Field-based generic empirical flame length–fireline intensity relationships for wildland surface fires
Carlos G. Rossa



A
B
Abstract
Fireline intensity (If) quantifies the power of the fireline and is used for various purposes. If and flame length (Lf) are relatable to each other using an empirical power function, which has been considered fuel-specific.
The aim of this study was to develop generic Lf − If relationships based on a robust set of field head fires from the literature (n = 797) conducted worldwide in forest, shrubland and grassland.
Lf was determined from the base of the fuel bed for comparability across fires in different fuel heights, and the effect of vegetation type was examined.
Although If could be approximately described using the same function in forest and shrubland, fires in grassland required different fitted coefficients; we speculate that fuel particles’ surface area-to-mass ratio is the main fuel metric influencing flame structure.
Fuel-generic relationships for If are reasonably accurate and encompass the high end of surface fire If. Previous studies suggested their unviability, most likely because of limitations in the number of observations and data ranges, difficulty in objectively measuring Lf and variation in Lf definition.
The generic relationships presented in this work will be of interest for research and management purposes when specific models for If are non-existent.
Keywords: combustion metrics, fire behaviour, fire management, forest, fuel metrics, grassland, head fires, shrubland, surface area-to-mass ratio.
Introduction
Fireline intensity (If), also referred to as frontal fire intensity (usually in units of kW m−1), quantifies the energy released per unit time (power) by unit fireline length of a vegetation fire and has been described as ‘the single most valid characteristic of a fire’s general behaviour’ (Alexander 1982, p. 350). If is useful for various ends, namely the appraisal of aboveground fire effects (e.g. Weber et al. 1987), or as a guide for fire suppression difficulty (Hirsch and Martell 1996). By definition (Byram 1959), If is calculated as
where H, w and R are, respectively, fuel heat yield, fuel load consumed by flaming combustion and fire spread rate. Byram (1959) established flame length (Lf) as a power function of If. The reciprocal of the relationship, or of empirically derived relationships of the same form, has been subsequently widely used to estimate If from Lf (Alexander and Cruz 2012):
where a and b are fitted coefficients. The simplicity of Eqn 2 is appealing, but the existence of a wide variety of reported relationships has led to the assumption that its coefficients must be fuel-dependent (Alexander 1982; Cheney 1990; Alexander and Cruz 2012). Yet, the usual practice, namely in North-American fire modelling systems (Andrews 2018), is to assume a single Lf − If equation for general use regardless of fuel bed nature and structure.
Some of the existing Lf − If models for surface head fires have been derived from laboratory data in both natural fuel beds, like slash (Anderson et al. 1966), and artificial fuels, like wood cribs (Fons et al. 1963; Thomas 1963) and excelsior (Weise and Biging 1996). However, the majority of available Lf − If relationships were obtained from field fires in forest (Byram 1959; Nelson 1980; Burrows 1994; Fernandes et al. 2009), shrubland (Van Wilgen 1986; Catchpole et al. 1998; Vega et al. 1998; Fernandes et al. 2000) and grassland (Nelson 1980; Clark 1983). A visual assessment of all plotted functions (Alexander and Cruz 2012) for field head fires seems to suggest that grassland requires shorter flames to produce the same If of fires in forest and shrubland, but the extant wide variation among the fitted relationships is not conclusive in this regard.
A proper evaluation of the feasibility of using generic Lf − If relationships is impaired mostly by three limiting factors. Firstly, both laboratory and field fire measurements have important shortcomings: (1) laboratory experiments have scale issues and are limited in terms of the maximum Lf values that can be obtained; and (2) in field fires, it is usually difficult to accurately assess vegetation metrics and the amount of fuel consumed. Secondly, individual studies are usually: (1) limited in terms of the number of observations; and (2) developed for specific fuel complexes and thus limited in terms of the range in fuel structure descriptors. Thirdly, estimates of average Lf can be very subjective because: (1) flame pulsation causes great variations in instantaneous Lf (Byram and Nelson 1970); and (2) Lf is often assessed by visual estimation, which is known to vary among observers (Johnson 1982).
An additional difficulty in evaluating Lf is that many studies do not specify if the measure is taken from the fuel bed base or from its top (e.g. Fons et al. 1963; Anderson et al. 1966; Clark 1983). This typically makes little difference in litter fuels because fuel height (h) is usually small when compared with Lf but has the potential to cause great discrepancies in deeper fuel complexes, such as tall shrubland. Whether it is possible or not to derive generic Lf − If relationships, an appropriate comparison between fuel-specific functions can only be made if Lf is assessed from the base of the fuel bed, as proposed for example by Rothermel and Deeming (1980). Because Lf is a visual manifestation of the combustion rate, i.e. the amount of fuel burnt per unit time (Byram 1959) and thus of the released thermal power, we must consider all visible flame, including within the fuel bed, to correspond as accurately as possible with the actual combustion rate. Fig. 1 shows the flame geometry of a wind-driven head fire (Rossa and Fernandes 2018a), as considered in the present study; favourable slope produces a similar flame configuration (Dupuy et al. 2011), because its effect is equivalent to that of wind in tilting fire towards the unburned fuel.
Schematic representation of the cross-section of a wind-driven head fire showing flame length (Lf) as considered in the present study, i.e. assessed along its axis from the tip of the flame to the base of the fuel bed.
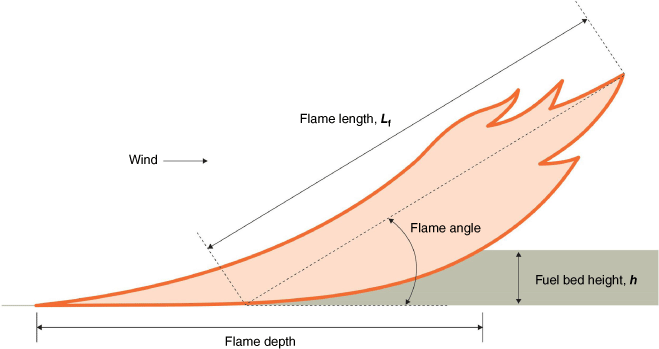
The Lf − If relationship of Byram (1959) is currently almost universally used. However, it was seemingly developed from a limited number of fires (n = 41) in a single fuel type (pine forest with a grassy understorey), and the validity of its widespread application has never been properly evaluated. Lf − If functions, suitable for a wide diversity of fuel complexes, would thus be of great interest for both scientific and operational purposes. Here we have developed such relationships, based on a large number of well-documented worldwide experimental fires in forest, shrubland and grassland.
Methods
Data sources
The BONFIRE worldwide database (Fernandes et al. 2020) was the source of data for this study. BONFIRE comprises outdoors fire behaviour characteristics and the corresponding fire environment descriptors gathered from an exhaustive search of the peer-reviewed and grey literature. Included are data pertaining to the forward spread of individual experimental fires in any vegetation type, as well as from prescribed fire operations and wildfires, but excluding fires initiated by exceedingly narrow ignition lines (<2-m length) or featuring potential interaction between fire fronts.
Each BONFIRE record includes data reliability ratings as per Cheney et al. (2012) and was attributed a generic vegetation type (forest, shrubland, grassland), a broad fuel type (e.g. long needle conifer) and a fuel complex defined by the fuel layer(s) carrying the fire (e.g. litter + shrub). The compiled records were considered eligible for our analysis if they pertained to an experimental study represented by a minimum of three fires and if rated with high reliability. After applying these restrictions, we identified just 797 fires for which all variables required by the analysis (w, R and Lf) were available. Nonetheless, this BONFIRE subset spans worldwide and covers a diverse array of fuel types and fuel complexes, as can be seen in Table 1, where the original data sources are indicated.
Vegetation type | Broad fuel type (fuel complex) | Reference | Country | n | h (m) | w (kg m−2) | ρb (kg m−3) | R (m s−1) | Lf (m) | If (kW m−1) | |
---|---|---|---|---|---|---|---|---|---|---|---|
Forest | Deciduous broadleaf (litter) | Bova and Dickinson (2008) | USA | 12 | 0.02–0.29 | 0.17–1.05 | 2.57–9.09 | 0.01–0.13 | 1.17–1.44 | 70–1544 | |
Deciduous broadleaf (litter + shrub) | Patterson et al. (2005) | USA | 3 | 0.52–1.03 | 0.50–0.55 | 0.54–0.99 | 0.01–0.08 | 1.09–4.5 | 107–727 | ||
Eucalypt (litter) | Pinto et al. (2013) | Portugal | 22 | 0.04–0.13 | 0.33–1.78 | 6.42–19.36 | 0.00–0.06 | 0.18–1.05 | 18–523 | ||
Eucalypt (litter + grass) | Lacy (2008) | Australia | 29 | 0.1–0.64 | 0.32–1.26 | 0.81–8.34 | 0.00–0.09 | 0.5–2.54 | 28–1084 | ||
Eucalypt (litter + grass/shrub) | Gould et al. (2008) | Australia | 95 | 0.01–0.56 | 0.34–2.15 | 1.62–72.74 | 0.00–0.32 | 0.29–8.94 | 53–7379 | ||
Long needle conifer (litter) | Botelho et al. (1994) | Portugal | 3 | 0.05–0.06 | 0.49–0.64 | 9.63–12.50 | 0.01–0.02 | 0.33–0.79 | 111–191 | ||
Fernandes et al. (2009) | Portugal | 32 | 0.02–0.21 | 0.28–1.20 | 4.04–18.00 | 0.00–0.11 | 0.14–2.93 | 29–1220 | |||
Sparks et al. (2017) | USA | 9 | 0.05–0.08 | 0.20–2.30 | 2.38–40.35 | 0.00–0.10 | 0.31–1.12 | 22–859 | |||
Long needle conifer (litter + grass) | Fernandes et al. (2009) | Portugal | 6 | 0.21–0.25 | 0.79–1.53 | 3.20–6.24 | 0.01–0.23 | 1.15–4.36 | 154–3410 | ||
Long needle conifer (litter + grass/shrub) | Alvarado (1986) | Mexico | 38 | 0.07–0.40 | 0.09–1.45 | 0.46–12.57 | 0.00–0.09 | 0.16–2.36 | 9–957 | ||
Fernandes et al. (2009) | Portugal | 13 | 0.27–0.33 | 0.81–1.21 | 2.45–3.99 | 0.01–0.14 | 1.03–3.70 | 195–1964 | |||
Long needle conifer (litter + shrub) | Botelho (1996) | Portugal | 1 | 0.19 | 0.59 | 3.06 | 0.01 | 1.00 | 99 | ||
Botelho et al. (1994) | Portugal | 5 | 0.21–0.50 | 0.60–1.29 | 1.68–3.58 | 0.01–0.07 | 0.94–3.18 | 84–982 | |||
Fernandes et al. (2004) | Portugal | 3 | 0.09–0.45 | 0.80–2.47 | 5.48–9.77 | 0.02–0.10 | 1.31–4.67 | 267–2419 | |||
Fernandes et al. (2009) | Portugal | 36 | 0.33–0.61 | 0.82–1.85 | 1.83–3.74 | 0.01–0.13 | 0.88–4.66 | 156–2415 | |||
Patterson et al. (2005) | USA | 3 | 0.23–0.61 | 0.55–0.77 | 1.26–2.65 | 0.02–0.14 | 0.64–3.39 | 167–1764 | |||
UTAD unpublished data on file | Portugal | 7 | 0.10–0.45 | 0.48–2.26 | 2.34–5.02 | 0.01–0.07 | 0.61–1.96 | 192–1249 | |||
Mixed conifer–deciduous (litter) | Norton-Jansen (2005) | USA | 3 | 0.05–0.09 | 0.75–0.81 | 8.23–15.55 | 0.02–0.03 | 0.34–0.55 | 253–346 | ||
Mixed conifer–deciduous (litter + shrub) | Norton-Jansen (2005) | USA | 3 | 0.17–0.20 | 1.32–1.56 | 6.79–9.33 | 0.02–0.07 | 1.08–1.57 | 442–1473 | ||
Short needle conifer (litter) | Lawson (1972) | Canada | 8 | 0.02 | 0.97 | 49.43 | 0.01 | 0.35–0.87 | 90–212 | ||
Short needle conifer (litter + moss/lichen) | Lawson (1972) | Canada | 20 | 0.02 | 0.77–0.97 | 35.5–49.43 | 0.00–0.03 | 0.11–1.85 | 60–529 | ||
Conifer slash (slash) | Kucuk et al. (2008) | Turkey | 30 | 0.10–0.38 | 0.64–4.99 | 4.57–17.08 | 0.00–0.05 | 0.20–2.58 | 36–4273 | ||
Brown (1972) | USA | 25 | 0.16–0.89 | 0.54–2.10 | 1.85–7.41 | 0.00–0.04 | 0.33–2.87 | 15–1308 | |||
Shrubland | Deciduous shrub (litter + shrub) | Patterson et al. (2005) | USA | 3 | 0.20–0.84 | 0.54–0.65 | 0.67–2.77 | 0.01–0.07 | 0.47–1.97 | 93–672 | |
Evergreen shrub (grass + shrub) | Anderson et al. (2015) | Australia | 2 | 0.40 | 1.62–1.62 | 4.05–4.05 | 0.13–0.14 | 8.30–8.30 | 3582–3716 | ||
Anderson et al. (2015) | New Zealand | 11 | 0.30–2.50 | 0.10–2.22 | 0.12–1.17 | 0.02–0.42 | 1.30–9.90 | 99–15 326 | |||
Marsden-Smedley and Catchpole (1995) | Australia | 85 | 0.20–0.50 | 0.37–2.51 | 1.52–6.28 | 0.00–0.24 | 0.70–10.91 | 37–4905 | |||
Evergreen shrub (litter/moss + shrub) | Davies and Legg (2011) | Scotland | 19 | 0.16–0.45 | 0.72–1.67 | 3.34–5.38 | 0.01–0.20 | 0.59–3.30 | 159–4337 | ||
Evergreen shrub (shrub) | Anderson et al. (2015) | Australia | 13 | 0.25–4.80 | 0.30–3.82 | 0.20–3.53 | 0.05–0.43 | 1.85–16.70 | 249–27 127 | ||
Anderson et al. (2015) | New Zealand | 15 | 0.60–3.60 | 1.16–4.81 | 0.57–3.22 | 0.14–0.45 | 3.10–14.10 | 3620–25 259 | |||
Anderson et al. (2015) | Portugal | 19 | 0.28–1.90 | 0.53–2.79 | 0.86–3.00 | 0.01–0.33 | 1.20–7.80 | 130–10 061 | |||
Fernandes (2001) | Portugal | 6 | 0.46–0.63 | 1.74–4.09 | 3.63–6.89 | 0.07–0.15 | 3.68–5.46 | 2540–7340 | |||
Van Wilgen et al. (1985) | South Africa | 6 | 0.84–1.15 | 0.59–1.48 | 0.51–1.54 | 0.21–0.47 | 2.86–5.35 | 3015–9064 | |||
Open evergreen shrub (grass + shrub) | Bushey (1985) | USA | 6 | 1.32–2.29 | 0.05–0.29 | 0.03–0.20 | 0.03–0.55 | 1.72–3.97 | 24–2677 | ||
Open evergreen shrub (shrub) | McCaw (1997) | Australia | 17 | 0.21–2.40 | 0.48–1.67 | 0.52–2.44 | 0.02–0.72 | 0.65–13.4 | 119–14 737 | ||
Open evergreen shrub (shrub) | Van Wilgen et al. (1985) | South Africa | 5 | 1.00–1.23 | 1.05–1.70 | 0.94–1.38 | 0.04–0.55 | 2.61–7.57 | 752–15 524 | ||
Grassland | Cereal stubble (grass) | New Zealand Forest Research (2002) | New Zealand | 27 | 0.10–0.50 | 0.19–0.75 | 0.94–1.97 | 0.27–1.78 | 0.62–6.00 | 1029–17 065 | |
Grass (grass) | Van Wilgen and Wills (1988) | South Africa | 10 | 0.44 | 0.37–0.37 | 0.85–0.85 | 0.04–1.00 | 1.44–4.94 | 231–5562 | ||
Clark (1983) | USA | 60 | 0.04–0.57 | 0.10–0.89 | 0.75–4.80 | 0.01–0.92 | 0.15–4.76 | 52–10 492 | |||
Cruz et al. (2018) | Australia | 14 | 0.29–0.90 | 0.67–1.04 | 0.85–2.83 | 0.37–1.18 | 3.00–5.23 | 5233–14 890 | |||
Hély et al. (2003) | Zambia | 8 | 0.20–0.44 | 0.36–0.60 | 0.89–1.82 | 0.02–1.76 | 0.50–4.61 | 106–9590 | |||
Kunst et al. (2001) | Argentina | 7 | 0.36–0.66 | 0.20–0.78 | 0.55–1.20 | 0.21–0.56 | 4.00–4.91 | 630–6427 | |||
Pearce et al. (2009) | New Zealand | 4 | 0.48–0.56 | 3.25–3.76 | 6.63–7.56 | 0.10–0.14 | 2.48–3.02 | 5458–7641 | |||
Sneeuwjagt and Frandsen (1977) | USA | 42 | 0.09–0.53 | 0.04–0.47 | 0.44–2.33 | 0.00–1.02 | 0.21–3.58 | 1–6353 | |||
Grass (grass + shrub) | Kunst et al. (2001) | Argentina | 12 | 0.45 | 0.66–1.24 | 1.47–2.75 | 0.23–0.70 | 2.10–3.95 | 2864–12 219 |
Variables used are: h (m), fuel bed height; w (kg m−2), fine fuel load; ρb (kg m−3), fuel bed density; R (m s−1), fire spread rate; Lf (m), average flame length; If (kW m−1), fireline intensity.
Fireline intensity and flame length
If was computed as per Eqn 1. H can be obtained as a fraction of fuel heat of combustion determined in a bomb calorimeter, accounting for the heat losses occurring during outdoors combustion, like moisture vaporisation, incomplete combustion and radiative heat losses by the convection column (Byram 1959). We retrieved average values for the heat of combustion of forest and shrubland fuels (22 111 kJ kg−1) and grass fuels (19 850 kJ kg−1) from Susott (1982). We considered that the fraction of the heat of combustion assumed to be H was 0.75, which was roughly the value calculated by Byram (1959) and Nelson and Adkins (1986, 1988). As a result, we obtained H = 16 583 kJ kg−1 for forest and shrubland fuels and H = 14 888 kJ kg−1 for grass fuels.
Quantifying precisely how much fuel is consumed in the active flame zone of a field fire is nearly impossible. We therefore adopted the common and reasonable assumption that w equals surface fine (<6 mm thickness) fuel load (live and dead) and that most of the consumption of coarser fuels (when existent) occurs in the form of residual or glowing combustion and, therefore, has a minor contribution to the flame generated at the fire front (Alexander 1982).
Although Lf is typically measured above the fuel bed by default (Alexander 1982), Eqn 2 uses Lf measured from the base of the fuel bed, being assessed from the tip of the flame along its axis (Fig. 1). Because in many fires flame angle was not provided, we estimated Lf by adding fuel height h, instead of h/sin(flame angle), to the reported flame length. With increasing wind or slope, flames tilt towards the unburned fuel, flame angle diminishes and Lf becomes progressively underestimated. However, because Lf is expected to increase, the error also becomes less significant. The number of fires was unevenly distributed across the If range, with many more low-If fires. For this reason, for each vegetation type, we ordered the experiments by increasing If, grouped the fires into 100 kW m−1 bins ([0–100], [100–200], etc.) and averaged Lf and If within those groups to obtain a better distribution of data points across the If range, and thus more reliable fits.
Data analysis
Coefficients a and b were determined by fitting the log-transformed form of Eqn 2 by least-squares (e.g. Cheney et al. 2012). We fitted Eqn 2 using the full dataset and examined the effect of vegetation type (forest, shrubland, grassland) as a categorical variable (significant at P < 0.05) to probe for the possibility of using the same coefficients for more than one fuel complex, and we proceeded with model development based on the results from this analysis. The bias from model back-transformation was corrected according to Snowdon (1991).
Predictions were evaluated based on the coefficient of determination (R2), root mean square error (RMSE), mean absolute error (MAE) and mean bias error (MBE). Residuals were checked for normality with the Shapiro–Wilk test (P > 0.05) or, when significance was below the threshold value, for approximate normality by visually inspecting their histograms. Independence from predicted values was evaluated by correlation analysis. We determined the reciprocals of the developed models (Lf as a function of If). We also plotted Byram’s (1959) Lf − If relationship with the present models for a graphical comparison.
Results
Parameter ranges and data points
The wide span in fuel bed structure and flame dimensions inherent to the dataset can be inferred from the h and Lf ranges (Table 1): 0.1–1.0 m and 0.1–8.9 m for forest (n = 406), 0.16–4.8 m and 0.5–16.7 m for shrubland (n = 207) and 0.04–0.9 m and 0.2–6 m for grassland (n = 184). Wind speed and slope angle were not reported in all studies. For those who did, ranges were 0.5–22 km h−1 (measured at 1.5–2-m height) and 0–22° in forest, 0.3–33.9 km h−1 and 0–30° in shrubland and 2.5–53.1 km h−1 and 0–7° in grassland. As a result of averaging Lf and If within 100 kW m−1 intervals, the distribution of data points across the If range became much more balanced. We obtained 45 Lf − If data points for forest, 73 for shrubland and 85 for grassland.
Model development and evaluation
In the joint analysis of the full dataset, vegetation type was significant, yet differences between forest and shrubland were small, with the confidence interval estimates for the latter totally included within the confidence interval for the former, so we separately fitted Eqn 2 to forest–shrubland data and to grassland data. The back-transformed forest–shrubland equation (Fig. 2) accounted for 76.6% (Table 2) of the existing variability, with an MAE of 1812 kW m−1. The explanation of variability (82.1%) was higher for grasslands (Fig. 3) and MAE was slightly lower (1734 kW m−1). MBE was approximately zero in both cases. Residuals were approximately normally distributed for forest–shrubland, normally distributed for grassland, and weakly correlated with predicted values. Reciprocals of Eqn 2 are Lf = 0.04001 If0.5846 for forest–shrubland and Lf = 0.03888 If0.5111 for grassland. Byram’s (1959) equation is Lf = 0.0775 If0.46; its reciprocal yields similar results to the equation derived for grassland (Fig. 4) but severely, and increasingly, overestimates If for flames longer than ~2 m in forest and shrubland.
Eqn 2 fit and evaluation for forest–shrubland after back transformation: (a) Fireline intensity (If) as a function of flame length (Lf), dashed lines are the 95% CI; and (b) observed vs predicted If values. Fitted coefficients and evaluation metrics are given in Table 2.
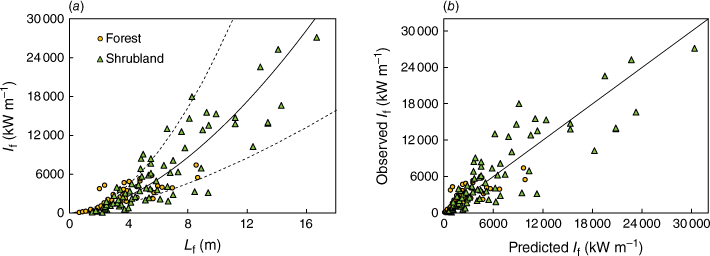
Vegetation type | n data points | a | b | R2 | RMSE (kW m−1) | MAE (kW m−1) | MBE (kW m−1) | |
---|---|---|---|---|---|---|---|---|
Forest–shrubland | 118 | 246.0 (186.9–323.9) | 1.711 (1.536–1.885) | 0.766 | 2689 | 1812 | 0 | |
Grassland | 85 | 574.2 (468.9–703.1) | 1.956 (1.757–2.156) | 0.821 | 2368 | 1734 | 0 |
95% confidence intervals for fitted coefficients a and b are shown in parenthesis. R2, coefficient of determination; RMSE, root mean square error; MAE, mean absolute error; MBE, mean bias error.
Eqn 2 fit and evaluation for grassland after back-transformation: (a) Fireline intensity (If) as a function of flame length (Lf), dashed lines are the 95% CI; and (b) observed vs predicted If values. Fitted coefficients and evaluation metrics are given in Table 2.
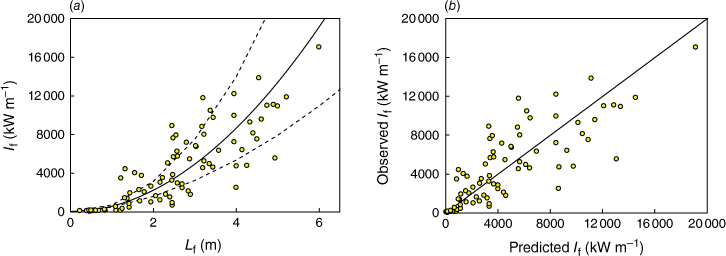
Graphical representation of Byram’s (1959) fireline intensity (If)–flame length (Lf) relationship (If = 259.8 Lf2.174) and the corresponding models developed in the present study (Table 2).
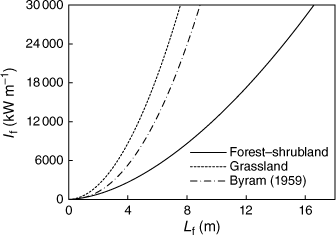
Discussion
Model performance and influence of fuel properties
The Lf − If models performed well, explaining about 80% of the observed variability and providing unbiased estimates. In forest and shrubland fires, If can be estimated from Lf using the same empirical relationship. Fires in grassland need different fitted coefficients, thus revealing differences in flame characteristics, which have already been noted by Cheney (1990). However, Lf scales approximately with the square root of If in both cases, as expected from theory (Nelson 1980).
Fuel consumption will not exactly equal fine fuel load. Consequently, If calculation with the latter in lieu of the former is expected to introduce uncertainty when fitting a Lf − If relationship, but judging from model evaluation statistics, this simplification was not an influent factor. The wide data range in w and fuel bed density (ρb), shown by their interquartile ranges for forest (0.66–1.3 kg m−2, 2.9–11.0 kg m−3), shrubland (0.76–1.7 kg m−2, 1.3–3.7 kg m−3) and grassland (0.25–0.67 kg m−2, 1.0–2.0 kg m−3), and the fact that these metrics are not required as predictors to produce accurate Lf − If models, leaves no doubt about their reduced influence on flame structure. Also, it is reasonable to assume that variation in fuel bed structure descriptors (like h, w and ρb) would similarly affect flame properties in forest–shrubland or grassland. On the other hand, a fuel metric that fundamentally distinguishes forest–shrubland and grassland, and significantly influences R, is the surface area-to-mass ratio of the fuel particles (Sm) (Rossa and Fernandes 2018a). R increases with Sm, which is much higher for grassland. In fact, the Sm of mediterranean forest–shrubland foliage follows a normal distribution, with an average value of 8.2 m2 kg−1, contrasting with the much higher values of 20–40 m2 kg−1 observed for herbaceous fuels (Rossa and Fernandes 2018b).
Lf is a visible manifestation of the hot combustion gases, and the chemical composition of wildland fuels is very similar, thus producing little variation between H values. Thus, the same ‘amount of flame’ should be produced for the same If, regardless of the fuel bed. Contrasting Sm fuels will produce structurally different flames, which seem to become more compact as Sm increases. Additional evidence supports this idea: based on laboratory experiments in conifer slash fuel, Anderson et al. (1966) obtained an Lf − If relationship that predicts Lf = 10 m to yield If = 2000 kW m−1, whereas our models result in Lf = 4 m for forest–shrubland (Fig. 2a) and Lf = 2 m for grassland (Fig. 3a). Even the thinnest woody fuels within a slash fuel bed have very low Sm; for example, a 4 mm round eucalypt twig will have Sm = 1.5 m2 kg−1, contrasting with typical values of 8.2 m2 kg−1 for foliar fuels and 30 m2 kg−1 for grass fuels (Rossa and Fernandes 2018b).
Model applicability and application
Although our models performed better within the fire behaviour range most commonly associated to surface fire spread, say up to an If of 4000 kW m−1, a relevant feature of the fitted equations is the inclusion of high-intensity fire data in their development. For more flammable fuel complexes, namely tall shrublands and forests with a well-developed woody understorey, this is expected to improve Lf or If estimates over those afforded by the previously available relationships. Flame size quantification in forest crown fires is scarce, but a cursory inspection of the predictive ability of our Lf − If relationship in such cases is warranted. Flame height data is available for five active crown fires in jack pine stands in Canada (Stocks et al. 2004; Butler et al. 2004a), for which mean If varied between 39 896 and 78 533 kW m−1, calculated as previously described. Assuming that Lf equals flame height, i.e. inputting underestimates of Lf, our Lf − If equation estimates If with a mean absolute percentage error of 13.2% (range 1.8–35.2%), which is encouraging.
Beyond the already mentioned putative Sm effect, the supposed influence of fuel structure or other fuel bed-specific effects on fire behaviour properties does not preclude generic Lf − If relationships from producing useful estimates, especially for operational fire management purposes. For low-If fires, MAE represents a greater percentage of the observed If values. Thus, the present models are more appealing for use in high-If fires (>~2000 kW m−1), which typically occur under low fuel moisture content and strong winds (high R) in tall fuel complexes (high w). To obtain accurate If estimates for low-If fires, the use of fuel bed-specific Lf − If relationships may be a better option. Finally, different Lf − If relationships have been reported for backing and heading fires in the same fuel type (Clark 1983; Fernandes et al. 2009). The Lf of fires spreading under calm conditions theoretically scales with the 2/3 power of If (Fons et al. 1963; Thomas 1963; Albini 1981), which also applies to fires backing into the wind (Nelson 1980). Consequently, our Lf − If relationships should not be extrapolated to backing fires.
The Lf − If relationships can be applied for various purposes and in different ways. The reciprocals of Eqn 2 can be integrated in fire behaviour prediction schemes to produce estimates of Lf from If whenever the former is a variable of interest, e.g. in the frame of fire suppression and firebreak construction (Alexander 2000), or for fire hazard or fire risk assessment and mapping (e.g. Thompson et al. 2011). The usefulness of If estimates is thoroughly covered by Alexander and Cruz (2020). Estimation of If from Lf is expedient when the latter can be measured or calculated based on visual observation, either during or after the fire. If fire-spread rate is concurrently assessed, fine fuel consumption can be estimated as well (as per Eqn 1), without the need for destructive pre-burn and post-burn sampling, which is relevant for prescribed burning operations and fire effects studies. Estimates by personnel in the fireline can thus assist decision-making during fire control or fire use operations and can be used to anticipate fire effects such as crown scorch height and tree mortality. Likewise, post-fire surveys are able to translate fire effects in terms of the associated fireline intensity, which is useful for prescribed burning monitoring and wildfire study cases.
Comparison with Byram’s relationship
The pioneer Lf − If relationship of Byram (1959) is the best known and most widely used. Oddly, although Byram’s model is most commonly viewed as applicable to surface heading fires (Nelson 1980; Alexander and Cruz 2012), it was presumably based mostly on backing fires (34 out of a total of 41). Interestingly, it was derived from outdoor fires in a forest fuel type with a grass component, which might explain why it compares better with our grassland equation instead of that for forest–shrubland. Our results highlight the inadequacy of using the Byram (1959) model for forest and shrubland fires with Lf above ~2 m, consistent with the findings of recent laboratory experiments (Finney and Grumstrup 2023).
Rothermel (1991) stated that Byram’s equation severely underestimates the Lf of crown fires, and as such, recommended the more realistic equation of Thomas (1963), where Lf is proportional to If0.67, as in the equation of Butler et al. (2004b) for crown-fire Lf. The Lf − If relationship for the forest–shrubland variant displays b = 0.58, midway of the coefficients of Byram (b = 0.46) and Thomas. This may be an outcome of the substantial presence in the dataset of high-intensity observations in fuel complexes dominated by an elevated and aerated component, either in shrubland or in forest.
Study relevance
The discrepancies between previous results suggesting that generic Lf − If functions are unviable (Alexander and Cruz 2012) are most likely explained by the following reasons: (1) individual studies are usually limited in the number of fires and observed If range, which may lead to substantial differences in predicted values when models are extrapolated much beyond the development data range; (2) flame pulsation makes Lf evaluations subjective and originates discrepancies between measurements taken in real time or using video images (allowing for a visual average) and measurements based on photographs (capturing a single snapshot); and (3) most studies do not specify how the flame is measured and lack a standard method of measurement. We advocate that Lf should be assessed from the fuel base level to enable comparability between fires in different fuel heights and adequacy to heat transfer modelling (Nelson and Adkins 1986; Anderson et al. 2006), whereas Alexander (1982) proposes measuring Lf from the flame-depth midpoint at the fuel surface level to the tip of the flame.
If is a versatile fire metric that can be used for a wide variety of purposes; it can be estimated from Lf, and vice versa. Although Lf assessment is subjective and greatly depends on its definition and mode of observation and calculation, it is a readily apparent descriptor (Rothermel 1991) and therefore practical to use. Particular situations may benefit from the use of fuel bed-specific Lf − If relationships to assure increased accuracy of If estimates, e.g. for prescribed burning planning purposes for which the most accurate relationships between fire behaviour and fire effects are required (Fernandes et al. 2012; Hiers et al. 2020). However, deriving specific models for all existing fuel complexes is not feasible. As a consequence, the generic relationships developed in the present study will be of interest in many situations. Moreover, our results are based on a great amount of data from very diverse literature sources reporting field fires conducted worldwide – in a wide range of fuel species, vegetation structure, wind speed, slope angle and flame dimensions, and thus are robust.
Conclusions
We found that the generic description of If from Lf should be based on different functions for forest–shrubland and for grassland fires, and we speculate that Sm is the main fuel metric influencing flame structure. Mean Lf must be assessed from the base of the fuel bed for comparability between fires burning in fuel beds with different depths. The absence of standards to assess Lf, and the fact that many studies do not specify if the measure is taken from the base or the top of the fuel bed, has likely contributed to some of the discrepancies among previous results, which suggested fuel-specific relationships are unfeasible. Because If is a frequently used fire metric and developing specific models for all existing fuel complexes is not practical, the generic relationships presented in this work will be of interest for both research and management purposes, namely in higher-intensity surface fires.
List of symbols, units and definitions
a and b | fitted coefficients |
h (m) | fuel bed height |
H (kJ kg−1) | heat yield per unit mass of fuel |
If (kW m−1) | fireline intensity |
Lf (m) | average flame length (measured from the base of the fuel bed, unless otherwise specified) |
R (m s−1) | fire spread rate |
Sm (m2 kg−1) | surface area-to-mass ratio of the fuel particles |
w (kg m−2) | fuel load consumed by flaming combustion (approximated to fine fuel load) |
ρb (kg m−3) | fuel bed density |
Conflicts of interest
Paulo Fernandes is an Associate Editor of the International Journal of Wildland Fire but was blinded from the review process to mitigate the potential conflict of interest.
Declaration of funding
The study was supported by FEDER – Fundo Europeu de Desenvolvimento Regional (funds from COMPETE 2020 – POCI) and by Portuguese funds through FCT- Portuguese Foundation for Science and Technology, project POCI-01-0145-FEDER-016727 (PTDC/AAG-MAA/2656/2014) and project UIDB/04033/2020 (https://doi.org/10.54499/UIDB/04033/2020).
Acknowledgements
The authors thank Jim Gould and Miguel Cruz from the CSIRO Bushfire Behaviour and Suppression Team for supplying the Project VESTA data.
References
Albini FA (1981) A model for the wind-blown flame from a line fire. Combustion and Flame 43, 155-174.
| Crossref | Google Scholar |
Alexander ME (1982) Calculating and interpreting forest fire intensities. Canadian Journal of Botany 60, 349-357.
| Crossref | Google Scholar |
Alexander ME (2000) ‘Fire behaviour as a factor in forest and rural fire suppression’. Forest Research, Rotorua, in association with the National Rural Fire Authority, Wellington. Forest Research Bulletin No. 197, Forest and Rural Fire Scientific and Technical Series, Report No. 5. (Forest Research Institute, New Zealand Forest Service.)
Alexander ME, Cruz MG (2012) Interdependencies between flame length and fireline intensity in predicting crown fire initiation and crown scorch height. International Journal of Wildland Fire 21, 95-113.
| Crossref | Google Scholar |
Anderson W, Pastor E, Butler B, Catchpole E, Dupuy JL, Fernandes PM, Guijarro M, Mendes-Lopes JM (2006) Evaluating models to estimate flame characteristics for free-burning fires using laboratory and field data. In ‘Proceedings of 5th International Conference on Forest Fire Research’, Figueira da Foz, Portugal, 27–30 November 2006. (Ed. DX Viegas) (Elsevier BV: Amsterdam, The Netherlands)
Anderson WR, Cruz MG, Fernandes PM, McCaw L, Vega JA, Bradstock RA, Fogarty L, Gould J, McCarthy G, Marsden-Smedley JB, Matthews S, Mattingley G, Pearce HG, van Wilgen BW (2015) A generic, empirical-based model for predicting rate of fire spread in shrublands. International Journal of Wildland Fire 24, 443-460.
| Crossref | Google Scholar |
Botelho H, Vega JA, Fernandes PM, Rego FC (1994) Prescribed fire behavior and fine fuel consumption in Northern Portugal and Galiza maritime pine stands. In ‘Proceedings of the Second International Conference on Forest Fire Research. Vol. 1’, 21–24 November 1994. pp. 343–353. (Universidade de Coimbra)
Bova AS, Dickinson MB (2008) Beyond ‘fire temperatures’: calibrating thermocouple probes and modeling their response to surface fires in hardwood fuels. Canadian Journal of Forest Research 38, 1008-1020.
| Crossref | Google Scholar |
Butler BW, Cohen J, Latham DJ, Schuette RD, Sopko P, Shannon KS, Jimenez D, Bradshaw LS (2004a) Measurements of radiant emissive power and temperatures in crown fires. Canadian Journal of Forest Research 34, 1577-1587.
| Crossref | Google Scholar |
Butler BW, Finney MA, Andrews PL, Albini FA (2004b) A radiation-driven model of crown fire spread. Canadian Journal of Forest Research 34, 1588-1599.
| Crossref | Google Scholar |
Byram GM, Nelson RM (1970) The modeling of pulsating fires. Fire Technology 6, 102-110.
| Crossref | Google Scholar |
Catchpole WR, Bradstock RA, Choate J, Fogarty LG, Gellie N, McCarthy G, McCaw WL, Marsden-Smedley JB, Pearce G (1998) Cooperative development of equations for heathland fire behaviour. In ‘Proceedings of 3rd International Conference on Forest Fire Research and 14th Conference on Fire and Forest Meteorology. Vol. II’, 16–20 November 1998, Luso–Coimbra, Portugal. (Ed. DX Viegas) pp. 631–645. (University of Coimbra: Coimbra, Portugal)
Cheney NP (1990) Quantifying bushfires. Mathematical and Computer Modelling 13, 9-15.
| Crossref | Google Scholar |
Cheney NP, Gould JS, McCaw WL, Anderson WR (2012) Predicting fire behaviour in dry eucalypt forest in southern Australia. Forest Ecology and Management 280, 120-131.
| Crossref | Google Scholar |
Cruz MG, Sullivan AL, Gould JS, Hurley RJ, Plucinski MP (2018) Got to burn to learn: the effect of fuel load on grassland fire behaviour and its management implications. International Journal of Wildland Fire 27, 727-741.
| Crossref | Google Scholar |
Davies GM, Legg CJ (2011) Fuel Moisture Thresholds in the Flammability of Calluna vulgaris. Fire Technology 47, 421-436.
| Crossref | Google Scholar |
Dupuy JL, Maréchal J, Portier D, Valette JC (2011) The effects of slope and fuel bed width on laboratory fire behaviour. International Journal of Wildland Fire 20, 272-288.
| Crossref | Google Scholar |
Fernandes PAM (2001) Fire spread prediction in shrub fuels in Portugal. Forest Ecology and Management 144, 67-74.
| Crossref | Google Scholar |
Fernandes PM, Catchpole WR, Rego FC (2000) Shrubland fire behaviour modelling with microplot data. Canadian Journal of Forest Research 30, 889-899.
| Crossref | Google Scholar |
Fernandes PAM, Loureiro CA, Botelho S (2004) Fire behaviour and severity in a maritime pine stand under differing fuel conditions. Annals of Forest Science 61, 537-544.
| Crossref | Google Scholar |
Fernandes PM, Botelho HS, Rego FC, Loureiro C (2009) Empirical modelling of surface fire behaviour in maritime pine stands. International Journal of Wildland Fire 18, 698-710.
| Crossref | Google Scholar |
Fernandes PM, Loureiro C, Botelho H (2012) PiroPinus: a spreadsheet application to guide prescribed burning operations in maritime pine forest. Computers and Electronics in Agriculture 81, 58-61.
| Crossref | Google Scholar |
Fernandes PM, Sil A, Rossa CG, Ascoli D, Cruz MG, Alexander ME (2020) Characterizing Fire Behavior Across the Globe. In: ‘The Fire Continuum—Preparing for the Future of Wildland Fire: Proceedings of the Fire Continuum Conference’, 21–24 May 2018, Missoula, MT. (Tech. Eds S Hood, S Drury, T Steelman, R Steffens) Proc. RMRS-P-78. pp. 258–263. (Fort Collins, CO: U.S. Department of Agriculture, Forest Service, Rocky Mountain Research Station)
Finney MA, Grumstrup TP (2023) Effect of flame zone depth on the correlation of flame length with fireline intensity. International Journal of Wildland Fire 32, 1135-1147.
| Crossref | Google Scholar |
Fons WL, Clements HB, George PM (1963) Scale effects on propagation rate of laboratory crib fires. Symposium (International) on Combustion 9, 860-866.
| Crossref | Google Scholar |
Hély C, Alleaume S, Swap RJ, Shugart HH, Justice CO (2003) SAFARI-2000 characterization of fuels, fire behavior, combustion completeness, and emissions from experimental burns in infertile grass savannas in western Zambia. Journal of Arid Environments 54, 381-394.
| Crossref | Google Scholar |
Hiers JK, O’Brien JJ, Varner JM, Butler BW, Dickinson M, Furman J, Gallagher M, Godwin D, Goodrick SL, Hood SM, Hudak A, Kobziar LN, Linn R, Loudermilk EL, McCaffrey S, Robertson K, Rowell EM, Skowronski N, Watts AC, Yedinak KM (2020) Prescribed fire science: the case for a refined research agenda. Fire Ecology 16, 11.
| Crossref | Google Scholar |
Hirsch KG, Martell DL (1996) A review of initial attack fire crew productivity and effectiveness. International Journal of Wildland Fire 6, 199-215.
| Crossref | Google Scholar |
Johnson VJ (1982) The dilemma of flame length and intensity. Fire Management Notes 43, 3-7.
| Google Scholar |
Kucuk O, Bilgili E, Saglam B, Baskaya S, Dinc Durmaz B (2008) Some parameters affecting fire behavior in anatolian black pine slash. Turkish Journal of Agriculture and Forestry 32, 121-129.
| Crossref | Google Scholar |
Kunst C, Bravo S, Moscovich F, Herrera J, Godoy J, Velez S (2001) Control de tusca (Acacia aroma Gill ap. H. et A.) mediante fuego prescripto. Revista Argentina de Producción Animal 20, 199-213 [In Spanish].
| Google Scholar |
Marsden-Smedley J, Catchpole W (1995) Fire Behaviour Modelling in Tasmanian Buttongrass Moorlands: II. Fire Behaviour. International Journal of Wildland Fire 5, 215-228.
| Crossref | Google Scholar |
Nelson Jr RM, Adkins CW (1986) Flame characteristics of wind-driven surface fires. Canadian Journal of Forest Research 16, 1293-1300.
| Crossref | Google Scholar |
Nelson Jr RM, Adkins CW (1988) A dimensionless correlation for the spread of wind-driven fires. Canadian Journal of Forest Research 18, 391-397.
| Crossref | Google Scholar |
Patterson III WA, Clarke G, Haggerty S, Sievert P, Kelty M (2005) Wildland Fuel Management Options for the Central Plains of Martha’s Vineyard: Impacts on Fuel Loads, Fire Behavior and Rare Plant and Insect Species. pp. 1–140. (Department of Natural Resources Conservation, University of Massachusetts: Amherst)
Pinto A, Fernandes PM, Espinosa-Prieto J (2013) FIREglobulus: Estudo Experimental do Comportamento e Efeitos do Fogo em Eucaliptal. Silva Lusitana 21, 143-155 [In Portuguese].
| Google Scholar |
Rossa CG, Fernandes PM (2018a) An empirical model for the effect of wind on fire spread rate. Fire 1, 31.
| Crossref | Google Scholar |
Rossa CG, Fernandes PM (2018b) Empirical modelling of fire spread rate in no-wind and no-slope conditions. Forest Science 64, 358-370.
| Crossref | Google Scholar |
Sneeuwjagt RJ, Frandsen WH (1977) Behavior of experimental grass fires vs. predictions based on Rothermel’s fire model. Canadian Journal of Forest Research 7, 357-367.
| Crossref | Google Scholar |
Snowdon P (1991) A ratio estimator for bias correction in logarithmic regressions. Canadian Journal of Forest Research 21, 720-724.
| Crossref | Google Scholar |
Sparks AM, Smith AMS, Talhelm AF, Kolden CA, Yedinak KM, Johnson DM (2017) Impacts of fire radiative flux on mature Pinus ponderosa growth and vulnerability to secondary mortality agents. International Journal of Wildland Fire 26, 95-106.
| Crossref | Google Scholar |
Stocks BJ, Alexander ME, Wotton BM, Stefner CN, Flannigan MD, Taylor SW, Lavoie N, Mason JA, Hartley GR, Maffey ME, Dalrymple GN, Blake TW, Cruz MG, Lanoville RA (2004) Crown fire behaviour in a northern jack pine–black spruce forest. Canadian Journal of Forest Research 34, 1548-1560.
| Crossref | Google Scholar |
Susott RA (1982) Characterization of the thermal properties of forest fuels by combustible gas analysis. Forest Science 28, 404-420.
| Crossref | Google Scholar |
Thompson MP, Calkin DE, Finney MA, Ager AA, Gilbertson-Day JW (2011) Integrated national-scale assessment of wildfire risk to human and ecological values. Stochastic Environmental Research and Risk Assessment 25, 761-780.
| Crossref | Google Scholar |
Van Wilgen BW (1986) A simple relationship for estimating the intensity of fires in natural vegetation. South African Journal of Botany 52, 384-385.
| Crossref | Google Scholar |
Van Wilgen B, Wills AJ (1988) Fire behaviour prediction in savanna vegetation. South African Journal of Wildlife Research 18, 41-46.
| Google Scholar |
Van Wilgen BW, Le Maitre DC, Kruger FJ (1985) Fire Behaviour in South African Fynbos (Macchia) Vegetation and Predictions from Rothermel’s Fire Model. Journal of Applied Ecology 22, 207-216.
| Crossref | Google Scholar |
Vega JA, Cuinas P, Fonturbel T, Perez-Gorostiaga P, Fernandez C (1998) Predicting fire behaviour in Galician (NW Spain) shrubland fuel complexes. In ‘Proceedings of 3rd International Conference on Forest Fire Research and 14th Conference on Fire and Forest Meteorology. Vol. II’, 16–20 November 1998, Luso–Coimbra, Portugal. (Ed. DX Viegas) pp. 713–728. (University of Coimbra: Coimbra, Portugal)
Weber MG, Hummel M, Van Wagner CE (1987) Selected parameters of fire behavior and Pinus banksiana Lamb. regeneration in eastern Ontario. Forestry Chronicle 63, 340-346.
| Crossref | Google Scholar |
Weise DR, Biging GS (1996) Effects of wind velocity and slope on flame properties. Canadian Journal of Forest Research 26, 1849-1858.
| Crossref | Google Scholar |