Can predators influence small rodent foraging activity rates immediately after wildfires?
Roger Puig-Gironès
A
B
International Journal of Wildland Fire 32(10) 1391-1403 https://doi.org/10.1071/WF23023
Submitted: 11 April 2022 Accepted: 24 August 2023 Published: 20 September 2023
Abstract
Habitat complexity, predation risk, and intraspecific competition shape rodent communities and impact foraging. Wildfires remove vegetation shelter, which increases the predation risk perception and leads to changes in trophic resources availability. Consequently, prey adjust their foraging activity levels to minimise the likelihood of encounters with predators. Rodents select safe habitats and can detect predators’ scents, which allows them to reduce the predation risk when foraging.
To evaluate the effects of carnivore occurrence and habitat structure on rodent foraging activity rates immediately after fires using mixed models and structural equation modelling.
This study used 900-m linear transects to analyse environmental variables, acorn removal by rodents, and carnivore activity in three recently burnt areas.
In areas with higher stone marten abundances, rodents removed more acorns. However, acorn removal was also higher in structurally complex habitats with greater rodent abundance.
Rodents’ foraging activity is driven by increased interspecific competition and the predation risk perception due to the simplicity of the burnt habitat. Additionally, stone martens and rodents share the same preferences for habitat complexity after fires.
Habitat complexity increases seed holding by rodents, which positively contributes to fire recovery and attracts predators, thereby increasing species diversity.
Keywords: carnivores, foraging activity, habitat structure, Mediterranean ecosystems, predator–prey interaction, rodents, seed dispersal, shelter, wildfires.
Introduction
Foraging activity is crucial to consumer fitness. Prey species adjust their foraging activity levels as a response to their predation risk, which represents the potential danger of being captured by a predator (Lima and Dill 1990; Houston et al. 1993). The availability of shelter and camouflage is a key determinant in prey foraging activity (Embar et al. 2011) because predator–prey encounters are relatively rare. The structure of the habitat, therefore, appears to be the main driver of the predation risk (Verdolin 2006). Small rodents are common prey items for a wide range of predators. Food availability determines the time required to obtain sufficient food, which in turn affects the risk of predator encounters because the cost of manipulating and carrying seeds can be high (Berger-Tal et al. 2009). For instance, the wood mouse (Apodemus sylvaticus L.), the most common small rodent in the Mediterranean Basin, selects safe habitats and microhabitats when foraging (Kotler et al. 2002; Díaz et al. 2005; Perea et al. 2011a). Shrubs typically provide shelter from predators and a place where rodents can forage and feed safely (Manson and Stiles 1998; Muñoz et al. 2009). Wood mice can also detect and respond to scents of predators such as the common genet (Genetta genetta L.), which further helps reduce the predation risk while foraging (Sunyer et al. 2013; Feldman et al. 2019). However, high levels of predation pressure at high-risk sites that lack shelter may lead to an increase in rodents’ foraging efficiency (Chaby et al. 2015). The predation risk may also affect the choices made by wood mice: in more open high-risk conditions – as opposed to sites where there is good cover – mice preferred to remove larger acorns (Leaver and Daly 2003; Pérez‐Ramos et al. 2008; Perea et al. 2011a). Intraspecific competitors, referring to individuals of the same species, have a significant influence on the foraging activity. In places where there is greater conspecific competition, it is expected that there will be an increase in foraging activity. This is because competition for resources intensifies individual foraging efforts to secure an adequate supply of food to maintain their fitness (Murray et al. 2006; Lichti et al. 2017).
Wildfires have an impact on faunal responses due to changes in habitat structure, direct mortality and greater predation risk (Morris et al. 2011; He et al. 2019). Fires modify predator–prey interactions by altering food availability and habitat structure, and thus influence predation rates (Persson and Eklov 1995; Janssen et al. 2007) and foraging activity (Vander Wall 2010; Conner et al. 2011; Perea et al. 2011b; Leahy et al. 2016; Morán-López et al. 2016). Burnt areas lead to changes in prey abundance, distribution, and behaviour, and affect predators’ diets (Doherty et al. 2022). Predators with flexible diets can exploit changes in prey availability by targeting more profitable species in terms of availability and ease of capture (Perea et al. 2011a). After fire in south-eastern Australia, the red foxes (Vulpes vulpes L.) exhibited a two-fold increase in consuming medium-sized native mammals, and their preference for long-nosed bandicoots (Perameles nasuta Geoffroy) and short-beaked echidnas (Tachyglossus aculeatus Shaw) also increased (Hradsky et al. 2017a). Predators can affect prey directly if they are attracted to burnt areas, or indirectly through changes in habitat structure (McGregor et al. 2016; Puig-Gironès and Pons 2020; Doherty et al. 2022). Certain prey species may benefit from habitat openness, which will enable them to detect and avoid predators more easily (Cherry et al. 2018), whereas others require denser cover to reduce the predation risk (Perea et al. 2011a; Doherty et al. 2015). Numbers of certain prey species (such as wood mice) increase in burnt areas following plant recovery (Monimeau et al. 2002; Torre and Díaz 2004) because they are able to find more resources and competition is less severe compared with unburnt areas (Puig-Gironès and Pons 2023). However, prey species may use sites with structurally complex habitats or make convoluted movements when foraging as a result of heightened predation risk (Fordyce et al. 2016; Lees et al. 2022). Habitats that provide cover, such as piles of wood debris, can create shelter for prey species (Puig-Gironès et al. 2020). On the other hand, animals under predation risk may be more selective and drop low-profit items from their diet, or be less selective because selectivity demands more extensive foraging activity and greater exposure to risk (Leaver and Daly 2003; Chaby et al. 2015).
The ‘oak-wood mouse–common genet’ model has been well studied because the genet is the most specialised predator of mice in Mediterranean forests (Virgós et al. 1999; Díaz et al. 2005; Rosalino et al. 2011; Sunyer et al. 2013; Feldman et al. 2019). However, the common genet appears to be rare in Mediterranean burnt areas (Birtsas et al. 2012; Puig-Gironès and Pons 2020). Nevertheless, stone martens (Martes foina (Erxleben, 1777)) and red foxes may take advantage of the higher abundance of mice and lower availability of shelter in such areas. The former is a territorial species that prefers areas with well-developed tree cover (Vergara et al. 2016). However, it is positively attracted to recently burnt areas, especially when the habitat structure is complex (Puig-Gironès and Pons 2020), which matches the habitat preferences of rodents. The stone marten has a wide-ranging diet that is well adapted to the availability of local resources. Its main food items consist of small mammals and, particularly, fleshy fruits (Baghli et al. 2002; Rosalino and Santos‐Reis 2009). Red foxes may also predate on small rodents. However, despite their preference for open habitats, fox abundances could affect the foraging activity of rodents that choose preferentially denser cover (Manson and Stiles 1998; Muñoz et al. 2009; Puig-Gironès et al. 2018; Puig-Gironès and Pons 2020).
The aim of this study was to describe the possible effects of predator occurrence (stone martens and red foxes) on small rodent foraging activity rates (hereafter ‘SRFAR’) in three recently burnt forests in Catalonia (W Mediterranean Basin). It was predicted that the presence of predators would increase rodents’ predation risk directly, due to their physical presence, and indirectly via cues such as scents and faeces (Herman and Valone 2000). Accordingly, the following hypotheses and predictions were generated: (1) stone marten occurrence will increase rodents’ predation risk and modify their foraging activity rates; (2) red fox occurrence will not significantly affect rodent foraging rates in burnt areas because their utilisation differs between the two species (Birtsas et al. 2012; Puig-Gironès and Pons 2020); (3) rodent foraging rates will be higher in areas with greater shrub cover, and their foraging activity will increase in concert with time since fire due to greater habitat complexity (Muñoz et al. 2009; Perea et al. 2011b; Puig-Gironès et al. 2018); and (4) foraging activity rates will be reduced with increasing distance from shelter, and resources further from shelter will be utilised less frequently due to higher predation risk. Therefore, shelter such as shrubs or piles of woody debris will provide a release from predation pressure and promote increased in foraging activity in recently burnt habitats (Puig-Gironès et al. 2020).
Material and methods
Study area
Three recent (less than 6 months beforehand) severely burnt areas (over 200 ha) in Catalonia (NE Iberian Peninsula; Fig. 1) were studied. Two of the study areas (La Jonquera and Rasquera) were located in lowlands with Mediterranean climate and one (Ger) in the Pyrenees with a humid Mediterranean climate. All sites were studied for 30 months after fires. Their main characteristics are given in Table 1, and more information on the study sites can be found in Puig-Gironès et al. (2018).
Location and schematic representation of the sampling methods. Location of the three burnt study areas (La Jonquera, Ger and Rasquera) in Catalonia (NE Iberian Peninsula), using the La Jonquera burnt area as an example in black, and schematic representation of linear transects, perpendicular to the burnt area perimeter with sampling stations (grey dots) at increasing distances from the burnt area perimeter. In each sampling station of 5 m diameter, I measured acorn removed by rodents (with an acorn device), the relative abundance of rodents (with a Sherman live trap), and habitat structure (plant cover and height, herbaceous cover and height (as opposed to woody)).
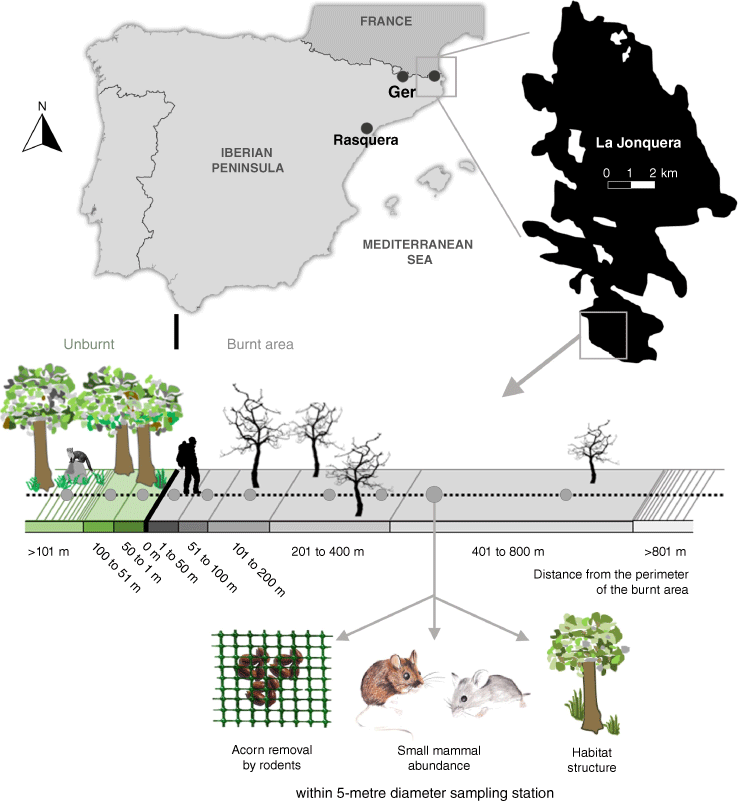
Wildfire | La Jonquera | Rasquera | Ger | ||
---|---|---|---|---|---|
Region | Mediterranean | Mediterranean | Pyrenees | ||
Bioclimate | Subhumid Mediterranean | Dry continental Mediterranean | Humid Mediterranean mountain | ||
Altitude min/average/max (m) | 90/263/574 | 317/348/409 | 1461/1672/1893 | ||
Burnt area (ha) | 13088 | 3082 | 250 | ||
Date of burnt | August 2012 | May 2012 | August 2012 | ||
Time after fire in first sampling (weeks) | 2 | 18 | 1 | ||
Last sampling week | 117 | 138 | 118 | ||
Pre-fire habitat | Oak, pine and shrubland | Pine and shrubland | Pine and shrubland | ||
Number of transects | 9 | 5 | 11 | ||
Sampling occasions | 15 | 7 | 16 | ||
Number of stations | Unburnt | 27 | 15 | 33 | |
Burnt (including perimeter) | 63 | 35 | 77 |
Description of the four burnt areas sampled for 2.5 years. The number of burnt stations includes the station located on the burnt area perimeter (for more information see Puig-Gironès et al. 2018).
Sampling design and field methods
To collect data on SRFAR and carnivore activity (hereafter ‘signs’), linear transects (approximately 900 m long and 10 m wide) were established in each study area. Transects were placed perpendicular to the linear edge of the burnt area (Table 1, Fig. 1) to avoid any influence of border geometry on edge effects (Fernández et al. 2002). Both burnt and unburnt areas were included in the transects. Nearby transects were located, on average, 2048 m ± 540 s.e. from each other, with a minimum distance of 250 m at the closest point. In all, 61% of nearby transects were separated by at least 500 m, a distance that ensured that there was reasonable spatial independence to avoid double counts.
To survey carnivore activity, the observer slowly walked each transect and, within a 3-m-wide band on each side of transect, looked for signs (faeces) (Fig. 1). For each sign found, the species was determined, UTM coordinates were noted, and the faeces were removed from transect. Field signs did not allow us to estimate carnivore abundance due to differences in species detectability caused by their particular ways of marking the territory. Nevertheless, given that red foxes and stone martens deposit their faeces in clearly visible places, field signs could be used as a proxy of carnivore activity at species level (see Ruiz-Capillas et al. (2013)).
Predators have great territorial mobility but low-density populations, so each transect was divided into two categories in terms of the distance from the burnt area perimeter and eight further categories in terms of the time since fire to maximise the information derived from detected stone marten signs. Negative distance from the burnt area perimeter values were assigned to points within the unburnt area (−1 = 1–50 m; −2 = 51–100 m; and −3 = over 100 m from the burnt area perimeter), whereas positive values indicated distances within the burnt area (0 = 0 m; 1 = 1–50 m; 2 = 51–100 m; 3 = 101–200 m; 4 = 201–400 m; 5 = 401–800 m; and 6 = over 800 m from the perimeter). The time since fire values were grouped into monthly categories (1 = 1–2 months after fire; 2 = 3–4; 3 = 5–6; 4 = 7–10; 5 = 11–15; 6 = 16–20; 7 = 21–25; and 8 = 26 months from the end of the sampling period).
Each transect was visited a minimum of seven and maximum of 16 times. At La Jonquera and Ger, the sampling frequency decreased with time after the fire, being monthly in the first 6 months, bimonthly at 7–24 months, and every 3 months thereafter until 30 months had elapsed since the fire. This variation in the sampling frequency during the study ensured that all of the most relevant information regarding the recolonisation process was gathered. Indeed, small rodents are known to be early colonisers (Sainz-Elipe et al. 2012; Puig-Gironès et al. 2018), and it was therefore important to sample more intensely during the first months after fire. Rasquera was, however, sampled constantly every 3 months starting 18 and 23 weeks after fire, respectively (Table 1).
Along each transect 10 5-m-diameter rodent–vegetation sampling stations were established, three in the unburnt area (at approximately 25, 50, and 100 m from the perimeter of the burnt area), one on the perimeter of the burnt area, and six inside the burnt area (at 25, 50, 100, 200, 400, and 800 m from the perimeter) (Fig. 2). At each sampling station, the SRFAR, rodent relative abundance, and other environmental variables (Fig. 1) were measured. The acorns removed by rodents were taken to be a surrogate of SRFAR because acorns are easy to collect and naturally available in and/or near the study sites, and are positively selected by small rodents (Pons and Pausas 2007). The devices offering acorns to rodents (henceforth, ‘acorn devices’) were placed in the centre of each sampling station and consisted of a 50 × 50-cm meshed cage (1.27 cm2 mesh size) containing 20 cork oak (Quercus suber Linnaeus, 1753) acorns. The acorn devices were always installed in the same places, generally below shrubs or, if not possible, very close (less than 20 cm) to shrubs, herbs, or a stone wall to minimise the microhabitat effect on removal. Each device had four small entrances (5 × 5 cm) that prevented jays (Garrulus glandarius (Linnaeus, 1758)) and other birds from entering. The cork oak acorns were all of similar sizes (2.5 ± 0.2 cm in length; mean ± s.e.) to avoid any effect of acorn size on the removal probability. All acorns, collected from cork oak forests near the study area at La Jonquera, were externally analysed to rule out arthropod perforations and then conserved in a dry freezer.
Most Parsimonious Structural Equation Models (SEM). (a) All paths in the initial models were treated as optional and were thus able to be removed during model simplification (from 31 to 14 parameters) using maximum likelihood estimation. (b and c) As a final model, I selected the model with the lowest AICc score. One-way arrows represent paths between variables. Values adjacent to paths indicate standardised direct coefficients, with significance indicated by *, P < 0.05; **, P < 0.01; ***, P < 0.001.
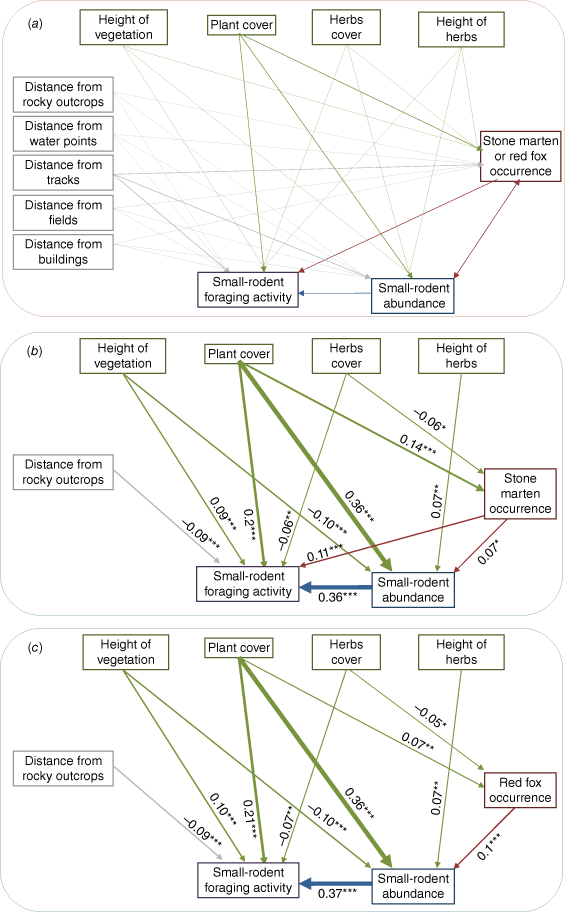
A Sherman live trap for small mammals (5.1 × 6.4 × 22.3 cm, Sherman, Tallahassee, Florida, USA), placed in the centre of each sampling station, was baited with a mixture of tuna, flour, oil, and a piece of apple (Torre and Díaz 2004) and thermally protected. Sherman traps were placed following the criteria used for the acorn devices. Captured small mammals were identified to species level, sexed, and marked with ear tags (National Band Co., USA). All sampling procedures met ASM Care & Use guidelines (Sikes et al. 2011). Three rodent species were live-trapped: wood mouse; Algerian mouse (Mus spretus Lataste, 1883); and common vole (Microtus arvalis (Pallas, 1778)). In subsequent analyses, rodent abundance was used as a proxy for interspecific competition among rodents. Acorn devices and Sherman traps were installed alternatively at sampling stations to avoid interference between devices. They were checked on the first day after installation, and the Sherman traps were used to capture rodents on three consecutive mornings after installation. In this way, each sampling station was active for a minimum of 4 days during each sampling event.
The habitat structure and the dominant vegetation type (herbaceous or woody/shrub) were sampled on each sampling occasion and at each 5-m sampling station concurrently with the sampling of the animal population. Habitat structure was characterised at each sampling station using the following measurements: foliage cover (%), an indicator of vegetation recovery, was estimated using a reference chart for six virtual vegetation height layers: 0–0.25 (C0); 0.25–0.5 (C25); 0.50–1 (C50); 1–2 (C100); 2–4 (C200); and >4 m (C400) (Prodon and Lebreton 1981). A principal component analysis (PCA) was then used to summarise the information obtained from the six variables after arcsine-transforming cover values. The first component (henceforth plant cover; 42.6% of explained variance) corresponded to the extent of plant cover, and the second (henceforth height of vegetation; 27.9% of explained variance) ranked stations in terms of the height of the dominant vegetation layers (Supplementary File S1a). Also, the cover percentage of woody species was estimated at three height layers, 0–0.25, 0.25–0.5, and 0.50–1 m, which was the inverse of the proportion of the herb or non-woody species foliage cover. A principal component analysis (PCA) was used to summarise the information in which the first component (henceforth herb cover; 54% of explained variance) corresponded to the extent of the herb species foliage cover, and the second (PC2, henceforth height of herbs; 28% of explained variance) ranked stations in terms of the maximum height of the herb cover (Supplementary File S1b). These four components of the habitat structure and plant dominance (plant cover, height of vegetation, herb cover, and height of herbs) were used as independent variables in the analyses.
The possible role of other potential shelters for fauna was characterised by measuring their distance from the centre of the sampling stations in the burnt area. Rocky outcrops or piles of stones (distance from rocky outcrops), water sources (ponds, streams, etc.; distance from water), rural constructions (dry-stone walls, farmhouses, barns, etc.; distance from buildings), tracks and paths (distance from tracks), and cropland (both active and abandoned; distance from fields) were also regarded as potential shelters.
Finally, 25 sampling stations along seven transects were selected in a burnt area where a post-fire treatment involving the creation of piles of woody debris had been performed. The sampling stations were randomly selected, with preference given to those with well-built piles. At each sampling station, an acorn device and a Sherman trap were set up in three places: (1) underneath the pile; (2) 5 m from the pile; and (3) 10 m from the pile (see Puig-Gironès et al. (2020) for more details about logging process). The sampling of these piles was conducted at the Ger study area simultaneously with the sampling of the unlogged transects (15 occasions, at 5–118 days after fire). For each sampling station (pile-centred), the exact distance was measured from the burnt area perimeter, as was the foliage cover (%) of the two virtual vegetation height layers (0–0.25 (C0) and 0.25–0.5 (C25)) and the ]rock cover. The woody debris piles were considered as potential shelter in areas with little cover in order to test the effect of shelter availability after fire on foraging rates under varying predation risk.
Statistical analyses
I used the proportion of the maximum number of acorns that could potentially be removed (limited to 20 acorns per sampling event) on the first night after the installation as a surrogate for the acorns removed by rodents, i.e. the foraging activity. A single night of acorn removal was used to avoid the possible throw-off foraging rate produced by discovering a source of abundant food on the first night. Therefore, SRFAR refers to the proportion of acorns removed by rodents. Signs (faeces) were used as occurrence at each transect and visit (0–1) for both red foxes and stone martens, given the heterogeneity of their detectability due to their habit of marking their territories and the transect habitat structure. Because a maximum of three rodents could be trapped per sampling event (three consecutive nights), the proportion of this number caught was used as a surrogate of rodent abundance, so ‘relative abundance’ refers to the proportion of traps occupied by rodents (0, 0.33, 0.66 or 1).
Mixed models were used to assess the importance of predator presence, habitat, and shelter on SRFAR. To evaluate the predation risk on rodents, a general linear mixed model (GLMM) was used to analyse the effects of red fox occurrence and stone marten occurrence on acorns removed by rodents. In addition, for each analysis, variables derived from habitat structure (plant cover, height of vegetation, herb cover, and height of herbs), variables related to fire (the categories of distance from the burnt area perimeter and time–distance interaction), variables describing shelter (distance from rocks, water, buildings, tracks, and fields), and variables describing interspecific competition (rodent abundance) were incorporated as fixed factors. The particular Transect was included as random factor to control possible site-based differences and temporal pseudo replication. Rodent abundance can be a confounding variable for carnivores, habitat structure, and rodent foraging rates, so rodent abundance was added as a random variable (random slope) to avoid any possible masking of the real relationship between the other variables. Random assignment minimises the effects of confounding variables by distributing them equally among all the experimental groups. Finally, the GLMM was used to test the effects of the microhabitat (shelter) on (1) one night, (2) three nights after the acorn device installation, and (3) rodent abundance. Here, the distance to shelter, distance from the burnt area perimeter, time since fire, the foliage cover (%) of 0–0.25 (C0) and 0.25–0.5 (C25), and the cover of rocks were used as fixed factors.
A multi‐model inference approach was used to select the most important variables based on all possible sub‐models developed from the acorn removal explanatory variable. Before model analysis, data were tested for multicollinearity diagnostics (Zuur et al. 2009) and variability (outliers) (Zuur et al. 2010). A check for multicollinearity was performed by quantifying variance-inflation factors (VIF) and generalised variance-inflation factors (GVIF[1/(2df)]), which were calculated for each fixed factor (Fox and Monette 1992). Large VIF or GVIF values (arbitrary threshold of ≤2.5 suggesting collinearity) were sequentially dropped from further analysis. However, no collinearity was found between variables. All possible models were then ranked according to their Akaike Information Criteria corrected for small samples (AICc) (Burnham and Anderson 2002). From the set of variables selected, all the possible combinations of the predictor variables (described above) generating different biologically meaningful models were explored. For each model, the AIC weight (AICω) was calculated (total AICω adds 1) (Wagenmakers and Farrell 2004). Furthermore, if there was no clear most-parsimonious model (i.e. one or more models with an AICc difference less than 2 from the best model), the average final model was estimated from all the models (Burnham and Anderson 2002). The weight of each variable in the average final model – the AIC weight (ωi) – was calculated as the relative variable importance (Supplementary Table S1). To interpret the magnitude of each variable in the average final model, the relative importance of each variable (RVI) was weighted based on the sum of ωi for each variable. The RVI ranges from 0 to 1, so the explanatory variable was considered robust if it had an RVI > 0.9, a moderate effect between 0.6 and 0.9, a weak effect between 0.5 and 0.6, and no effect below 0.5 (Kennedy et al. 2013; Chiaradia et al. 2016). Additionally, if standard errors (s.e.) were large (1.96 × s.e. >parameter appreciation), the estimate of the parameter was considered imprecise and was removed from the final model. To perform these analyses, R software (R Development Core Team 2017) with the lme4 (Bates et al. 2015) and MuMIn (Bartoń 2016) packages was used.
Although the above-described GLMM checked the effects of the initial hypotheses, it was also possible that acorn removal depended on another variable or was affected by a series of complex indirect effects. Therefore, to control for these possible indirect and confounding variables, structural equation modelling (SEM) was used with the R package Lavaan (Rosseel 2012) to distinguish between potential causal pathways and to control for possible indirect effects. To reduce SEM complexity and for each system, two initial (maximal) models (Fig. 2a) were constructed with a variety of pathways allowing for (1) stone marten occurrence or (2) red fox occurrence and environmental and temporal variables to affect the SRFAR. All paths in the original model were treated as optional and could thus be removed during model simplification. Non-significant terms were removed until the model fit (assessed using Akaike Information Criterion) no longer improved. When the model fit did not differ significantly between two competing models (i.e. the difference in the AICc score was <2), the most parsimonious (the model with the fewest parameters) and the most appropriate models were selected (Supplementary Table S2). The appropriateness was assessed by employing the greater comparative fitness index (CFI values greater than 0.95), the root means square error of approximation (RMSEA less than 0.08), and the standardised root mean square residual (SRMR less than 0.08) criteria (Hu and Bentler 1999; Hooper et al. 2008). The CFI is an incremental fitness index that compares the fitness of a hypothetical model with that of a baseline model (i.e. the model with the worst fit). Conversely, RMSEA is an absolute fitness index, because it assesses how far a hypothesised model is from a perfect model. SRMR is a standardised effect size of the overall misfit suitable for covariance structure models.
Results
Of all the carnivores, the red fox and stone marten were the most common species in the studied areas; genet, European badger (Meles meles Linnaeus, 1758) and least weasel (Mustela nivalis Linnaeus, 1766) were also present, albeit in much smaller numbers (Puig-Gironès and Pons 2020). During the study, 196 signs (faeces) of red fox (111) and stone marten (85) were detected, 7050 acorns (11.1% of the total number supplied) were removed by rodents on the first night after acorn supply (2.22 ± 0.14 mean ( ± s.e.) acorns removed per site and night) and 902 rodents were captured (74.7% of which were wood mice, 20.3% Algerian mice, and 5% common voles).
Time since fire, plant cover, rodent abundance, and stone marten occurrence were the principal factors explaining SRFAR. Rodent abundance had the largest standardised total effect on SRFAR, and plant cover had the second-largest effect on the structural equation model (SEM) (Fig. 2). Stone marten occurrence had a significant positive effect on acorn removal rates by rodents (Fig. 2b), and red fox occurrence had no effect on SRFAR. Both predators also indirectly affected removal rates (Supplementary Tables S3, S4), which were mediated positively by rodent abundance and plant cover. On the other hand, other habitat variables such as height of vegetation, distance from rocky outcrops, and herb cover affected the number of acorns removed by rodents. The rate of acorn removal from the acorn device decreased at greater distances from shelter such as rocky outcrops. Although plant cover and height of vegetation increased over time, herb cover and height stopped increasing after 7 months and remained stable thereafter (Supplementary Fig. S1). Removal rates were especially high immediately after fire, when the availability of natural resources was practically null.
Although general linear mixed models (GLMM) showed that the greater the presence of martens, the higher the rates of acorn removal (Table 2), this effect did not seem to be sufficient or relevant (RVI less than 0.5) in the model. Furthermore, the presence of red foxes did not affect rodent foraging rates. However, a more complex habitat structure (plant cover) also prompted greater rates of acorn removal by rodents. The number of acorns removed varied in terms of the time since fire, with a notable increase in acorn removal immediately after fire to 2.5 years afterwards. In addition, a greater abundance of rodents implied that more acorns were removed (Fig. 3).
Explicative variables | Coefficient | Standard error | t-value | P-value | RVI | |
---|---|---|---|---|---|---|
Intercept | 0.14 | 0.010 | 10.19 | <0.001 | ||
Time since fire | 0.01 | 0.002 | 6.08 | <0.001 | 1.00 | |
Plant cover | 0.04 | 0.004 | 10.27 | <0.001 | 1.00 | |
Rodent relative abundance | 0.46 | 0.030 | 5.34 | <0.001 | 1.00 | |
Stone marten occurrence | 0.09 | 0.030 | 2.53 | 0.010 | 0.42 |
Summary of the selected model, derived from generalised linear mixed models (GLMM) analyses of number of acorns removed by rodents. The table shows the model parameter coefficient, its standard error (±s.e.), and the associated t-value and P-values. The relative importance of each variable (RVI) ranges from 0 to 1, so the explanatory variable was considered robust if it had an RIV > 0.9, a moderate effect between 0.6 and 0.9, a weak effect between 0.5 and 0.6, and no effect below 0.5. Intercept is the value of acorns removed by rodents when all the covariates are = 0, and P-value indicates whether it is significantly different from 0.
Model predictions of variables over rodent acorn removal rates. Marginal effects (measuring the instantaneous rate of change) of the model predictors on rodent foraging activity rates according to stone marten occurrence, plant cover, time since fire, and rodent relative abundance. Trend line and standard error shown were obtained from GLMM model estimates.
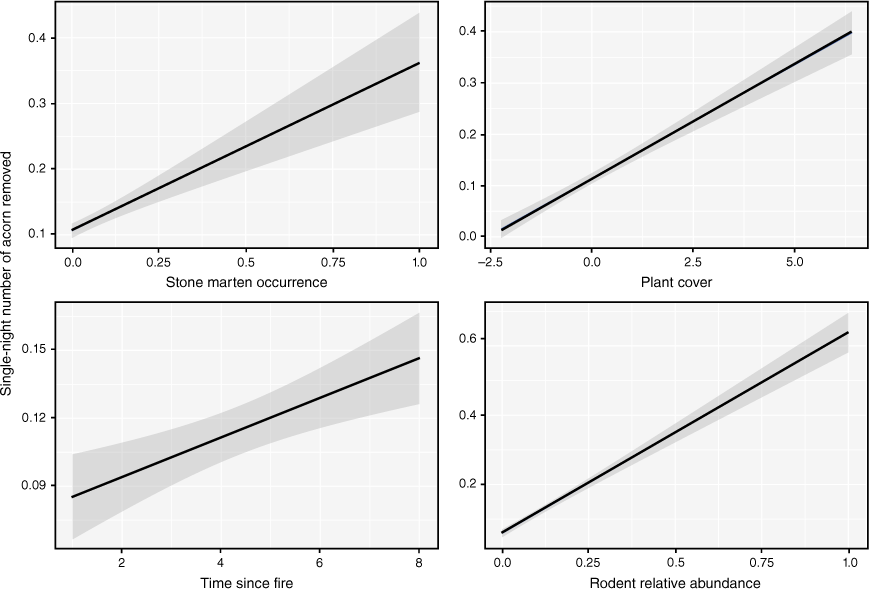
Finally, the experimental woody debris piles showed that the variables of distance to shelter and foliage cover between 25 and 50 cm (Supplementary Table S5) were the most effective in explaining foraging activity by rodents and the positive effects of protection associated with the predation risk immediately after fire. Specifically, both variables had a significant positive effect on acorn removal one and three nights after installation and on rodent captures (Fig. 4).
Acorn removal and rodent abundance over distance to shelter. The number of acorns removed by rodents (a) one night, (b) three nights after offering, and (c) the proportion of rodent abundance depending on the distance to the shelter (wood debris pile): below, at 5 m and at 10 m, and on the percentage of foliage cover between 25 and 50 cm in height are shown.
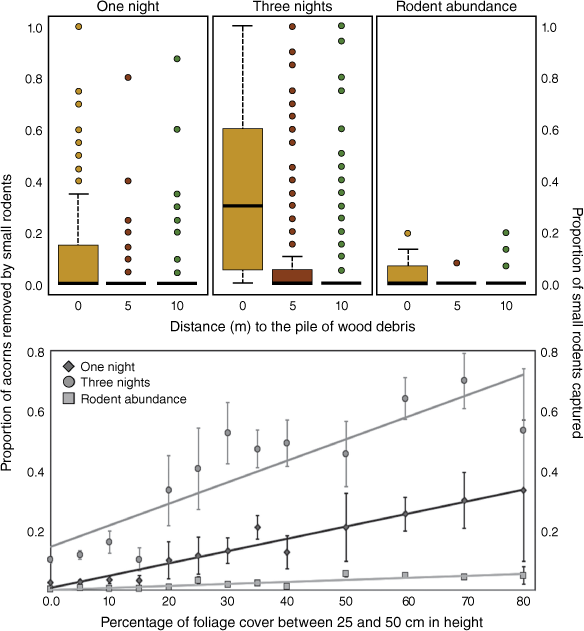
Discussion
The results identified a relationship between acorn removal by rodents and stone marten occurrence, thereby suggesting that this predator has the capacity to influence rodent foraging activity rates, which would lead us to accept the first hypothesis. Removal rates suggest that rodents select high-profitability items (acorns), spend less time exploring for food, and have greater urgency when food collecting if they have a higher danger (Leaver and Daly 2003; Chaby et al. 2015; Lichti et al. 2017). However, acorn removal increased over time where the habitat was structurally more complex because higher abundances of mice find cover in such habitats (Fordyce et al. 2016; Puig-Gironès et al. 2018; Lees et al. 2022). Conversely, this may also attract more stone martens to burnt areas (Puig-Gironès and Pons 2020; Doherty et al. 2022). In this sense, the importance of microhabitats for foraging in habitats in the experiment immediately after fires was clear. In areas with bare ground, the presence and proximity of protection is key, as has been reported by other authors (Ceradini and Chalfoun 2017; Crego et al. 2018; Abdulwahab et al. 2019). Moreover, the results show that the presence of red foxes did not affect acorn removal by rodents, as predicted in the second hypothesis. Accordingly, it could be argued that foraging activity by rodents after fires could be driven by both the direct and indirect effects of predation (i.e. by the direct presence of predators) and by the predation risk due to the scarcity of shelter and the simplicity of the burnt habitat (although that was not explicitly measured in this study).
Small mammals can detect and recognise signs that betray the presence of carnivores, so their responses to predators will vary depending on the direct risk of being captured or on indirect factors such as the quality of the available shelter or amount of moonlight (Perea et al. 2011a; Sunyer et al. 2013; Hegab et al. 2014). Stone martens are regular consumers of small mammals, which represent about 30–35% of their diets (Ballesteros et al. 2000; Padial et al. 2002). They probably take advantage of burnt areas to increase the frequency of rodents in their diet, as has been shown in other carnivores (Doherty et al. 2022). Thus, stone marten occurrence in burnt areas may force rodents to spend less time foraging to avoid increasing their exposure to predation (Brown 1988) and oblige them to select positively high-profitability acorns (McMahon et al. 2018). The presence and proximity of shelter (such as shrub cover or rocky outcrops) are very important factors that interact and affect rodent foraging activity rates. In addition, the ability of wood mice to hoard acorns may potentially affect acorn removal rates if acorn devices are placed near suitable storage locations. Consequently, greater predation risk may also increase acorn removal; i.e. wood mice will remove acorns sequentially and store them in a more secure location for later consumption. By contrast, Algerian mice tend to store acorns, often beyond the vegetation canopy when pilferage rates are low and if seed availability is low and competitors are abundant (Muñoz and Bonal 2011). Consequently, higher acorn removal may be due to increased foraging activity associated with higher rodent abundance, so predation by stone martens may not in fact be the key driver of the fate of seeds, as is suggested by the structural equation model (SEM). Additionally, the positive relationship between stone martens and acorn extraction rates is probably due to the spatial distribution of stone martens relative to mice distribution (Vergara et al. 2016; Puig-Gironès and Pons 2020). This may also explain why the risk of predation by foxes did not influence foraging rates by rodents. Foxes were more abundant in the study areas due to their preference for open habitats (Hradsky et al. 2017b; Puig-Gironès and Pons 2020).
The type of shelter (vegetation or rocks) affected foraging activity: stations with appropriate herb cover had lower acorn removal rates, lower rodent abundance and lower stone marten occurrence. By contrast, in places with shrub cover, rodent activity increased as predicted and has been reported in other studies (Smit et al. 2008). Immediately after fire, acorn removal activity was higher as a reaction to a scarcity of resources and the increased perception of the predation risk due to the lack of plant cover. Wood mice have a preference for dense shrub cover when conditions are unfavourable (Ouin et al. 2000), which affords protection and shelter (Diaz 1992). These results are thus similar to those for fragmented forests, in which a more complex habitat structure is the main driver of prey-foraging decisions (Morán-López et al. 2015). Low shelter availability minimises prey movements, and the interplay between intraspecific competition and predation risk has important effects on caching rates (Vander Wall 2010; Perea et al. 2011b; Morán-López et al. 2016). Therefore, post-fire habitat structure, microhabitats, and the predation risk affect rodent foraging activity (Conner et al. 2011; Leahy et al. 2016) and draw predators to burnt areas if prey is abundant and vulnerable to predation (McGregor et al. 2016). Throughout Catalonia, rodent foraging activity increased considerably in burnt areas 15 months after fire (Puig-Gironès et al. 2018, 2020). However, although rodent numbers remained high in burnt areas in the first weeks after fire, a lack of plant cover may slow down the colonisation by stone martens. Immediately after fire, stone martens frequented the perimeter of the burnt areas (Puig-Gironès and Pons 2020), where refuges and food resources were more available (Puig-Gironès et al. 2018). In conclusion, the results seem to indicate that in the study area, rodents reacted to the perception of the predation risk rather than to the occurrence of predators, and that stone martens and rodents have similar habitat preferences.
The increase in rodent abundance over time and the quantity of shelter (due to habitat complexity) lead to an increase in competition for resources and ultimately an increase in acorn removal rates. Functional responses of rodents are influenced by resource availability, as well as by the relative abundances of different seeds and competitors (Murray et al. 2006; Klinger and Rejmánek 2009; Ostoja et al. 2013). Foraging and hoarding locations may also vary spatially and temporally as a function of habitat structure, competition, perceived predation risk, and pilferage risks (Pérez‐Ramos et al. 2008; Muñoz et al. 2009; Muñoz and Bonal 2011; Perea et al. 2011b). However, such competition may not benefit plant regeneration, because less-intense competition and less pilferage pressure may lead hoarders to invest less heavily in seed transport, thereby resulting in lower rates of seed dispersal (Murray et al. 2006).
Implications
This study focused on the foraging activity rates of rodents under natural conditions. Seed removal plays an important role in the dispersal mechanisms of animal-dispersed plants. Thus, the rodent foraging decision-making process may be fundamental in animal-mediated seed dispersal in burnt areas. Consequently, the presence of predators may alter seed dispersal rates by prey items (Xiao et al. 2005) and thus have implications for post-fire plant and ecosystem regeneration. The wood mouse is a pioneer species in burnt habitats, which it recolonises from unburnt and ecotone areas (Torre and Díaz 2004; Puig-Gironès et al. 2018), and plays an important role as a prey item and as a seed dispersal agent (and thus in plant regeneration). Changes in feeding behaviour may decrease rodent exposure to the negative effects of the presence of predators. Mice that experienced predation pressure demonstrated improved seed movement abilities compared with mice without predator exposure, because they were capable of moving seeds over longer distances and with greater effort (Leaver and Daly 2003; Feldman et al. 2019). The results suggest a causal relationship between foraging activity rates by rodents, stone marten occurrence, and habitat complexity. Therefore, rodents play a crucial role in ecosystems as both prey and seed dispersers, being an indicator of microhabitat complexity and landscape diversity in burnt areas after fires (Stein et al. 2014; Kelly and Brotons 2017). This complexity will benefit plant regeneration by rodents and thus make a positive contribution to biodiversity in burnt habitats.
Conclusions
The effects of stone marten and red fox occurrence in the short term on Mediterranean rodent foraging activity can be summarised as follows:
The responses of predators and prey to wildfires are influenced by the way fire simplifies habitat structure, resulting in an increase in the predation risk.
Habitat complexity and interspecific competition increase rodent foraging activity rates.
Stone marten and rodents share similar habitat preferences, which means that the predator signs and the perception of predation risk contribute to the rodent foraging activity rates.
Red fox occurrence does not affect rodent foraging activity rates.
In places with high herb cover or no plant cover, acorn removal is lower than in places dominated by shrubs.
The proximity to rocky outcrops is related to greater stone marten occurrence and higher rodent foraging activity rates.
Data availability
The datasets analysed during the current study are available in the figshare repository: https://figshare.com/articles/dataset/Stone_marten_modifies_small-rodent_foraging_activity_rates_after_wildfire-Dataset/15168078. doi: 10.6084/m9.figshare.15168078.
Acknowledgements
Thanks to Pere Pons and Xavies Santos for support, long conversations, corrections, and guidelines given. Thanks also to Cristina Sanches Cascante for the rodent illustrations and M. Lockwood for the remarkable improvements in document readability, and to Gemma Vila, Pilar Eijo, and Jesús Scrofa for their help during this study. I am also grateful for different reviewers’ comments, especially reviewer 1, because they have helped me to prepare this paper in an efficient, critical, and accurate manner. This work derives from the dataset generated during my doctoral thesis.
References
Abdulwahab UA, Osinubi ST, Abalaka J (2019) Risk of predation: a critical force driving habitat quality perception and foraging behavior of granivorous birds in a Nigerian forest reserve. Avian Research 10, 33.
| Crossref | Google Scholar |
Baghli A, Engel E, Verhagen R (2002) Feeding habits and trophic niche overlap of two sympatric Mustelidae, the polecat Mustela putorius and the beech marten Martes foina. Zeitschrift für Jagdwissenschaft 48, 217-225.
| Crossref | Google Scholar |
Bates D, Mächler M, Bolker B, Walker S (2015) Fitting Linear Mixed-Effects Models Using lme4. Journal of Statistical Software 67, 1-48.
| Crossref | Google Scholar |
Berger-Tal O, Mukherjee S, Kotler BP, Brown JS (2009) Look before you leap: is risk of injury a foraging cost? Behavioral Ecology and Sociobiology 63, 1821-1827.
| Crossref | Google Scholar | PubMed |
Birtsas P, Sokos C, Exadactylos S (2012) Carnivores in burned and adjacent unburned areas in a Mediterranean ecosystem. Mammalia 76, 407-415.
| Crossref | Google Scholar |
Brown JS (1988) Patch use as an indicator of habitat preference, predation risk, and competition. Behavioral Ecology and Sociobiology 22, 37-47.
| Crossref | Google Scholar |
Ceradini JP, Chalfoun AD (2017) When perception reflects reality: Non‐native grass invasion alters small mammal risk landscapes and survival. Ecology and Evolution 7, 1823-1835.
| Crossref | Google Scholar | PubMed |
Chaby LE, Sheriff MJ, Hirrlinger AM, Braithwaite VA (2015) Does early stress prepare individuals for a stressful future? Stress during adolescence improves foraging under threat. Animal Behaviour 105, 37-45.
| Crossref | Google Scholar |
Cherry MJ, Chandler RB, Garrison EP, Crawford DA, Kelly BD, Shindle DB, Godsea KG, Miller KV, Conner LM (2018) Wildfire affects space use and movement of white-tailed deer in a tropical pyric landscape. Forest Ecology and Management 409, 161-169.
| Crossref | Google Scholar |
Chiaradia A, Ramírez F, Forero MG, Hobson KA (2016) Stable Isotopes (δ13C, δ15N) combined with conventional dietary approaches reveal plasticity in central-place foraging behavior of little penguins Eudyptula minor. Frontiers in Ecology and Evolution 3, 154.
| Crossref | Google Scholar |
Conner LM, Castleberry SB, Derrick AM (2011) Effects of mesopredators and prescribed fire on hispid cotton rat survival and cause‐specific mortality. The Journal of Wildlife Management 75, 938-944.
| Crossref | Google Scholar |
Crego RD, Jiménez JE, Rozzi R (2018) Macro-and micro-habitat selection of small rodents and their predation risk perception under a novel invasive predator at the southern end of the Americas. Mammal Research 63, 267-275.
| Crossref | Google Scholar |
Diaz M (1992) Rodent seed predation in cereal crop areas of central Spain: effects of physiognomy, food availability, and predation risk. Ecography 15, 77-85.
| Crossref | Google Scholar |
Díaz M, Torre I, Peris A, Tena L (2005) Foraging behavior of wood mice as related to presence and activity of genets. Journal of Mammalogy 86, 1178-1185.
| Crossref | Google Scholar |
Doherty TS, Davis RA, van Etten EJB (2015) A game of cat-and-mouse: microhabitat influences rodent foraging in recently burnt but not long unburnt shrublands. Journal of Mammalogy 96, 324-331.
| Crossref | Google Scholar |
Doherty TS, Geary WL, Jolly CJ, Macdonald KJ, Miritis V, Watchorn DJ, Cherry MJ, Conner LM, González TM, Legge SM, Ritchie EG, Stawski C, Dickman CR (2022) Fire as a driver and mediator of predator–prey interactions. Biological Reviews 97, 1539-1558.
| Crossref | Google Scholar | PubMed |
Embar K, Kotler BP, Mukherjee S (2011) Risk management in optimal foragers: the effect of sightlines and predator type on patch use, time allocation, and vigilance in gerbils. Oikos 120, 1657-1666.
| Crossref | Google Scholar |
Feldman M, Ferrandiz-Rovira M, Espelta JM, Muñoz A (2019) Evidence of high individual variability in seed management by scatter-hoarding rodents: does ‘personality’ matter? Animal Behaviour 150, 167-174.
| Crossref | Google Scholar |
Fernández C, Acosta FJ, Abellá G, López F, Dı’az M (2002) Complex edge effect fields as additive processes in patches of ecological systems. Ecological Modelling 149, 273-283.
| Crossref | Google Scholar |
Fordyce A, Hradsky BA, Ritchie EG, Di Stefano J (2016) Fire affects microhabitat selection, movement patterns, and body condition of an Australian rodent (Rattus fuscipes). Journal of Mammalogy 97, 102-111.
| Crossref | Google Scholar |
Fox J, Monette G (1992) Generalized Collinearity Diagnostics. Journal of the American Statistical Association 87(417), 178-183.
| Crossref | Google Scholar |
He T, Lamont BB, Pausas JG (2019) Fire as a key driver of Earth’s biodiversity. Biological Reviews 94, 1983-2010.
| Crossref | Google Scholar | PubMed |
Hegab IM, Wang A, Yin B, Yang S, Wanhong W (2014) Behavioral and neuroendocrine response of Brandt’s voles, Lasiopodomys brandtii, to odors of different species. European Journal of Wildlife Research 60, 331-340.
| Crossref | Google Scholar |
Herman CS, Valone TJ (2000) The effect of mammalian predator scent on the foraging behavior of Dipodomys merriami. Oikos 91, 139-145.
| Crossref | Google Scholar |
Hooper D, Coughlan J, Mullen M (2008) Structural equation modelling: guidelines for determining model fit. The Electronic Journal of Business Research Methods 6, 53-60.
| Crossref | Google Scholar |
Houston AI, McNamara JM, Hutchinson JM (1993) General results concerning the trade-off between gaining energy and avoiding predation. Philosophical Transactions of the Royal Society. B: Biological Sciences 341, 375-397.
| Crossref | Google Scholar |
Hradsky BA, Mildwaters C, Ritchie EG, Christie F, Di Stefano J (2017a) Responses of invasive predators and native prey to a prescribed forest fire. Journal of Mammalogy 98, 835-847.
| Crossref | Google Scholar |
Hradsky BA, Penman TD, Ababei D, Hanea A, Ritchie EG, York A, Di Stefano J (2017b) Bayesian networks elucidate interactions between fire and other drivers of terrestrial fauna distributions. Ecosphere 8, e01926.
| Crossref | Google Scholar |
Hu L-t, Bentler PM (1999) Cutoff criteria for fit indexes in covariance structure analysis: conventional criteria versus new alternatives. Structural Equation Modeling 6, 1-55.
| Crossref | Google Scholar |
Janssen A, Sabelis MW, Magalhães S, Montserrat M, van der Hammen T (2007) Habitat structure affects intraguild predation. Ecology 88, 2713-2719.
| Crossref | Google Scholar | PubMed |
Kelly LT, Brotons L (2017) Using fire to promote biodiversity. Science 355, 1264-1265.
| Crossref | Google Scholar | PubMed |
Kennedy CM, Lonsdorf E, Neel MC, Williams NM, Ricketts TH, Winfree R, Bommarco R, Brittain C, Burley AL, Cariveau D, Carvalheiro LG, Chacoff NP, Cunningham SA, Danforth BN, Dudenhöffer JH, Elle E, Gaines HR, Garibaldi LA, Gratton C, Holzschuh A, Isaacs R, Javorek SK, Jha S, Klein AM, Krewenka K, Mandelik Y, Mayfield MM, Morandin L, Neame LA, Otieno M, Park M, Potts SG, Rundlöf M, Saez A, Steffan-Dewenter I, Taki H, Viana BF, Westphal C, Wilson JK, Greenleaf SS, Kremen C (2013) A global quantitative synthesis of local and landscape effects on wild bee pollinators in agroecosystems. Ecology letters 16, 584-599.
| Crossref | Google Scholar | PubMed |
Klinger R, Rejmánek M (2009) The numerical and functional responses of a granivorous rodent and the fate of Neotropical tree seeds. Ecology 90, 1549-1563.
| Crossref | Google Scholar | PubMed |
Kotler BP, Brown JS, Dall SR, Gresser S, Ganey D, Bouskila A (2002) Foraging games between gerbils and their predators: temporal dynamics of resource depletion and apprehension in gerbils. Evolutionary Ecology Research 4, 495-518.
| Google Scholar |
Leahy L, Legge SM, Tuft K, McGregor HW, Barmuta LA, Jones ME, Johnson CN (2016) Amplified predation after fire suppresses rodent populations in Australia’s tropical savannas. Wildlife Research 42, 705-716.
| Crossref | Google Scholar |
Leaver LA, Daly M (2003) Effect of predation risk on selectivity in heteromyid rodents. Behavioural Processes 64, 71-75.
| Crossref | Google Scholar | PubMed |
Lees DM, Watchorn DJ, Driscoll DA, Doherty TS (2022) Microhabitat selection by small mammals in response to fire. Australian Journal of Zoology 69, 67-79.
| Crossref | Google Scholar |
Lichti NI, Steele MA, Swihart RK (2017) Seed fate and decision‐making processes in scatter‐hoarding rodents. Biological Reviews 92, 474-504.
| Crossref | Google Scholar | PubMed |
Lima SL, Dill LM (1990) Behavioral decisions made under the risk of predation: a review and prospectus. Canadian Journal of Zoology 68, 619-640.
| Crossref | Google Scholar |
Manson RH, Stiles EW (1998) Links between microhabitat preferences and seed predation by small mammals in old fields. Oikos 82, 37-50.
| Crossref | Google Scholar |
McGregor HW, Legge S, Jones ME, Johnson CN (2016) Extraterritorial hunting expeditions to intense fire scars by feral cats. Scientific Reports 6, 22559.
| Crossref | Google Scholar | PubMed |
McMahon JD, Lashley MA, Brooks CP, Barton BT (2018) Covariance between predation risk and nutritional preferences confounds interpretations of giving‐up density experiments. Ecology 99, 1517-1522.
| Crossref | Google Scholar | PubMed |
Monimeau L, Mouillot D, Fons R, Prodon R, Marchand B (2002) Impact of prescribed burning on the survival rates of the wood mouse (Apodemus sylvaticus). Acta Oecologica 23, 51-58.
| Crossref | Google Scholar |
Morán-López T, Fernández M, Alonso CL, Flores-Rentería D, Valladares F, Díaz M (2015) Effects of forest fragmentation on the oak–rodent mutualism. Oikos 124, 1482-1491.
| Crossref | Google Scholar |
Morán-López T, Wiegand T, Morales JM, Valladares F, Díaz M (2016) Predicting forest management effects on oak–rodent mutualisms. Oikos 125, 1445-1457.
| Crossref | Google Scholar |
Morris G, Hostetler JA, Oli MK, Conner LM (2011) Effects of predation, fire, and supplemental feeding on populations of two species of Peromyscus mice. Journal of Mammalogy 92, 934-944.
| Crossref | Google Scholar |
Muñoz A, Bonal R (2011) Linking seed dispersal to cache protection strategies. Journal of Ecology 99, 1016-1025.
| Crossref | Google Scholar |
Muñoz A, Bonal R, Díaz M (2009) Ungulates, rodents, shrubs: interactions in a diverse Mediterranean ecosystem. Basic and Applied Ecology 10, 151-160.
| Crossref | Google Scholar |
Murray AL, Barber AM, Jenkins SH, Longland WS (2006) Competitive environment affects food-hoarding behavior of Merriam’s kangaroo rats (Dipodomys merriami). Journal of Mammalogy 87, 571-578.
| Crossref | Google Scholar |
Ostoja SM, Schupp EW, Durham S, Klinger R (2013) Seed harvesting is influenced by associational effects in mixed seed neighbourhoods, not just by seed density. Functional Ecology 27, 775-785.
| Crossref | Google Scholar |
Ouin A, Paillat G, Butet A, Burel F (2000) Spatial dynamics of wood mouse (Apodemus sylvaticus) in an agricultural landscape under intensive use in the Mont Saint Michel Bay (France). Agriculture, Ecosystems & Environment 78, 159-165.
| Crossref | Google Scholar |
Padial JM, Avila E, Sanchez JM (2002) Feeding habits and overlap among red fox (Vulpes vulpes) and stone marten (Martes foina) in two Mediterranean mountain habitats. Mammalian Biology 67, 137-146.
| Crossref | Google Scholar |
Perea R, González R, San Miguel A, Gil L (2011a) Moonlight and shelter cause differential seed selection and removal by rodents. Animal Behaviour 82, 717-723.
| Crossref | Google Scholar |
Perea R, San Miguel A, Gil L (2011b) Acorn dispersal by rodents: the importance of re-dispersal and distance to shelter. Basic and Applied Ecology 12, 432-439.
| Crossref | Google Scholar |
Pérez‐Ramos IM, Urbieta IR, Marañón T, Zavala MA, Kobe RK (2008) Seed removal in two coexisting oak species: ecological consequences of seed size, plant cover and seed‐drop timing. Oikos 117, 1386-1396.
| Crossref | Google Scholar |
Persson L, Eklov P (1995) Prey refuges affecting interactions between piscivorous perch and juvenile perch and roach. Ecology 76, 70-81.
| Crossref | Google Scholar |
Pons J, Pausas JG (2007) Rodent acorn selection in a Mediterranean oak landscape. Ecological Research 22, 535-541.
| Crossref | Google Scholar |
Prodon R, Lebreton J-D (1981) Breeding avifauna of a Mediterranean succession: the holm oak and cork oak series in the eastern Pyrenees, 1. Analysis and modelling of the structure gradient. Oikos 37, 21-38.
| Crossref | Google Scholar |
Puig-Gironès R, Pons P (2020) Mice and habitat complexity attract carnivorans to recently burnt forests. Forests 11, 855.
| Crossref | Google Scholar |
Puig-Gironès R, Pons P (2023) Mice population dynamics and structure over time and space after wildfires. Journal of Zoology 1-14.
| Crossref | Google Scholar |
Puig-Gironès R, Clavero M, Pons P (2018) Importance of internal refuges and the external unburnt area in the recovery of rodent populations after wildfire. International Journal of Wildland Fire 27, 425-436.
| Crossref | Google Scholar |
Puig-Gironès R, Imbeau L, Clavero M, Rost J, Pons P (2020) Does post-fire salvage logging affect foraging activity by rodents. European Journal of Forest Research 139, 777-790.
| Crossref | Google Scholar |
Rosalino LM, Ferreira D, Leitão I, Santos-Reis M (2011) Usage patterns of Mediterranean agro-forest habitat components by wood mice Apodemus sylvaticus. Mammalian Biology 76, 268-273.
| Crossref | Google Scholar |
Rosalino LM, Santos‐Reis M (2009) Fruit consumption by carnivores in Mediterranean Europe. Mammal Review 39, 67-78.
| Crossref | Google Scholar |
Rosseel Y (2012) lavaan: an R package for structural equation modeling. Journal of Statistical Software 48, 1-36.
| Crossref | Google Scholar |
Ruiz-Capillas P, Mata C, Malo JE (2013) Community response of mammalian predators and their prey to motorways: implications for predator–prey dynamics. Ecosystems 16, 617-626.
| Crossref | Google Scholar |
Sainz-Elipe S, Sáez-Durán S, Galán-Puchades MT, Fuentes MV (2012) Small mammal (Soricomorpha and Rodentia) dynamics after a wildfire in a Mediterranean ecosystem. Mammalia 76, 251-259.
| Crossref | Google Scholar |
Sikes RS, Gannon WL, , The Animal Care, Use Committee of the American Society of Mammalogists (2011) Guidelines of the American Society of Mammalogists for the use of wild mammals in research. Journal of Mammalogy 92, 235-253.
| Crossref | Google Scholar |
Smit C, den Ouden J, Díaz M (2008) Facilitation of Quercus ilex recruitment by shrubs in Mediterranean open woodlands. Journal of Vegetation Science 19, 193-200.
| Crossref | Google Scholar |
Stein A, Gerstner K, Kreft H (2014) Environmental heterogeneity as a universal driver of species richness across taxa, biomes and spatial scales. Ecology Letters 17, 866-880.
| Crossref | Google Scholar | PubMed |
Sunyer P, Muñoz A, Bonal R, Espelta JM (2013) The ecology of seed dispersal by small rodents: a role for predator and conspecific scents. Functional Ecology 27, 1313-1321.
| Crossref | Google Scholar |
Torre I, Díaz M (2004) Small mammal abundance in Mediterranean post-fire habitats: a role for predators? Acta Oecologica 25, 137-142.
| Crossref | Google Scholar |
Vander Wall SB (2010) How plants manipulate the scatter-hoarding behaviour of seed-dispersing animals. Philosophical Transactions of the Royal Society. B: Biological Sciences 365, 989-997.
| Crossref | Google Scholar | PubMed |
Verdolin JL (2006) Meta-analysis of foraging and predation risk trade-offs in terrestrial systems. Behavioral Ecology and Sociobiology 60, 457-464.
| Crossref | Google Scholar |
Vergara M, Cushman SA, Urra F, Ruiz-González A (2016) Shaken but not stirred: multiscale habitat suitability modeling of sympatric marten species (Martes martes and Martes foina) in the northern Iberian Peninsula. Landscape Ecology 31, 1241-1260.
| Crossref | Google Scholar |
Virgós E, Llorente M, Cortés Y (1999) Geographical variation in genet (Genetta genetta L.) diet: a literature review. Mammal Review 29, 117-126.
| Crossref | Google Scholar |
Wagenmakers E-J, Farrell S (2004) AIC model selection using Akaike weights. Psychonomic Bulletin & Review 11, 192-196.
| Crossref | Google Scholar | PubMed |
Xiao Z, Zhang Z, Wang Y (2005) Effects of seed size on dispersal distance in five rodent-dispersed fagaceous species. Acta Oecologica 28, 221-229.
| Crossref | Google Scholar |
Zuur AF, Ieno EN, Elphick CS (2010) A protocol for data exploration to avoid common statistical problems. Methods in Ecology and Evolution 1, 3-14.
| Crossref | Google Scholar |