Conversion of ecological vegetation classes (EVCs) to fuel types and calculation of equivalent fine fuel loads with time since fire. Victoria 1-7.
| Google Scholar |
Tolhurst K, Duff T, Chong D (2013) From ‘Wildland–Urban Interface’ to ‘Wildfire Interface Zone’ using dynamic fire modelling. In ‘Proceedings of MODSIM2013, 20th International Congress on Modelling and Simulation'. (Eds J Piantadosi, RS Anderssen, J Boland) pp. 1–6. (Modelling and Simulation Society of Australia and New Zealand: Adelaide, SA)
Wang Z, Cai H, Yang X (2024) A new method for mapping vegetation structure parameters in forested areas using GEDI data. Ecological Indicators 164, 112157.
| Crossref | Google Scholar |
Wilson BA, Charlotte FYO, Heathcott M, Milne D, McCaffrey TM, Ghitter G, Franklin SE (1994) Landsat MSS classification of fire fuel types in Wood Buffalo National Park, Northern Canada. Global Ecology and Biogeography Letters 4(2), 33-39.
| Crossref | Google Scholar |
Appendix 1.Mapping Australian hummock grasslands
Mapping of the BFC fuel types required knowledge of the distribution of hummock (spinifex) grasslands. These grasslands are an important fuel type across much of inland Australia but are poorly mapped at the national scale (Allan and Southgate 2002). A new map (Fig. A1) was developed for this analysis that was based on a priori knowledge of hummock grass characteristics and growing conditions. Here, hummock grasslands areas can include scattered trees and shrubs and can occur as the understorey in woodland and open shrubland vegetation types. Here, we describe how the Hummock grasslands map was generated.
Description of inputs
The inputs used to generate the Hummock grassland layer are listed in Table A1. Any variables not already described in the main text are described below.
Variable | Notation | Units | Spatial resolution (m) | Data currency | Reference | |
---|---|---|---|---|---|---|
Brown cover | C n | % | 500 | 2001–2022 | Guerschman and Hill (2018) | |
Total foliage cover | C t | % | 250 | 2001–2022 | Donohue and Renzullo (2025) | |
Sand content | S sand | % | 90 | – | Malone and Searle (2022) | |
Limestone | G lim | Binary (1 = limestone) | – | – | Raymond and Retter (2014) | |
Water cover frequency | Z | % (of year) | 250 | 2001–2022 | Donohue et al. (2022) | |
C4 proportion | P C4 | Fraction | 250 | 2001–2022 | Donohue (2021) | |
Precipitation | R | mm/year | 5000 | 1991–2022 | Jones et al. (2009) |
Brown cover (Cn) is the fraction of ground covered by non-photosynthetically active vegetation, and includes standing and fallen branches and twigs, and dead leaves. Brown cover was generated using the method of Guerschman and Hill (2018), which is based on the 500 m MODIS NDVI product (MCD43A4 Collection 6.0). We derived a long-term (2001–2020) annual average brown cover fraction.
Sand content (Ssand and Sclay) is the percentage of soil volume composed of sand particles. This represents the average of the top 30 cm of soil volume.
Ruleset for identifying Hummock grasslands
Hummock grassland distribution was estimated using the ruleset in Table A2.
Appendix 2.Mapping the wildland–urban interface and built-up fuel types from building footprint data
Wildland–urban interface and Built-up fuel types were mapped as a function of building density (Tolhurst et al. 2013). Building density was generated from the building footprint polygon layer from National Housing Data Exchange Australia (AHDAP 2022). This layer comprises polygons of building outlines from which the area (ha) of building can be derived. We used this building area information to calculate building area density (ha/ha, i.e. built area per area of ground) and then derived the Wildland–urban interface (WUI 1, 2 and 3 as per Cruz et al. 2018a) and Built-up fuel types (Table A3). Considering an average size of an Australian house of 250 m2 (ABS 2023), WUI1, WUI2 and WUI3 correspond to the ‘Rural’, ‘Rural residential’ and ‘Urban’ areas of Tolhurst et al. (2013), respectively. Built-up as a fuel type was not defined in Cruz et al. (2018a). Built-up areas have a building density above 0.125 ha/ha, i.e. typically more than 5 houses per ha. Built-up areas are not supposed to sustain active fire propagation.
Fuel type | Ruleset | |
---|---|---|
WUI 1 | 0 < D ≤ 0.0025 | |
WUI 2 | 0.0025 < D ≤ 0.025 | |
WUI 3 | 0.025 < D ≤ 0.125 | |
Built-up | D > 0.125 |
The spatial calculation of built area follows:
Convert building polygons to a 90 m raster describing the total building area present within each grid cell.
Using a moving window of 7 × 7 pixels (630 × 630 m), estimate the total building area within the window.
Calculate the building density (D, ha/ha) within the window area by dividing the total building area with window area and allocate that density value to the central pixel.
Classify pixels into WUI or Built-up fuel types based on building density (Table A3).
An example of the WUI and Built-up classes is given for the Australia Capital Territory and surrounding areas in Fig. A2. Here Built-up areas are shown in black while WUIs are shown in red, purple and orange for WUI 1–WUI 3 respectively.
Appendix 3.Linking BFC fuel types with Australian fire spread models
Fuel type classes aggregate fuel complexes that share similar structural attributes and hence share potential fire behavioural characteristics. One purpose of classifying fuel complexes into fuel types and generating fuel type geospatial data is so that the most appropriate fire spread model (which each assumes a particular set of fuel characteristics) can be appropriately employed at any given location. In the Australian fire behaviour modelling paradigm, each fuel type has an associated fire spread model, although the diversity of fuel types and the limitations of fire spread models (e.g. bounds of original data used in model development) make this association unclear in a number of situations. Table A4 presents the rulesets that associate the main fire spread models currently used in Australia (Cruz et al. 2015b; Plucinski et al. 2017; Kenny et al. 2024) to the BFC top (Table 3) and mid-tier (Table 4) fuel type classes developed in the current study. These association rules should not be considered universal, as there will be situations where the specifics of a local fuel complex may not fit the fuel type–fire spread model association. Fig. A3 provides a visualisation of the spatial distribution of recommended fire spread models across Australia.
Fire spread model | Ruleset | |
---|---|---|
Forest | “Tall closed forest” OR | |
Cheney et al. (2012), Cruz et al. (2022) | “Closed forest” OR | |
“Tall open forest” OR | ||
“Broadleaf plantation” OR | ||
“Other plantation” OR | ||
“Conifer plantation” (of non-Pinus genus) OR | ||
(Ta ≤ 27°C AND (“Open forest” OR “Low open forest”)) | ||
Pine | “Conifer plantation” (of Pinus genus) | |
Cruz et al. (2008) | ||
Northern grassland | “Tall woodland with grassy understorey” OR | |
Cheney and Sullivan (2008) | “Woodland with grassy understorey” OR | |
“Low woodland with grassy understorey OR | ||
(Ta > 27°C AND (“Open forest” OR “Low open forest”)) | ||
Mallee heath | R ≤ 550 mm AND | |
Cruz et al. (2013) | (“Low woodland with shrubby understorey” OR “Tall shrubland” OR “Shrubland”) | |
Shrubland | “Woodland with shrubby understorey” OR | |
Anderson et al. (2015) | (R > 550 mm AND (“Low woodland with shrubby understorey” OR “Tall shrubland” OR “Shrubland”)) | |
Spinifex | “Woodland with spinifex understorey” OR | |
Burrows et al. (2018) | “Low woodland with spinifex understorey” OR | |
“Open shrubland with spinifex understorey” OR | ||
“Hummock grassland” | ||
Grassland | “Sparse grassland” OR “Open grassland” OR | |
Cheney et al. (1998) | “Grassland” OR “Cropland” OR | |
“Woodland with sparse understorey” OR | ||
“Low woodland with sparse understorey” OR | ||
“Open shrubland with grassy understorey” OR | ||
“Open shrubland with sparse understorey” OR | ||
“Horticulture” | ||
Buttongrass | “Sedgeland” | |
Marsden-Smedley and Catchpole (1995) | ||
Non-combustible | “Wetlands” OR | |
“Water” OR | ||
“Wildland–urban interface” OR | ||
“Built-up” OR | ||
“Bare ground” |
The rules are applied sequentially in the table, from top to bottom, with any given rule not being applied to previously classified areas. Quoted descriptions in the rulesets are the BFC fuel type classes.
Spatial distribution of Australian fire spread models as generated from ruleset applied to BFC fuel types (Table A4).
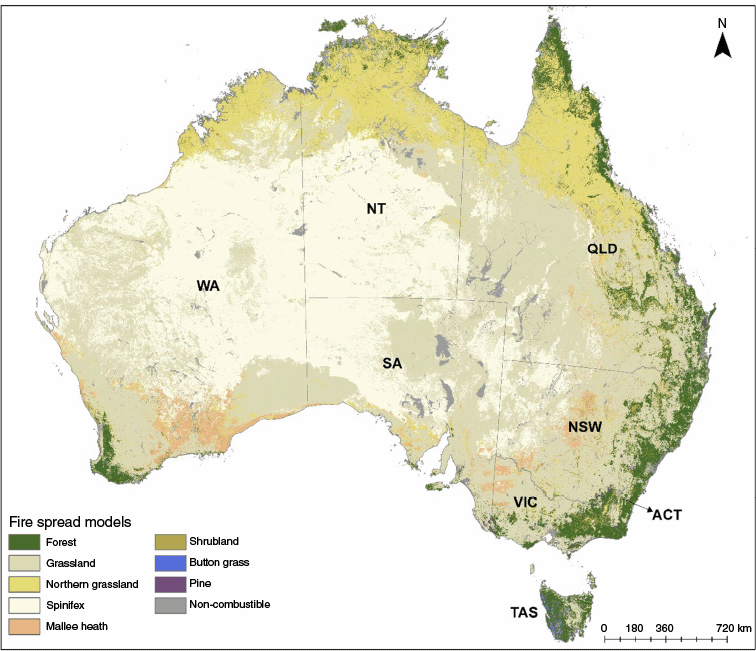